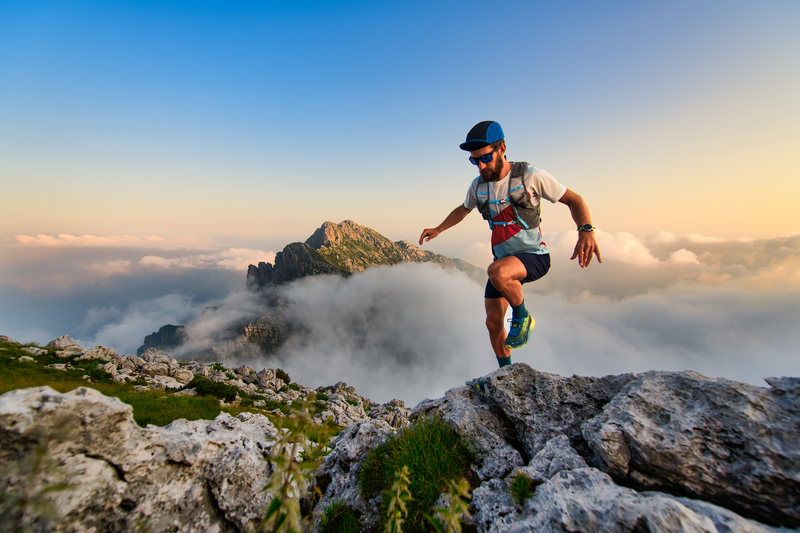
95% of researchers rate our articles as excellent or good
Learn more about the work of our research integrity team to safeguard the quality of each article we publish.
Find out more
ORIGINAL RESEARCH article
Front. Endocrinol. , 15 August 2022
Sec. Endocrinology of Aging
Volume 13 - 2022 | https://doi.org/10.3389/fendo.2022.895489
This article is part of the Research Topic Menopause: Mood Disorders and Obesity View all 7 articles
Background: Pre-diabetes precedes Diabetes Mellitus (DM) disease and is a critical period for hyperglycemia treatment, especially for menopausal women, considering all metabolic alterations due to hormonal changes. Recently, the literature has demonstrated the role of physical exercise in epigenetic reprogramming to modulate the gene expression patterns of metabolic conditions, such as hyperglycemia, and prevent DM development. In the present study, we hypothesized that physical exercise training could modify the epigenetic patterns of women with poor glycemic control.
Methods: 48 post-menopause women aged 60.3 ± 4.5 years were divided according to their fasting blood glucose levels into two groups: Prediabetes Group, PG (n=24), and Normal Glucose Group, NGG (n=24). All participants performed 14 weeks of physical exercise three times a week. The Infinium Methylation EPIC BeadChip measured the participants’ Different Methylated Regions (DMRs).
Results: Before the intervention, the PG group had 12 DMRs compared to NGG. After the intervention, five DMRs remained different. Interestingly, when comparing the PG group before and after training, 118 DMRs were found. The enrichment analysis revealed that the genes were related to different biological functions such as energy metabolism, cell differentiation, and tumor suppression.
Conclusion: Physical exercise is a relevant alternative in treating hyperglycemia and preventing DM in post-menopause women with poor glycemic control.
Diabetes Mellitus (DM) is characterized by chronic hyperglycemia resulting from deficiencies in insulin secretion, insulin action, or both. Type 2 Diabetes (T2D) results from a progressive loss of pancreatic islet function followed by long-term insulin resistance and elevated insulin secretion (1) Prediabetes is an intermediate condition that precedes TD2. These conditions could occur during menopause and are related to sex hormone levels and aging changes. Postmenopausal women tend to have fluctuations in the hormone’s estrogen and progesterone, these changes affect how cells respond to insulin. The process of hormonal changes after menopause causes fluctuations in blood sugar levels in women (2). During menopause, women could also experience predictable symptoms, such as increased total cholesterol and triglycerides (TG), decreased high-density lipoprotein cholesterol (HDL-c), increased android fat distribution, and impaired vascular function (2). Preventive action to manage hyperglycemia should be appropriately implemented in the prediabetes stage since glycemic control is the common factor determining complications from DM, especially in menopause (2, 3).
In this context, studies have emphasized the importance of epigenetic regulation considering its role in activating or deactivating genes that impact metabolic outcomes (4). Studies using animal models demonstrated epigenetic variations associated with the susceptibility to the development of metabolic diseases, such as DM (5).
Another factor that could influence hyperglycemia and metabolic alterations is exposure to heavy metals in the environment. Some metals, such as lead (Pb208), can increase oxidative stress-inducing metabolic changes related to DM (6). Correlations between heavy metals and glycosylated hemoglobin (HbA1c), an indicator of hyperglycemia and long-term alterations in glucose metabolism, have been found in cross-sectional studies in France and Saudi Arabia (7).
Exercise training can promote health and improve metabolism through different mechanisms. The impact of exercise training on epigenetic programming has been generating exciting results (8). Yahaya (9) demonstrated that myocyte enhancer factor 2 (MEF2) migrates to the nucleus during exercise after being phosphorylated, interacting with peroxisome proliferative active receptor gamma coactivator 1A (PPARγC1A), and histone acetyltransferases (HATs).
Physical exercise can increase the production of reactive oxygen species, both in skeletal muscle and in immune cells, leading to the development of oxidative stress, lipid, protein, and DNA modifications (10). Therefore, physical exercise can cause changes in DNA methylation (9, 11). Studies show that the response to physical exercise depends on genetic code and on the epigenetic signature, which can cause hypo or hypermethylation, of genes involved in muscle metabolism (9, 12). One study showed that chronic physical exercise caused hypomethylation of the promoter region of the nuclear receptor factor, fatty acid transporter (SLC27A4) and GLUT4 in patients with obesity and diabetes (13). Many studies involving physical exercise and DNA methylation, as Sailani et al. (14), showed that DNA methylation was lower in 714 promoter regions in physically active men compared to inactive men. Furthermore, it suggests that physical exercise is associated with the DNA methylation pattern increasing insulin sensitivity (14).
These modifications resulted in the acetylation of type 4 glucose transporter (GLUT4), improving the expression of the gene and insulin tolerance in the muscles (15). Exercise training can also ameliorate the effects of heavy metal accumulation by increasing urinary elimination (16). Due to all these benefits, exercise training could prevent hyperglycemia and its complications. In the present study, we hypothesized that physical exercise training could modify the epigenetic patterns of women with poor glycemic control. Thus, we aimed to analyze if the 14-week combined physical exercise intervention could modify epigenetic variables and improve the glucose serum levels.
The present study was approved by the Ethics Committee for Research with Humans of the School of Physical Education and Sport of Ribeirão Preto, University of São Paulo (EEFERP-USP). The registration number is CAAE: 79582817.0.0000.5656. The study was also registered in the Brazilian Registry of Clinical Trials under RBR-3g38dx. Participants were recruited in the Physical Education Program for the Elderly at EEFERP – USP. The inclusion criteria were women between 50 and 70 years with a medical clearance certificate to perform physical exercise and who were sedentary at the baseline. The exclusion criteria included diagnostic limitations to physical training or physical test, current smokers, caffeine consumption in the last 24 hours before any blood draw, and more than 25% combined training absence.
Forty-eight post-menopause women participated in the study. They were divided into two groups according to the fasting blood glucose levels determined by the American Diabetes Society (2020): Pre-diabetes Group (PG), composed of 24 women with a fasting blood glucose level higher than 100mg/dL and the Normal Glucose Group (NGG), composed of 24 women with a fasting blood glucose level lower than 100mg/dL (9).
The anthropometric evaluation was assessed before and after 14 weeks of combined training. The height was measured using a vertical stadiometer - Balmak - EST-223 and weight using the G-Tech digital scale - Balgl200, G-Tech. Subsequently, the body mass index was calculated using the formula (weight/(height*height)) (17). Waist and hip circumferences were measured following the recommendations of Malachias et al. (17). Participants’ systolic and diastolic blood pressure was measured using an Automatic Digital Upper Arm Blood Pressure Monitor Meter (SBH, 2010 - OMRON®, model HEM-7113) (17).
The biochemical analyses included uric acid, total cholesterol, high-density lipoproteins (HDL-C), low-density lipoproteins cholesterol (LDL-C), triglycerides (TG), blood glucose, and insulin. We also calculated the HORMAR IR (Blood (mmol) x Insulin (Ul/mL) ÷ 22. 5).
To investigate the physical benefits of 14 weeks of combined training, the elbow flexion and extension, sitting and standing up from a chair, agility dynamics, and the six-minute walk test was adopted. Also, the 6MWT (m) methodology was used to measure aerobic capacity (18).
We also determined the total serum concentration of metals (Hg202, Al27, As75, Cd111, Pb208, Ni60, Li7) through inductively coupled plasma mass spectrometry (ICP-MS), following the protocol described by Batista et al. (19).
Combined training was performed for 14 weeks, three times a week, lasting 60 minutes. The training was divided into strength exercises (30 minutes) and aerobic training (treadmill or bicycle, with a total duration of 30 minutes) (14).
The training periodization followed the first two weeks. The participants performed two sets of 15 to 17 maximum repetitions and 50% of the aerobic training in the reserve heart rate. The participants started to do 10 to 12 maximum repetitions in the remaining weeks, and aerobic training became 70% of the reserve heart rate. The participants-maintained values between three and six, a score presented on Borg’s subjective effort scale. The score we adopted on a scale from 0 to 10 represents moderate intensity. For the selection of exercises for strength training, the need to train the main muscles was considered, totaling five exercises for the muscles of the upper limbs and three exercises for the muscles of the lower limbs. Our group previously detailed the protocol (14).
The DNA methylation was analyzed at the baseline and after the 14 weeks of training. Salting-out technique (20) was used to extract DNA from leukocytes. Biodrop spectrophotometry was used to assess DNA quality and concentration using. 1% agarose gel test was used to guarantee genomic DNA integrity. The run time was 90 minutes at 80W (21).
Following the manufacturer’s recommendations, the bisulfite method was applied using the DNA EZ methylation kit (Zymo Research, CA, USA). Illumina BeadChip EPIC Infinium Methylation BeadChip 850k was used for the methylation experiment. All recommendations for the Infinium® HD Assay Methylation Protocol Guide were followed (22).
The raw data files were exported from iScan. A Samplesheet file was created to associate the collected information with raw data (23). The RStudio software (version 4.0.2) was used to process the methylation data. The data was organized as the participants’ codes, age, anthropometric, physical, biochemical, and metal information. The Sentrix Position column informs which row and column each sample was located in. The Sentrix_ID column provides the corresponding code of the BeadChip (23). The Bioconductor ChAMP data package was used for data normalization through the MyNorm function (24).
Differentially methylated regions (DMRs) were analyzed using the champ.DMR() function to compare PG vs. NGG groups. The bumphunter method was used to aggregate all probes into small groups. The random permutation method was applied to estimate DMRs with p < 0.05. Based on text mining and literature review, all DMRs were functionally annotated (24).
After identifying the DMRs inter and intra-group, the singular value decomposition (SVD) analysis method was used to evaluate the number and nature of significant components, correlating with various components of interest (24). Methylation data were normalized via minfi’s ssNoob (25). DNAmAge was calculated using the Methylclock package through the DNAmAge function (26). We also investigated the epigenetic clock of participants from both groups for intrinsic epigenetic age acceleration (IEAA) and extrinsic epigenetic age acceleration (EEAA). The epigenetic clock is a biomarker for age-related epigenetic changes as well as disease-specific changes (27). This clock has been recently developed by using specific DNA methylation sites to describe biological age at the level of the cell, tissue, and organ (28). The multi-tissue algorithm by Horvath generates age prediction, which highly correlates with chronological age above r=0.9 (29). In this study, DNAm Age was calculated using Horvath’s epigenetic clock algorithm using 353 CpGs (3).
In SVD analysis, the champ algorithm first does SVD deconvolution on the beta dataset. Then, the Random Theory Matrix method in the “isva” package is used to calculate the numbers of the latent variable and the top components of the SVD result. This analysis is comparable to a Principal Components Analysis (24)
Two researchers verified the resulting data to maintain the values’ reliability and accuracy. The Statistica 7.0 software (StatSoft Statistica®) was used to perform statistical comparisons. The Shapiro-Wilk test tested the normality. The Levene test assessed the equality of variance between groups. Then, repeated-measures ANOVA was performed, considering p <0.05. Fisher’s post hoc compared all means and controlled the error rate at the significance level. Then, a Spearman correlation was performed for the non-parametric data and a Pearson correlation for the parametric data to identify possible correlations. The Student’s t-test compared means in the descriptive analysis.
The mean age of participants in the PG was 61.3 ± 5.2 years, while in the NGG was 59.9 ± 4.8 years (Table 1). The 14 weeks of combined training had an effect of time in systolic blood pressure (F=24.304; p<0.001), diastolic blood pressure (F=6.180; p=0.016), total cholesterol (F=7.191); p=0.010), HDL-C (F=8.168; p=0.006), TG (F=11.176; p=0.001), and glucose (F=25.281; p<0.001). No undesirable effects were noted, but we did not observe group-time effect or group-time interaction (Table 1).
Table 1 Mean ± standard deviation of anthropometric, blood pressure, and biochemical variables pre and post-14 weeks of combined training (PG: n = 24; NGG: n = 24).
We observed the effect of time on elbow flexion and extension (F=17.028; p<0.001) for upper limb strength; sitting and standing up from a chair (F=20.264; p<0.001) for lower limb; agility test (F=51.248; p<0.001); and six-minute walk test (F=23.872; p<0.001) for aerobic fitness. The effect of time in both groups demonstrated an improvement in physical skills after 14 weeks of combined training. No group-time effect or group-time interaction was observed (Table 2).
Table 2 Mean ± standard deviation of the PRE AND POST motor variables 14 weeks of combined training (PG: n 24; NGG: n 24).
Analyzing metal levels and combined training together, we observed the effect of time decreasing mercury (Hg) (F=5.028; p=0.030) and lead (Pb) (F=12.100; p=0.001) in both groups. No effect or group-time interaction was observed (Table 3). There was a positive correlation between the arterial blood pressure and Pb208 in the PGpre (PAS: r=0.389, p=0.003; PAD: r=0.337, p=0.010). After the intervention, the correlation was not significant. The epigenetic clock was not different between groups and did not change after the 14-week physical intervention (Figure 1).
Table 3 Mean ± standard deviation of PRE and POST metal values 14 weeks of combined training (PG: n 24; NGG: n 24).
Figure 1 Differences in epigenetic age in PRE and POST comparisons 14 weeks of combined training. PG, prediabetes group; NGG, normal glucose group; EEAA, extrinsic epigenetic age acceleration; IEAA, intrinsic epigenetic age acceleration; Pre and pos-intervention comparison for the epigenetic clock in the PG group (panels A–C). Pre and post-intervention comparison for the epigenetic clock in the NGG group (panels D–F). (repeated measures two-way ANOVA was used). The presented values were not statically different between groups.
Before the physical exercise training, we found 12 DMRs between the PGpre and the NGGpre (Table 4). After the 14 weeks of exercise, five regions remained Differentially Methylated between the two groups (Table 5).
Table 4 Differentially Methylated Regions (DMRs) of the Prediabetes Group (PG) compared to the Normal Glucose Group (NGG) before 14 weeks of physical activity intervention.
Table 5 Differentially Methylated Regions (DMRs) of the Prediabetes Group (PG) compared to the Normal Glucose Group (NGG) before 14 weeks of physical activity intervention.
After the 14-week of exercise, we found 118 DMRs in the PGpost compared to the PGpre (Table 6), and we performed a Singular Value Decomposition Analysis (SVD) to verify if the covariables of interest could explain the DNA methylation patterns (Figure 2).
Table 6 Differentially Methylated Regions (DMRs) from the PGpost compared to the PGpre after 14-week physical intervention activity.
We also performed a Linear Regression of significant traits (Pb and glucose) and the DNA methylation targets of the PG group. We found three DMRs for Pb and 25 DMRs for glucose (Figure 3). BRCA1 was hypomethylated after a 14-week physical exercise intervention and was associated with glucose in the PG group. There was also an interesting overlap between the DMRs related to Pb and glucose enriched in the gene SLFN12. Notably, this gene region was differently methylated in the PGpre group than NGGpre and associated with Pb in the PG. After the intervention, a decrease in Pb was observed, and the methylation patterns of SLFN12 in PGpost became similar to NGGpost.,,
Figure 3 The intersection of Differently Methylated Regions of the prediabetes group (PG) between post-intervention, lead (Pb), and glucose. BRCA1 gene is related to 14-week intervention and glucose. SLFN12 gene is related to glucose and lead (Pb).
One of the aims of this study was to investigate the effect of combined training on epigenetic patterns in the prediabetes (PG) and normal glucose group (NGG). Combined training is an alternative program involving aerobic and strength exercises in the same training session (30). Its effects are widely recognized in the literature for the numerous benefits to the cardiorespiratory system, prevention of oxidative stress, and critical metabolic alterations in the glycemic balance (31). According to Bellazi et al. (12), the regulating factor of blood glucose concentration is achieved due to the power of action of the three variables: insulin, adequate meals, and physical exercise (32). Indeed, after 14 weeks of training, we found a decrease in glycemic values in the PG group, and in both groups, we observed decreased levels of total cholesterol and TG levels, indicating an improvement in metabolic indicators of cardiovascular health.
Moreover, the combined training improved both groups’ systolic and diastolic blood pressure. A study by Schroeder et al. (13) observed that the combined training protocol was the only one that promoted significant changes in blood pressure values, with positive reductions in diastolic pressure (33). They demonstrated that the combined training had positive cumulative results in all cardiovascular outcomes (33).
Combined training also demonstrated benefits in functional capacity in our study, providing a time effect for the PG and NGG groups. These findings corroborated with Medeiros et al. (14), demonstrating an increase in maximal and functional strength after 12 weeks of training in 58 women aged 50-75 years (34).
Regarding heavy metal concentration, we observed a decrease in mercury and lead levels after 14 weeks of combined training. Another finding of our study was the positive correlation between lead and systolic and diastolic blood pressure values in the PGpre group. Lead is widespread in the environment, and even low blood levels of this toxic metal may raise the risk of cardiovascular diseases, stroke, and chronic kidney disease. It is estimated that in adult populations lead exposures account for 5% of the population attributable risk for high blood pressure. Further, single toxicant exposures provide some evidence for developmental nephrotoxicity demonstrated by altered estimated glomerular filtration rate (eGFR) and other kidney biomarkers, increasing a risk for nephropathy in individuals with T2D (35). In a cross-sectional study carried out by Asgary et al. (15), the authors demonstrated that lead, mercury, and cadmium levels were higher in patients with coronary artery disease (36).
Our findings demonstrated that genes in differentially methylated regions of the PG group were related to inflammation and insulin signaling. Physical activity has improved glycemic control in adults with type 2 diabetes (27). Moreover, it can induce metabolic adaptations and changes in DNA methylation due to energy requirements (14). These changes in DNA methylation allowed for increased insulin sensitivity, expression of genes involved in energy metabolism, myogenesis, contractile properties, and oxidative stress (14). In the Figure 4 is shown some metabolic pathways functionally enriched from the obtained DMRs.
Figure 4 Representation of the pathways possibly influenced by differentially methylated regions (DMRs) in PG vs. NGG.
Figure 5 shows hypermethylated pathways that are potentially altered after 14-week physical exercise intervention. Here we described specific hypermethylated genes’ role in PG observed in our study. HLA-DPB1 plays a central role in the immune system by presenting peptides derived from extracellular proteins. Also, it is linked with inflammation and systemic insulin resistance (37). RWDD3 gene also plays an immune function. The isoforms RWDD3 positively regulate the NF-kappa-β signaling pathway by enhancing the NF-kappa-β inhibitor, promoting its stabilization, and regulating the hypoxia-inducible factor-1 alpha (38). Other genes are found hypermethylated in PG group-related metabolism and genomic stability. The gene TYW3/CRYZ is related to levels of resistin. Previous studies reported associations between elevated circulating resistin levels and increased risk of cardiovascular disease (28). PM20D1 is an enzyme that condenses fatty acids and amino acids. The PM20D1 locus shows genome-wide association studies (GWAS) with body mass index (BMI) and weaker associations with obesity-related conditions like type 2 diabetes and HDL-C levels (39). Aurora C kinases (AURKC) are essential kinases for cell division via regulating mitosis, especially the process of chromosomal segregation, associated with malignant cell transformation and genomic instability (16). PDE3A binding to SLFN12 results in cell killing and optimizing cancer therapeutics (40). WFIKKN2 is a follistatin domain-containing protein that binds GDF8/GDF11 proteins with high affinity, both of which have been implicated in DM development (41). The hypermethylation of these genes in individuals of the PG group could indicate a compromised metabolic function which could be associated with a higher risk of T2DM (42).
Figure 5 Representation of the pathways influenced possibly by hypermethylated regions in PG after the 14-week physical exercise intervention.
After the 14-week physical exercise intervention, 118 DMRs were detected. Three of these genes were hypermethylated: CALD1, RNF121, and MSI2. The CALD1 was previously related to diabetes nephropathy (43), while the role of RNF121 in the VEGF‐induced endothelial cell proliferation inhibition has been associated with diabetic retinopathy and age-related macular degeneration (44). The MSI2 hypomethylation has already been described as an epigenetic biomarker for hyperglycemic individuals by the Korean Genome Epidemiology Study (KoGES) (45).
The additional 115 genes were hypomethylated and involved in many biological functions (Figure 6). Regarding the effects on general metabolism, the FDPS (Farnesyl pyrophosphate synthase) is a crucial enzyme for several classes of essential metabolites, including sterols, dolichols, carotenoids, and ubiquinones. The BHLHE40 Class E basic helix-loop-helix protein 40 is also a target that may influence the general metabolism due to its role as a transcriptional repressor in regulating the circadian rhythm and negatively regulating the activity of the clock genes and clock-controlled genes (46).
Figure 6 Representation of the functions possibly influenced by hypomethylated regions in PG after the 14-week physical exercise intervention. The Functional Annotation was done using the Genecards Database and literature text mining.
The mechanisms related to metabolism also included targets related to macronutrients, such as lipids and carbohydrates, which are important in the etiology of hyperglycemia (47). The NR1H2 (Oxysterols receptor LXR-beta; Nuclear receptor) gene was differentially methylated and can regulate cholesterol uptake through the ubiquitination of LDLR, VLDLR, and LRP8. Indeed, the PLD3 - Phospholipase D3 is an important key in lipids metabolism and may be involved in the Amyloid Precursor Protein (APP), already been related to Alzheimer´s Disease (AD). Three genes were differentially methylated in carbohydrate metabolism: IDI1, AGL, and SIDT1. ID1 has already been related to nephropathy, a condition connected to advanced stages of T2D (48). The AGL gene encodes the glycogen debrancher enzyme involved in glycogen degradation (49). The SIDT1 gene is associated with insulin secretion, and its expression was decreased in individuals with hyperglycemia (50).
Four differentially methylated genes were associated with energetic metabolism: ATP6V1A, ATP5A1, NDUFA4, and SLC25A19. The ATP6V1A is responsible for acidifying a variety of intracellular molecules, leading to HIF1A hydroxylation and catalyzing AMP formation (51). The ATP5A1 produces ATP from ADP (52). NDUFA4 is a cytochrome c oxidase subunit NDUFA4 and catalyzes oxygen reduction in water (53). The SLC25A19 mitochondrial thiamine pyrophosphate carrier is a transporter mediating thiamine pyrophosphate (ThPP) entrance into mitochondria (54).
The most interesting genes involved with the immune function were the COPS4 signalosome complex subunit 4, involved in phosphorylation of p53/TP53, c-jun/JUN, IkappaBalpha/NFKBIA, which leads to modulation of the inflammasome (55); theWDR62 and DMP-1 related to oxidative stress; and the SLFN11, interferon (IFN)-induced target (16).
The tumor suppression mechanisms, insulin resistance, and metabolic diseases have a common path (56). The CMTM6 depletion significantly decreases tumor-specific T cell activity (57). Indeed, the hypermethylation of two CpG sites upstream of the CASP8AP2 promoter influenced gene expression and treatment outcomes in lymphoblastic leukemia (58). In the present study, CASP8AP2 was hypomethylated after the intervention, suggesting that physical exercise training may decrease the risk of developing cancer in this population. The VWA5A plays a role in tumorigenesis as a tumor suppressor (59). BRCA1 is another important tumor suppressor, and studies have shown that individuals with hyperglycemia had this gene naturally more methylated than normoglycemic individuals (60).
Physical exercise can promote many alterations at a cellular level. Liu et al., 2020, demonstrated that miRNAs could epigenetically modulate the myogenesis and adipogenesis processes after a 12-week physical exercise intervention in older individuals (61). In the present study, important differentially methylated regions in the PG were related to myogenesis and adipogenesis after the intervention. The DMRT2 directly regulates early activation of the myogenic determination. It is required to initiate or maintain the proper organization of the sclerotome, dermomyotome, and myotome (by similarity). Mutations in the MYOF gene can cause muscle weakness, which is involved in membrane regeneration and repair. Reduced KLF14 expression increases pre-adipocyte proliferation and decreases lipogenesis. In mice, the deletion of KLF14 partially reproduces the human phenotype of insulin resistance, dyslipidemia, and T2D (62). The deregulation of the KLF14/PLK1 cascade plays a crucial role in thrombin-induced endothelial dysfunction in T2DM patients (63).
There is substantial epidemiological evidence that exposure to low levels of various environmental chemicals can influence the development of chronic metabolic diseases, including diabetes (64). It has been well demonstrated that lead shows potential genotoxicity to various research subjects under different conditions (65, 66). A study with human lymphoblastoid TK6 cells found that lead exposure results in DNA damage via promoting oxidative stress and the promoter methylation of DNA repair genes, such as BRCA1 (67).
The BRCA1 plays a critical role in DNA repair. Mutations in the gene that lead to loss of protein function can accumulate damaged DNA within the cell tumor-suppressor gene, which is strongly linked to an increased risk of breast cancer development (68). Recent studies demonstrated that BRCA1 is expressed in skeletal muscle and is a critical regulator of metabolic function in cultured human myotubes (69). Also, deletion of BRCA1 expression in vascular cells results in the reduced catabolic breakdown of free fatty acids, and it can influence the metabolic phenotype of cultured cells and potentially the cells’ ability to respond to nutrient overload conditions (70).
Schlafens (SLFN) are intriguing proteins that play different roles in regulating cell proliferation, cell differentiation, immune cell growth and maturation, and inhibiting viral replication (17, 71).. However, it is still poorly understood how SLFNs interfere with the cell cycle machinery. It is a particularly tempting target for further research, as this gene is known to be down-regulated during T cell activation and up-regulated by type I interferons (IFNs) (18). It has also been suggested in cancer therapy since its expression was positively correlated with the growth inhibitory action of topoisomerase inhibitors on human cancer cells (19).
Acute exercise seems to be associated with active demethylation pathways. The mechanism proposed for active demethylation involves ten-eleven translocation (TET) proteins, suggesting that metabolites produced during exercise could alter the activity of TET proteins (20).
This study’s strengths were demonstrating the modulation of biochemical and physical parameters after a physical exercise intervention between different groups. In addition to verifying differently methylated regions in the PG group compared to the NGG group using a large-scale DNA methylation array (Infinium-EPIC beadchips) and the effect of combined training. The limitations were that analyzes of RNA expressions of the differentially methylated genes were not performed.
The 14-week combined physical exercise intervention led to the modulation of biochemical and physical parameters and the epigenetic reprogramming of genes involved in many biological functions such as metabolism, cell differentiation, tumor suppression, and immune function. Moreover, exercise training decreases mercury and lead serum concentrations. In future studies, longer intervention could be proposed to check the sustained effects of long-term combined physical exercise practice.
The datasets presented in this study can be found in online repositories. The names of the repository/repositories and accession number can be found below: GEO, GSE199700.
The present study was approved by the ethics committee for Research with Human of the School of Physical Education and Sport of Ribeirão Preto, University of São Paulo (EEFERP-USP). The registration number is CAAE: 79582817.0.0000.5656. The study was also registered in the Brazilian Registry of Clinical Trials under RBR-3g38dx. The patients/participants provided their written informed consent to participate in this study.
Conceptualization: NN and GR; methodology: NN, IN, and GR; formal analysis: NN, GR, CS-L, AC, and KR; resources: CBu and CN; data curation: GR, NN, and IN; performed experiments: NN, GR, LW, MP, DM, WS, and MA; writing—original draft preparation: NN, GR, IN, TP, CBr, and KR; writing—review and editing: TP, NN, GR, IN, and CBr; visualization: All authors; supervision: FB, CBu, and CN; funding acquisition: FB, CBu, and CN. All authors contributed to the article and approved the submitted version.
São Paulo Research Foundation (FAPESP) (#2017/21361-2) and (#2018/24069-3); National Council for Scientific and Technological Development (CNPq: #408292/2018-0). Personal funding: (FAPESP: #2014/16740-6; #2020/08687-9) and Academic Excellence Program from Coordination for higher Education Staff Development (CAPES:88882.180020/2018-01) and (CAPES:88882.180033/2018-01).
We would like to thank the Graduate Program in Clinical Medicine at the University of São Paulo, Ribeirão Preto, Foundation for Support of Teaching, Research and Assistance at HCFMRP-USP, Faculty of Medicine of São José do Rio Preto and School of Physical Education and Sport at the University from Sao Paulo and FAPEMIG (Foundation for Research Support of the State of Minas Gerais), process APQ-02169-21.
The authors declare that the research was conducted in the absence of any commercial or financial relationships that could be construed as a potential conflict of interest.
All claims expressed in this article are solely those of the authors and do not necessarily represent those of their affiliated organizations, or those of the publisher, the editors and the reviewers. Any product that may be evaluated in this article, or claim that may be made by its manufacturer, is not guaranteed or endorsed by the publisher.
1. Association, AD. Diagnosis and classification of diabetes mellitus. Diabetes Care (2009) 32:S62–7. doi: 10.2337/dc09-S062
2. Kaaja RJ. Metabolic syndrome and the menopause. Menopause Int (2008) 14(1):21–5. doi: 10.1258/mi.2007.007032
3. Luc K, Schramm-Luc A, Guzik TJ, Mikolajczyk TP. Oxidative stress and inflammatory markers in prediabetes and diabetes. J Physiol Pharmacol (2019) 70:809–24. doi: 10.26402/jpp.2019.6.01
4. Muka T, Asllanaj E, Avazverdi N, Jaspers L, Stringa N, Milic J, et al. Age at natural menopause and risk of type 2 diabetes: A prospective cohort study. Diabetologia (2017) 60:1951–60. doi: 10.1007/s00125-017-4346-8
5. Kato M, Natarajan R. Epigenetics and epigenomics in diabetic kidney disease and metabolic memory. Nat Rev Nephrol (2019) 15:327–45. doi: 10.1038/s41581-019-0135-6
6. Rosen ED, Kaestner KH, Natarajan R, Patti M-E, Sallari R, Sander M, et al. Epigenetics and epigenomics: Implications for diabetes and obesity. Diabetes (2018) 67:1923–31. doi: 10.2337/db18-0537
7. Javaid A, Akbar I, Javed H, Khan U, Iftikhar H, Zahra D, et al. Role of heavy metals in diabetes: Mechanisms and treatment strategies. Crit Rev Eukaryot Gene Expr (2021) 31:65–80. doi: 10.1615/CritRevEukaryotGeneExpr.2021037971
8. Cai J, Li Y, Liu S, Liu Q, Xu M, Zhang J, et al. Associations between multiple heavy metals exposure and glycated hemoglobin in a Chinese population. Chemosphere (2021) 287:132159. doi: 10.1016/j.chemosphere.2021.132159
9. Yahaya T. Role of epigenetics in the pathogenesis and management of type 2 diabetes mellitus. Translation: Univ Toledo J Med Sci (2019) 6:20–8. doi: 10.46570/utjms.vol6-2019-319
10. Denham J. Exercise and epigenetic inheritance of disease risk. Acta Physiol (Oxf) (2018) 222:1–20. doi: 10.1111/apha.12881
11. Tang S, Yu X, Wu C. Comparison of the levels of five heavy metals in human urine and sweat after strenuous exercise by ICP-MS. J Appl Mathematics Phys (2016) 4:183–8. doi: 10.4236/jamp.2016.42022
12. Bellazzi R, Nucci G, Cobelli C. The subcutaneous route to insulin-dependent diabetes therapy. IEEE Eng Med Biol Mag (2001) 20:54–64. doi: 10.1109/51.897828
13. Schroeder EC, Franke WD, Sharp RL, Lee D-C. Comparative effectiveness of aerobic, resistance, and combined training on cardiovascular disease risk factors: A randomized controlled trial. PloS One (2019) 14:e0210292. doi: 10.1371/journal.pone.0210292
14. Medeiros LHL, Sandbakk SB, Bertazone TMA, Bueno Júnior CR. Comparison of periodization models of concurrent training in recreationally active postmenopausal women. J Strength Cond Res (2020) 36:977–83. doi: 10.1519/JSC.0000000000003559
15. Asgary S, Movahedian A, Keshvari M, Taleghani M, Sahebkar A, Sarrafzadegan N. Serum levels of lead, mercury and cadmium in relation to coronary artery disease in the elderly: A cross-sectional study. Chemosphere (2017) 180:540–4. doi: 10.1016/j.chemosphere.2017.03.069
16. Anguita-Ruiz A, Bustos-Aibar M, Plaza-Díaz J, Mendez-Gutierrez A, Alcalá-Fdez J, Aguilera CM, et al. Omics approaches in adipose tissue and skeletal muscle addressing the role of extracellular matrix in obesity and metabolic dysfunction. Int J Mol Sci (2021) 22:2756. doi: 10.3390/ijms22052756
17. Malachias MVB. 7th Brazilian guideline of arterial hypertension: Presentation. Arq Bras Cardiol (2016) 107:XV–XIX. doi: 10.5935/abc.20160140
18. Rikli RE, Jones CJ. Development and validation of a functional fitness test for community-residing older adults. J Aging Phys Act (1999) 7(2):129–61. doi: 10.1123/japa.7.2.129
19. Batista BL, Rodrigues JL, Nunes JA, Souza VC, de O, Barbosa F. Exploiting dynamic reaction cell inductively coupled plasma mass spectrometry (DRC-ICP-MS) for sequential determination of trace elements in blood using a dilute-and-Shoot procedure. Anal Chim Acta (2009) 639:13–8. doi: 10.1016/j.aca.2009.03.016
20. Cabo R, Hernes S, Slettan A, Haugen M, Ye S, Blomhoff R, et al. Effects of polymorphisms in endothelial nitric oxide synthase and folate metabolizing genes on the concentration of serum nitrate, folate, and plasma total homocysteine after folic acid supplementation: A double-blind crossover study. Nutrition (2015) 31:337–44. doi: 10.1016/j.nut.2014.08.009
21. De Santis MA, Giuliani MM, Giuzio L, De Vita P, Lovegrove A, Shewry PR, et al. Differences in gluten protein composition between old and modern durum wheat genotypes in relation to 20th century breeding in Italy. Eur J Agron (2017) 87:19–29. doi: 10.1016/j.eja.2017.04.003
22. Li H, Chen R, Cai J, Cui X, Huang N, Kan H. Short-term exposure to fine particulate air pollution and genome-wide DNA methylation: A randomized, double-blind, crossover trial. Environ Int (2018) 120:130–6. doi: 10.1016/j.envint.2018.07.041
23. Masser DR, Stanford DR, Freeman WM. Targeted DNA methylation analysis by next-generation sequencing. J Vis Exp (2015) 96:52488. doi: 10.3791/52488
24. Tian Y, Morris TJ, Webster AP, Yang Z, Beck S, Feber A, et al. ChAMP: Updated methylation analysis pipeline for illumina BeadChips. Bioinformatics (2017) 33:3982–4. doi: 10.1093/bioinformatics/btx513
25. Fortin J-P, Triche TJ, Hansen KD. Preprocessing, normalization and integration of the illumina HumanMethylationEPIC array with minfi. Bioinformatics (2017) 33:558–60. doi: 10.1093/bioinformatics/btw691
26. Pelegí-Sisó D, de Prado P, Ronkainen J, Bustamante M, González JR. Methylclock: A bioconductor package to estimate DNA methylation age. Bioinformatics (2021) 37:1759–60. doi: 10.1093/bioinformatics/btaa825
27. Carbia-Nagashima A, Gerez J, Perez-Castro C, Paez-Pereda M, Silberstein S, Stalla GK, et al. RSUME, a small RWD-containing protein, enhances SUMO conjugation and stabilizes HIF-1alpha during hypoxia. Cell (2007) 131:309–23. doi: 10.1016/j.cell.2007.07.044
28. Qi Q, Menzaghi C, Smith S, Liang L, de Rekeneire N, Garcia ME, et al. Genome-wide association analysis identifies TYW3/CRYZ and NDST4 loci associated with circulating resistin levels. Hum Mol Genet (2012) 21:4774–80. doi: 10.1093/hmg/dds300
29. Benson KK, Hu W, Weller AH, Bennett AH, Chen ER, Khetarpal SA, et al. Natural human genetic variation determines basal and inducible expression of PM20D1, an obesity-associated gene. PNAS (2019) 116:23232–42. doi: 10.1073/pnas.1913199116
30. Lewis TA, de Waal L, Wu X, Youngsaye W, Wengner A, Kopitz C, et al. Optimization of PDE3A modulators for SLFN12-dependent cancer cell killing. ACS Med Chem Lett (2019) 10:1537–42. doi: 10.1021/acsmedchemlett.9b00360
31. Gudmundsdottir V, Zaghlool SB, Emilsson V, Aspelund T, Ilkov M, Gudmundsson EF, et al. Circulating protein signatures and causal candidates for type 2 diabetes. Diabetes (2020) 69:1843–53. doi: 10.2337/db19-1070
32. Kondás K, Szláma G, Trexler M, Patthy L. Both WFIKKN1 and WFIKKN2 have high affinity for growth and differentiation factors 8 and 11. J Biol Chem (2008) 283:23677–84. doi: 10.1074/jbc.M803025200
33. Félétou M, Galizzi J-P, Levens NR. NPY receptors as drug targets for the central regulation of body weight. CNS Neurol Disord Drug Targets (2006) 5:263–74. doi: 10.2174/187152706777452236
34. Wang J, Chen L, Qiang P. The role of IGF2BP2, an M6A reader gene, in human metabolic diseases and cancers. Cancer Cell Int (2021) 21:99. doi: 10.1186/s12935-021-01799-x
35. Chen Y, Gong W, Dai W, Pan Z, Xu X, Jiang H. MiR-596 down regulates SOX4 expression and is a potential novel biomarker for gastric cancer. Trans Cancer Res (2020) 9:1294–302. doi: 10.21037/tcr.2020.02.02
36. Wang Z, Wang Z, Zhou Z, Ren Y. Crucial genes associated with diabetic nephropathy explored by microarray analysis. BMC Nephrol (2016) 17:128. doi: 10.1186/s12882-016-0343-2
37. Maghsoudlou A, Meyer R, Rezazadeh K, Arafa E, Pudney J, Hartsough EJ, et al. RNF121 inhibits angiogenic growth factor signaling by restricting cell surface expression of VEGFR-2. Traffic (2016) 17:289–300. doi: 10.1111/tra.12353
38. Ahmed SAH, Ansari SA, Mensah-Brown EPK, Emerald BS. The role of DNA methylation in the pathogenesis of type 2 diabetes mellitus. Clin Epigenet (2020) 12:104. doi: 10.1186/s13148-020-00896-4
39. Sato F, Muragaki Y, Zhang Y. DEC1 negatively regulates AMPK activity via LKB1. Biochem Biophys Res Commun (2015) 467:711–6. doi: 10.1016/j.bbrc.2015.10.077
40. Chiu C-J, Taylor A. Dietary hyperglycemia, glycemic index and metabolic retinal diseases. Prog Retin Eye Res (2011) 30:18–53. doi: 10.1016/j.preteyeres.2010.09.001
41. Schisler JC, Charles PC, Parker JS, Hilliard EG, Mapara S, Meredith D, et al. Stable patterns of gene expression regulating carbohydrate metabolism determined by geographic ancestry. PloS One (2009) 4:e8183. doi: 10.1371/journal.pone.0008183
42. Perveen S, Gupta N, Kumar M, Kaur P, Chowdhury MR, Kabra M. Spectrum of amyloglucosidase mutations in Asian Indian patients with glycogen storage disease type III. Am J Med Genet A (2020) 182:1190–200. doi: 10.1002/ajmg.a.61547
43. Ottosson-Laakso E, Krus U, Storm P, Prasad RB, Oskolkov N, Ahlqvist E, et al. Glucose-induced changes in gene expression in human pancreatic islets: Causes or consequences of chronic hyperglycemia. Diabetes (2017) 66:3013–28. doi: 10.2337/db17-0311
44. Alzamora R, Al-Bataineh MM, Liu W, Gong F, Li H, Thali RF, et al. AMP-activated protein kinase regulates the vacuolar h+-ATPase via direct phosphorylation of the a subunit (ATP6V1A) in the kidney. Am J Physiology-Renal Physiol (2013) 305:F943–56. doi: 10.1152/ajprenal.00303.2013
45. Chomova M, Balazova M, Muchova J. Diabetes-induced abnormalities of mitochondrial function in rat brain cortex: The effect of n-3 fatty acid diet. Mol Cell Biochem (2017) 435:109–31. doi: 10.1007/s11010-017-3061-6
46. Pitceathly RDS, Rahman S, Wedatilake Y, Polke JM, Cirak S, Foley AR, et al. NDUFA4 mutations underlie dysfunction of a cytochrome c oxidase subunit linked to human neurological disease. Cell Rep (2013) 3:1795–805. doi: 10.1016/j.celrep.2013.05.005
47. Kang J, Samuels DC. The evidence that the DNC (SLC25A19) is not the mitochondrial deoxyribonucleotide carrier. Mitochondrion (2008) 8:103–8. doi: 10.1016/j.mito.2008.01.001
48. Bech-Otschir D, Kraft R, Huang X, Henklein P, Kapelari B, Pollmann C, et al. COP9 signalosome-specific phosphorylation targets P53 to degradation by the ubiquitin system. EMBO J (2001) 20:1630–9. doi: 10.1093/emboj/20.7.1630
49. Kaul K, Misri S, Ramaswamy B, Ganju RK. Contribution of the tumor and obese microenvironment to triple negative breast cancer. Cancer Lett (2021) 509:115–20. doi: 10.1016/j.canlet.2021.03.024
50. Burr ML, Sparbier CE, Chan Y-C, Williamson JC, Woods K, Beavis PA, et al. CMTM6 maintains the expression of PD-L1 and regulates anti-tumour immunity. Nature (2017) 549:101–5. doi: 10.1038/nature23643
51. Li Z-G, Jiao Y, Li W-J, Deng G-R, Cui L, Gao C, et al. Hypermethylation of two CpG sites upstream of CASP8AP2 promoter influences gene expression and treatment outcome in childhood acute lymphoblastic leukemia. Leuk Res (2013) 37:1287–93. doi: 10.1016/j.leukres.2013.07.018
52. Anghel SI, Correa-Rocha R, Correa-Rochal R, Budinska E, Boligan KF, Boliganl KF, et al. Breast cancer suppressor candidate-1 (BCSC-1) is a melanoma tumor suppressor that down regulates MITF. Pigment Cell Melanoma Res (2012) 25:482–7. doi: 10.1111/j.1755-148X.2012.01018.x
53. Karachanak-Yankova S, Dimova R, Nikolova D, Nesheva D, Koprinarova M, Maslyankov S, et al. Epigenetic alterations in patients with type 2 diabetes mellitus. Balkan J Med Genet (2016) 18:15–24. doi: 10.1515/bjmg-2015-0081
54. Liu H-W, Cheng H-C, Tsai S-H, Sun W-H. Effect of progressive resistance training on circulating adipogenesis-, myogenesis-, and inflammation-related MicroRNAs in healthy older adults: An exploratory study. GER (2020) 66:562–70. doi: 10.1159/000510148
55. Small KS, Todorčević M, Civelek M, El-Sayed Moustafa JS, Wang X, Simon MM, et al. Regulatory variants at KLF14 influence type 2 diabetes risk via a female-specific effect on adipocyte size and body composition. Nat Genet (2018) 50:572–80. doi: 10.1038/s41588-018-0088-x
56. Hao J-S, Zhu C-J, Yan B-Y, Yan C-Y, Ling R. Stimulation of KLF14/PLK1 pathway by thrombin signaling potentiates endothelial dysfunction in type 2 diabetes mellitus. BioMed Pharmacother (2018) 99:859–66. doi: 10.1016/j.biopha.2018.01.151
57. Zhu H, Ge K, Lu J, Jia C. Downregulation of GNAI3 promotes the pathogenesis of Methionine/Choline-deficient diet-induced nonalcoholic fatty liver disease. Gut Liver (2020) 14:492–9. doi: 10.5009/gnl19115
58. Jones OA, Maguire ML, Griffin JL. Environmental pollution and diabetes: A neglected association. Lancet (2008) 371:287–8. doi: 10.1016/S0140-6736(08)60147-6
59. Pasha Shaik A, Sankar S, Reddy SC, Das PG, Jamil K. Lead-induced genotoxicity in lymphocytes from peripheral blood samples of humans: In vitro studies. Drug Chem Toxicol (2006) 29:111–24. doi: 10.1080/01480540500408739
60. Nersesyan A, Kundi M, Waldherr M, Setayesh T, Mišík M, Wultsch G, et al. Results of micronucleus assays with individuals who are occupationally and environmentally exposed to mercury, lead and cadmium. Mutat Res Rev Mutat Res (2016) 770:119–39. doi: 10.1016/j.mrrev.2016.04.002
61. García-Lestón J, Méndez J, Pásaro E, Laffon B. Genotoxic effects of lead: An updated review. Environ Int (2010) 36:623–36. doi: 10.1016/j.envint.2010.04.011
62. Liu X, Wu J, Shi W, Shi W, Liu H, Wu X. Lead induces genotoxicity via oxidative stress and promoter methylation of DNA repair genes in human lymphoblastoid TK6 cells. Med Sci Monit (2018) 24:4295–304. doi: 10.12659/MSM.908425
63. Roy R, Chun J, Powell SN. BRCA1 and BRCA2: Different roles in a common pathway of genome protection. Nat Rev Cancer (2012) 12:68–78. doi: 10.1038/nrc3181
64. Jackson KC, Tarpey MD, Valencia AP, Iñigo MR, Pratt SJ, Patteson DJ, et al. Induced cre-mediated knockdown of Brca1 in skeletal muscle reduces mitochondrial respiration and prevents glucose intolerance in adult mice on a high-fat diet. FASEB J (2018) 32:3070–84. doi: 10.1096/fj.201700464R
65. Jackson KC, Gidlund E-K, Norrbom J, Valencia AP, Thomson DM, Schuh RA, et al. BRCA1 is a novel regulator of metabolic function in skeletal muscle. J Lipid Res (2014) 55:668–80. doi: 10.1194/jlr.M043851
66. Privat M, Radosevic-Robin N, Aubel C, Cayre A, Penault-Llorca F, Marceau G, et al. BRCA1 induces major energetic metabolism reprogramming in breast cancer cells. PloS One (2014) 9:e102438. doi: 10.1371/journal.pone.0102438
67. Vomhof-DeKrey EE, Lee J, Lansing J, Brown C, Darland D, Basson MD. Schlafen 3 knockout mice display gender-specific differences in weight gain, food efficiency, and expression of markers of intestinal epithelial differentiation, metabolism, and immune cell function. PloS One (2019) 14:e0219267. doi: 10.1371/journal.pone.0219267
68. Kovalenko PL, Basson MD. Schlafen 12 expression modulates prostate cancer cell differentiation. J Surg Res (2014) 190:177–84. doi: 10.1016/j.jss.2014.03.069
69. Katsoulidis E, Carayol N, Woodard J, Konieczna I, Majchrzak-Kita B, Jordan A, et al. Role of schlafen 2 (SLFN2) in the generation of interferon α-induced growth inhibitory responses. J Biol Chem (2009) 284:25051–64. doi: 10.1074/jbc.M109.030445
70. Li D, Chen J, Ai Y, Gu X, Li L, Che D, et al. Estrogen-related hormones induce apoptosis by stabilizing schlafen-12 protein turnover. Mol Cell (2019) 75:1103–1116.e9. doi: 10.1016/j.molcel.2019.06.040
Keywords: physical exercise, DNA methylation, combined physical exercise, epigenomics, older women
Citation: Yumi Noronha N, da Silva Rodrigues G, Harumi Yonehara Noma I, Fernanda Cunha Brandao C, Pereira Rodrigues K, Colello Bruno A, Sae-Lee C, Moriguchi Watanabe L, Augusta de Souza Pinhel M, Mello Schineider I, Luciano de Almeida M, Barbosa Júnior F, Araújo Morais D, Tavares de Sousa Júnior W, Plösch T, Roberto Bueno Junior C and Barbosa Nonino C (2022) 14-weeks combined exercise epigenetically modulated 118 genes of menopausal women with prediabetes. Front. Endocrinol. 13:895489. doi: 10.3389/fendo.2022.895489
Received: 13 March 2022; Accepted: 19 July 2022;
Published: 15 August 2022.
Edited by:
Michal Masternak, University of Central Florida, United StatesReviewed by:
Xunxun Feng, Beijing Anzhen Hospital, Capital Medical University, ChinaCopyright © 2022 Yumi Noronha, da Silva Rodrigues, Harumi Yonehara Noma, Fernanda Cunha Brandao, Pereira Rodrigues, Colello Bruno, Sae-Lee, Moriguchi Watanabe, Augusta de Souza Pinhel, Mello Schineider, Luciano de Almeida, Barbosa Júnior, Araújo Morais, Tavares de Sousa Júnior, Plösch, Roberto Bueno Junior and Barbosa Nonino. This is an open-access article distributed under the terms of the Creative Commons Attribution License (CC BY). The use, distribution or reproduction in other forums is permitted, provided the original author(s) and the copyright owner(s) are credited and that the original publication in this journal is cited, in accordance with accepted academic practice. No use, distribution or reproduction is permitted which does not comply with these terms.
*Correspondence: Guilherme da Silva Rodrigues, Z3Vpcm9kcmlndWVzQHVzcC5icg==
†These authors have contributed equal to this work
Disclaimer: All claims expressed in this article are solely those of the authors and do not necessarily represent those of their affiliated organizations, or those of the publisher, the editors and the reviewers. Any product that may be evaluated in this article or claim that may be made by its manufacturer is not guaranteed or endorsed by the publisher.
Research integrity at Frontiers
Learn more about the work of our research integrity team to safeguard the quality of each article we publish.