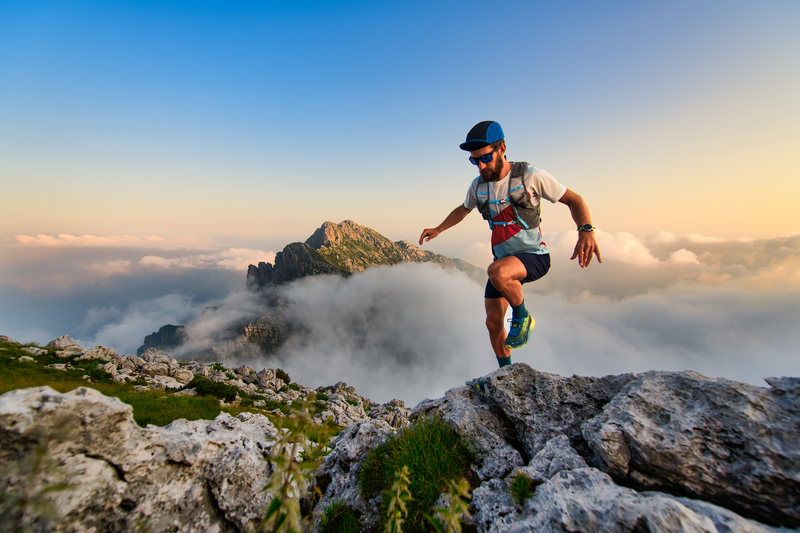
95% of researchers rate our articles as excellent or good
Learn more about the work of our research integrity team to safeguard the quality of each article we publish.
Find out more
ORIGINAL RESEARCH article
Front. Endocrinol. , 13 June 2022
Sec. Clinical Diabetes
Volume 13 - 2022 | https://doi.org/10.3389/fendo.2022.881633
We conducted the first genome-wide association study of prediabetes status change (to diabetes or normal glycaemia) among 900 White participants of the Atherosclerosis Risk in Communities (ARIC) study. Single nucleotide polymorphism (SNP)-based analysis was performed by logistic regression models, controlling for age, gender, body mass index, and the first 3 genetic principal components. Gene-based analysis was conducted by combining SNP-based p values using effective Chi-square test method. Promising SNPs (p < 1×10-5) and genes (p < 1×10-4) were further evaluated for replication among 514 White participants of the Framingham Heart Study (FHS). To accommodate familial correlations, generalized estimation equation models were applied for SNP-based analyses in the FHS. Analysis results across ARIC and FHS were combined using inverse-variance-weighted meta-analysis method for SNPs and Fisher’s method for genes. We robustly identified 5 novel genes that are associated with prediabetes status change using gene-based analyses, including SGCZ (ARIC p = 9.93×10-6, FHS p = 2.00×10-3, Meta p = 3.72×10-7) at 8p22, HPSE2 (ARIC p = 8.26×10-19, FHS p = 5.85×10-3, Meta p < 8.26×10-19) at 10q24.2, ADGRA1 (ARIC p = 1.34×10-5, FHS p = 1.13×10-3, Meta p = 2.88×10-7) at 10q26.3, GLB1L3 (ARIC p = 3.71×10-6, FHS p = 4.51×10-3, Meta p = 3.16×10-7) at 11q25, and PCSK6 (ARIC p = 6.51×10-6, FHS p = 1.10×10-2, Meta p = 1.25×10-6) at 15q26.3. eQTL analysis indicated that these genes were highly expressed in tissues related to diabetes development. However, we were not able to identify any novel locus in single SNP-based analysis. Future large scale genomic studies of prediabetes status change are warranted.
Diabetes is a major global public health challenge due to its high prevalence and associated morbidities and mortality (1). Prediabetes is a serious health condition where blood glucose levels are higher than normal, but not high enough to be diagnosed as type 2 diabetes (2). Approximately 88 million American adults aged 18 years or older, or more than 1 in 3 Americans, are estimated to have prediabetes (2). As the overweight and obesity rates continue to rise, these figures are expected to increase as well (3). To date, diabetes has emerged as a leading cause of blindness and end-stage renal failure and the seventh cause of mortality in the United States (2). The disease burden resulting from diabetes translates into a substantial economic toll. For example, the estimated total direct and indirect costs of diagnosed diabetes in the United States was $327 billion in 2017 (4).
Among people with prediabetes, about 5-10% will progress to overt diabetes annually, and a similar proportion will be converted back to normal (5). Prediabetes is also a critical time window for lifestyle interventions. Several landmark diabetes prevention clinical trials have provided robust evidence that participation in structured lifestyle interventions, focused on increased physical activity (2.5 to 4 hours/week), dietary modification (increased intake of whole grains, fiber, vegetables, and fruits; reduced intake of total and saturated fat, sugar, and refined grains), as well as weight reduction, improves blood glucose control and reduces more than 50% risk of diabetes (6–8).
Diabetes is a highly inheritable trait (9). Current genomic studies have identified many loci for diabetes and explained about 10% of the heritability (10). A large proportion of the heritability is still missing and many genes for diabetes are yet to be identified. Previous genomic studies of diabetes have primarily focused on the incidence of diabetes among population-based cohorts or have compared diabetes cases with controls in case-control studies (10–14). Several studies investigated genomic loci for diabetes phenotypes among participants with prediabetes (15, 16). None of those studies have investigated the prediabetes status change. Such investigation may help to identify novel genes for prediabetes status change. Therefore, the purpose of the current study was to identify genetic variants/genes associated with prediabetes status change by conducting genome-wide single nucleotide polymorphism (SNP)-based and gene-based association analyses among prediabetes participants of the Atherosclerosis Risk in Communities Study (ARIC).
The ARIC is a population-based epidemiologic study among a total of 15,792 Black and White participants recruited from 4 communities (17). ARIC participants received extensive medical examinations every three years since the baseline in 1987-89 (17). The fourth follow-up visit was conducted in 1996-98 (18). ARIC data on genotypes, diabetes related measures, and important covariates were cataloged on the database of genotype and phenotype (dbGaP). We’ve received approval to use the data from both the Institute Review Board (IRB) at Tulane University and the dbGaP.
Due to very few Black participants with prediabetes in the ARIC study, our analysis was only conducted among White participants. As shown in the flow chart in Figure 1, a total of 3,464 White participants had prediabetes at baseline, diabetes related data was measured for 2,497 at the fourth clinical visit in 1996-98, and genome-wide genotypes were available for 2,205 of the participants on dbGaP. Among the 2,205 participants, 354 progressed to diabetes, 546 reversed to normal glycaemia, and 1,202 remained prediabetic. Our discovery stage analysis was conducted by comparing the 354 individual who progressed diabetes with the 546 participants who reversed to normal glycemia.
Genome-wide autosomal SNPs were genotyped using the Affymetrix 6.0 platform for a total of 8,620 unrelated White ARIC participants, and are available on dbGaP. Related pairs, duplicates, or gender misclassification were already evaluated and quality controlled in the genomic data. We performed further quality control and removed SNPs with Hardy-Weinberg equilibrium p < 1×10-6, missing rate>10%, or minor allele frequency (MAF) < 1% before genotype imputation. Individuals with missing genotype rate > 80% were also removed. After quality control, a total of 703,117 SNPs remained for genotype imputation. Imputation from the ALL ancestry panel of the 1000 Genome Phase III integrate Release Version 5 (19) was conducted for all White ARIC participants using MiniMac software (20). After imputation, SNPs with r2 < 0.30, MAF < 1%, or Hardy-Weinberg equilibrium p < 1×10-6 were removed, and a total of 10,008,913 SNPs, with fractional values ranging from 0 to 2, were retrieved for the 900 participants with prediabetes with changes in status for analysis.
In the ARIC, data on fasting blood glucose and diabetes medication use was collected at both baseline and the fourth clinical visit. This information was used to identify participants with prediabetes at baseline and to evaluate prediabetes status change in the fourth clinical visit according to the diagnosis guideline of the American Diabetes Association (21). Prediabetes was defined as fasting glucose level between 100 and 126 mg/dl and not taking glucose lowering medications. Diabetes was defined as fasting glucose ≥ 126 mg/dl, taking glucose lowering medications, or random glucose level ≥ 200 mg/dl. Those who had fasting or non-fasting glucose level <100 mg/dl and were not taking diabetes medication were defined as normal glycaemia.
Covariates included age, sex, body mass index (BMI), and the first 3 genetic principal components in the European-American sample. Age and sex were determined by self-report. Sex identity was checked by examining both X chromosome heterozygosity and the means of the intensive of SNP probes on the X and Y chromosomes (22). Population structure was investigated using principal components as described by Patterson et al. (23), and was available on the dbGaP. We adjusted for the first 3 genetic principal components to control for population substructure. Body weight and height were measured with participants wearing scrub suits and no shoes (24). Baseline BMI calculated as kg/m2 was included as a covariate.
We attempted to replicate promising ARIC findings among participants of the Framingham Heart Study (FHS). In FHS, fasting glucose was measured in visits 7, 8, 9, 10, and 13-23 in the original Framingham cohort. To be compatible with the ARIC study in length of follow-up time (≈ 9 years) and maximize study sample size, we treated visit 8 in 1962-66 as baseline and visit 13 in 1972-76 as the end follow-up period. In the offspring cohort, visit 1 in 1971-75 was selected as baseline and visit 2 in 1979-83 as end follow-up period. In the third-generation cohort, baseline was visit 1 in 2002-05 and end follow-up period was visit 2 in 2008-11. As shown in Figure 2, a total of 1,774 White participants with prediabetes were selected from the three Framingham cohorts, and 1,319 had genotypes available on the dbGaP. Diabetes status was determined cumulatively, and was available among a total of 1,146 participants with genotypes. In about 9 years’ follow-up, 147 participants developed diabetes, 367 participants reversed to normal, and 632 participants remained to be prediabetic. The replication analysis was performed by comparing the 147 individuals who progressed to diabetes with the 367 participants reversed to normal glycemia.
Genome-wide SNPs were genotyped using Affymetrix and Illumina platforms in FHS. The 1000 Genome genotype data for FHS was already imputed and cataloged on the dbGaP. According to the document of the FHS (25), before imputation, quality control removed SNPs with Hardy-Weinberg equilibrium p < 1×10-6, missing rate > 3.1%, MAF < 1%, missing physical position or not mapped to build 37 positions, Mendelian errors > 1000, or duplicate SNPs. MACH software was used for genotype phasing, followed by imputation using Minimac software (19, 20). SNPs within 3-Mb regions surrounding identified SNPs or SNPs in a promising gene in ARIC were imputed based on the ALL ancestry panel from the 1000G Phase I Integrated Release Version 3 Haplotypes (19, 20). After imputation, SNPs with r2 < 0.30, MAF < 1%, or Hardy-Weinberg equilibrium p < 1×10-6 were removed.
The current analysis focuses on the comparison between prediabetes participants progressed to diabetes vs. those reversed to normal glycemia. We conducted both single SNP-based analysis and gene-based analysis as follows:
Logistic regression models were used to examine SNP-prediabetes status change associations (diabetes vs. normal glycaemia), after controlling for age, sex, body mass index, and the first 3 genetic principal components for population substructures in the ARIC. To accommodate familial relationships, generalized estimating equation models with compound symmetry correlation matrix were used to test SNP- prediabetes status change associations in the FHS, adjusting for the same covariates as in the ARIC. SNPs with discovery stage p < 1×10-5 in the ARIC were further evaluated in the FHS. Results from the ARIC and FHS were combined using inverse-variance-weighted meta-analysis method implemented in METAL software (26). After ensuring that the effect directions were consistent, SNPs with replication stage p < 0.05, and meta-analysis p < 5×10-8 were considered significant.
Similar to previous gene-based studies (27–29), SNPs within the 5-kb flanking regions of a gene were first mapped to the gene according to physical position. SNPs within 5-kb flanking regions of 2 genes were assigned to both genes. P values from single marker analysis were used to generate gene-based P values using the effective Chi-square test (ECS) method implemented in KGG software (30, 31). The ECS method uses SNP p values and LD information from the 1000G reference population of European ancestry to generate gene-based p values (30, 31). This method is more powerful for genes harboring multiple dense independent risk variants compared to the commonly used gene-based association test using Simes procedure (GATES) method (30, 31). Similar to previous genome-wide gene-based studies (28, 29), genes with p < 1×10-4 in the discovery stage analysis in ARIC were further evaluated for replication among FHS participants. In the FHS, SNPs from promising genes were tested for associations with prediabetes status change using methods described in the above single marker based analysis, and p values of these SNPs were again used to generate gene-based p values using the ECS method (30, 31). Fisher’s method was applied to combine gene-based p values across the ARIC and FHS (32). Genes with replication stage p < 0.05 and combined p < 2.5×10-6 (correcting for 20,000 genes across the genome: 0.05/20,000 = 2.5×10-6) were considered significant.
For significant SNPs and/or genes, we plotted regional SNP association plots using the KGG software (30), and searched their expression profiles in the Genotype-Tissue Expression (GTEx) project (33). The GETx project tested cis-eQTLs for all SNPs within 1 Mb flanking regions of the transcriptional start site of each gene in each tissue, using linear regression after correction for known and inferred technical covariates (34). Gene-level expression values were quantile normalized. Permutation-adjusted p value was computed for the most significant SNP in a gene, and was used to represent gene specific significance level. This approach corrects for multiple SNPs per gene (34). The eGene is defined as a gene with at least one SNP in cis significant association with expression differences of that gene after false discovery rate correction (34).
Characteristics of both ARIC and FHS participants are shown in Table 1. ARIC participants were older and less likely to be male, compared to FHS participants. Participants of both studies were, on average, overweight or obese.
Population substructures were well controlled (genomic inflation lambda = 1.027). Five independent loci (r2 < 0.3) reached suggestive significance (p < 1×10-5) in the discovery stage genome-wide analysis (Figures 3, 4). As shown in Table 2, none of them were replicated in FHS. GPR176 variant rs41497851 had a replication stage p = 0.0012. However, the direction of effect estimate was not consistent with that in the ARIC.
A total of 36 genes located in 30 loci (within 1 mega base regions) had p < 1×10-4 in the discovery stage gene-based analysis (Figures 5, 6), and were further evaluated for replication among FHS participants. Meta-analysis results for significant genes are shown in Table 3. Eight genes reached genome-wide significance in the discovery stage gene-based analysis, including ZNF717 at (p = 1.20×10-8) 3p12.3, DIP2C (p = 1.99×10-7) at 10p15.3, HPSE2 (p = 8.26×10-19) at 10q24.2, UROS (p = 1.39×10-8) at 10q26.2, SIK3 (p = 2.85×10-23) at 11q23.3, HHIPL1 (p = 4.27×10-13) at 14q23.2, LINC00523 (p = 2.87×10-10) at 14q32.2, and LOC102723354 (p = 5.34×10-11) at 14q32.33. The HPSE2 was previously reported to be associated with type 1 diabetes (35) and the LINC00523 was associated with type 2 diabetes (36). The combined analysis among ARIC and FHS identified five novel genes that reached genome-wide significance, including SGCZ (ARIC p = 9.93×10-6, FHS p = 2.00×10-3, Meta p = 3.72×10-7) at 8p22, HPSE2 (ARIC p = 8.26×10-19, FHS p = 5.85×10-3, Meta p < 8.26×10-19) at 10q24.2, ADGRA1 (ARIC p = 1.34×10-5, FHS p = 1.13×10-3, Meta p = 2.88×10-7) at 10q26.3, GLB1L3 (ARIC p = 3.71×10-6, FHS p = 4.51×10-3, Meta p = 3.16×10-7) at 11q25, and PCSK6 (ARIC p = 6.51×10-6, FHS p = 1.10×10-2, Meta p = 1.25×10-6) at 15q26.3. In addition, gene SIK3 (ARIC p = 2.85×10-23) was marginally significant in FHS (p = 6.01×10-2), and reached genome-wide significance in the combined analysis (p < 1×10-8). Regional association plots for the 6 genes are demonstrated in Figure 7.
eQTL analysis results are shown in Table 4. The 6 significant genes had eGenes in a variety of tissues, ranging from 2 tissues for the SGCZ gene to 14 tissues for the HPSE2 gene. eGenes were identified for 5 genes in esophagus tissues (including mucosa and muscularis), 4 genes in tibial nerve tissue, 4 genes in adipose tissues (subcutaneous or visceral), 4 genes in aorta or coronary arteries, 4 genes in brain tissues, and 3 genes in thyroid tissue. eGenes were also identified for 2 genes in tissues of tibial artery, EBV-transformed lymphocytes, sigmoid colon, heart, lung, skeletal muscle, pituitary, stomach, vagina, testis, suprapubic skin, and lower leg skin, respectively. eGenes were identified in single tissue for 3 genes, including the ADGRA1 in adrenal gland, liver, and spleen tissues, the PCSK6 in atrial appendage, and pancreas tissues, and the GLB1L3 in uterus.
In the first genome-wide single SNP-based and gene-based analysis on prediabetes status change conducted in participants of European ancestry, we identified 5 novel genes, SGCZ at 8p22, HPSE2 at 10q24.2, ADGRA1 at 10q26.3, GLB1L3 at 11q25, and PCSK6 at 15q26.3 that were associated with prediabetes status change. In addition, gene-based analysis replicated a previously reported association between LINC00523 gene and type 2 diabetes (36).
Gene SGCZ encoding zeta-sarcoglycan protein of the sarcoglycan complex (37) was associated with prediabetes status change in the current gene-based analysis. The SGCZ gene has been reported in genome-wide association studies (GWAS) of BMI (38) and obesity-related traits (39). These two phenotypes are highly associated with diabetes (40). In addition, another gene SGCG encoding protein in the sarcoglycan complex has been identified for diabetes among Punjabi Sikhs population in India (41). Animal studies have provided further evidence for the involvement of the SGCZ gene in diabetes development. For example, Groh and colleagues demonstrated that mice with sarcoglycan complex deficiency in adipose tissue and skeletal muscle had glucose-intolerance and insulin resistance specifically due to impaired insulin-stimulated glucose uptake in skeletal muscles (42). Despite biologically relevant in diabetes development, our study provided the first evidence that the SGCZ gene is involved in prediabetes status change in humans. Future large-scale genomic and functional studies of this gene are warranted to identify variants within this gene that are associated with prediabetes status change.
HPSE2 gene encoding heparanase 2 (43), an enzyme that degrades heparin sulfate proteoglycans (44), was associated with prediabetes status change in the current study. The gene was suggested to be in association with type 1 diabetes in a previous GWAS meta-analysis (35). Furthermore, experimental studies indicated that the encoded heparanase was engaged in diabetes initiation and progression. Ziolkowski et al. reported that activation of heparanase was related to the destruction of pancreatic islets and inhibition of heparanase preserved intra-islet heparin sulfate, and protected mice from type 1 diabetes (45). In addition, degradation of heparin sulfate proteoglycans by heparanase creates a burst of cytokine release and can possibly promote beta cell death (46). In humans, studies demonstrated that serum and urinary heparanase levels were markedly elevated in type 2 diabetes patients compared to health controls (47) and were essential for the development of diabetic nephropathy (48). Our study provided robust evidence from population-based studies for the involvement of this gene in diabetes development. Further studies with a larger sample size and higher resolution genotypes are warranted to identify causal variants within this gene for prediabetes status change.
ADGRA1 gene was associated with prediabetes status change in the current analysis. ADGRA1 encodes adhesion G protein-coupled receptor A1 that belongs to the adhesion family of G-protein-coupled receptors (49). Receptors of this family regulate blood pressure (50), immune response (51), food intake (52) and development (53). These important functions are all related to glucose regulation. Therefore, G protein-coupled receptors have been new therapeutic targets for type 2 diabetes (54). Our study provided the first evidence that the ADGRA1 gene is involved in prediabetes status change. Future studies are warranted to investigate the therapeutic role of this gene or its encoded protein in diabetes prevention among prediabetics.
GLB1L3 gene encoding galactosidase beta 1 like 3 (55) was associated with prediabetes status change in the gene-based analysis. The biological relevance of this to glucose metabolism is not very clear. It may be involved in lactate production through converting serum lactose into glucose and galactose (56). Our study provided the first evidence of this gene in prediabetes status change in humans. Future works are warranted to delineate the causal role of this gene in blood glucose regulation.
Gene-based analysis also identified PCSK6 gene that was associated with prediabetes status change. PCSK6 gene encodes proprotein convertase subtilisin/kexin type 6, and plays important roles in the maturation of insulin receptor isoform B, and cholesterol and fatty acid metabolism (57, 58). In previous GWASs, PCSK6 was reported to be associated with relative hand skill (59, 60). Large population based study indicated that left handedness increased risk of diabetes by 25% (61). Our finding may explain the mechanisms underlying the two observed associations. In addition, the PCSK6 gene also activates corin, an important biomarker for salt-sensitive hypertension and diabetes (62, 63). More importantly, eQTL analysis identified that the PCSK6 gene had SNPs in significant cis associations with its expression in the pancreas, pituitary, and omental adipose tissues. All these tissues are related to diabetes (64, 65). However, these are preliminary findings, and future functional study of the PCSK6 gene in diabetes development are warranted.
SIK3 gene was marginally significant in the replication stage analysis among FHS participants (p = 0.06), however, reached genome-wide significance in the combined analysis. This gene encoding SIK family kinase 3, is also biologically relevant to glucose metabolism, and is a potential target for diabetes therapeutics (66). SIK3 knocked-out mice had a high expression level of gluconeogenic gene, were leaner and more resistant to high-fat diet, and had excessive hypoglycemia (67). In humans, preliminary studies showed that SIK3 was downregulated in adipose tissues from obese or insulin-resistant individuals (68). Our study provided further evidence for the involvement of this gene in prediabetes status change. Future larger population-based studies are warranted to investigate the role of this gene in prediabetes status change.
eQTL analysis provided further evidence for the involvement of these 6 novel genes in diabetes development. Each of the novel genes had significant cis eQTL in tissues related to diabetes. For example, the PCSK6 gene has significant eGene in the pancreas tissue. In addition, significant cis eQTLs were identified for more than 3 genes in tissues of esophagus, tibial nerve, adipose, aorta or coronary arteries, brain, and thyroid, respectively. Previous studies have shown that these tissues were all involved in diabetes pathogenesis. For example, diabetes may increase the risk of Barrett’s esophagus, indicating that genes involved in diabetes may contribute to Barrett’s esophagus pathogenesis (69). The cross-sectional area of the posterior tibial nerve was larger in patients with diabetes compared to healthy controls (70). Adipose tissue has long been a key target for diabetes pathophysiology and treatment (71). In addition, aortic, coronary, brain, and thyroid functions were also strongly associated with diabetes (72–74).
In comparison with previous GWAS meta-analysis of diabetes conducted in European population, the current study was able to identify several novel loci with a relatively small sample size. Such findings highlight the importance of examining novel disease phenotype (prediabetes status change) and conducting gene-based analysis to identify genomic mechanisms of diabetes risk. Furthermore, these findings contribute to understanding the mechanisms of diabetes development. Sequencing along with functional studies are needed to help delineate causal variants underlying the strong signals identified here. Finally, although it is not the aim of this current study, future research investigating the individual and overall contributions, such as polygenic risk scores, of previously reported genomic loci for diabetes phenotypes considering lifestyle behaviors and environmental exposures to the progression of prediabetes are warranted.
Our study represents the first GWAS of prediabetes status change conducted in participants of European ancestry. Additional study strengths included stringent quality control methods used in genotyping, genotype imputation, phenotypes, and measures of covariates for both the discovery and the replication stage samples. This can reduce errors in phenotype measures and increase statistical power in identifying both SNPs and genes underlying prediabetes status change. Furthermore, we used gene-based analysis to combine contributions of all variants in a gene. As noted above, this approach is more powerful than single SNP-based analysis (30, 31). More importantly, the longitudinal nature of the current study provided robust evidence that genetic factors play important roles in prediabetes status change. Findings from the current study may help to identify patients with prediabetes who were more likely to change their prediabetes status based on their genomic profiles and provided insight into the mechanisms underlying prediabetes status change.
There are also limitations for the study. First, due to limited sample size, we were not able to identify any single SNP associated with prediabetes status change. Future large-scale genomic studies among persons with prediabetes are warranted to robustly identify additional loci underlying prediabetes status change and to identify SNPs within genes reported in the current study. Second, In addition, the study findings may not be generalized to participants with prediabetes of other ancestry. Similar studies are needed to identify and map the relevant genes and SNPs involved in prediabetes status change in other populations. Third, the reason we compared participants who progressed to diabetes with those who reverted to normoglycemia mainly due to concerns of statistical power. We think if there are genetic variants involved in prediabetes status change, difference of the variants will be more prominent between the two groups, and therefore, we had higher statistical power to detect such variants. However, the genes for progression to diabetes and reversion to normoglycemia could be different, and these genes were not identified in this analysis, but is the goal of ongoing analyses. Finally, functions of the identified genes associated with prediabetes progression need to be investigated in cell lines and/or animal models to delineate their roles in diabetes development.
In conclusion, we conducted the first GWAS of prediabetes status change among participants of European ancestry using both single SNP-based and gene-based analyses. We robustly identified 5 novel genes associated with prediabetes status change through powerful gene-based analysis. The 5 genes are biologically relevant to diabetes and glucose regulation and warrant further investigations. Due to limited sample size, we were not able to identify any locus associated with prediabetes status change in the single SNP-based analysis. Future large-scale genomic studies among patients with prediabetes are warranted.
The data presented in the study are deposited in the database of Genotypes and Phenotypes (dbGaP) repository, accession number phs000090.v5.p1 for the ARIC study and phs000342.v20.p13 for the Framingham Heart Study.
The studies involving human participants were reviewed and approved by Tulane University IRB. The ethics committee waived the requirement of written informed consent for participation.
TL, HL, and CL substantial contributed to conception and design. YC, BP, and JW contributed to acquisition of data. W-JL and CL contributed to analysis and interpretation of data. TL and HL contributed to draft the article. All authors contributed to the article and approved the submitted version.
This study is funded by the National Institutes of Health (1P20GM109036-01A1) awarded to Changwei Li.
The authors declare that the research was conducted in the absence of any commercial or financial relationships that could be construed as a potential conflict of interest.
All claims expressed in this article are solely those of the authors and do not necessarily represent those of their affiliated organizations, or those of the publisher, the editors and the reviewers. Any product that may be evaluated in this article, or claim that may be made by its manufacturer, is not guaranteed or endorsed by the publisher.
2. Centers for Disease Control and Prevention. National Diabetes Statistics Report, 2020. Atlanta, GA: Centers for Disease Control and Prevention, US Department of Health and Human Services. (2020).
3. Hales CM, Carroll MD, Fryar CD, Ogden CL. Prevalence of Obesity and Severe Obesity Among Adults: United States, 2017-2018. NCHS Data Brief (2020) 360):1–8.
4. American Diabetes Association. Economic Costs of Diabetes in the U.S. @ in 2017. Diabetes Care (2018) 41(5):917–28. doi: 10.2337/dci18-0007
5. DeFronzo RA, Tripathy D, Schwenke DC, Banerji M, Bray GA, Buchanan TA, et al. Pioglitazone for Diabetes Prevention in Impaired Glucose Tolerance. N Engl J Med (2011) 364(12):1104–15. doi: 10.1056/NEJMoa1010949
6. Pan XR, Li GW, Hu YH, Wang JX, Yang WY, An ZX, et al. Effects of Diet and Exercise in Preventing NIDDM in People With Impaired Glucose Tolerance. The Da Qing IGT and Diabetes Study. Diabetes Care (1997) 20(4):537–44. doi: 10.2337/diacare.20.4.537
7. Knowler WC, Barrett-Connor E, Fowler SE, Hamman RF, Lachin JM, Walker EA, et al. Reduction in the Incidence of Type 2 Diabetes With Lifestyle Intervention or Metformin. New Engl J Med (2002) 346:393–403. doi: 10.1056/NEJMoa012512
8. Tuomilehto J, Lindström J, Eriksson JG, Hämäläinen H, Ilanne-Parikka P, Keinänen-Kiukaanniemi S, et al. Prevention of Type 2 Diabetes Mellitus by Changes in Lifestyle Among Subjects With Impaired Glucose Tolerance. New Engl J Med (2001) 344:1343–50. doi: 10.1056/NEJM200105033441801
9. Willemsen G, Ward KJ, Bell CG, Christensen K, Bowden J, Dalgård C, et al. The Concordance and Heritability of Type 2 Diabetes in 34,166 Twin Pairs From International Twin Registers: The Discordant Twin (DISCOTWIN) Consortium. Twin Res Hum Genet (2015) 18(6):762–71. doi: 10.1017/thg.2015.83
10. Imamura M, Takahashi A, Yamauchi T, Hara K, Yasuda K, Grarup N, et al. Genome-Wide Association Studies in the Japanese Population Identify Seven Novel Loci for Type 2 Diabetes. Nat Commun (2016) 7:10531. doi: 10.1038/ncomms10531
11. Fuchsberger C, Flannick J, Teslovich TM, Mahajan A, Agarwala V, Gaulton KJ, et al. The Genetic Architecture of Type 2 Diabetes. Nature (2016) 536(7614):41–7. doi: 10.1038/nature18642
12. Gaulton KJ, Ferreira T, Lee Y, Raimondo A, Mägi R, Reschen ME, et al. Genetic Fine Mapping and Genomic Annotation Defines Causal Mechanisms at Type 2 Diabetes Susceptibility Loci. Nat Genet (2015) 47(12):1415–25. doi: 10.1038/ng.3437
13. Liu JZ, van Sommeren S, Huang H, Ng SC, Alberts R, Takahashi A, et al. Association Analyses Identify 38 Susceptibility Loci for Inflammatory Bowel Disease and Highlight Shared Genetic Risk Across Populations. Nat Genet (2015) 47(9):979–86. doi: 10.1038/ng.3359
14. Morris AP, Voight BF, Teslovich TM, Ferreira T, Segrè AV, Steinthorsdottir V, et al. Large-Scale Association Analysis Provides Insights Into the Genetic Architecture and Pathophysiology of Type 2 Diabetes. Nat Genet (2012) 44(9):981–90. doi: 10.1038/ng.2383
15. Lin L, Fang T, Ou Q, Zhang H, Chen K, Quan H. Genetic Variants Relate to Fasting Plasma Glucose, 2-Hour Postprandial Glucose, Glycosylated Hemoglobin, and BMI in Prediabetes. Front Endocrinol (Lausanne) (2022) 13:778069. doi: 10.3389/fendo.2022.778069
16. Choi JW, Moon S, Jang EJ, Lee CH, Park JS. Association of Prediabetes-Associated Single Nucleotide Polymorphisms With Microalbuminuria. PloS One (2017) 12(2):e0171367. doi: 10.1371/journal.pone.0171367
17. ARIC Investigators. The Atherosclerosis Risk in Communities (ARIC) Study: Design and Objectives. The ARIC Investigators. Am J Epidemiol (1989) 129(4):687–702. doi: 10.1093/oxfordjournals.aje.a115184
18. Fretz A, Schneider AL, McEvoy JW, Hoogeveen R, Ballantyne CM, Coresh J, et al. The Association of Socioeconomic Status With Subclinical Myocardial Damage, Incident Cardiovascular Events, and Mortality in the ARIC Study. Am J Epidemiol (2016) 183(5):452–61. doi: 10.1093/aje/kwv253
19. Auton A, Brooks LD, Durbin RM, Garrison EP, Kang HM, Korbel JO, et al. A Global Reference for Human Genetic Variation. Nature (2015) 526(7571):68–74. doi: 10.1038/nature15393
20. Das S, Forer L, Schönherr S, Sidore C, Locke AE, Kwong A, et al. Next-Generation Genotype Imputation Service and Methods. Nat Genet (2016) 48(10):1284–7. doi: 10.1038/ng.3656
21. Draznin B, Aroda VR, Bakris G, Benson G, Brown FM, Freeman R, et al. 2. Classification and Diagnosis of Diabetes: Standards of Medical Care in Diabetes-2022. Diabetes Care (2022) 45(Supplement_1):S17–38. doi: 10.2337/dc22-S002
22. GENEVA ARIC Project. Quality Control Report for the ARIC GWAS Database. Bethesda, MD: The National Institutes of Health Database of Genotypes and Phenotypes (dbGaP) (2009). dbGaP accession No. phs000090.v1.p1 ed.
23. Patterson N, Price AL, Reich D. Population Structure and Eigenanalysis. PloS Genet (2006) 2(12):e190. doi: 10.1371/journal.pgen.0020190
24. Demerath EW, Guan W, Grove ML, Aslibekyan S, Mendelson M, Zhou YH, et al. Epigenome-Wide Association Study (EWAS) of BMI, BMI Change and Waist Circumference in African American Adults Identifies Multiple Replicated Loci. Hum Mol Genet (2015) 24(15):4464–79. doi: 10.1093/hmg/ddv161
25. de Vries PS, Sabater-Lleal M, Huffman JE, Marten J, Song C, Pankratz N, et al. A Genome-Wide Association Study Identifies New Loci for Factor VII and Implicates Factor VII in Ischemic Stroke Etiology. Blood (2010) 133(9):967–77. doi: 10.1182/blood-2018-05-849240
26. Willer CJ, Li Y, Abecasis GR. METAL: Fast and Efficient Meta-Analysis of Genomewide Association Scans. Bioinformatics (2010) 26(17):2190–1. doi: 10.1093/bioinformatics/btq340
27. Li C, Yang X, He J, Hixson JE, Gu D, Rao DC, et al. A Gene-Based Analysis of Variants in the Serum/Glucocorticoid Regulated Kinase (SGK) Genes With Blood Pressure Responses to Sodium Intake: The GenSalt Study. PloS One (2014) 9(5):e98432. doi: 10.1371/journal.pone.0098432
28. Li C, He J, Chen J, Zhao J, Gu D, Hixson JE, et al. Genome-Wide Gene-Sodium Interaction Analyses on Blood Pressure: The Genetic Epidemiology Network of Salt-Sensitivity Study. Hypertension (2016) 68(2):348–55. doi: 10.1161/HYPERTENSIONAHA.115.06765
29. Li C, Kim YK, Dorajoo R, Li H, Lee IT, Cheng CY, et al. Genome-Wide Association Study Meta-Analysis of Long-Term Average Blood Pressure in East Asians. Circ Cardiovasc Genet (2017) 10(2):e001527. doi: 10.1161/CIRCGENETICS.116.001527
30. Li M-X, Jiang L, Li J. KGG: A Systematic Biological Knowledge-Based Mining System for Genome-Wide Genetic Studies. (2017).
31. Li M, Jiang L, Mak TSH, Kwan JSH, Xue C, Chen P, et al. A Powerful Conditional Gene-Based Association Approach Implicated Functionally Important Genes for Schizophrenia. Bioinformatics (2019) 35(4):628–35. doi: 10.1093/bioinformatics/bty682
32. Borenstein M, Hedges LV, Higgins JPT, Rothstein HR. Introduction to Meta-Analysis. 2nd ed. Hoboken, NJ: Wiley (2009).
33. GTEx Consortium. The Genotype-Tissue Expression (GTEx) Project. Nat Genet (2013) 45(6):580–5. doi: 10.1038/ng.2653
34. GTEx Consortium. Human Genomics. The Genotype-Tissue Expression (GTEx) Pilot Analysis: Multitissue Gene Regulation in Humans. Science (2015) 348(6235):648–60. doi: 10.1126/science.1262110
35. Bradfield JP, Qu HQ, Wang K, Zhang H, Sleiman PM, Kim CE, et al. A Genome-Wide Meta-Analysis of Six Type 1 Diabetes Cohorts Identifies Multiple Associated Loci. PloS Genet (2011) 7(9):e1002293. doi: 10.1371/journal.pgen.1002293
36. Parra EJ, Below JE, Krithika S, Valladares A, Barta JL, Cox NJ, et al. Genome-Wide Association Study of Type 2 Diabetes in a Sample From Mexico City and a Meta-Analysis of a Mexican-American Sample From Starr County, Texas. Diabetologia (2011) 54(8):2038–46. doi: 10.1007/s00125-011-2172-y
37. Gerhard DS, Wagner L, Feingold EA, Shenmen CM, Grouse LH, Schuler G, et al. The Status, Quality, and Expansion of the NIH Full-Length cDNA Project: The Mammalian Gene Collection (MGC). Genome Res (2004) 14(10B):2121–7. doi: 10.1101/gr.2596504
38. Locke AE, Kahali B, Berndt SI, Justice AE, Pers TH, Day FR, et al. Genetic Studies of Body Mass Index Yield New Insights for Obesity Biology. Nature (2015) 518(7538):197–206. doi: 10.1038/nature14177
39. Comuzzie AG, Cole SA, Laston SL, Voruganti VS, Haack K, Gibbs RA, et al. Novel Genetic Loci Identified for the Pathophysiology of Childhood Obesity in the Hispanic Population. PloS One (2012) 7(12):e51954. doi: 10.1371/journal.pone.0051954
40. Nedeltcheva AV, Scheer FA. Metabolic Effects of Sleep Disruption, Links to Obesity and Diabetes. Curr Opin Endocrinol Diabetes Obes (2014) 21(4):293–8. doi: 10.1097/MED.0000000000000082
41. Saxena R, Saleheen D, Been LF, Garavito ML, Braun T, Bjonnes A, et al. Genome-Wide Association Study Identifies a Novel Locus Contributing to Type 2 Diabetes Susceptibility in Sikhs of Punjabi Origin From India. Diabetes (2013) 62(5):1746–55. doi: 10.2337/db12-1077
42. Groh S, Zong H, Goddeeris MM, Lebakken CS, Venzke D, Pessin JE, et al. Sarcoglycan Complex: Implications for Metabolic Defects in Muscular Dystrophies. J Biol Chem (2009) 284(29):19178–82. doi: 10.1074/jbc.C109.010728
43. McKenzie E, Tyson K, Stamps A, Smith P, Turner P, Barry R, et al. Cloning and Expression Profiling of Hpa2, a Novel Mammalian Heparanase Family Member. Biochem Biophys Res Commun (2000) 276(3):1170–7. doi: 10.1006/bbrc.2000.3586
44. Gil N, Goldberg R, Neuman T, Garsen M, Zcharia E, Rubinstein AM, et al. Heparanase is Essential for the Development of Diabetic Nephropathy in Mice. Diabetes (2012) 61(1):208–16. doi: 10.2337/db11-1024
45. Ziolkowski AF, Popp SK, Freeman C, Parish CR, Simeonovic CJ. Heparan Sulfate and Heparanase Play Key Roles in Mouse β Cell Survival and Autoimmune Diabetes. J Clin Invest (2012) 122(1):132–41. doi: 10.1172/JCI46177
46. Wang F, Wan A, Rodrigues B. The Function of Heparanase in Diabetes and its Complications. Can J Diabetes (2013) 37(5):332–8. doi: 10.1016/j.jcjd.2013.05.008
47. Shafat I, Ilan N, Zoabi S, Vlodavsky I, Nakhoul F. Heparanase Levels are Elevated in the Urine and Plasma of Type 2 Diabetes Patients and Associate With Blood Glucose Levels. PloS One (2011) 6(2):e17312. doi: 10.1371/journal.pone.0017312
48. Goldberg R, Rubinstein AM, Gil N, Hermano E, Li JP, van der Vlag J, et al. Role of Heparanase-Driven Inflammatory Cascade in Pathogenesis of Diabetic Nephropathy. Diabetes (2014) 63(12):4302–13. doi: 10.2337/db14-0001
49. Bjarnadóttir TK, Geirardsdóttir K, Ingemansson M, Mirza MA, Fredriksson R, Schiöth HB. Identification of Novel Splice Variants of Adhesion G Protein-Coupled Receptors. Gene (2007) 387(1-2):38–48. doi: 10.1016/j.gene.2006.07.039
50. Yang J, Villar VA, Armando I, Jose PA, Zeng C. G Protein-Coupled Receptor Kinases: Crucial Regulators of Blood Pressure. J Am Heart Assoc (2016) 5(7):e003519. doi: 10.1161/JAHA.116.003519
51. Kavelaars A, Vroon A, Raatgever RP, Fong AM, Premont RT, Patel DD, et al. Increased Acute Inflammation, Leukotriene B4-Induced Chemotaxis, and Signaling in Mice Deficient for G Protein-Coupled Receptor Kinase 6. J Immunol (2003) 171(11):6128–34. doi: 10.4049/jimmunol.171.11.6128
52. Pocai A, Carrington PE, Adams JR, Wright M, Eiermann G, Zhu L, et al. Glucagon-Like Peptide 1/Glucagon Receptor Dual Agonism Reverses Obesity in Mice. Diabetes (2009) 58(10):2258–66. doi: 10.2337/db09-0278
53. Latronico AC, Hochberg Z. G Protein-Coupled Receptors in Child Development, Growth, and Maturation. Sci Signal (2010) 3(143):re7. doi: 10.1126/scisignal.3143re7
54. Reimann F, Gribble FM. G Protein-Coupled Receptors as New Therapeutic Targets for Type 2 Diabetes. Diabetologia (2016) 59(2):229–33. doi: 10.1007/s00125-015-3825-z
55. Taylor TD, Noguchi H, Totoki Y, Toyoda A, Kuroki Y, Dewar K, et al. Human Chromosome 11 DNA Sequence and Analysis Including Novel Gene Identification. Nature (2006) 440(7083):497–500. doi: 10.1038/nature04632
56. Le Carré J, Schorderet DF, Cottet S. Altered Expression of β-Galactosidase-1-Like Protein 3 (Glb1l3) in the Retinal Pigment Epithelium (RPE)-Specific 65-kDa Protein Knock-Out Mouse Model of Leber's Congenital Amaurosis. Mol Vis (2011) 17:1287–97.
57. Kara I, Poggi M, Bonardo B, Govers R, Landrier JF, Tian S, et al. The Paired Basic Amino Acid-Cleaving Enzyme 4 (PACE4) is Involved in the Maturation of Insulin Receptor Isoform B: An Opportunity to Reduce the Specific Insulin Receptor-Dependent Effects of Insulin-Like Growth Factor 2 (IGF2). J Biol Chem (2015) 290(5):2812–21. doi: 10.1074/jbc.M114.592543
58. Jin W, Wang X, Millar JS, Quertermous T, Rothblat GH, Glick JM, et al. Hepatic Proprotein Convertases Modulate HDL Metabolism. Cell Metab (2007) 6(2):129–36. doi: 10.1016/j.cmet.2007.07.009
59. Scerri TS, Brandler WM, Paracchini S, Morris AP, Ring SM, Richardson AJ, et al. PCSK6 is Associated With Handedness in Individuals With Dyslexia. Hum Mol Genet (2011) 20(3):608–14. doi: 10.1093/hmg/ddq475
60. Brandler WM, Morris AP, Evans DM, Scerri TS, Kemp JP, Timpson NJ, et al. Common Variants in Left/Right Asymmetry Genes and Pathways are Associated With Relative Hand Skill. PloS Genet (2013) 9(9):e1003751. doi: 10.1371/journal.pgen.1003751
61. Bonnet F, Affret A, Boutron-Ruault MC, Balkau B, Clavel-Chapelon F, Fagherazzi G. Association Between Handedness and Type 2 Diabetes: The E3N Study. Diabetes Care (2015) 38(12):e199. doi: 10.2337/dc15-1669
62. Chen S, Cao P, Dong N, Peng J, Zhang C, Wang H, et al. PCSK6-Mediated Corin Activation is Essential for Normal Blood Pressure. Nat Med (2015) 21(9):1048–53. doi: 10.1038/nm.3920
63. Liu Y, Peng H, Zhang Q, Zhang P, Tian Y, Chao X, et al. Association Between Serum Soluble Corin and Hyperglycaemia: A Cross-Sectional Study Among Chinese Adults. BMJ Open (2015) 5(12):e009085. doi: 10.1136/bmjopen-2015-009085
64. Guglielmi V, Cardellini M, Cinti F, Corgosinho F, Cardolini I, D'Adamo M, et al. Omental Adipose Tissue Fibrosis and Insulin Resistance in Severe Obesity. Nutr Diabetes (2015) 5:e175. doi: 10.1038/nutd.2015.22
65. WM BALFOUR, RG SPRAGUE. Association of Diabetes Mellitus and Disorders of the Anterior Pituitary, Thyroid and Adrenal Cortex. Am J Med (1949) 7(5):596–608. doi: 10.1016/0002-9343(49)90381-2
66. Lizcano JM, Göransson O, Toth R, Deak M, Morrice NA, Boudeau J, et al. LKB1 is a Master Kinase That Activates 13 Kinases of the AMPK Subfamily, Including MARK/PAR-1. EMBO J (2004) 23(4):833–43. doi: 10.1038/sj.emboj.7600110
67. Uebi T, Itoh Y, Hatano O, Kumagai A, Sanosaka M, Sasaki T, et al. Involvement of SIK3 in Glucose and Lipid Homeostasis in Mice. PloS One (2012) 7(5):e37803. doi: 10.1371/journal.pone.0037803
68. Säll J, Pettersson AM, Björk C, Henriksson E, Wasserstrom S, Linder W, et al. Salt-Inducible Kinase 2 and -3 are Downregulated in Adipose Tissue From Obese or Insulin-Resistant Individuals: Implications for Insulin Signalling and Glucose Uptake in Human Adipocytes. Diabetologia (2017) 60(2):314–23. doi: 10.1007/s00125-016-4141-y
69. Iyer PG, Borah BJ, Heien HC, Das A, Cooper GS, Chak A. Association of Barrett's Esophagus With Type II Diabetes Mellitus: Results From a Large Population-Based Case-Control Study. Clin Gastroenterol Hepatol (2013) 11(9):1108–14.e5. doi: 10.1016/j.cgh.2013.03.024
70. Riazi S, Bril V, Perkins BA, Abbas S, Chan VW, Ngo M, et al. Can Ultrasound of the Tibial Nerve Detect Diabetic Peripheral Neuropathy? A Cross-Sectional Study. Diabetes Care (2012) 35(12):2575–9. doi: 10.2337/dc12-0739
71. Frayn KN, Tan GD, Karpe F. Adipose Tissue: A Key Target for Diabetes Pathophysiology and Treatment? Horm Metab Res (2007) 39(10):739–42. doi: 10.1055/s-2007-990270
72. Tanaka A, Ishii H, Oshima H, Narita Y, Kodama A, Suzuki S, et al. Inverse Association Between Diabetes and Aortic Dilatation in Patients With Advanced Coronary Artery Disease. Atherosclerosis (2015) 242(1):123–7. doi: 10.1016/j.atherosclerosis.2015.07.016
73. Seaquist ER. The Final Frontier: How Does Diabetes Affect the Brain? Diabetes (2010) 59(1):4–5. doi: 10.2337/db09-1600
Keywords: prediabetes status change, genome-wide association study, diabetes mellitus, type 2, normoglycemia
Citation: Liu T, Li H, Conley YP, Primack BA, Wang J, Lo W-J and Li C (2022) A Genome-Wide Association Study of Prediabetes Status Change. Front. Endocrinol. 13:881633. doi: 10.3389/fendo.2022.881633
Received: 22 February 2022; Accepted: 29 April 2022;
Published: 13 June 2022.
Edited by:
Ping Wang, Michigan State University, United StatesCopyright © 2022 Liu, Li, Conley, Primack, Wang, Lo and Li. This is an open-access article distributed under the terms of the Creative Commons Attribution License (CC BY). The use, distribution or reproduction in other forums is permitted, provided the original author(s) and the copyright owner(s) are credited and that the original publication in this journal is cited, in accordance with accepted academic practice. No use, distribution or reproduction is permitted which does not comply with these terms.
*Correspondence: Changwei Li, Y2xpOEB0dWxhbmUuZWR1
Disclaimer: All claims expressed in this article are solely those of the authors and do not necessarily represent those of their affiliated organizations, or those of the publisher, the editors and the reviewers. Any product that may be evaluated in this article or claim that may be made by its manufacturer is not guaranteed or endorsed by the publisher.
Research integrity at Frontiers
Learn more about the work of our research integrity team to safeguard the quality of each article we publish.