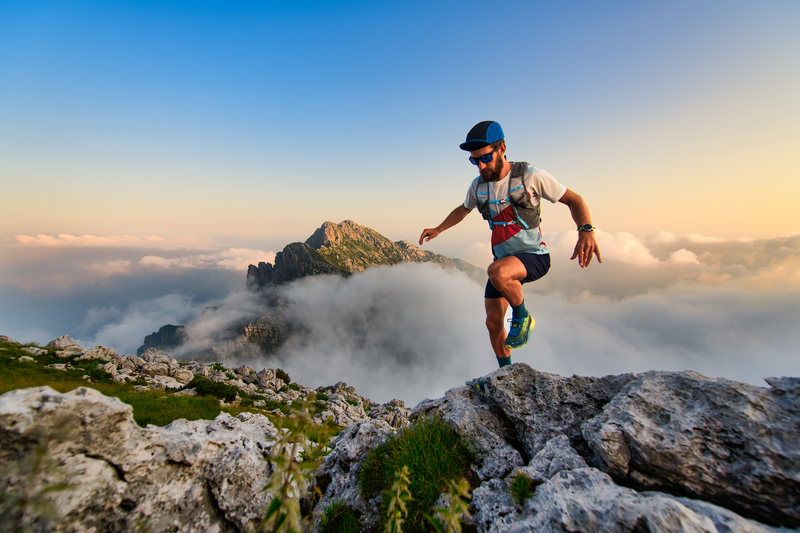
95% of researchers rate our articles as excellent or good
Learn more about the work of our research integrity team to safeguard the quality of each article we publish.
Find out more
ORIGINAL RESEARCH article
Front. Endocrinol. , 16 May 2022
Sec. Obesity
Volume 13 - 2022 | https://doi.org/10.3389/fendo.2022.881093
This article is part of the Research Topic Association of Novel Anthropometric Indexes with Metabolic Syndrome View all 15 articles
Aim: We aimed to investigate the association between obesity-related parameters and polyneuropathy (PN) and to evaluate inflammatory and fibrotic gene expression of fat as a potential mediator in subjects scheduled to undergo metabolic bariatric surgery (MBS).
Methods: This was a cross-sectional study of MBS cohort. Body composition and visceral fat area (VFA) were quantified by bioimpedance analysis and computed tomography scan. PN was defined by Michigan Neuropathy Screening Instrument–Physical Examination score was > 2. We measured mRNA expression level of FN1, TIMP1, CCL2, and CXCL8 in omental fat tissue.
Results: Of 189 subjects (mean age, 39.4 years; 69 [36.5%] male; mean body mass index, 38.5 kg/m2), prevalence of PN was 9.1% in subjects without diabetes (n = 110) and 20.3% in those with diabetes (n = 79). Nondiabetic subjects with PN had higher homeostatic model assessment-insulin resistance (6.8 ± 3.5 vs 4.5 ± 2.8, p = 0.041), and increased fat mass (58.5 ± 12.5 kg vs 50.5 ± 10.7 kg, p = 0.034), and VFA (309.4 ± 117.6 cm2 vs 243.5 ± 94.2 cm2, p = 0.046) compared to those without PN. These obesity-related parameters were significantly associated with the presence of PN after adjusting for conventional risk factors of PN only in subjects without diabetes. In contrast, a fibrotic gene such as TIMP1 was independently associated with PN (adjusted odds ratio of 1.56; 95% confidence interval 1.06, 2.30) only in subjects with diabetes.
Conclusion: Increased adiposity was independently associated with PN in obese subjects without diabetes. In contrast, this association was not significant after adjusting conventional risk factors of PN in obese subjects with diabetes but increased fibrotic gene expression in fat was associated with PN in this group.
Polyneuropathy (PN), one of the most common types of peripheral neuropathy, is prevalent in subjects with diabetes and even in those with prediabetes. While increased duration of diabetes and poor glycemic control are established risk factors for diabetic PN (1, 2), intensive glycemic control shows limited efficacy in prevention of PN in type 2 diabetes (3). This suggests that risk factors other than glucose, such as the components of metabolic syndrome, might contribute to nerve damage to a considerable extent.
The Cooperative Health Research in the Region of Augsburg (KORA) study (4) and the Anglo–Danish–Dutch study of Intensive Treatment in People with Screen-Detected Diabetes in Primary Care (ADDITION) study (5) revealed an association between general and abdominal obesity (weight and waist circumference) and development of PN. Besides the amount of fat, the quality of the fat tissue could induce detrimental effects on the metabolic milieu. Considering that chronic adipose tissue inflammation was associated with obesity-related metabolic complications (6) and adipose tissue is the main site where systemic inflammation begins (7), it is logically reasonable to investigate an association of the expression level of inflammatory and fibrosis genes in fat tissue with PN.
Meanwhile, a previous observational study showed that 11.1% of obese subjects already had PN despite normoglycemia, and that the presence of PN is positively associated with waist circumference (8). This suggests that the association between obesity and PN needs to be analyzed in a subgroup stratified by diabetes status.
Therefore, in this study, we aimed to investigate the association between obesity-related parameters and PN in obese subjects stratified by diabetes status. We used computed tomography scan to assess the quantity of VAT, and analyzed mRNA levels of inflammatory and fibrosis genes such as FN1 (9), TIMP1 (10), CCL2 (11), and CXCL8 (12) from omental fat tissue. These genes have been studies as a driver of systemic inflammation and insulin resistance.
We recruited subjects scheduled to undergo metabolic bariatric surgery (MBS) and intensively evaluated metabolic parameters and the presence of diabetic vascular complications. A total of 205 obese subjects were enrolled from Seoul National University Bundang Hospital (SNUBH), a tertiary academic hospital from April 2019 to December 2020, the aim of original prospective observational study is to discover predictive markers for weight loss and metabolic improvement after MBS. The original cohort study has been registered at Clinical research Information Service (CRIS Registration No. KCT0005777). Inclusion criteria were age ≥ 20 years old and a body mass index (BMI) ≥ 35 kg/m2 with no comorbidity; BMI ≥ 30 kg/m2 with at least one comorbidity; or BMI ≥ 27.5 kg/m2 with medically uncontrolled type 2 diabetes. Type 2 diabetes was defined according to the criteria of American Diabetes Association: fasting plasma glucose (FPG) ≥ 7.0 mmol/l or HbA1c ≥ 6.5% (48 mmol/mol) (13). Subjects on diabetes medications were also considered to have diabetes. We excluded the subjects who did not meet the indication of MBS according to the local guideline (14). We also excluded the subjects who previously underwent MBS. This study was a cross-sectional study analyzing data of 189 participants (Figure 1) after excluding subjects with missing information of PN (n = 16). We applied 2:1 propensity score matching using age, sex, and BMI to compare mRNA expression levels in omental fat tissue between subjects without PN and those with PN. However, values were excluded in analysis if quality of cDNA sample was unacceptable. The study was approved by the Institutional Review Board of SNUBH (no. B-2111-718-301), and each participant provided written informed consent. The study was conducted in accordance with the Declaration of Helsinki.
Figure 1 Flow chart of the selection of subjects for the analysis. Among 205 obese subjects, after excluding subjects with missing information of polyneuropathy, 189 subjects were included in the final analysis. They were divided into two groups according to diabetes status.
Anthropometric indices were measured by a well-trained research nurse. BMI was calculated as weight (kg) divided by the square of the height in meters. Waist circumference was measured at the midpoint between the margin of the lowest rib and the iliac crest. Systolic blood pressure (BP) and diastolic BP were measured by an electronic BP monitor after 10 minutes of rest in a sitting position. Smoking status was classified as never smoker (< 100 cigarettes in a lifetime and currently a nonsmoker), ex-smoker (≥ 100 cigarettes in a lifetime and currently a nonsmoker), and current smoker (≥ 100 cigarettes in a lifetime and currently a smoker). We defined drinkers as those who drank any alcoholic beverage more than once a month. Positive exercise was defined as exercising for > 150 min/week. Blood samples were collected after an overnight fast. FPG levels were measured by the hexokinase method, and glycated hemoglobin (HbA1c) levels were measured by high-performance liquid chromatography (Bio-Rad, Hercules, CA, USA). Serum insulin levels were measured by immunoradiometric assay (DIAsource, Nivelles, Belgium). Total cholesterol, triglyceride, high-density lipoprotein (HDL) cholesterol, and low-density lipoprotein (LDL) cholesterol were measured by enzymatic colorimetric assay. Creatinine was measured by the protocol of the central laboratory of SNUBH, and estimated glomerular filtration rate (eGFR) was calculated by the Modification of Diet in Renal Disease equation (15). Homeostasis model assessment-insulin resistance (HOMA-IR) was calculated using the following formula: HOMA-IR = (fasting insulin [μU/ml] × FPG [mmol/l]/22.5) (16).
Body fat mass, fat percent, and lean body mass (LBM) were estimated by a bioimpedance analysis (InBody770, InBody, Seoul, Korea). Regarding the visceral fat area (VFA), cross-sectional abdominal computed tomography (CT) images at the level of the third lumbar vertebral body (L3) were acquired, and VFA was calculated from areas within a range of –150 to –50 Hounsfield units (17).
We used the Michigan Neuropathy Screening Instrument (MNSI), which includes two separate assessments, a 15-item self-administered questionnaire (MNSI-Q) and a lower-extremity physical examination (MNSI-PE) (18). PN was diagnosed when the MNSI-PE score was > 2. A trained nurse performed all neurologic examinations.
Omental fat tissue was obtained during MBS and stored at –80°C. Total RNA was extracted from frozen human fat tissue samples using TRIzol (Thermo Fisher Scientific, Waltham, MA, USA). For quantitative real-time PCR analysis, 1 μg of total RNA was reverse-transcribed using the High-Capacity cDNA Reverse Transcription kit (Thermo Fisher Scientific, Waltham, MA, USA). SYBR Green reactions using the SYBR Green PCR Master mix (Enzynomics, Daejeon, Korea) were assembled along with primers according to the manufacturer’s instructions and were performed using the QuantStudio 7 Flex Real-Time PCR System (Thermo Fisher Scientific, Waltham, MA, USA). Relative mRNA levels were calculated using the comparative threshold cycle method and normalized to cyclophilin mRNA. All primers used are listed with their sequences in Supplementary Table 1.
Data were expressed as the mean ± standard deviation or number (%). For checking normality of distribution of variables, Kolmogorov-Smirnov and Shapiro-Wilk tests were used. Categorical variables were compared using χ2 tests, and continuous variables were compared using Student’s unpaired t tests for parametric data or Mann–Whitney U tests for nonparametric data. Since variables were not normally distributed, Spearman’s correlation coefficient was used to evaluate the correlation between variables. Univariable and multivariable logistic regression models were used to analyze the associations between obesity-related parameters and PN. In all cases, p < 0.05 was considered statistically significant. Statistical analyses were performed using IBM SPSS version 25.0 (IBM Inc., Armonk, NY, USA). Figures were drawn using GraphPad Prism software (version 9.1.2; GraphPad Software Inc., CA, USA).
Among 189 subjects who were candidates for MBS, 79 (41.8%) had diabetes. Prevalence of PN was 9.1% in subjects without diabetes and 20.3% in those with diabetes. Among subjects without diabetes, insulin and HOMA-IR were higher in subjects with PN compared with those without PN. Among subjects with diabetes, body weight, BMI, waist circumference, eGFR, and the proportion of subjects taking insulin therapy were higher in subjects with PN compared with those without PN. However, diabetes duration, FPG, and HbA1c were comparable between subjects with PN and those without PN (Table 1). Body composition analysis showed that fat mass and VFA were higher in subjects with PN compared with those without PN, irrespective of diabetes status. Among subjects with diabetes, LBM was higher in subjects with PN compared with those without PN (Table 1).
Among subjects without diabetes, unadjusted odds ratio (OR) (95% confidence interval [CI]) for PN was 1.23 (1.02, 1.49) for HOMA-IR and 1.06 (1.00, 1.12) for fat mass. Among subjects with diabetes, unadjusted OR (95% CI) for PN was 1.05 (1.01, 1.09) for waist circumference, 1.13 (1.03, 1.23) for BMI, 1.04 (1.01, 1.08) for fat mass, and 1.01 (1.00, 1.02) for VFA. After adjustment of known risk factors for PN (19), adjusted OR (95% CI) for PN was 1.47 (1.10, 1.95) for HOMA-IR, 1.08 (1.00, 1.16) for fat mass, and 1.01 (1.00, 1.02) for VFA in subjects without diabetes. In contrast, waist circumference, BMI, fat mass, and VFA showed no significant association with PN in subjects with diabetes after adjustment of covariates (Table 2).
Among subjects without diabetes, any mRNA expression of omental fat was not different according to the presence of PN. In contrast, among subjects with diabetes, mRNA expression of TIMP1 and CXCL8 was significantly higher in subjects with PN (Figure 2), and positively correlated with MNSI-PE scores (rho = 0.469, p = 0.001; rho = 0.454, p = 0.002) (Figure 3). In addition, adjusted OR (95% CI) for PN was 1.56 (1.06, 2.30) for TIMP1 in subjects with diabetes (Table 2).
Figure 2 mRNA expression of inflammatory and fibrotic genes of omental fat tissue in subjects without diabetes (A) and those with diabetes (B). *p < 0.05.
Figure 3 Correlations between mRNA expression level of TIMP1 (A) or CXCL8 (B), and MNSI-PE scores in subjects with diabetes.
In this study, we found increased fat mass and VFA, and higher HOMA-IR in subjects with PN compared with those without PN among subjects without diabetes. These variables were still significantly associated with PN even after adjusting for known risk factors for PN in this group. On the other hand, among subjects with diabetes we found no difference in HOMA-IR between subjects with or without PN, and the association of fat mass and VFA with PN was not significant after adjusting covariates. In contrast to these insignificant associations, TIMP1 gene expression as a marker of fat fibrosis was significantly higher in the presence of PN in the diabetes subgroup.
Hyperglycemia is a major risk factor for PN (19), and glucose control is the mainstay of prevention and management of PN (20). However, enhanced glucose control modestly reduced (21), or did not reduce the incidence of PN in type 2 diabetes (22, 23). Therefore, it is necessary to identify other risk factors for PN in subjects with type 2 diabetes or at high risk for type 2 diabetes. From the data of obese subjects without diabetes, we confirmed that obesity is the main metabolic driver of PN. Furthermore, insulin resistance assessed by HOMA-IR was also an independent risk factor for PN in this population. Considering that insulin is a neurotrophic factor responsible for neuronal growth, survival, and differentiation (24, 25), it is possible that disruption of insulin signaling due to insulin resistance contributed to the pathogenesis of PN. However, the association between HOMA-IR and PN was not significant in subjects with overt diabetes, a finding that might be due to the strong influence of severe insulin resistance in these subjects who were candidates for MBS. Therefore, the impact of obesity on PN might depend on whether the severity of insulin resistance of subjects caused diabetes.
A prospective study of the general population with and without diabetes from the KORA cohort showed that serum levels of CCL7, CXCL10, and DNER partly mediated the association between obesity and PN (4). Another prospective study of subjects with diabetes showed an association of plasma levels of TNF and ICAM1 with development of PN (26). A cross-sectional study showed associations of plasma levels of MMP9 and TIMP1 with PN in type 1 diabetes (27). Considering that adipose tissue is the main site where systemic inflammation begins (7), it is reasonable to investigate an association of the expression level of inflammatory and fibrosis genes in fat tissue with PN. In our analysis, including subjects with diabetes, mRNA levels of TIMP1 and CXCL8 were significantly higher in subjects with PN compared with their counterparts. These results suggest the possibility that inflammatory cytokines released from VAT during tissue inflammation and fibrosis contribute to the pathogenesis of PN in diabetes. In contrast, no significant association between inflammatory gene expression in the omental fat and PN was found in the nondiabetic subgroup. Therefore, inflammation or fibrosis of fat tissue might contribute to PN differently according to diabetes status, however this hypothesis needs to be investigated in further study.
The current study has a number of strengths. First, we performed analysis of PN stratified by diabetes status, thereby suggesting the step-wise contribution of hyperglycemia and obesity involved in the pathogenesis of PN. Second, VFA was measured by CT scan, the gold standard method. Finally, we directly measured gene expression from omental fat tissue. To the best of our knowledge, this study is the first analysis combining gene expression of fat tissue and PN. Nevertheless, this study has some limitations. First, there were no lean controls, and we analyzed the mRNA data in the subgroup due to the availability of fat tissue. Second, a neurophysiological study was not used to confirm PN. Therefore, the diagnosis of PN is not confirmative, but possible PN was adopted. Third, we cannot establish a causal relationship based on the cross-sectional study design. Fourth, because the original cohort is based on a single center in Korea, results cannot be generalized to other races or ethnicities. Finally, we did not separate subjects with prediabetes from subjects without diabetes.
In conclusion, fat mass, VFA (“quantity of fat”), and HOMA-IR were independent risk factors for PN in obese subjects without diabetes. In contrast, advanced pathology of fat tissue such as fibrosis (“quality of fat”) might be a considerable abnormality for PN in obese subjects who already have diabetes. Therefore, the early evaluation of PN and the early management of obesity might be necessary in obese subjects at high risk of diabetes. Future studies are needed to test whether weight loss intervention can prevent and delay PN in these high-risk subjects.
The original contributions presented in the study are included in the article/Supplementary Material, further inquiries can be directed to the corresponding author.
The studies involving human participants were reviewed and approved by the Institutional Review Board of SNUBH. The patients/participants provided their written informed consent to participate in this study.
KK drafted the article. TJO, YSP, WC, SHC, and HCJ contributed to the conception and design of the study. KK performed the statistical analyses. KK, TJO, YSP, WC, HCC, JL, and YKL contributed to the acquisition of data. TJO is the guarantor of this work. All authors critically reviewed the manuscript and approved this version to be published.
This work was supported by the Korean Society for the Study of Obesity (Grant No. KSSO201904); the National Research Foundation of Korea (NRF) (Grant No. NRF-2020R1C1C1013766); the Medical Research Center through the NRF (Grant No. NRF-2018R1A5A2024425) funded by the Korea Government; and the SNUBH Research Fund (Grant No. 13-2019-0013).
The authors declare that the research was conducted in the absence of any commercial or financial relationships that could be construed as a potential conflict of interest.
All claims expressed in this article are solely those of the authors and do not necessarily represent those of their affiliated organizations, or those of the publisher, the editors and the reviewers. Any product that may be evaluated in this article, or claim that may be made by its manufacturer, is not guaranteed or endorsed by the publisher.
The authors sincerely thank all the participants for their cooperation. In addition, we thank Ms. Chae Won Kim for assistance.
The Supplementary Material for this article can be found online at: https://www.frontiersin.org/articles/10.3389/fendo.2022.881093/full#supplementary-material
ADDITION, Anglo–Danish–Dutch study of Intensive Treatment in People with Screen-Detected Diabetes in Primary Care; BMI, Body mass index; BP, Blood pressure; CI, confidence interval; CT, Computed tomography; DNER, Delta/Notch-like epidermal growth factor-related receptor; eGFR, Estimated glomerular filtration rate; FPG, Fasting plasma glucose; HbA1c, Glycated hemoglobin; HDL, High-density lipoprotein; HOMA-IR, Homeostasis model assessment-insulin resistance; KORA, Cooperative Health Research in the Region of Augsburg; LBM, Lean body mass; LDL, Low-density lipoprotein; MBS, Metabolic bariatric surgery; MNSI-PE, Michigan Neuropathy Screening Instrument-Physical Examination; MNSI-Q, Michigan Neuropathy Screening Instrument-Questionnaire; OR, odds ratio; PN, Polyneuropathy; SAT, Subcutaneous adipose tissue; SNUBH, Seoul National University Bundang Hospital; VAT, Visceral adipose tissue; VFA, Visceral fat area.
1. Adler AI, Boyko EJ, Ahroni JH, Stensel V, Forsberg RC, Smith DG. Risk Factors for Diabetic Peripheral Sensory Neuropathy. Results of the Seattle Prospective Diabetic Foot Study. Diabetes Care (1997) 20(7):1162–7. doi: 10.2337/diacare.20.7.1162
2. Yang H, Sloan G, Ye Y, Wang S, Duan B, Tesfaye S, et al. New Perspective in Diabetic Neuropathy: From the Periphery to the Brain, A Call for Early Detection, and Precision Medicine. Front Endocrinol (Lausanne) (2019) 10:929. doi: 10.3389/fendo.2019.00929
3. Callaghan BC, Little AA, Feldman EL, Hughes RA. Enhanced Glucose Control for Preventing and Treating Diabetic Neuropathy. Cochrane Database Syst Rev (2012), CD007543. doi: 10.1002/14651858.CD007543.pub2
4. Schlesinger S, Herder C, Kannenberg JM, Huth C, Carstensen-Kirberg M, Rathmann W, et al. General and Abdominal Obesity and Incident Distal Sensorimotor Polyneuropathy: Insights Into Inflammatory Biomarkers as Potential Mediators in the Kora F4/Ff4 Cohort. Diabetes Care (2019) 42(2):240–7. doi: 10.2337/dc18-1842
5. Andersen ST, Witte DR, Dalsgaard EM, Andersen H, Nawroth P, Fleming T, et al. Risk Factors for Incident Diabetic Polyneuropathy in a Cohort With Screen-Detected Type 2 Diabetes Followed for 13 Years: Addition-Denmark. Diabetes Care (2018) 41(5):1068–75. doi: 10.2337/dc17-2062
6. Zatterale F, Longo M, Naderi J, Raciti GA, Desiderio A, Miele C, et al. Chronic Adipose Tissue Inflammation Linking Obesity to Insulin Resistance and Type 2 Diabetes. Front Physiol (2019) 10:1607. doi: 10.3389/fphys.2019.01607
7. Xu H, Barnes GT, Yang Q, Tan G, Yang D, Chou CJ, et al. Chronic Inflammation in Fat Plays a Crucial Role in the Development of Obesity-Related Insulin Resistance. J Clin Invest (2003) 112(12):1821–30. doi: 10.1172/JCI19451
8. Callaghan BC, Xia R, Reynolds E, Banerjee M, Rothberg AE, Burant CF, et al. Association Between Metabolic Syndrome Components and Polyneuropathy in an Obese Population. JAMA Neurol (2016) 73(12):1468–76. doi: 10.1001/jamaneurol.2016.3745
9. Chun TH. Peri-Adipocyte Ecm Remodeling in Obesity and Adipose Tissue Fibrosis. Adipocyte (2012) 1(2):89–95. doi: 10.4161/adip.19752
10. Meissburger B, Stachorski L, Roder E, Rudofsky G, Wolfrum C. Tissue Inhibitor of Matrix Metalloproteinase 1 (Timp1) Controls Adipogenesis in Obesity in Mice and in Humans. Diabetologia (2011) 54(6):1468–79. doi: 10.1007/s00125-011-2093-9
11. Dahlman I, Kaaman M, Olsson T, Tan GD, Bickerton AS, Wahlen K, et al. A Unique Role of Monocyte Chemoattractant Protein 1 Among Chemokines in Adipose Tissue of Obese Subjects. J Clin Endocrinol Metab (2005) 90(10):5834–40. doi: 10.1210/jc.2005-0369
12. Kobashi C, Asamizu S, Ishiki M, Iwata M, Usui I, Yamazaki K, et al. Inhibitory Effect of Il-8 on Insulin Action in Human Adipocytes Via Map Kinase Pathway. J Inflammation (Lond) (2009) 6:25. doi: 10.1186/1476-9255-6-25
13. American Diabetes A. 2. Classification and Diagnosis of Diabetes: Standards of Medical Care in Diabetes-2021. Diabetes Care (2021) 44(Suppl 1):S15–33. doi: 10.2337/dc21-S002
14. Kim BY, Kang SM, Kang JH, Kang SY, Kim KK, Kim KB, et al. 2020 Korean Society for the Study of Obesity Guidelines for the Management of Obesity in Korea. J Obes Metab Syndr (2021) 30(2):81–92. doi: 10.7570/jomes21022
15. Levey AS, Coresh J, Greene T, Stevens LA, Zhang YL, Hendriksen S, et al. Using Standardized Serum Creatinine Values in the Modification of Diet in Renal Disease Study Equation for Estimating Glomerular Filtration Rate. Ann Intern Med (2006) 145(4):247–54. doi: 10.7326/0003-4819-145-4-200608150-00004
16. Matthews DR, Hosker JP, Rudenski AS, Naylor BA, Treacher DF, Turner RC. Homeostasis Model Assessment: Insulin Resistance and Beta-Cell Function From Fasting Plasma Glucose and Insulin Concentrations in Man. Diabetologia (1985) 28(7):412–9. doi: 10.1007/BF00280883
17. Lee JK, Park YS, Kim K, Oh TJ, Chang W. Comparison of Bioelectrical Impedance Analysis and Computed Tomography on Body Composition Changes Including Visceral Fat After Bariatric Surgery in Asian Patients With Obesity. Obes Surg (2021) 31(10):4243–50. doi: 10.1007/s11695-021-05569-6
18. Feldman EL, Stevens MJ, Thomas PK, Brown MB, Canal N, Greene DA. A Practical Two-Step Quantitative Clinical and Electrophysiological Assessment for the Diagnosis and Staging of Diabetic Neuropathy. Diabetes Care (1994) 17(11):1281–9. doi: 10.2337/diacare.17.11.1281
19. Papanas N, Ziegler D. Risk Factors and Comorbidities in Diabetic Neuropathy: An Update 2015. Rev Diabetes Stud (2015) 12(1-2):48–62. doi: 10.1900/RDS.2015.12.48
20. Pop-Busui R, Boulton AJ, Feldman EL, Bril V, Freeman R, Malik RA, et al. Diabetic Neuropathy: A Position Statement by the American Diabetes Association. Diabetes Care (2017) 40(1):136–54. doi: 10.2337/dc16-2042
21. Ismail-Beigi F, Craven T, Banerji MA, Basile J, Calles J, Cohen RM, et al. Effect of Intensive Treatment of Hyperglycaemia on Microvascular Outcomes in Type 2 Diabetes: An Analysis of the Accord Randomised Trial. Lancet (2010) 376(9739):419–30. doi: 10.1016/S0140-6736(10)60576-4
22. Charles M, Ejskjaer N, Witte DR, Borch-Johnsen K, Lauritzen T, Sandbaek A. Prevalence of Neuropathy and Peripheral Arterial Disease and the Impact of Treatment in People With Screen-Detected Type 2 Diabetes: The Addition-Denmark Study. Diabetes Care (2011) 34(10):2244–9. doi: 10.2337/dc11-0903
23. Duckworth W, Abraira C, Moritz T, Reda D, Emanuele N, Reaven PD, et al. Glucose Control and Vascular Complications in Veterans With Type 2 Diabetes. N Engl J Med (2009) 360(2):129–39. doi: 10.1056/NEJMoa0808431
24. Recio-Pinto E, Rechler MM, Ishii DN. Effects of Insulin, Insulin-Like Growth Factor-Ii, and Nerve Growth Factor on Neurite Formation and Survival in Cultured Sympathetic and Sensory Neurons. J Neurosci (1986) 6(5):1211–9. doi: 10.1523/JNEUROSCI.06-05-01211.1986
25. Brussee V, Cunningham FA, Zochodne DW. Direct Insulin Signaling of Neurons Reverses Diabetic Neuropathy. Diabetes (2004) 53(7):1824–30. doi: 10.2337/diabetes.53.7.1824
26. Zheng H, Sun W, Zhang Q, Zhang Y, Ji L, Liu X, et al. Proinflammatory Cytokines Predict the Incidence of Diabetic Peripheral Neuropathy Over 5 Years in Chinese Type 2 Diabetes Patients: A Prospective Cohort Study. EClinicalMedicine (2021) 31:100649. doi: 10.1016/j.eclinm.2020.100649
27. Papadopoulou-Marketou N, Whiss PA, Eriksson AC, Hyllienmark L, Papassotiriou I, Wahlberg J. Plasma Levels of Tissue Inhibitor of Metalloproteinase-1 in Patients With Type 1 Diabetes Mellitus Associate With Early Diabetic Neuropathy and Nephropathy. Diabetes Vasc Dis Res (2021) 18(2):14791641211002470. doi: 10.1177/14791641211002470
Keywords: fibrosis, insulin resistance, obesity, polyneuropathy, bariatric surgery
Citation: Kim K, Oh TJ, Park YS, Chang W, Cho HC, Lee J, Lee YK, Choi SH and Jang HC (2022) Association Between Fat Mass or Fat Fibrotic Gene Expression and Polyneuropathy in Subjects With Obesity: A Korean Metabolic Bariatric Surgery Cohort. Front. Endocrinol. 13:881093. doi: 10.3389/fendo.2022.881093
Received: 22 February 2022; Accepted: 28 March 2022;
Published: 16 May 2022.
Edited by:
Mostafa Qorbani, Alborz University of Medical Sciences, IranReviewed by:
Solaleh Emamgholipour, Tehran University of Medical Sciences, IranCopyright © 2022 Kim, Oh, Park, Chang, Cho, Lee, Lee, Choi and Jang. This is an open-access article distributed under the terms of the Creative Commons Attribution License (CC BY). The use, distribution or reproduction in other forums is permitted, provided the original author(s) and the copyright owner(s) are credited and that the original publication in this journal is cited, in accordance with accepted academic practice. No use, distribution or reproduction is permitted which does not comply with these terms.
*Correspondence: Tae Jung Oh, b2h0am1kQGdtYWlsLmNvbQ==
Disclaimer: All claims expressed in this article are solely those of the authors and do not necessarily represent those of their affiliated organizations, or those of the publisher, the editors and the reviewers. Any product that may be evaluated in this article or claim that may be made by its manufacturer is not guaranteed or endorsed by the publisher.
Research integrity at Frontiers
Learn more about the work of our research integrity team to safeguard the quality of each article we publish.