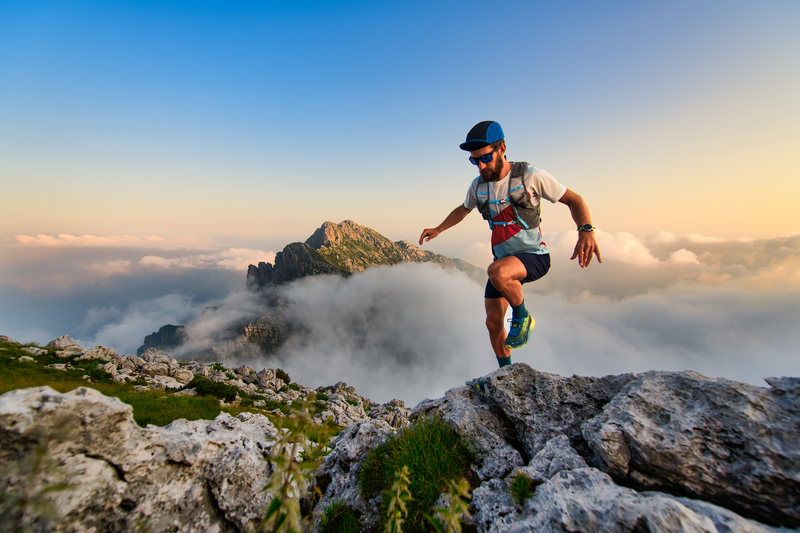
94% of researchers rate our articles as excellent or good
Learn more about the work of our research integrity team to safeguard the quality of each article we publish.
Find out more
ORIGINAL RESEARCH article
Front. Endocrinol. , 22 July 2022
Sec. Systems Endocrinology
Volume 13 - 2022 | https://doi.org/10.3389/fendo.2022.950030
This article is part of the Research Topic Genetic Variants and Metabolic Diseases View all 10 articles
Background: Osteoporosis and atherosclerosis are common in the elderly population, conferring a heavy worldwide burden. Evidence links osteoporosis and atherosclerosis but the exact underlying common mechanism of its occurrence is unclear. The purpose of this study is to further explore the molecular mechanism between osteoporosis and atherosclerosis through integrated bioinformatic analysis.
Methods: The microarray data of osteoporosis and atherosclerosis in the Gene Expression Omnibus (GEO) database were downloaded. The Weighted Gene Co-Expression Network Analysis (WGCNA) and differentially expressed genes (DEGs) analysis were used to identify the co-expression genes related to osteoporosis and atherosclerosis. In addition, the common gene targets of osteoporosis and atherosclerosis were analyzed and screened through three public databases (CTD, DISEASES, and GeneCards). Gene Ontology (GO) and Kyoto Encyclopedia of Genes and Genomes (KEGG) enrichment analyses were performed by Metascape. Then, the common microRNAs (miRNAs) in osteoporosis and atherosclerosis were screened out from the Human microRNA Disease Database (HMDD) and the target genes of whom were predicted through the miRTarbase. Finally, the common miRNAs–genes network was constructed by Cytoscape software.
Results: The results of common genes analysis showed that immune and inflammatory response may be a common feature in the pathophysiology of osteoporosis and atherosclerosis. Six hub genes (namely, COL1A1, IBSP, CTSD, RAC2, MAF, and THBS1) were obtained via taking interaction of different analysis results. The miRNAs–genes network showed that has-let-7g might play an important role in the common mechanisms between osteoporosis and atherosclerosis.
Conclusion: This study provides new sights into shared molecular mechanisms between osteoporosis and atherosclerosis. These common pathways and hub genes may offer promising clues for further experimental studies.
More and more pieces of evidence show that vascular system diseases are related to bone metabolism. Some scholars presented a concept of a bone-vascular axis to explore the correlation between them (1, 2). They supported that there exited cellular, endocrine, and metabolic signals that flow bidirectionally between the vasculature and bone, and vascular and skeletal disease may occur concurrently when dysmetabolic states perturbed the bone–vascular axis (2). Osteoporosis and atherosclerosis are the most common skeletal and vascular disease while also being the chronic systemic diseases and major public health problems worldwide (3). Epidemiology studies have linked osteoporosis and atherosclerosis, suggesting that postmenopausal women with osteoporosis are often accompanied by atherosclerosis (4). Decreased bone mineral density and osteoporotic fractures were significantly associated with the development of echogenic plaques in carotid artery (5). Regardless of no widely consensus on the exact cellular and molecular basis underlying the high comorbidity between osteoporosis and atherosclerosis, it has been testified that they shared risk factors, common pathogenesis and genetic factors, and a causal association (6).
Several risk factors involving ageing, postmenopausal status, smoking habit, physical inactivity, and alcohol intake were considered as the common factors shared by osteoporosis and atherosclerosis (7). Osteoblast activity and survival decline with aging, whereas osteoclast activity increases, contributing to the age-associated decline of bone mass (8). Simultaneously, atherosclerosis, a high prevalence and incidence disease in the elderly causing most heart attacks and strokes, is considered a hallmark of aging process (9). However, there is growing evidence that a potential link exists between osteoporosis and atherosclerosis beyond aging (10). Several pathophysiological mechanisms including inflammatory cytokines, lipid oxidation products, and vitamin D and K deficiency were identified for the interplay between the skeletal and vascular systems (7, 11). Inflammatory cytokines [including tumor necrosis factor–alpha (TNF-α) and interleukin (IL)-1, IL-6, and IL-17] and chemokines have been shown to be associated with atherosclerosis, increased cardiovascular morbidity and mortality, and increased bone loss (12, 13). Dyslipidemia, including elevated level of total serum cholesterol, triglycerides, and low-density lipoprotein (LDL) cholesterol, was considered to promote atherosclerosis progression and also influenced bone metabolism (14). In addition, there also exists similarity of the treatment for osteoporosis and atherosclerosis. Statins, cholesterol-lowering drugs in preventing and treating cardiovascular disease, have potential positive effects on bone mineral density and decreasing osteoporotic fracture (15). Bisphosphonate therapy for osteoporosis reduces progression of vascular calcification (16). These findings strongly suggested the interaction of these two pathological conditions, simultaneously exerting an influence on the development of osteoporosis and atherosclerosis. However, they were mainly from clinical perspectives, and few studies have investigated genomic relationship between osteoporosis and atherosclerosis.
Contemporary, the quick development of bioinformatics approaches allows us to get a better grasp of disease pathobiology more deeply from the genetic level (17). Recently, a system-level analysis of whole blood genome−wide expression data identified several enriched biological pathways and three genes (NOSIP, GXYLT2, and TRIM63) that were significantly associated with early traits of both osteoporosis and atherosclerosis, supporting the idea that they were comorbid based on transcriptomic evidence (18). However, the representativeness of their samples was limited, and studies integrating gene data from public databases with both osteoporosis and atherosclerosis are lacking. In view of this, the purpose of this study is to integrate and analyze gene data related to the pathogenesis of osteoporosis complicated with atherosclerosis from the public databases, which provide new insights into the biological mechanisms of these two diseases, and it will help to develop dual-purpose prevention methods. The research flowchart of this research was shown in Figure 1.
Microarray datasets were downloaded from the Gene Expression Omnibus (GEO) database (http://www.ncbi.nlm.nih.gov/geo/), which contain a great deal of high-throughput sequencing and expression microarray data. The keywords “osteoporosis” and “atherosclerosis” were used to search related gene expression datasets and non-human tested specimens were excluded. Finally, the datasets numbered GSE22829, GSE56815, GSE35958, GSE100927, GSE43292, and GSE35956 were downloaded from GEO database. In addition, the co-expressed genes of osteoporosis and atherosclerosis were screened through three disease database, including Comparative Toxicogenomics Database (CTD) (http://ctdbase.org/) (19), GeneCards (https://www.genecards.org/) (20), and DISEASES (https://diseases.jensenlab.org/) database (21).
A system biological approach called Weighted Gene Co-Expression Network Analysis (WGCNA) analysis co-expressed gene modules that have high biological significance and explores the relation between gene networks and diseases (22). Therefore, the WGCNA was used to analyze GSE56815 dataset and GSE22829 dataset to obtain the osteoporosis and atherosclerosis associated modules. Using R language, the Hclust function was used prior to the analysis of eliminating outlier samples from the hierarchical clustering analysis. Based on the criterion of R2 > 0.85, an appropriate soft-thresholding power β (ranged from 1 to 20) is calculated to achieve the scale-free topology. Then, through hierarchical clustering, co-expression modules were identified, and the hierarchical clustering tree was obtained. The minimum number of module genes was set as 25, and the modules were merged for the second time according to the modules with a correlation greater than 50%. Finally, the module eigengene and the correlation between the module eigengene and clinical features were calculated to obtain the expression profiles of each module. As a result, we focused on modules with high correlation coefficients with clinical features and later selected genes from these modules for further analysis.
Using the Pearson correlation coefficient and the P-value of eigengenes and disease traits of each module, we identified key modules in osteoporosis and atherosclerosis. Then, the genes in key modules positively associated with osteoporosis and atherosclerosis were used to obtain shared genes via ImageGP (23).
GEO2R (www.ncbi.nlm.nih.gov/geo/ge2r) is an online analysis tool developed based on two R packages (GEO query and Limma) (24). The differentially expressed genes (DEGs) in GSE35958 and GSE100927 datasets were determined by comparing gene expression profiles between the diseased and control groups using GEO2R. Log|FC| > 1 and adj. P-value < 0.05 were considered to indicate statistical significance. The common DEGs were obtained by Venn diagram.
In order to analyze the biological functions and pathways involved in common genes, Gene Ontology (GO) and Kyoto Encyclopedia of Genes and Genomes (KEGG) enrichment analyses were performed using Metascape, which is a web-based portal designed to provide a comprehensive gene list annotation and analysis resource for experimental biologists (25). Min overlap = 3 and Min Enrichment = 1.5 were the screening conditions. The P-value < 0.01 was considered significant.
The protein–protein interaction (PPI) network was analyzed using the Search Tool for the Retrieval of Interacting Genes (STRING; http://string-db.org). An interaction with a combined score > 0.4 was selected and used to construct a PPI network with Cytoscape software (version 3.7.0). The cluster analysis used Cytoscape’s plug-in molecular complex detection technology (MCODE) with default parameters: K-core = 2, degree cutoff = 2, max depth = 100, and node score cutoff = 0.2.
The common osteoporosis-related genes and the atherosclerosis-related genes that were shared between the three public databases were obtained using the Venn diagram. Then, taking the intersection of them, we obtained the common gene targets between osteoporosis and atherosclerosis.
Common gene targets between osteoporosis and atherosclerosis were obtained through disease database screening. At the same time, shared genes were also gained from WGCNA and DEG analysis. Then, the intersection of common targets from disease databases and genes from WGCNA and DEG analysis was selected as hub genes. Furthermore, the expression of hub genes was verified in GSE43292 and GSE35956. The comparison between the two sets of data was performed with the t-test. P-value < 0.05 was considered significant.
In order to explore the relationship between hub genes and diseases, CTD was used to isolate the inference score and reference count of hub genes associated with osteoporosis and atherosclerosis. Interaction between hub genes and atherosclerosis, cardiovascular diseases, vascular diseases, osteoporosis, bone diseases, and bone resorption was analyzed in CTD. The inference score and reference count were visualized by the Histogram.
Small non-coding RNAs called microRNAs can modulate gene expression by promoting or inhibiting mRNA degradation and translation (26). We therefore investigate whether some miRNAs share a common regulatory mechanism and development process in osteoporosis and atherosclerosis. Osteoporosis-associated and atherosclerosis-associated miRNAs were obtained from the Human microRNA Disease Database (HMDD), which presents more detailed and comprehensive annotations to the human miRNA-disease association data, including miRNA-disease association data from the evidence of genetics, epigenetics, circulating miRNAs, and miRNA-target interactions (27). Based on a published literature, we further identified the expression levels of these miRNAs in osteoporosis and atherosclerosis, and only miRNAs with the same disorder types were further analyzed. In particular, GO analysis of these common miRNAs was performed using the online software mirPath (v.3) from DIANA tools. The GO terms with P-values < 0.01 were considered significant.
Target gene information of common miRNAs was collected from miRTarbase (http://mirtarbase.mbc.nctu.edu.tw/php/index.php), which is an experimentally validated miRNA-target interactions database (28). The intersection of target genes of common miRNAs and shared genes in osteoporosis and atherosclerosis was used to construct the miRNAs–genes regulated network. Cytoscape software was used to visualize the network.
Six GEO datasets (namely, GSE56815, GSE28829, GSE100927, GSE35958, GSE43292, and GSE35956) were selected in all. Detailed information of these six datasets is shown in Table 1, such as GSE number, detection platforms, and samples. GSE56815 and GSE28829 were paired used for the WGCNA analysis, GSE35958 and GSE100927 were paired used for the DEG analysis, and GSE35956 and GSE43292 were used to verify the hub gene expression levels.
As shown in Figures 2A–C, gene modules associated with the bone mineral density phenotype were obtained by WGCNA analysis of GSE56815 dataset, including circulating monocytes from females with 40 high bone mineral density and 40 low bone mineral density. Clustering analysis of GSE56815 showed that the GSM1369791 sample was poorly clustered (Supplementary Figure 1A, B). Therefore, this sample was excluded as an outlier in the WGCNA analysis. The analysis of soft threshold selection revealed that gene associations were maximally consistent with the scale-free distribution and when β = 7 (scale free R2 = 0:85). Then, a total of eight modules were identified in the weighted gene co-expression network by merging modules with feature factors greater than 0.5 and setting the minimum number of genes in a module to 25. We found that, as shown in Figure 2C, MEbrown module (r = 0.462, p = 0.0031) was positively correlated with osteoporosis in non-gray modules. Genes in the MEbrown module were further used to the analysis, including 257 genes.
Figure 2 Weighted genes correlation network analysis (WGCNA) of GSE56815 and GSE28829 datasets. (A) Soft threshold analysis in osteoporosis. (B) Module correlations in osteoporosis. (C) Heatmap of the module–trait relationship in osteoporosis. Each cell contains the corresponding correlation and P-value. (D) Soft threshold analysis in atherosclerosis. (E) Module correlations in atherosclerosis. (F) Heatmap of the module–trait relationship in atherosclerosis. Each cell contains the corresponding correlation and P-value. OP, osteoporosis; CON, control; AAS, advanced atherosclerosis; EAS, early atherosclerosis.
As shown in Figures 2D–F, a total of 12 gene modules were obtained through the WGCNA analysis of GSE28829 dataset, including 16 advanced atherosclerotic segments and 13 early atherosclerotic segments from human. Clustering analysis showed that no sample was excluded as an outlier in the WGCNA analysis (Supplementary Figures 1C, D). Similarly, a heatmap was mapped about module–trait relationships according to the Spearman’s correlation coefficient to evaluate the association between each module and the disease. Among these 12 modules, the correlations of two modules “MEturquoise” and “MEpink” was high and they were positively correlated with atherosclerosis (MEturquoise module: r = 0.755, p = 3.4e-06; MEpink modules: r = 0.659, p = 0.00014), including 446 and 69 genes, respectively (Figure 2F).
The common genes were screened between atherosclerosis positively related modules (MEturquoise and MEpink modules) and osteoporosis positively related modules (MEbrown module). Then, 44 common genes were identified in the three positivity related modules of osteoporosis and atherosclerosis (Figure 3A). PPI network was further constructed by Cytoscape (Figure 3B). To explore the potential functions of these genes, GO and KEGG enrichment analyses were performed using Metascape. Results showed that these common genes were mainly enriched in leishmaniasis, immune receptor activity, inflammatory response, tertiary granule, and regulation of leukocyte activation, which indicated that most of them were involved in immune- and inflammatory-related functions (Figure 3C).
Figure 3 Analysis of shared genes through WGCNA. (A) The shared genes between the MEpink and MEturquoise modules of atherosclerosis and MEbrown module of osteoporosis by overlapping them. (B) The PPI network of the shared genes. (C) GO and KEGG enrichment analysis of the shared genes. OP, osteoporosis; AS, atherosclerosis.
In order to investigate the possible pathogenesis of osteoporosis and atherosclerosis, enrichment analysis of genes in their positive modules was performed. Function enrichment analysis showed that the genes in osteoporosis MEbrown module were mainly associated with immune and inflammatory response. Interestingly, we also found that they were also mainly enriched in lipid and atherosclerosis pathway, which is closely associated with the development and progression of atherosclerosis. Therefore, it may hint that a close link exists between osteoporosis and atherosclerosis at the molecular level (Figure 4A).
Figure 4 Enrichment analysis of positive related modules in osteoporosis and atherosclerosis. (A) GO and KEGG enrichment analysis of MEbrown module in osteoporosis. (B) GO and KEGG enrichment analysis of MEturquoise module in atherosclerosis. (C) GO and KEGG enrichment analysis of MEpink module in atherosclerosis. OP, osteoporosis; AS, atherosclerosis.
The MEturquoise and MEpink modules were closely associated with atherosclerosis, and the genes shared with osteoporosis were mainly located in the MEturquoise module. As shown in the Figures 4B, C, the turquoise and pink modules were also mainly related to inflammatory response, immune effector process, and leukocyte activation. The results described above indicated that immune and inflammatory response may play important roles in both osteoporosis and atherosclerosis and be major contributors to atherosclerosis complicted with osteoporosis.
GSE35958 and GSE100927 datasets were used to DEG analysis. The GSE35958 dataset contains four osteoporosis mesenchymal stem cell samples and five non-osteoporosis mesenchymal stem cell samples from human bone marrow. GSE100927 consists of 69 atherosclerotic plaque samples and 35 control artery samples from human peripheral arteries. After analysis of GEO2R, a total of 575 and 2,619 DEGs were identified in GSE100927 and GSE35958, respectively. The overall distribution of DEGs (fold change > 1 and adj. P-value < 0.05) was reflected by the volcanic map (Supplementary Figure 2). After taking the intersection of the Venn diagram, 40 common upregulated DEGs in both datasets were obtained (Figure 5A). PPI network showed that TNF, ITGB2, CTSD, and LAPTM5 had high degree (Figure 5B). Function enrichment analysis indicated that these common DEGs were enriched in regulation of cell adhesion, lysosomal lumen, specific granule, and inflammatory response (Figure 5C). Interestingly, we found that immune and inflammatory response–related functions were significantly enriched again, which were consistent with the results of WGCNA analysis.
Figure 5 Analysis of common DEGs between osteoporosis and atherosclerosis. (A) The Venn diagram of the common DEGs in GSE35958 and GSE100927. (B) The PPI network of the common DEGs. (C) GO and KEGG enrichment analysis of the common DEGs.
In order to integrate the reported biological data, we combined osteoporosis- and atherosclerosis-related genes available in CTD, DISEASES, and GeneCards databases by Venn diagram software, respectively. Then, 315 genes related to osteoporosis and 481 genes to atherosclerosis were selected, and 202 common gene targets between osteoporosis and atherosclerosis were mapped, which hints that osteoporosis and atherosclerosis share a large common set of genes (Figures 6A–C). The list of 202 common gene targets of osteoporosis and atherosclerosis was showed in Supplementary Table 1.
Figure 6 Analysis of common targets between osteoporosis and atherosclerosis from three public databases. (A–C) Venn diagram of common targets between atherosclerosis and osteoporosis. (D) KEGG enrichment analysis of the common targets. (E) GO enrichment analysis of the common targets.
KEGG enrichment analysis of these 202 common genes revealed that most of the shared genes related pathways are linked to lipid and atherosclerosis, PI3K-Akt signaling pathway, and some immune and inflammation-related pathways, including cytokine–cytokine receptor interaction, IL-17 signaling pathway, and T-cell differentiation, which were consistent with our enrichment analysis results above. Figure 6D contains the top 15 results and Supplementary Table 2 contains the top 20 signalling pathways. Figure 6E contains the top five results of three GO enrichment analysis, including biological process (BP), cellular component (CC), and molecular function (MF). Supplementary Table 3 contains the top 20 results of BP analysis results.
The PPI network of the common targets was constructed with combined scores greater than 0.4 using Cytoscape, which contained 200 nodes and 1,697 edges (Figure 7A). Three closely connected gene modules were obtained through MCODE plug-in of Cytoscape (Figures 7B–D). Enrichment analysis results of these three clusters showed that the immune and inflammatory response–related functions were enriched again.
Figure 7 The PPI network and clusters analysis of common targets. (A) PPI network of 202 common targets. (B–D) Three significant gene clustering modules and enrichment analysis of the modular genes.
After taking the intersection of three sets, six hub genes (COL1A1, IBSP, CTSD, RAC2, MAF, and THBS1) were identified (Figure 8). In order to verify the reliability of these hub gene expression levels, we then selected GSE35956 dataset to analyze the expression levels of these hub genes in osteoporosis, and GSE43292 dataset to analyze the expression levels of hub genes in atherosclerosis. Interestingly, all hub genes were significantly upregulated in both the osteoporosis and atherosclerosis group compared with the control group (Figures 9A, B).
Figure 9 Verification of hub genes. (A) The expression level of hub genes in GSE35956. (B) The expression level of hub genes in GSE43292. The comparison between the two sets of data uses the mean t-test; P-value < 0.05 was considered statistically significant. *p < 0.05; **p < 0.01; ***p < 0.001. Inference score (C) and reference count (D) between hub genes and atherosclerosis, cardiovascular diseases, vascular diseases, osteoporosis, bone diseases, and bone resorption in CTD.
In addition, we find that these six hub genes were associated with not only osteoporosis but also atherosclerosis with different inference scores and reference counts in CTD (Table 2). The information of interaction between hub genes and diseases was shown in Figures 9C, D.
A total of 119 miRNAs associated with atherosclerosis and 32 miRNAs associated with osteoporosis were screened out from the HMDD database (Supplementary Data 1). After taking the intersection of them, 17 common miRNAs between osteoporosis and atherosclerosis were obtained. According to the published literature provided by the HMDD database, we obtained the disorder types of these common miRNAs; there were six miRNAs (hsa-miR-133b, hsa-miR-205-5p, hsa-miR-21-3p, hsa-miR-320a, hsa-miR-23b-3p, and hsa-miR-181a-5p) upregulated and two miRNAs (hsa-miR-150-5p and hsa-let-7g-5p) downregulated in both osteoporosis and atherosclerosis. Then, the eight miRNAs were further studied. The enrichment analysis showed that the functions of these miRNAs were involved in multiple BPs including immune response related functions (Supplementary Figure 3).
A total of 5,015 target genes of eight common miRNAs were predicted using miRTarbase, and the miRNAs–genes network was constructed by taking the intersection of them and shared genes (obtained from WGCNA and DEGs). Finally, the miRNAs–genes network contained seven miRNAs and 23 shared genes, including three hub genes (CTSD, COL1A1, and THBS1) (Figure 10). It is evident from the network that hsa-let-7g-5p regulated the most downstream target genes. Moreover, target genes predicted by has-let-7g were closely related to the inflammatory and immune response using the online software mirPath (v.3) from DIANA tools. Therefore, we speculate that has-let-7g might function importantly in the common mechanism of osteoporosis and atherosclerosis.
Figure 10 miRNAs–shared genes regulatory network. The V-shape represents miRNA, ellipse shape represents gene, and triangle shape represents hub gene. Blue represents gene from WGCNA and green represent gene from DEGs.
Osteoporosis and atherosclerosis are both widely prevalent disorders and often seen concurrently, exerting a severe impact on public health (29). The common pathophysiological mechanisms between osteoporosis and atherosclerosis have been attracting intense research interest around the world. Previous studies identified some molecular lipid species associated with early markers of both osteoporosis and atherosclerosis based on the Young Finns Study cohort, supporting the osteoporosis and atherosclerosis comorbidity hypothesis (14, 30). Latest research found that platelet-derived growth factor–BB (PDGF-BB), secreted from preosteoclasts, worked as an important mediator of vascular stiffening in response to aging and metabolic stress (31). Extracellular vesicles derived from aged bone matrix during bone resorption promote bone marrow mesenchymal stem cells adipogenesis rather than osteogenesis and augment calcification of vascular smooth muscle cells (32). These results have deepened our knowledge of the concept of a bone-vascular axis and may help to reveal the molecular mechanism between osteoporosis and atherosclerosis. However, it seems that few studies have explore the common pathogenesis of osteoporosis and atherosclerosis on a genetic level. To our knowledge, this is the first time to investigate osteoporosis and atherosclerosis comorbidity hypothesis by integrating data from a variety of public databases to identify the common mechanisms of osteoporosis and atherosclerosis.
Global gene expression studies can help us better understand the specific pathobiology between osteoporosis and atherosclerosis. The results of WGCNA and DEG analysis showed that immune and inflammatory response–related functions may play an important role in both osteoporosis and atherosclerosis. At the same time, we found that T-cell differentiation and inflammatory signaling pathways were also enriched in the enrichment analysis results of common genes from public disease databases. Therefore, an inflammatory environment caused by the immune and inflammatory response may be a common feature in the pathophysiology of osteoporosis and atherosclerosis.
Under physiological conditions, the bone homeostasis is regulated by osteoblast-mediated bone formation and osteoclast-mediated bone resorption through receptor activator of nuclear factor–κB (RANK), ligand for a RANK receptor (RANKL), and osteoprotegerin (OPG) interactions (33). Any imbalance of this control leads to an increase in the activity of osteoclast, resulting in osteoporosis. Although initially, it was thought that hormonal imbalance was the leading cause of osteoporosis, the role of the immune system in osteoporosis slowly came into view with advanced studies (34, 35). In pathological states such as rheumatoid arthritis, multiple sclerosis, osteoarthritis, and bone tumors, a host of inflammatory cytokines and activated immune cells disrupts this balance in favor of osteoclast-mediated bone resorption (36). Accumulated pieces of evidence suggested that immune and inflammatory response induced by both innate and adaptive immune cells can affect bone metabolism through several pathways.
Immune cell activation often goes along with inflammatory mediators’ production, such as reactive oxygen species, and pro-inflammatory cytokines and chemokines, which directly or indirectly influence bone metabolism and promote the development of osteoporosis (37). In innate immune system, pro-inflammatory cytokines such as TNF-a and IL-6 can stimulate the polarization of macrophages into M1 macrophages (both an inflammatory phenotype and a precursor of osteoclast), associating with bone catabolic activity (38). The monocytes show high levels of C-C chemokine receptor 2 (CCR2) in inflammatory microenvironment and can serve as osteoclast precursor, participating in bone remodeling by producing cytokines (39). In addition, dendritic cells and neutrophils can activate T cells, and the activated T cells produce cytokines and soluble factors that participate in bone resorption process (40). In adaptive immune system, T cells are major players of it and the results of our enrichment analysis were also involved in TH1, TH2, and TH17 cell differentiation and inflammatory factor–related pathways. Previous studies have found that TH1 and TH2 cells inhibit osteoclastogenesis via secreting IFN-γ and IL-4 cytokines and thus act as an osteoprotective role (41). Lower serum levels of IFN-γ and IL-4 cytokines in postmenopausal osteoporotic patients further suggest its osteoprotective role (42). However, a study suggested that IFN-γ can also promote osteoblasts generation by inducing expression of RANKL on activated T cells and thus plays a dual role in bone remodeling (43). TH17 cells are osteoclastogenic subsets of T cells and can produce proinflammatory cytokine, including RANKL, TNF-α, IL-17, and IL-6, all of which augment osteoclastogenesis (44). Moreover, enhancement in the number of TH17 cells and enhanced expression of proinflammatory cytokines (IL-6, TNF-α, RANKL, and IL-17) were observed in osteoporotic mice (45). Recent research suggested that “pyroptosis” of osteoblast (a programmed cell death mechanism) correlates with inflammation and contributes to excessive differentiation of osteoclasts via producing NLRP3 and also IL-1β and IL-18 (46). In summary, the concept of osteoimmunology was consistent with the enrichment results above in our study. Zhang et al. (47) performed a GO analysis of genes corresponding to differentially expressed proteins in osteoporosis and also found that immune inflammation and related functions were significantly enriched, consistent with our analysis.
Atherosclerosis is a chronic disease with an autoimmune component due to its accompaniment with a chronic, low-grade inflammatory response that attracts cells of the innate and adaptive immune systems into the atherosclerotic plaque (48). The important role of immunity and inflammation in atherosclerosis has been demonstrated by overwhelming experimental and clinical evidence. In our study, the analysis of positively correlated modules in atherosclerosis also supported this view. A genome-wide association study for coronary artery disease identified some locus linked to inflammation (49). The most robust genetic association has been identified for single-nucleotide polymorphisms in the 9p21 locus, which has been implicated in regulation of IFN-γ signaling (50). Oxidized LDL (oxLDL), the well-established precursor particles of atherosclerosis, can trigger inflammation of the arterial wall by binding to Toll-like receptors (TLRs) (51), and it can also reduce osteoblast viability (52).
In the vessel wall, oxLDL and inflammatory statement elicit an influx of monocytes that differentiate into macrophages and then into foam cells, accumulate intracellular cholesterol, and produce inflammatory mediators (53). CD4+ T cells are also recruited to the forming lesion, and single-cell data from human atherosclerotic plaques showed that the majority of CD4+ T cells in the plaque are TH1 and TH2 cells (54). In our study, we similarly found a significant enrichment for TH1 and TH2 cell differentiation pathway. Proinflammatory mediators such as IFN-γ, TNF-α, IL-2, and IL-3, produced by TH1 and TH2 cells, can activate macrophages and other plaque cells and thereby accelerate the inflammatory response (54). Knocking IFN-γ and its receptor protects mice from atherosclerosis (55). In addition, TH17 cells also can be induced by oxLDL and constitute a minor population in plaques, promoting the vascular inflammation (56). Ongoing inflammatory and hemodynamic assaults on the atherosclerotic lesion might eventually cause local dysfunction or breakdown of endothelial integrity. One study identified the DEGs in atherosclerosis, compared with healthy controls. The bioinformatics analysis showed that immune response, inflammatory response, and vascular smooth muscle contraction were the unique gene signatures in atherosclerosis, which were consistent with our analysis (57).
As mentioned above, we found that immune and inflammatory response were enrich in both atherosclerosis and osteoporosis, and most of common genes obtained from WGCNA, DEGs, and three public databases were associated with immune response. The immune-associated genes in both atherosclerosis and osteoporosis from WGCNA mainly included TREM1, CYBB, CCR1, CD83, CD52, IL7R, and THBS1. The immune-associated genes from DEGs mainly included TNF, ITGB2, CD74, CCR5, and MAF. The immune-associated genes from three public databases mainly included IFNG, IL2, IL4, CCL2, TGFB1, and TLR2. Therefore, the occurrence and development of atherosclerosis and osteoporosis are complicated and these genes may provide clues for the common underlying mechanisms between them. Suppression of inflammation may have beneficial effects on bone and on vasculature.
Then, six hub genes (COL1A1, IBSP, CTSD, RAC2, MAF, and THBS1) were obtained through the integration of multisource databases and they were all significantly upregulated in both the osteoporosis and atherosclerosis group compared with the control group. According to CTD, COL1A1 was closely associated with osteoporosis and atherosclerosis and it had the highest inference score and reference count. COL1A1 is mainly involved in bone matrix formation, coding for collagen type 1 which is the most abundant extracellular protein in bone and serves as an indicator of bone formation (58). Mutations in COL1A1 gene have been demonstrated to be responsible for the autosomal dominant form of osteogenesis imperfecta, with severe osteoporosis (59). Studies have confirmed that COL1A1 is overexpressed in artery at animals with atherogenic diet and the increase was contributed to the inflammatory process and the activate the cytokine TGF-β, which supports the fibrotic process through expressing COL1A1 (60). In addition, previous studies have shown that COL1A1 may influence the prognosis in tumors by affecting infiltrating immune cells (61). Therefore, COL1A1 may be involved in the pathogenesis of osteoporosis and atherosclerosis by influencing bone formation, vascular fibrosis, immune response, but these related molecular mechanisms warrant additional investigation. Thrombospondin-1 (THBS1), an immune-associated gene, can affect endothelial cell proliferation, migration, and apoptosis by antagonizing the activity of VEGF, participating in the regulation of vascular formation (62). The latest research suggests that CD68+ macrophages activate TGF-β1 by expression and secretion of THBS1 and consequently induce bone information (63). Importantly, THBS1 was closely related to inflammatory response and is found to be elevated in inflammatory processes (64). However, few studies directly analyze the role of THBS1 in osteoporosis and atherosclerosis, which emphasizes its importance in future research. Other hub genes were also involved in the pathogenesis of osteoporosis and atherosclerosis by regulating different BPs and believe that the specific mechanism is worthy of further exploration.
Finally, by constructing miRNAs–genes network, we found that let-7g had the most target genes and it also involved in immune and inflammatory response. It was reported that the let-7g was significantly downregulated in patients with recent osteoporotic fractures and it could enhance osteoblast formation in vitro and in vivo by targeting HMGA2 (65). In addition, it also can regulate osteoblast formation by targeting COL1A2 (66). More importantly, high-fat diet can suppress let-7g expression (67) and activate NF-κB signaling pathway, which serves as a pivotal mediator of inflammatory responses (68). let-7g can reduce macrophage transformation and alleviates foam cell apoptosis by suppressing NF-κB pathways to prevent atherosclerosis (69). Because the expression of let-7g is regulated by a number of cellular elements which are associated with inflammatory states and chemokine release (70), it is possible that inflammatory conditions contribute to the relative paucity of let-7g resulting in reducing osteogenic formation and increasing endothelial and foam cell apoptosis, in favor of both osteoporosis and atherosclerosis. In view of the critical role of let-7g in inflammatory response, let-7g might be an important potential target for the treatment of osteoporosis and atherosclerosis.
In conclusion, our work revealed that the immune and inflammatory response might be a common susceptible factor for both osteoporosis and atherosclerosis and identified novel gene candidates who could be used as biomarkers or as potential therapeutic targets. It may provide some clues for detailed molecular mechanisms underlying bone–vascular axis. However, the results of our study need to be further verified in cell or animal experiments, which will be critical direction for future research.
The original contributions presented in the study are included in the article/Supplementary Material. Further inquiries can be directed to the corresponding authors.
LM, CM, and YL contributed to the conception of the study; LM, CM, ZW, and JL performed the analysis; CZ, WN, ZC, and WH contributed significantly to analysis and manuscript preparation; LM and CM performed the data analyses and wrote the manuscript; LM, WH, ZC, CZ and YL helped perform the analysis and constructive discussions. YL, CZ, and ZC gained the founding; All authors contributed to the article and approved the submitted version.
This research was supported by Guangzhou Science and Technology Bureau (Grant No. 202102020930), National Natural Science Foundation of China (Grant No. 82104883, No. 81573996), Natural Science Foundation of Guangdong Province (Grant No. 2021A1515011484), Traditional Chinese Medicine Bureau of Guangdong Province (Grant No. 20221136) and Double First-rate Discipline and High-level University Construction Projects of Guangzhou University of Chinese Medicine (Grant No. 2021xk46).
The authors declare that the research was conducted in the absence of any commercial or financial relationships that could be construed as a potential conflict of interest.
All claims expressed in this article are solely those of the authors and do not necessarily represent those of their affiliated organizations, or those of the publisher, the editors and the reviewers. Any product that may be evaluated in this article, or claim that may be made by its manufacturer, is not guaranteed or endorsed by the publisher.
The Supplementary Material for this article can be found online at: https://www.frontiersin.org/articles/10.3389/fendo.2022.950030/full#supplementary-material
Supplementary Figure 1 | Sample clustering analysis (A) and clustering diagram (B) of GSE56815; Sample clustering analysis (C) and clustering diagram (D) of GSE28829. OP: osteoporosis; CON: control; AAS: advanced atherosclerosis; EAS: early atherosclerosis.
Supplementary Figure 2 | Volcanic map of DEGs (|logFC| > 1 and adj. p-value < 0.05) in GSE35958 (A) and in GSE100927 (B).
Supplementary Figure 3 | The functional enrichment analysis of eight common miRNAs through HMDD database.
1. Shao JS, Cai J, Towler DA. Molecular Mechanisms of Vascular Calcification: Lessons Learned From the Aorta. Arterioscler Thromb Vasc Biol (2006) 26:1423–30. doi: 10.1161/01.ATV.0000220441.42041.20
2. Thompson B, Towler DA. Arterial Calcification and Bone Physiology: Role of the Bone-Vascular Axis. Nat Rev Endocrinol (2012) 8:529–43. doi: 10.1038/nrendo.2012.36
3. Prabhakaran D, Anand S, Watkins D, Gaziano T, Wu Y, Mbanya JC, et al. Disease Control Priorities-3 Cardiovascular R, Related Disorders Author G: Cardiovascular, Respiratory, and Related Disorders: Key Messages From Disease Control Priorities, 3rd Edition. Lancet (2018) 391:1224–36. doi: 10.1016/S0140-6736(17)32471-6
4. Lee SN, Cho JY, Eun YM, Song SW, Moon KW. Associations Between Osteoporosis and Coronary Artery Disease in Postmenopausal Women. Climacteric (2016) 19:458–62. doi: 10.1080/13697137.2016.1200550
5. Hamada M, Kajita E, Tamaki J, Kouda K, Sato Y, Tachiki T, et al. Decreased Bone Mineral Density and Osteoporotic Fractures are Associated With the Development of Echogenic Plaques in the Carotid Arteries Over a 10-Year Follow-Up Period: The Japanese Population-Based Osteoporosis (JPOS) Cohort Study. Maturitas (2020) 131:40–7. doi: 10.1016/j.maturitas.2019.10.010
6. Lello S, Capozzi A, Scambia G. Osteoporosis and Cardiovascular Disease: An Update. Gynecol Endocrinol (2015) 31:590–4. doi: 10.3109/09513590.2015.1041908
7. Szekanecz Z, Raterman HG, Petho Z, Lems WF. Common Mechanisms and Holistic Care in Atherosclerosis and Osteoporosis. Arthritis Res Ther (2019) 21:15. doi: 10.1186/s13075-018-1805-7
8. Sugiyama T, Oda H. Osteoporosis Therapy: Bone Modeling During Growth and Aging. Front Endocrinol (Lausanne) (2017) 8:46. doi: 10.3389/fendo.2017.00046
9. Villella E, Cho JS. Effect of Aging on the Vascular System Plus Monitoring and Support. Surg Clin North Am (2015) 95:37–51. doi: 10.1016/j.suc.2014.09.007
10. Anagnostis P, Karagiannis A, Kakafika AI, Tziomalos K, Athyros VG, Mikhailidis DP. Atherosclerosis and Osteoporosis: Age-Dependent Degenerative Processes or Related Entities? Osteoporos Int (2009) 20:197–207. doi: 10.1007/s00198-008-0648-5
11. den Uyl D, Nurmohamed MT, van Tuyl LH, Raterman HG, Lems WF. (Sub)clinical Cardiovascular Disease is Associated With Increased Bone Loss and Fracture Risk; a Systematic Review of the Association Between Cardiovascular Disease and Osteoporosis. Arthritis Res Ther (2011) 13:R5. doi: 10.1186/ar3224
12. Szekanecz Z, Kerekes G, Vegh E, Kardos Z, Barath Z, Tamasi L, et al. Autoimmune Atherosclerosis in 3D: How it Develops, How to Diagnose and What to do. Autoimmun Rev (2016) 15:756–69. doi: 10.1016/j.autrev.2016.03.014
13. Szentpetery A, Horvath A, Gulyas K, Petho Z, Bhattoa HP, Szanto S, et al. Effects of Targeted Therapies on the Bone in Arthritides. Autoimmun Rev (2017) 16:313–20. doi: 10.1016/j.autrev.2017.01.014
14. Mishra BH, Mishra PP, Mononen N, Hilvo M, Sievanen H, Juonala M, et al. Uncovering the Shared Lipidomic Markers of Subclinical Osteoporosis-Atherosclerosis Comorbidity: The Young Finns Study. Bone (2021) 151:116030. doi: 10.1016/j.bone.2021.116030
15. An T, Hao J, Sun S, Li R, Yang M, Cheng G, et al. Efficacy of Statins for Osteoporosis: A Systematic Review and Meta-Analysis. Osteoporos Int (2017) 28:47–57. doi: 10.1007/s00198-016-3844-8
16. Hildebrand S, Cunningham J. Is There a Role for Bisphosphonates in Vascular Calcification in Chronic Kidney Disease? Bone (2021) 142:115751. doi: 10.1016/j.bone.2020.115751
17. Yao M, Zhang C, Gao C, Wang Q, Dai M, Yue R, et al. Exploration of the Shared Gene Signatures and Molecular Mechanisms Between Systemic Lupus Erythematosus and Pulmonary Arterial Hypertension: Evidence From Transcriptome Data. Front Immunol (2021) 12:658341. doi: 10.3389/fimmu.2021.658341
18. Mishra BH, Mishra PP, Raitoharju E, Marttila S, Mononen N, Sievanen H, et al. Et Al: Modular Genome-Wide Gene Expression Architecture Shared by Early Traits of Osteoporosis and Atherosclerosis in the Young Finns Study. Sci Rep (2021) 11:7111. doi: 10.1038/s41598-021-86536-0
19. Davis AP, Grondin CJ, Johnson RJ, Sciaky D, Wiegers J, Wiegers TC, et al. Comparative Toxicogenomics Database (CTD): Update 2021. Nucleic Acids Res (2021) 49:D1138–43. doi: 10.1093/nar/gkaa891
20. Safran M, Dalah I, Alexander J, Rosen N, Iny Stein T, Shmoish M, et al. GeneCards Version 3: The Human Gene Integrator. Database (Oxford) (2010) 2010:baq020. doi: 10.1093/database/baq020
21. Pletscher-Frankild S, Palleja A, Tsafou K, Binder JX, Jensen LJ. DISEASES: Text Mining and Data Integration of Disease-Gene Associations. Methods (2015) 74:83–9. doi: 10.1016/j.ymeth.2014.11.020
22. Langfelder P, Horvath S. WGCNA: An R Package for Weighted Correlation Network Analysis. BMC Bioinf (2008) 9:559. doi: 10.1186/1471-2105-9-559
23. Chen T, Liu YX, Huang L. ImageGP: An Easy-to-Use Data Visualization Web Server for Scientific Researchers. iMeta (2022) 1:e5. doi: 10.1002/imt2.5
24. Barrett T, Wilhite SE, Ledoux P, Evangelista C, Kim IF, Tomashevsky M, et al. Et Al: NCBI GEO: Archive for Functional Genomics Data Sets–Update. Nucleic Acids Res (2013) 41:D991–995. doi: 10.1093/nar/gks1193
25. Zhou Y, Zhou B, Pache L, Chang M, Khodabakhshi AH, Tanaseichuk O, et al. Metascape Provides a Biologist-Oriented Resource for the Analysis of Systems-Level Datasets. Nat Commun (2019) 10:1523. doi: 10.1038/s41467-019-09234-6
26. Correia de Sousa M, Gjorgjieva M, Dolicka D, Sobolewski C, Foti M. Deciphering Mirnas' Action Through miRNA Editing. Int J Mol Sci (2019) 20(24):6249. doi: 10.3390/ijms20246249
27. Huang Z, Shi J, Gao Y, Cui C, Zhang S, Li J, et al. HMDD V3.0: A Database for Experimentally Supported Human microRNA-Disease Associations. Nucleic Acids Res (2019) 47:D1013–7. doi: 10.1093/nar/gky1010
28. Chou CH, Shrestha S, Yang CD, Chang NW, Lin YL, Liao KW, et al. Mirtarbase Update 2018: A Resource for Experimentally Validated microRNA-Target Interactions. Nucleic Acids Res (2018) 46:D296–302. doi: 10.1093/nar/gkx1067
29. Laroche M, Pecourneau V, Blain H, Breuil V, Chapurlat R, Cortet B, et al. Committee Gs: Osteoporosis and Ischemic Cardiovascular Disease. Joint Bone Spine (2017) 84:427–32. doi: 10.1016/j.jbspin.2016.09.022
30. Mishra BH, Mishra PP, Mononen N, Hilvo M, Sievanen H, Juonala M, et al. Et Al: Lipidomic Architecture Shared by Subclinical Markers of Osteoporosis and Atherosclerosis: The Cardiovascular Risk in Young Finns Study. Bone (2020) 131:115160. doi: 10.1016/j.bone.2019.115160
31. Santhanam L, Liu G, Jandu S, Su W, Wodu BP, Savage W, et al. Skeleton-Secreted PDGF-BB Mediates Arterial Stiffening. J Clin Invest (2021) 131(20):e147116. doi: 10.1172/JCI147116
32. Wang ZX, Luo ZW, Li FX, Cao J, Rao SS, Liu YW, et al. Aged Bone Matrix-Derived Extracellular Vesicles as a Messenger for Calcification Paradox. Nat Commun (2022) 13:1453. doi: 10.1038/s41467-022-29191-x
33. Curtis EM, Moon RJ, Dennison EM, Harvey NC, Cooper C. Recent Advances in the Pathogenesis and Treatment of Osteoporosis. Clin Med (Lond) (2016) 16:360–4. doi: 10.7861/clinmedicine.16-4-360
34. Horton JE, Raisz LG, Simmons HA, Oppenheim JJ, Mergenhagen SE. Bone Resorbing Activity in Supernatant Fluid From Cultured Human Peripheral Blood Leukocytes. Science (1972) 177:793–5. doi: 10.1126/science.177.4051.793
35. Takayanagi H, Ogasawara K, Hida S, Chiba T, Murata S, Sato K, et al. T-Cell-Mediated Regulation of Osteoclastogenesis by Signalling Cross-Talk Between RANKL and IFN-Gamma. Nature (2000) 408:600–5. doi: 10.1038/35046102
36. Weitzmann MN, Ofotokun I. Physiological and Pathophysiological Bone Turnover - Role of the Immune System. Nat Rev Endocrinol (2016) 12:518–32. doi: 10.1038/nrendo.2016.91
37. Cline-Smith A, Axelbaum A, Shashkova E, Chakraborty M, Sanford J, Panesar P, et al. Ovariectomy Activates Chronic Low-Grade Inflammation Mediated by Memory T Cells, Which Promotes Osteoporosis in Mice. J Bone Miner Res (2020) 35:1174–87. doi: 10.1002/jbmr.3966
38. Murray PJ. Macrophage Polarization. Annu Rev Physiol (2017) 79:541–66. doi: 10.1146/annurev-physiol-022516-034339
39. Sprangers S, de Vries TJ, Everts V. Monocyte Heterogeneity: Consequences for Monocyte-Derived Immune Cells. J Immunol Res (2016) 2016:1475435. doi: 10.1155/2016/1475435
40. Saxena Y, Routh S, Mukhopadhaya A. Immunoporosis: Role of Innate Immune Cells in Osteoporosis. Front Immunol (2021) 12:687037. doi: 10.3389/fimmu.2021.687037
41. Srivastava RK, Sapra L. The Rising Era of "Immunoporosis": Role of Immune System in the Pathophysiology of Osteoporosis. J Inflammation Res (2022) 15:1667–98. doi: 10.2147/JIR.S351918
42. Zhang J, Fu Q, Ren Z, Wang Y, Wang C, Shen T, et al. Changes of Serum Cytokines-Related Th1/Th2/Th17 Concentration in Patients With Postmenopausal Osteoporosis. Gynecol Endocrinol (2015) 31:183–90. doi: 10.3109/09513590.2014.975683
43. Gao Y, Grassi F, Ryan MR, Terauchi M, Page K, Yang X, et al. IFN-Gamma Stimulates Osteoclast Formation and Bone Loss In Vivo via Antigen-Driven T Cell Activation. J Clin Invest (2007) 117:122–32. doi: 10.1172/JCI30074
44. Srivastava RK, Dar HY, Mishra PK. Immunoporosis: Immunology of Osteoporosis-Role of T Cells. Front Immunol (2018) 9:657. doi: 10.3389/fimmu.2018.00657
45. Dar HY, Singh A, Shukla P, Anupam R, Mondal RK, Mishra PK, et al. High Dietary Salt Intake Correlates With Modulated Th17-Treg Cell Balance Resulting in Enhanced Bone Loss and Impaired Bone-Microarchitecture in Male Mice. Sci Rep (2018) 8:2503. doi: 10.1038/s41598-018-20896-y
46. Tao Z, Wang J, Wen K, Yao R, Da W, Zhou S, et al. Pyroptosis in Osteoblasts: A Novel Hypothesis Underlying the Pathogenesis of Osteoporosis. Front Endocrinol (Lausanne) (2020) 11:548812. doi: 10.3389/fendo.2020.548812
47. Zhang L, Yang Y, Geng D, Wu Y. Identification of Potential Therapeutic Targets and Molecular Regulatory Mechanisms for Osteoporosis by Bioinformatics Methods. BioMed Res Int (2021) 2021:8851421. doi: 10.1155/2021/8851421
49. Davies RW, Wells GA, Stewart AF, Erdmann J, Shah SH, Ferguson JF, et al. A Genome-Wide Association Study for Coronary Artery Disease Identifies a Novel Susceptibility Locus in the Major Histocompatibility Complex. Circ Cardiovasc Genet (2012) 5:217–25. doi: 10.1161/CIRCGENETICS.111.961243
50. Harismendy O, Notani D, Song X, Rahim NG, Tanasa B, Heintzman N, et al. 9p21 DNA Variants Associated With Coronary Artery Disease Impair Interferon-Gamma Signalling Response. Nature (2011) 470:264–8. doi: 10.1038/nature09753
51. van der Valk FM, Bekkering S, Kroon J, Yeang C, Van den Bossche J, van Buul JD, et al. Oxidized Phospholipids on Lipoprotein(a) Elicit Arterial Wall Inflammation and an Inflammatory Monocyte Response in Humans. Circulation (2016) 134:611–24. doi: 10.1161/CIRCULATIONAHA.116.020838
52. Brodeur MR, Brissette L, Falstrault L, Ouellet P, Moreau R. Influence of Oxidized Low-Density Lipoproteins (LDL) on the Viability of Osteoblastic Cells. Free Radic Biol Med (2008) 44:506–17. doi: 10.1016/j.freeradbiomed.2007.08.030
53. Libby P, Ridker PM, Hansson GK. Progress and Challenges in Translating the Biology of Atherosclerosis. Nature (2011) 473:317–25. doi: 10.1038/nature10146
54. Zhao TX, Mallat Z. Targeting the Immune System in Atherosclerosis: JACC State-Of-the-Art Review. J Am Coll Cardiol (2019) 73:1691–706. doi: 10.1016/j.jacc.2018.12.083
55. Gupta S, Pablo AM, Jiang X, Wang N, Tall AR, Schindler C. IFN-Gamma Potentiates Atherosclerosis in ApoE Knock-Out Mice. J Clin Invest (1997) 99:2752–61. doi: 10.1172/JCI119465
56. Lim H, Kim YU, Sun H, Lee JH, Reynolds JM, Hanabuchi S, et al. Proatherogenic Conditions Promote Autoimmune T Helper 17 Cell Responses. Vivo Immun (2014) 40:153–65. doi: 10.1016/j.immuni.2013.11.021
57. Lu Y, Zhang X, Hu W, Yang Q. The Identification of Candidate Biomarkers and Pathways in Atherosclerosis by Integrated Bioinformatics Analysis. Comput Math Methods Med (2021) 2021:6276480. doi: 10.1155/2021/6276480
58. Procopio A, Malucelli E, Pacureanu A, Cappadone C, Farruggia G, Sargenti A, et al. Chemical Fingerprint of Zn-Hydroxyapatite in the Early Stages of Osteogenic Differentiation. ACS Cent Sci (2019) 5:1449–60. doi: 10.1021/acscentsci.9b00509
59. Dytfeld J, Marcinkowska M, Drweska-Matelska N, Michalak M, Horst-Sikorska W, Slomski R. Association Analysis of the COL1A1 Polymorphism With Bone Mineral Density and Prevalent Fractures in Polish Postmenopausal Women With Osteoporosis. Arch Med Sci (2016) 12:288–94. doi: 10.5114/aoms.2016.59253
60. Comariţa IK, Vîlcu A, Constantin A, Procopciuc A, Safciuc F, Alexandru N, et al. Et Al: Therapeutic Potential of Stem Cell-Derived Extracellular Vesicles on Atherosclerosis-Induced Vascular Dysfunction and Its Key Molecular Players. Front Cell Dev Biol (2022) 10. doi: 10.3389/fcell.2022.817180
61. Geng Q, Shen Z, Li L, Zhao J. COL1A1 is a Prognostic Biomarker and Correlated With Immune Infiltrates in Lung Cancer. PeerJ (2021) 9:e11145. doi: 10.7717/peerj.11145
62. Chu LY, Ramakrishnan DP, Silverstein RL. Thrombospondin-1 Modulates VEGF Signaling via CD36 by Recruiting SHP-1 to VEGFR2 Complex in Microvascular Endothelial Cells. Blood (2013) 122:1822–32. doi: 10.1182/blood-2013-01-482315
63. Deng R, Li C, Wang X, Chang L, Ni S, Zhang W, et al. Periosteal CD68(+) F4/80(+) Macrophages Are Mechanosensitive for Cortical Bone Formation by Secretion and Activation of TGF-Beta1. Adv Sci (Weinh) (2022) 9:e2103343. doi: 10.1002/advs.202103343
64. Khaiboullina SF, Morzunov SP, St Jeor SC, Rizvanov AA, Lombardi VC. Hantavirus Infection Suppresses Thrombospondin-1 Expression in Cultured Endothelial Cells in a Strain-Specific Manner. Front Microbiol (2016) 7:1077. doi: 10.3389/fmicb.2016.01077
65. Weilner S, Skalicky S, Salzer B, Keider V, Wagner M, Hildner F, et al. Differentially Circulating miRNAs After Recent Osteoporotic Fractures can Influence Osteogenic Differentiation. Bone (2015) 79:43–51. doi: 10.1016/j.bone.2015.05.027
66. Jiang ZS, Hao ZH. An Insertion/Deletion Polymorphism Within the 3'untranslated Region of COL1A2 Confers Susceptibility to Osteoporosis. Mol Med Rep (2016) 14:4415–21. doi: 10.3892/mmr.2016.5755
67. Chen KC, Hsieh IC, Hsi E, Wang YS, Dai CY, Chou WW, et al. Negative Feedback Regulation Between microRNA Let-7g and the oxLDL Receptor LOX-1. J Cell Sci (2011) 124:4115–24. doi: 10.1242/jcs.092767
68. Lin HL, Yen HW, Hsieh SL, An LM, Shen KP. Low-Dose Aspirin Ameliorated Hyperlipidemia, Adhesion Molecule, and Chemokine Production Induced by High-Fat Diet in Sprague-Dawley Rats. Drug Dev Res (2014) 75:97–106. doi: 10.1002/ddr.21159
69. Wang YS, Hsi E, Cheng HY, Hsu SH, Liao YC, Juo SH. Let-7g Suppresses Both Canonical and non-Canonical NF-kappaB Pathways in Macrophages Leading to Anti-Atherosclerosis. Oncotarget (2017) 8:101026–41. doi: 10.18632/oncotarget.18197
Keywords: osteoporosis, atherosclerosis, bioinformatics, molecular mechanism, inflammation, immune
Citation: Mo L, Ma C, Wang Z, Li J, He C, Niu W, Chen Z, Zhou C and Liu Y (2022) Integrated Bioinformatic Analysis of the Shared Molecular Mechanisms Between Osteoporosis and Atherosclerosis. Front. Endocrinol. 13:950030. doi: 10.3389/fendo.2022.950030
Received: 22 May 2022; Accepted: 22 June 2022;
Published: 22 July 2022.
Edited by:
Tarunveer Singh Ahluwalia, Steno Diabetes Center Copenhagen (SDCC), DenmarkReviewed by:
Haiming Jin, Wenzhou Medical University, ChinaCopyright © 2022 Mo, Ma, Wang, Li, He, Niu, Chen, Zhou and Liu. This is an open-access article distributed under the terms of the Creative Commons Attribution License (CC BY). The use, distribution or reproduction in other forums is permitted, provided the original author(s) and the copyright owner(s) are credited and that the original publication in this journal is cited, in accordance with accepted academic practice. No use, distribution or reproduction is permitted which does not comply with these terms.
*Correspondence: Yuhao Liu, ZG9jdG9yX3l1aGFvbGl1QDEyNi5jb20=; Chi Zhou, emNteTFAMTYzLmNvbQ==; Zhengqiu Chen, Y2hlbnpoZW5xaXUyMDEyQDEyNi5jb20=
†These authors have contributed equally to this work
Disclaimer: All claims expressed in this article are solely those of the authors and do not necessarily represent those of their affiliated organizations, or those of the publisher, the editors and the reviewers. Any product that may be evaluated in this article or claim that may be made by its manufacturer is not guaranteed or endorsed by the publisher.
Research integrity at Frontiers
Learn more about the work of our research integrity team to safeguard the quality of each article we publish.