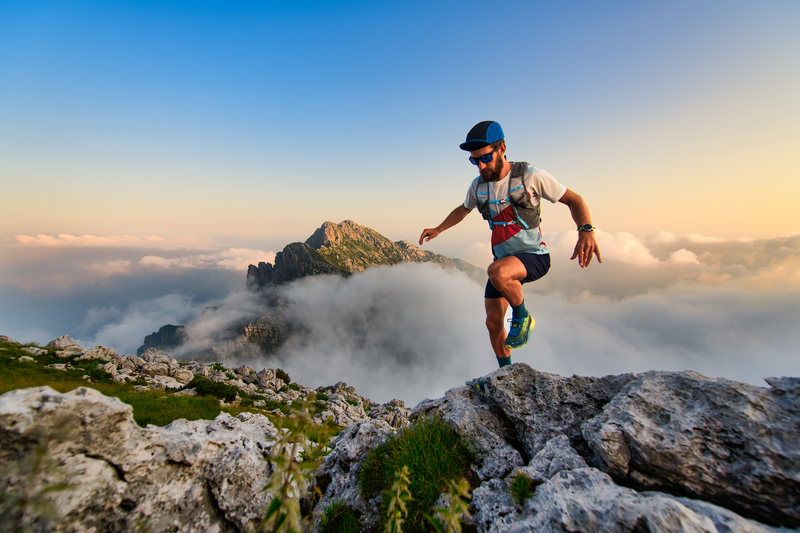
94% of researchers rate our articles as excellent or good
Learn more about the work of our research integrity team to safeguard the quality of each article we publish.
Find out more
ORIGINAL RESEARCH article
Front. Endocrinol. , 05 August 2022
Sec. Clinical Diabetes
Volume 13 - 2022 | https://doi.org/10.3389/fendo.2022.860274
This article is part of the Research Topic Prediabetes: New Insights on the Diagnosis, Risk Stratification, Comorbidites, Cardiovascular Disease, Microvascular Complications, and Treatment View all 7 articles
Purpose: The effect of hyperglycemia on periodontitis is mainly based on observational studies, and inconsistent results were found whether periodontal treatment favors glycemic control. The two-way relationship between periodontitis and hyperglycemia needs to be further elucidated. This study aims to evaluate the causal association of periodontitis with glycemic traits using bi-directional Mendelian randomization (MR) approach.
Methods: Summary statistics were sourced from large-scale genome-wide association study conducted for fasting glucose (N = 133,010), HbA1c (N = 123,665), type 2 diabetes (T2D, N = 659,316), and periodontitis (N = 506,594) among European ancestry. The causal relationship was estimated using the inverse-variance weighted (IVW) model and further validated through extensive complementary and sensitivity analyses.
Results: Overall, IVW showed that a genetically higher level of fasting glucose was significantly associated with periodontitis (OR = 1.119; 95% CI = 1.045–1.197; PFDR= 0.007) after removing the outlying instruments. Such association was robust and consistent through other MR models. Limited evidence was found suggesting the association of HbA1C with periodontitis after excluding the outliers (IVW OR = 1.123; 95% CI = 1.026–1.229; PFDR= 0.048). These linkages remained statistically significant in multivariate MR analyses, after adjusting for body mass index. The reverse direction MR analyses did not exhibit the causal association of genetic liability to periodontitis with any of the glycemic trait tested.
Conclusions: Our MR study reaffirms previous findings and extends evidence to substantiate the causal effect of hyperglycemia on periodontitis. Future studies with robust genetic instruments are needed to confirm the causal association of periodontitis with glycemic traits.
Periodontal disease, covering gingivitis and periodontitis, is a group of immuno-inflammatory disorders initiated by oral microbial dysbiosis, and if left untreated, periodontitis will lead to the destruction of tooth-supporting tissues and eventually tooth loss (1). As a highly prevalent non-communicable disease (NCD), severe periodontitis afflicts 10%–15% of the population worldwide, remaining a substantial socioeconomic burden (2–4).
Diabetes, characterized by poor glycemic control, is another common NCD with an estimated global prevalence of 9.3% (5). Prolonged hyperglycemia has been associated with a number of macrovascular and microvascular complications, leading to the damage of the corresponding organs such as heart, kidney, eyes, foot, and periodontal tissues (6, 7). Indeed, a two-way relationship has been proposed between diabetes and periodontitis (8). Diabetes is considered a risk for the development and progression of periodontitis. However, this evidence is mainly derived from observational studies, so that the causal inference is difficult to be established (9, 10). Moreover, the dose–response effect of fasting glucose and HbA1c on periodontitis has not been investigated. As for the reverse direction, the potential benefits of periodontal treatment on glycemic control remain unclear. Previous meta-analyses summarized that periodontal treatment reduced an average HbA1c level by 0.29% at 3–4 months, whereas such effect was not maintained after 4 months. Moreover, the conclusion should be interpreted with caution as the RCTs included are at high risk of bias due to the small sample size (11). Taken together, additional evidence for the causal inference on the two-way relationship between glycemic traits and periodontitis is highly warranted.
Two-sample Mendelian randomization (MR) is a powerful tool to explore the causal association in putative exposure–outcome pathways. It employs the genetic variants as unconfounded proxies for exposures to examine their effects on the outcome of interest (12). Because the genetic variants are randomly allocated at conception, MR is less vulnerable to confounding and reverse causation than observational studies. Furthermore, unlike RCT that describes the effect of an intervention in a certain period of time, MR assesses the impact of lifetime exposure to a genetic variant (13). In recent years, the increase in the accessible genome-wide association study (GWAS) data has fostered MR studies to employ single-nucleotide polymorphisms (SNPs) as instrumental variables (IVs) to estimate the causal effect of a modifiable risk factor on the disease, which supports or contradicts with previous epidemiological evidence (14). Recent MR studies have shown that genetically liability to periodontitis was not associated with glycemic control and type 2 diabetes (T2D) (15, 16). However, only study-level SNPs of periodontitis was adopted as proxy in this investigation, and the impact of glycemic traits such as glucose and HbA1c on periodontitis was not evaluated. In the present study, we investigated the relationship between periodontitis and glycemic trait (i.e., fasting glucose, HbA1c, and T2D) within the framework of bi-directional two-sample MR. The SNPs of both exposure and outcome were sourced from summary statistics of meta-analysis for GWAS in European ancestry populations.
The overall study design for the bi-directional two-sample MR analyses has been illustrated in Figure 1. MR analyses depend on three core assumptions (17). The genetic variant for IV (1) has to be strongly correlated with the exposure of interest, known as the “relevance” assumption; (2) should not be associated with any confounders of the exposure–outcome linkage, known as the “independence assumption”; and (3) can only affect the outcome through the exposure, also known as the “exclusion-restriction” assumption, which means no pleiotropy. By using the summary-level statistics from GWAS, this two-sample MR study assessed the effects of glycemic traits on periodontitis and then the reverse direction (i.e., periodontitis on glycemic traits). This study is conducted following the STROBE-MR (Strengthening the Reporting of Observational studies in Epidemiology - Mendelian randomization) statement (18).
Figure 1 Schematic diagram for the bidirectional MR study on the associations between periodontitis and glycemic traits (fasting glucose, HbA1c, and T2D).
The characteristics of the data sources are described in Supplemental Table 1. All participants included in the original GWAS were of European descent. Summary statistics for periodontitis were derived from the largest meta-analyses consisting of sources from Gene-Lifestyle Interactions in Dental Endpoints (GLIDE) consortium and UK Biobank (UKB) (19). The SNPs were associated with the combined trait consists clinically defined periodontitis from GLIDE (Ncases = 17,353, Ncontrols = 28,210) and self-reported loose teeth from UKB (Ncases = 18,979, Ncontrols = 442,052). Periodontitis cases were classified either by the Centers for Disease Control and Prevention/American Academy of Periodontology definition, similar criteria evaluated by probing depth (20), or self-reported. Summary statistics of glycemic traits including fasting glucose and HbA1c were retrieved from Meta-analyses of Glucose and Insulin-Related Traits Consortium (MAGIC), which is the largest publicly available meta-analyses conducted in individuals without diabetes (https://magicinvestigators.org). We exclude the glycemic traits from patients with diabetes because anti-diabetic medication or insulin may affect glucose metabolism beyond genetic influence. The genetic data of fasting glucose and HbA1c have been reported with (21) and without body mass index (BMI) adjustment (22, 23). Because the use of covariable-adjusted summary associations could have bias the MR estimates, summary level data for fasting glucose (N = 133,010) and HbA1c (N = 123,665) without BMI adjustment were selected. The dataset for type 2 diabetes was retrieved from DIAbetes Genetics Replication and Meta-analyses (DIAGRAM) and Genetic Epidemiology Research on Aging (GERA) with NCases = 62,892 and NControls = 596,424 (24). The details can be found in their original publications.
To satisfy the first MR assumption, SNPs included have to be robustly associated with the exposure of interest at a genome-wide significant threshold (P < 5 × 10−8). Linkage disequilibrium (LD) clumping was performed to ensure the SNPs are independent (LD R2 < 0.001, LD distance > 10,000 kb). After selection, a total of 33 SNPs of fasting glucose, 37 of HbA1c, and 120 of T2D were obtained as IV candidates. Because only one genome-wide significant SNP was identified for the combined trait of periodontitis–loose teeth, an arbitrary threshold for suggestive association (P < 5 × 10−6) was adopted, and 22 SNPs were selected accordingly.
We next removed exposure-related SNPs that cannot be proxied in the outcome dataset. Palindromic SNPs were excluded due to the difficulty in identifying the effect alleles in the exposure and outcome. Those SNPs showing direct associations with both exposure and outcome (P < 5 × 10−8) were excluded to reduce the potential pleiotropy (25). F statistics of each SNP were calculated with the formula to evaluate the strength of instruments (26, 27). SNPs with F < 10 were considered weak strength and removed.
The method of MR was conducted using R (version 4.1.0), through “TwoSampleMR (version 0.5.6)”, “MendelianRandomization (version 0.5.1)”, and “MR-PRESSO (version 1.0)” R packages. The priori statistic power was calculated using the online platform (28) (https://sb452.shinyapps.io/power/). The genetic correlation and latent causal variable (LCV) were calculated using the Complex-Traits Genetics Virtual Lab (https://vl.genoma.io/).
To evaluate whether periodontitis and glycemic traits are genetically correlated, we performed cross-trait LD score regression with GWAS summary statistics using the Complex-Traits Genetics Virtual Lab (29). Prior to MR analyses, the summary statistics were harmonized so that the effect allele of each SNP was consistent between exposure and outcomes. Inverse-variance weighted (IVW) model was adopted as the primary analysis because it is a meta-approach combining Wald estimates for each SNP, effectively treating each SNP as a valid natural experiment. Importantly, it forces a zero intercept in the regression slope so that the result could be biased if any IV is invalid (30, 31). Three additional MR models were adopted, i.e., MR-Egger regression (32), weighted median, and weighted mode (33), which tolerate the existence of horizontal pleiotropy but have lower statistical power than IVW. LCV model is an MR-equivalent method to distinguish genetic correlation from causation, and it is robust to sample overlap (34). We used LCV to determine whether the causal association of glycemic traits with periodontitis could be explained by the genetic correlation. MR-Egger regression intercept was evaluated to estimate the presence of horizontal pleiotropy. Intercept centered at the origin with 95% CI including the null indicates the absence of pleiotropy. Furthermore, Cochran’s Q test was used to assess the heterogenicity among SNPs in IVW estimates (P < 0.05 indicates significant heterogenicity). IVW random effect model was performed if statistically significant heterogenicity was found. Otherwise fixed effect model was adopted (35). If MR-Pleiotropy RESidual Sum and Outlier (MR-PRESSO) (36) detected heterogenicity, then we would remove the outlying variants (P < 0.05 in the MR-PRESSO outlier test) and perform the MR analyses again. If heterogenicity was still statistically significant (indicated by Cochran’s Q test), then SNPs with P < 1 in the MR-PRESSO outlier test would be further excluded before the MR analyses (37). The influential SNPs were evaluated on the basis of several plots (leave-one-out, funnel plot, forest plot, and scatter plot). To correct for multiple comparisons, we applied false discovery rate (FDR) correction in the final P-value estimates. An FDR-corrected P-value (PFDR) <5% was considered significant, and an unadjusted P-value < 0.05 was considered the evidence of a suggestive association.
For SNPs used as instruments variables, we extracted R2 (variance in exposure traits explained by SNPs) if provided in the original datasets. Otherwise, the following formula was used to calculate R2 (27).
To further verify the second assumption, we searched all the IVs in PhenoScanner (http://www.phenoscanner.medschl.cam.ac.uk) for association with previously reported confounders (P < 5 × 10−8) (38). We found IVs of BMI, which has been found to be associated hyperglycemia and periodontitis. We excluded these IVs of BMI in a sensitivity model and re-analyzed the MR estimates to gain a more direct cause-effect association. In addition, multivariate MR analysis was also conducted to minimize the correlated pleiotropy, which could have been introduced by indirect pathway (39). Summary statistics for BMI (N = ~700,000) were sourced from GIANT consortium (40).
The causal effects (i.e., T2D on periodontitis and vice versa) were converted from logit-scale to liability-scale using the following formula:
where KX and Ky are the population prevalence of exposure and outcome, and ZKX and ZKYare the height of standard normal distribution at such prevalence (41).
LD score regression showed statistically significant positive genetic correlations between periodontitis and fasting glucose (rg = 0.226; P = 0.005), HbA1c (rg = 0.198; P = 0.002), and T2D (rg = 0.296; P = 4.47 × 10−10) (Supplemental Table 2). In addition, LCV estimates provided a genetic causality proportion (GCP) at −0.53 (P = 1 × 10−6), suggesting a possible (GCP < 0.6) genetic causation of HbA1c with periodontitis. On the other hand, LCV showed no evidence suggesting the genetic causation of periodontitis with fasting glucose (GCP = 0.02; P = 0.619) and T2D (GCP = 0.43; P = 0.283) (Supplemental Table 3).
The stringent selection process of IVs of glycemic traits on periodontitis has been described in Supplemental Figure 1. Following the screening, SNPs associated with fasting glucose (n = 30), HbA1c (n = 37), and T2D (n = 117) were included as valid IV. The minimum F statistics of these IVs were all larger than 10 (range from 28.654 to 29.942), ensuring the “relevance” assumption that the weak instrument bias was not likely to influence the estimations of causal effects. The characteristics of all the SNPs included as IVs of glycemic traits were detailed in Supplemental Tables 4–6. Our MR analyses are sufficiently powered to detect the association of fasting glucose (88%), HbA1c (75%), and T2D (93%) with an OR of 1.1 and a type 1 error rate of 0.05 (Supplemental Table 7).
MR-PRESSO identified that rs6072275 and rs1280 were extreme outlying variants, which could markedly drive the MR association of fasting glucose with periodontitis. Following the removal of these two outliers, heterogeneity was no longer detected (Q = 27.872; P = 0.418). In addition, IVW showed a significant association [odds ratio (OR) = 1.119; 95% CI = 1.045–1.197; PFDR = 0.007], suggesting that per 1-standard deviation (0.73 mmol/L) increment in fasting glucose was associated with higher OR of periodontitis. Moreover, such association was consistent and significant through all the other MR methods including MR-Egger (OR = 1.257; 95% CI = 1.091–1.448; PFDR = 0.020), weight median (OR = 1.191; 95% CI = 1.078–1.315; PFDR = 0.007), and weighted mode (OR = 1.209; 95% CI = 1.092–1.339; PFDR = 0.007). Egger intercepts suggested no evidence of pleiotropy (P = 0.076). Taken together, these results suggested a causal association of genetically proxied higher fasting glucose with periodontitis. As for the association of HbA1c with periodontitis, we noted that rs11964178 and rs8192675 were extreme outlying IVs through MR-PRESSO. However, heterogenicity still existed (Q = 58.261; P = 0.008) after the removal of these two outliers. We further removed outliers with P-value less than 1 in the MR-PRESSO outlier test (rs560887, rs1387153, and rs17533903). Heterogenicity was no longer detected (Q = 29.907; P = 0.522), and pleiotropy was not found based on Egger intercept (P = 0.147). IVW results showed a significant association of HbA1c with periodontitis (OR = 1.123; 95% CI = 1.026–1.229; PFDR = 0.048). Such association was not supported by MR-Egger, weighted median, and weighted mode, suggesting limited evidence supporting the causal association of HbA1c with periodontitis. In addition, our results suggest a null association of T2D on periodontitis through any of the MR models (Table 1, Figure 2).
Table 1 Summary statistics for MR analyses of the potential causal effect of glycemic traits on periodontitis.
Figure 2 MR analyses for the causal association of glycemic traits (fasting glucose, HbA1c, and T2D) with periodontitis. IVW, inverse-variance weighted; T2D, type 2 diabetes. The error bar indicates the 95% confidence interval.
The associations of glycemic traits with periodontitis were further tested by removing IVs of BMI and multivariate MR to further reduce the potential horizontal pleiotropy introduced by confounders. The characteristics of the SNPs of BMI have been listed in Supplemental Table 8. We found a significant association of fasting glucose (IVW OR = 1.125; 95% CI = 1.043–1.214; P = 0.002) and HbA1c (IVW OR = 1.129; 95% CI = 1.024–1.245; P = 0.015) with periodontitis after excluding IVs of BMI (Figure 3). Furthermore, multivariate MR estimates also indicated a significant association of fasting glucose (IVW OR = 1.127; 95% CI = 1.022–1.244; P = 0.018) and HbA1c (IVW OR = 1.184; 95% CI = 1.026–1.366; P = 0.020) with periodontitis when BMI was accounted for (Figure 4).
Figure 3 MR analyses on the causal association of glycemic traits (fasting glucose, HbA1c, and T2D) with periodontitis after excluding SNPs of BMI. T2D, type 2 diabetes. The error bar indicates the 95% confidence interval.
Figure 4 Multivariate MR analyses on the causal association of glycemic traits (fasting glucose, HbA1c, and T2D) with periodontitis by adjusting for BMI. T2D, type 2 diabetes. The error bar indicates the 95% confidence interval.
There was no SNP substantially dominating the MR estimates as indicated by the “leave-one-out test”. Variant-specific causal estimates were visualized in funnel plot, scatter plot, and forest plot, indicating no considerable heterogenicity among the SNPs of fasting glucose, HbA1c, and type 2 diabetes (Supplemental Figures 2–4).
The workflow for IV selection of periodontitis is described in Supplemental Figure 5. After rigorous screening, eight SNPs associated with periodontitis were identified as valid IVs on HbA1c and 12 SNPs on T2D, respectively. The minimum F statistics for these IVs is 20.903 (all above 10), indicating that the weak instrument bias is neglectable. The SNPs associated with periodontitis were not available in the fasting glucose dataset, so that MR analyses were not performed. The characteristics of all the SNPs as IVs of periodontitis on glycemic traits were detailed in Supplemental Tables 9, 10. With a type 1 error rate of 0.05, our MR analyses are insufficiently powered when OR of periodontitis on HbA1c (31%) and T2D (53%) is set at 1.1 but adequately powered when OR is 1.2 (HbA1c, 80%; T2D, 97%) (Supplemental Table 7).
We found no evidence suggesting the causal effects of periodontitis on glycemic traits through different MR methods (Table 2, Figure 5). Significant heterogenicity and pleiotropy were not detected, as indicated by Cochran’s Q statistics and Egger intercept. “leave-one-out test” analysis indicated no single SNP substantially driving the causal estimates. Variant-specific causal estimates were visualized in funnel plot, scatter plot, and forest plot, suggesting no considerable heterogenicity among the SNPs of periodontitis (Supplemental Figures 6, 7).
Table 2 Summary statistics for MR analyses of the potential causal effect of periodontitis on glycemic traits.
Figure 5 MR analyses for the causal association of periodontitis with glycemic traits (HbA1c and T2D). IVW, inverse-variance weighted; T2D, type 2 diabetes. The error bar indicates the 95% confidence interval.
To the best of our knowledge, this is the first two-sample MR analysis investigating the bi-directional relationship between periodontitis and glycemic traits including fasting glucose, HbA1c, and T2D. By sourcing the summary-level data from previous GWAS, we found that genetically predisposition to a higher fasting glucose level is significantly associated with an increased risk of periodontitis. Limited evidence was found suggesting the association of HbA1c on periodontitis. Moreover, both fasting glucose and HbA1c retained a direct association with periodontitis in multivariate MR analyses adjusted for BMI. The reverse MR did not provide evidence supporting the causal inference of genetically proxied periodontitis on any of the glycemic traits of interest.
Epidemiological evidence from meta-analyses consisting of cross-sectional and longitudinal studies showed that hyperglycemia and T2D account for increased prevalence and severity of periodontitis, tooth loss, and edentulism (9). Although many of the above clinical studies have made adjustments for observed confounders, residual confounding may still exist to distort the association estimates. Moreover, causality is difficult to be obtained from these observational findings, and it is unethical and infeasible to conduct RCTs to determine the effects of hyperglycemia and diabetes on periodontitis. Unlike findings from the observational studies, the use of genetic variants as IVs in MR analyses is less vulnerable to modifiable confounders and reverse causality according to Mendel’s law of inheritance (13). Notably, our MR results extend previous literature by showing the pronounced association of fasting glucose on periodontitis. IVW estimates showed limited evidence supporting the association of HbA1c on periodontitis, likely due to the lower statistical power because our genetic instruments of HbA1c only explained 2.3% of the phenotypic variance, which is smaller than that of fasting glucose (3.2%). Another explanation is that the genetic loci affecting HbA1c through both glucose and erythrocytic pathways (23). The IVs associated with erythrocytic pathways might the limit the detection power. Considering that obesity is a common risk factor for hyperglycemia and periodontitis (42), we performed multivariate MR by adjusting BMI, further strengthening the directional causal association of fasting glucose and HbA1c on periodontitis. On the contrary to previous clinical findings, our results did not support the association of predisposition to T2D on the risk of periodontitis, which was in consistent with a recent MR study (16). The null association of T2D with periodontitis could be explained by the heterogenicity of T2D associated genetic loci (43). We hypothesized that the causal effect of T2D on periodontitis might arise from hyperglycemia, rather than insulin resistance or beta cell function.
Although the statistical significant causal association of fasting glucose with periodontitis was consistent across different MR methods, cautious interpretation on the clinical meaning of the MR estimates should be taken. It has to be noted that, like all other MR investigations, the causal estimates found in the current study should not be generally interpreted as the impact of intervening the glycemic traits on the risk of periodontitis in clinical practice. Instead, it should be better interpreted as a test statistic for a causal hypothesis, providing an alternative line of etiological evidence supporting the causal association of hyperglycemia with periodontitis (44, 45).
The association of periodontitis with systemic disease (e.g., cardiovascular disease, diabetes, and adverse pregnancy outcomes) has attracted considerable attention over the past decades. Although there has been a great progress in the mechanism underlying the periodontitis-systemic disease linkage, findings from RCTs testing the systemic effect of periodontal treatment are sometimes inconsistent and conflicting (46). A pooled estimate of about 0.3% reduction of HbA1c at 3 months following periodontal treatment has been noted in a Cochrane meta-analyses (11). However, inconsistent results were obtained from two RCTs with relatively large sample size in extended period. One (n = 264) showed a significant reduction of HbA1c at 12-month (−0.6%, 95% CI: −0.9% to −0.3%) and 6-month (−0.3%, 95% CI: −0.5% to 0.0%) follow-ups (47), whereas the other (n = 514) failed to detect a beneficial effect at 6-month (−0.05%, 95% CI: −0.23 to 0.12%) follow-ups (48). Moreover, a null effect was also observed in our earlier clinical trial (49). In the current study, the MR estimates did not show a robust causal association of periodontitis with any of the glycemic traits of interest, which is consistent with a previous MR using study-level SNPs (15). It has to be noted that null association could be explained by the lack of association strength of genetic variants for periodontitis. Although the estimated heritability of periodontitis is relatively high, GWAS of periodontitis defined by clinical criteria has had modest success to date, failing to identify consistent SNPs (50). In this meta-analysis GWAS dataset that we sourced, only one SNP reached genome-wide significance for the combined trait of periodontitis and loose teeth, whereas none for the single trait of periodontitis (19). A suggestive association (P < 5 × 10−6) was therefore adopted, but the genetic instruments only explained approximately 0.06% of the phenotypic variance. As such, we were not capable of detecting small effects although significant horizontal pleiotropy and weak instrument bias (all IVs with F statistics > 10) have been ruled out. In line with our findings, a recent MR study adopting genetic variants (n = 5) on the basis of single GWAS also found no causal effect of periodontitis on T2D. These genetic variants had a mean F of 4.89 (ranging from 0.895 to 7.294), suggesting a weak instrumental bias (16). Nevertheless, it is premature to conclude the null association of periodontitis with glycemic traits based on the current findings. Identifying more genetic instruments with robust association is highly warranted to boost the detection power in further MR studies using periodontitis as exposure.
A key strength of our study is that we sourced data of exposure and outcome from summary-level GWAS on different cohorts with large sample size. The effect size could be more precisely assessed with multiple sensitivity analyses in comparison to MR analyses using study-level data from a smaller cohort. Moreover, multiple sensitivity analyses were conducted to evaluate and minimize the potential heterogenicity and pleiotropy. In addition, horizontal pleiotropy was further reduced by the multivariate MR adjusting for BMI. Our study has several limitations. First, like all the other MR studies, the genetic variants represent lifelong exposure. The significant association found in our study is likely to reflect the life-long effect of hyperglycemia on periodontitis. Second, there are several overlapping cohorts contributing to the summary statistics of both exposure and outcome. However, the F statistics indicated that the included instruments are relatively strong, which could reduce the potential bias due to overlapping sampling (51). Third, although the analyses are restricted to European descents to minimize the bias from population stratification, our findings may not be generalized to other ethnicities. Fourth, the trait BMI is heterogeneous, which could bias the multivariate MR estimates. Because an increased BMI results from either excessive fat or fat-free mass, SNPs of BMI could be associated unfavorable adiposity or favorable adiposity, which may confer different metabolic effects on periodontitis (52).
In conclusion, we extended previous observational findings by demonstrating a significant causal association of genetically determined higher levels of fasting glucose with periodontitis, highlighting the importance of glycemic control for better oral/periodontal care. In the reverse direction, current results did not support a robust causal association of periodontitis with the glycemic traits of interest. Future GWAS identifying more genetic loci that strongly associated with periodontitis is needed to support or contradict this null association.
The original contributions presented in the study are included in the article/Supplementary Material. Further inquiries can be directed to the corresponding author/s.
This study only used publicly available data and the relevant ethical approval can be found in the corresponding studies referenced in the Materials and Methods section.
YW, RH, and HD contributed to the conception and design of the study. YW and TC organized the database and performed the statistical analysis. YG, SL, and LW performed the statistical analysis. YW, TC wrote the first draft of the manuscript. LJ, RH, and HD refined the manuscript. All authors contributed to manuscript revision, read, and approved the submitted version.
This study was financially supported by grants from the National Natural Foundation of China (No.82101029), Natural Science Foundation of Zhejiang Province (No. LQ20H140002), and Wenzhou Science and Technology Bureau (No. Y20190100 and ZY2020021). Zhejiang Provincial Public Welfare Science and Technology Project, Grant/Award Number: LGF21H140006.
The authors are enormously grateful to the investigators of the original GWAS for sharing their summary level data used in this study.
The authors declare that the research was conducted in the absence of any commercial or financial relationships that could be construed as a potential conflict of interest.
All claims expressed in this article are solely those of the authors and do not necessarily represent those of their affiliated organizations, or those of the publisher, the editors and the reviewers. Any product that may be evaluated in this article, or claim that may be made by its manufacturer, is not guaranteed or endorsed by the publisher.
The Supplementary Material for this article can be found online at: https://www.frontiersin.org/articles/10.3389/fendo.2022.860274/full#supplementary-material
1. Pihlstrom BL, Michalowicz BS, Johnson NW. Periodontal diseases. Lancet (2005) 366:1809–20. doi: 10.1016/S0140-6736(05)67728-8
2. Jin LJ, Lamster IB, Greenspan JS, Pitts NB, Scully C, Warnakulasuriya S. Global burden of oral diseases: emerging concepts, management and interplay with systemic health. Oral Dis (2016) 22:609–19. doi: 10.1111/odi.12428
3. Kassebaum NJ, Bernabe E, Dahiya M, Bhandari B, Murray CJ, Marcenes W. Global burden of severe periodontitis in 1990-2010: a systematic review and meta-regression. J Dent Res (2014) 93:1045–53. doi: 10.1177/0022034514552491
4. Tonetti MS, Jepsen S, Jin L, Otomo-Corgel J. Impact of the global burden of periodontal diseases on health, nutrition and wellbeing of mankind: A call for global action. J Clin Periodontol (2017) 44:456–62. doi: 10.1111/jcpe.12732
5. Sinclair A, Saeedi P, Kaundal A, Karuranga S, Malanda B, Williams R. Diabetes and global ageing among 65-99-year-old adults: Findings from the international diabetes federation diabetes atlas, 9(th) edition. Diabetes Res Clin Pract (2020) 162:108078. doi: 10.1016/j.diabres.2020.108078
6. Rask-Madsen C, King GL. Vascular complications of diabetes: mechanisms of injury and protective factors. Cell Metab (2013) 17:20–33. doi: 10.1016/j.cmet.2012.11.012
7. Preshaw PM, Bissett SM. Periodontitis: oral complication of diabetes. Endocrinol Metab Clin North Am (2013) 42:849–67. doi: 10.1016/j.ecl.2013.05.012
8. Sanz M, Ceriello A, Buysschaert M, Chapple I, Demmer RT, Graziani F, et al. Scientific evidence on the links between periodontal diseases and diabetes: Consensus report and guidelines of the joint workshop on periodontal diseases and diabetes by the international diabetes federation and the European federation of periodontology. J Clin Periodontol (2018) 45:138–49. doi: 10.1111/jcpe.12808
9. Chavarry NG, Vettore MV, Sansone C, Sheiham A. The relationship between diabetes mellitus and destructive periodontal disease: a meta-analysis. Oral Health Prev Dent (2009) 7:107–27. doi: 10.1111/j.1601-0825.2008.01470.x
10. Genco RJ, Borgnakke WS. Diabetes as a potential risk for periodontitis: association studies. Periodontol 2000 (2020) 83:40–5. doi: 10.1111/prd.12270
11. Simpson TC, Weldon JC, Worthington HV, Needleman I, Wild SH, Moles DR, et al. Treatment of periodontal disease for glycaemic control in people with diabetes mellitus. Cochrane Database Syst Rev (2015) 2015(11):CD004714. doi: 10.1002/14651858.CD004714.pub3
12. Burgess S, Butterworth A, Thompson SG. Mendelian randomization analysis with multiple genetic variants using summarized data. Genet Epidemiol (2013) 37:658–65. doi: 10.1002/gepi.21758
13. Davies NM, Holmes MV, Davey Smith G. Reading mendelian randomisation studies: a guide, glossary, and checklist for clinicians. BMJ (2018) 362:k601. doi: 10.1136/bmj.k601
14. Burgess S, Foley CN, Zuber V. Inferring causal relationships between risk factors and outcomes from genome-wide association study data. Annu Rev Genomics Hum Genet (2018) 19:303–27. doi: 10.1146/annurev-genom-083117-021731
15. Shah PD, Schooling CM, Borrell LN. Impact of liability to periodontitis on glycemic control and type II diabetes risk: A mendelian randomization study. Front Genet (2021) 12:767577. doi: 10.3389/fgene.2021.767577
16. Wang YB, Yan SY, Li XH, Huang Q, Luo LS, Wang YY, et al. Causal association between periodontitis and type 2 diabetes: A bidirectional two-sample mendelian randomization analysis. Front Genet (2021) 12:792396. doi: 10.3389/fgene.2021.792396
17. Lawlor DA. Commentary: Two-sample mendelian randomization: opportunities and challenges. Int J Epidemiol (2016) 45:908–15. doi: 10.1093/ije/dyw127
18. Skrivankova VW, Richmond RC, Woolf BAR, Yarmolinsky J, Davies NM, Swanson SA, et al. Strengthening the reporting of observational studies in epidemiology using mendelian randomization: The STROBE-MR statement. JAMA (2021) 326:1614–21. doi: 10.1001/jama.2021.18236
19. Shungin D, Haworth S, Divaris K, Agler CS, Kamatani Y, Keun Lee M, et al. Genome-wide analysis of dental caries and periodontitis combining clinical and self-reported data. Nat Commun (2019) 10:2773. doi: 10.1038/s41467-019-10630-1
20. Page RC, Eke PI. Case definitions for use in population-based surveillance of periodontitis. J Periodontol (2007) 78:1387–99. doi: 10.1902/jop.2007.060264
21. Chen J, Spracklen CN, Marenne G, Varshney A, Corbin LJ, Luan J, et al. The trans-ancestral genomic architecture of glycemic traits. Nat Genet (2021) 53:840–60. doi: 10.1038/s41588-021-00852-9
22. Scott RA, Lagou V, Welch RP, Wheeler E, Montasser ME, Luan J, et al. Large-Scale association analyses identify new loci influencing glycemic traits and provide insight into the underlying biological pathways. Nat Genet (2012) 44:991–1005. doi: 10.1038/ng.2385
23. Wheeler E, Leong A, Liu CT, Hivert MF, Strawbridge RJ, Podmore C, et al. Impact of common genetic determinants of hemoglobin A1c on type 2 diabetes risk and diagnosis in ancestrally diverse populations: A transethnic genome-wide meta-analysis. PLoS Med (2017) 14:e1002383. doi: 10.1371/journal.pmed.1002383
24. Xue A, Wu Y, Zhu Z, Zhang F, Kemper KE, Zheng Z, et al. Genome-wide association analyses identify 143 risk variants and putative regulatory mechanisms for type 2 diabetes. Nat Commun (2018) 9(1):2941. doi: 10.1038/s41467-018-04951-w
25. Hemani G, Zheng J, Elsworth B, Wade KH, Haberland V, Baird D, et al. The MR-base platform supports systematic causal inference across the human phenome. Elife (2018) 7:e34408. doi: 10.7554/eLife.34408
26. Wu P, Zhang X, Zhou P, Zhang W, Li D, Lv M, et al. Assessment of bidirectional relationships between polycystic ovary syndrome and periodontitis: Insights from a mendelian randomization analysis. Front Genet (2021) 12:644101. doi: 10.3389/fgene.2021.644101
27. Papadimitriou N, Dimou N, Tsilidis KK, Banbury B, Martin RM, Lewis SJ, et al. Physical activity and risks of breast and colorectal cancer: a mendelian randomisation analysis. Nat Commun (2020) 11(1):597. doi: 10.1038/s41467-020-14389-8
28. Brion MJ, Shakhbazov K, Visscher PM. Calculating statistical power in mendelian randomization studies. Int J Epidemiol (2013) 42:1497–501. doi: 10.1093/ije/dyt179
29. Bulik-Sullivan BK, Loh PR, Finucane HK, Ripke S, Yang J, Schizophrenia Working Group of the Psychiatric Genomics Consortium, et al. LD score regression distinguishes confounding from polygenicity in genome-wide association studies. Nat Genet (2015) 47:291–5. doi: 10.1038/ng.3211
30. Hartwig FP, Davey Smith G, Bowden J. Robust inference in summary data mendelian randomization via the zero modal pleiotropy assumption. Int J Epidemiol (2017) 46:1985–98. doi: 10.1093/ije/dyx102
31. Hwang LD, Lawlor DA, Freathy RM, Evans DM, Warrington NM. Using a two-sample mendelian randomization design to investigate a possible causal effect of maternal lipid concentrations on offspring birth weight. Int J Epidemiol (2019) 48:1457–67. doi: 10.1093/ije/dyz160
32. Bowden J, Davey Smith G, Burgess S. Mendelian randomization with invalid instruments: effect estimation and bias detection through egger regression. Int J Epidemiol (2015) 44:512–25. doi: 10.1093/ije/dyv080
33. Bowden J, Davey Smith G, Haycock PC, Burgess S. Consistent estimation in mendelian randomization with some invalid instruments using a weighted median estimator. Genet Epidemiol (2016) 40:304–14. doi: 10.1002/gepi.21965
34. O'Connor LJ, Price AL. Distinguishing genetic correlation from causation across 52 diseases and complex traits. Nat Genet (2018) 50:1728–34. doi: 10.1038/s41588-018-0255-0
35. Burgess S, Scott RA, Timpson NJ, Thompson SG, EPIC- InterAct Consortium. Using published data in mendelian randomization: a blueprint for efficient identification of causal risk factors. Eur J Epidemiol (2015) 30:543–52. doi: 10.1007/s10654-015-0011-z
36. Verbanck M, Chen CY, Neale B, Do R. Detection of widespread horizontal pleiotropy in causal relationships inferred from mendelian randomization between complex traits and diseases. Nat Genet (2018) 50:693–98. doi: 10.1038/s41588-018-0099-7
37. Wu F, Huang Y, Hu J, Shao Z. Mendelian randomization study of inflammatory bowel disease and bone mineral density. BMC Med (2020) 18:312. doi: 10.1186/s12916-020-01778-5
38. Kamat MA, Blackshaw JA, Young R, Surendran P, Burgess S, Danesh J, et al. PhenoScanner V2: an expanded tool for searching human genotype-phenotype associations. Bioinformatics (2019) 35:4851–53. doi: 10.1093/bioinformatics/btz469
39. Sanderson E, Davey Smith G, Windmeijer F, Bowden J. An examination of multivariable mendelian randomization in the single-sample and two-sample summary data settings. Int J Epidemiol (2019) 48:713–27. doi: 10.1093/ije/dyy262
40. Yengo L, Sidorenko J, Kemper KE, Zheng Z, Wood AR, Weedon MN, et al. Meta-analysis of genome-wide association studies for height and body mass index in approximately 700000 individuals of European ancestry. Hum Mol Genet (2018) 27:3641–49. doi: 10.1093/hmg/ddy271
41. Zhang H, Xiu X, Xue A, Yang Y, Yang Y, Zhao H. Mendelian randomization study reveals a population-specific putative causal effect of type 2 diabetes in risk of cataract. Int J Epidemiol (2022) 50(6):2024–37. doi: 10.1101/2021.02.08.430342
42. Chapple IL, Bouchard P, Cagetti MG, Campus G, Carra MC, Cocco F, et al. Interaction of lifestyle, behaviour or systemic diseases with dental caries and periodontal diseases: consensus report of group 2 of the joint EFP/ORCA workshop on the boundaries between caries and periodontal diseases. J Clin Periodontol (2017) 44 Suppl 18:S39–51. doi: 10.1111/jcpe.12685
43. Udler MS, Kim J, von Grotthuss M, Bonàs-Guarch S, Cole JB, Chiou J, et al. Type 2 diabetes genetic loci informed by multi-trait associations point to disease mechanisms and subtypes: A soft clustering analysis. PLoS Med (2018) 15:e1002654. doi: 10.1371/journal.pmed.1002654
44. Burgess S, Davey Smith G, Davies NM, Dudbridge F, Gill D, Glymour MM, et al. Guidelines for performing mendelian randomization investigations. Wellcome Open Res (2019) 4:186. doi: 10.12688/wellcomeopenres.15555.1
45. Burgess S, Butterworth A, Malarstig A, Thompson SG. Use of mendelian randomisation to assess potential benefit of clinical intervention. BMJ (2012) 345:e7325. doi: 10.1136/bmj.e7325
46. Beck JD, Papapanou PN, Philips KH, Offenbacher S. Periodontal medicine: 100 years of progress. J Dent Res (2019) 98:1053–62. doi: 10.1177/0022034519846113
47. D'Aiuto F, Gkranias N, Bhowruth D, Khan T, Orlandi M, Suvan J, et al. Systemic effects of periodontitis treatment in patients with type 2 diabetes: a 12 month, single-centre, investigator-masked, randomised trial. Lancet Diabetes Endocrinol (2018) 6:954–65. doi: 10.1016/S2213-8587(18)30038-X
48. Engebretson SP, Hyman LG, Michalowicz BS, Schoenfeld ER, Gelato MC, Hou W, et al. The effect of nonsurgical periodontal therapy on hemoglobin A1c levels in persons with type 2 diabetes and chronic periodontitis: a randomized clinical trial. JAMA (2013) 310:2523–32. doi: 10.1001/jama.2013.282431
49. Wang Y, Liu HN, Zhen Z, Pelekos G, Wu MZ, Chen Y, et al. A randomized controlled trial of the effects of non-surgical periodontal therapy on cardiac function assessed by echocardiography in type 2 diabetic patients. J Clin Periodontol (2020) 47:726–36. doi: 10.1111/jcpe.13291
50. Nibali L, Di Iorio A, Tu YK, Vieira AR. Host genetics role in the pathogenesis of periodontal disease and caries. J Clin Periodontol (2017) 44 Suppl 18:S52–78. doi: 10.1111/jcpe.12639
51. Pierce BL, Burgess S. Efficient design for mendelian randomization studies: subsample and 2-sample instrumental variable estimators. Am J Epidemiol (2013) 178:1177–84. doi: 10.1093/aje/kwt084
Keywords: Mendelian randomization, periodontitis, glycemic trait, T2D (type 2 diabetes), causal association
Citation: Wang Y, Chu T, Gong Y, Li S, Wu L, Jin L, Hu R and Deng H (2022) Mendelian randomization supports the causal role of fasting glucose on periodontitis. Front. Endocrinol. 13:860274. doi: 10.3389/fendo.2022.860274
Received: 22 January 2022; Accepted: 07 July 2022;
Published: 05 August 2022.
Edited by:
Martin Buysschaert, Catholic University of Louvain, BelgiumReviewed by:
Mario Luca Morieri, University of Padua, ItalyCopyright © 2022 Wang, Chu, Gong, Li, Wu, Jin, Hu and Deng. This is an open-access article distributed under the terms of the Creative Commons Attribution License (CC BY). The use, distribution or reproduction in other forums is permitted, provided the original author(s) and the copyright owner(s) are credited and that the original publication in this journal is cited, in accordance with accepted academic practice. No use, distribution or reproduction is permitted which does not comply with these terms.
*Correspondence: Hui Deng, ZGgwNzI2QDE2My5jb20=; Yi Wang, eS53YW5nQHdtdS5lZHUuY24=
†These authors have contributed equally to this work
Disclaimer: All claims expressed in this article are solely those of the authors and do not necessarily represent those of their affiliated organizations, or those of the publisher, the editors and the reviewers. Any product that may be evaluated in this article or claim that may be made by its manufacturer is not guaranteed or endorsed by the publisher.
Research integrity at Frontiers
Learn more about the work of our research integrity team to safeguard the quality of each article we publish.