- 1Department of Orthopedic Surgery, The First Affiliated Hospital of Chongqing Medical University, Chongqing, China
- 2Orthopedic Laboratory of Chongqing Medical University, Chongqing, China
Background: This Mendelian randomization (MR) study aimed to explore the causal relationship between polyunsaturated fatty acids (PUFAs) and bone mineral density (BMD).
Methods: We conducted a two-sample MR analysis to figure out if there is any causal effect of PUFAs on BMD through the summary data from the genome-wide association study (GWAS). Relationships were evaluated through inverse variance weighted (IVW), MR-Egger, weighted median, and maximum likelihood methods. The MR Pleiotropy RESidual Sum and Outlier (MR-PRESSO) test was performed to detect the horizontal pleiotropy.
Results: Our findings revealed that omega-6 fatty acids were negatively related to the TB-BMD (beta-estimate: −0.0515; 95% confidence interval [CI]: −0.0911 to −0.0119; standard error [SE]: 0.0201; p-value: 0.0106). The reverse direction MR analysis showed that TB-BMD was linked to the omega-6 FAs (beta-estimate: −0.0699; 95% CI: −0.1304 to −0.0095; SE: 0.0308; p-value: 0.0265). No statistically significant correlations between PUFAs and BMD were observed after adjusting the interactions between metabolites.
Conclusion: This two-sample MR analyses produced strong and new genomic evidence that there was a causal relationship between omega-6 FAs and BMD. Further investigations are still required to elucidate the potential mechanism.
Introduction
Osteoporosis is defined as a systematic musculoskeletal disease featured as the loss of bone mass and the degradation of the micro-architecture of the bone tissue, which is invariably predisposed to the increased fragility of bones and incidence of fractures (1, 2). As the global population is aging, it has been considered one of the most pressing public health concerns. According to the statistics, over 9 million osteoporosis-related fractures were confirmed worldwide annually, in which the direct financial losses incurred were estimated at a 17 billion dollars (3). Therefore, osteoporosis now imposes a major economic and clinical burden on society, in addition to inflicting pain and suffering to patients, especially the elderly (4). Nowadays, clinical diagnosis and assessment of osteoporosis rely heavily on bone mineral density (BMD) measurements, which have been proven to be reliable and effective (5, 6). Notably, both osteoporosis and BMD were demonstrated to be highly heritable and polygenic (7–9).
Optimal intake of certain nutrients is proven to participate in the regulation of BMD and is associated with the progress of osteoporosis (10), such as calcium (11) and retinol (12). Of these nutrients, dietary fats were thought to be critical to maintain normal musculoskeletal structure and functions (13–15). As an important component in our dietary fats, fatty acids (FAs) are mainly categorized as long-chain fatty acids including polyunsaturated fatty acids (PUFAs), monounsaturated fatty acids (MUFAs), saturated fatty acids (SFAs), medium-/short-chain fatty acids (MCFAs/SCFAs), and their metabolites (14). According to previous research, PUFAs may have a dual effect on bone metabolism depending on their structure, origin, relative concentration, and metabolic environment (13). In light of this, numerous studies had indicated that omega-6 promotes bone loss, whereas omega-3 favors bone remodeling. Several possible mechanisms had been proposed and clarified (16–18), which include calcium metabolism modulation (19), synthesis of prostaglandin (17), oxidation of fatty acids, genesis of osteoblast (20), and osteoclastogenesis.
Recently, numerous observational studies had indicated a link between PUFAs and BMD, although the findings remain controversial and conflicting. Besides that, observational studies have inherent limitations to infer causal association, such as reverse causality and confounding risk factors.
Mendelian randomization (MR) analyses, which use single nucleotide polymorphisms (SNPs) associated with exposure as instrumental variables (IVs) to evaluate the potentially causal effect between risk factors and outcomes, have evident advantages over conventional observation studies, according to this rationale (21). It is not affected by traditional confounders (environmental exposure and behaviors) and meets the plausibility of causal effect by time order (causes precede effects). A two-sample MR analysis means that IVs associated with exposure and those associated with outcome were obtained from different datasets of population, which could raise the statistical power.
Methods
To investigate the causal effect of PUFAs on BMD values, we conducted a two-sample MR study that highly relied upon the summary level GWAS data for analysis (22, 23). In a Mendelian randomization analysis, three core assumptions about instrumental variables must be fulfilled: (1) IVs must be strongly related to the exposure, (2) association with the outcome was solely due to the exposure, and (3) independent of any other confounding variables (21). First, we extracted SNPs strongly associated with each PUFAs as instrument values (p<5E−08). To ease the bias due to linkage disequilibrium (LD), we performed the clumping method (R2<0.001, window size=10,000 kb). To estimate the degree of LD, the individuals with European ancestors from the 1000 Genomes Project were used as a reference sample (24).
Second, the summary level data of SNPs related to exposure were retrieved in the outcome data.
Third, harmonization of the chosen SNP effect with the risk factors and outcomes was performed to align the palindromic SNPs (with A/T or G/C pairs). Possible palindromic SNPs were excluded.
Following that, we utilized PhenoScanner, a database of human genotype–phenotype associations, to see if any of the chosen SNPs were correlated with the potential confounders for BMD (25, 26). The threshold was set below: genome-wide significance (p<1E−5) and R2<0.8. Moreover, F-statistics was used to assess the strength of IVs, and an F-value >10 indicated strong instruments (27). The strength of each instrument was measured by calculating the F-statistic using the following formula: F = R2(N -2)/(1 – R2), where R2 was the proportion of variance in the phenotype explained by the genetic variants, and N was the sample size (28).
Genetic Association With PUFAs
The SNP summary data associated with PUFAs were derived from the Nightingale Health UK Biobank Initiative. The UK Biobank recruited 502,639 European participants aged 37–70 years in 22 assessment centers across the UK. All study participants reached the assessment centers by their own means, and enrollment was not performed at nursing homes (29). The biomarker profiles of 500,000 blood samples from UK Biobank were analyzed in Nightingale Health by utilizing nuclear magnetic resonance (NMR) and proprietary software, which could provide over 200 metabolic biomarkers in a single blood test including fatty acids (30). This first release covers biomarker data from approximately 118,000 EDTA plasma samples from baseline recruitment and 5,000 samples from repeat assessment (with 1,500 participants having both baseline and repeat-visit sample in the first data release). The metabolic biomarker dataset was open to any research institutions or individuals via the IEU GWAS database, which was a publicly accessible database of genetic correlation from GWAS summary datasets (23).
We only focused on some particular datasets of PUFAs, and three exposure data were selected, namely, omega-3 FAs, omega-6 FAs, and the ratio of omega-6 FAs to omega-3 FAs.
Genetic Association With BMD
A big GWAS meta-analysis of BMD enrolled 53,236 participants of European origin from GEnetic Factors for Osteoporosis Consortium (31). The femoral neck (FN), the lumbar spine (LS) (L1–L4), and the forearm (FA) were all measured for BMD by dual-energy X-ray absorptiometry (DXA) machines. Each variant with a minor allele frequency (MAF) >0.5% was checked for its effect on BMD, adjusting for sex, age, age2, and weight, and standardized to have a mean of zero and standard deviation of one to avoid the potential systematic differences caused by different measuring machines (31). In addition, the summary level data of total body (TB) BMD was employed from one large GWAS meta-analyses comprised of 30 studies and 66,628 individuals from America, Europe, and Australia, in which the majority of the participants came from population-based cohorts of European ancestry (86%) (32). TB-BMD (g/cm2) was measured by DXA according to the standard manufacturer protocols. Moreover, its value was corrected for age, weight, height, and genomic principal components (derived from GWAS data), and any additional study-specific covariates (e.g., recruiting center) (32). A detailed information related to the GWAS data is provided and shown in Supplementary Table S1.
Statistical Analyses
We performed the two-sample MR analysis with the inverse variance weighted (IVW) method (23, 33), MR-Egger method (34, 35), weighted median method (36), and maximum likelihood (33) method to estimate the effect of PUFAs for BMD. In algorithm principle, the IVW method might generate the most precise estimate by integrating the Wald ratios of each SNP’s causal effect through meta-analysis (23, 33). To avoid the bias caused by the horizontal pleiotropic effects, we conducted the MR-Egger method and weighted median method to analyze and test the potential directional bias caused by pleiotropy. When no <50% of the weight in the analysis is accounted for by the effective IVs, the weighted median method could offer a plausible estimate of the causal relationships (36). The MR-Egger method, which generated a weighted linear regression between exposure and outcome coefficients, was conducted to evaluate the pleiotropy better. Under the premise of meeting the basic assumption of Instrument Strength Independent of Direct Effect (InSIDE), the slope of the regression line could represent the asymptotically unbiased causal estimate. Apart from this, the horizontal pleiotropy in the average data of the whole genetic instruments could be quantified and presented by the intercept of the MR-Egger regression line (34, 35). Under the condition that the intercept of the regression line is not equal to 0, the intercept of the MR-Egger method can be applied to detect the horizontal pleiotropy. p<0.05 was considered to be statistically significant. Moreover, we also performed multivariable MR (MVMR) analysis to control potential interactions between metabolites. The bidirectional Mendelian randomization was also conducted to explore the reverse causation. All the MR analyses were conducted in R statistical software (Version 4.1.1) by utilizing the “TwoSampleMR” package (https://github.com/MRCIEU/TwoSampleMR) (23).
Sensitivity Analyses
For sensitivity analysis, several statistics approaches were applied. Cochran Q statistic was tested to assess and quantity heterogeneity (37). Depending on the degree of heterogeneities (Q>0.05 fixed-effect model; Q<0.05 random-effect model), the fixed- or random-effect model was used for further analysis. For quantitative analysis of heterogeneities, we also used I2 to evaluate the magnitude. It is generally accepted that I2>50% indicates significant heterogeneity. The directional pleiotropy was assessed through the intercept of the MR-Egger method. As a further step, we also conducted the MR Pleiotropy RESidual Sum and Outlier (MR-PRESSO) test to detect the horizontal pleiotropy and remove the outlier SNPs to reassess the cause estimate (38, 39). The “leave-one-out” sensitivity test was applied to figure out the potentially influential single SNP (Supplementary Figures S1–12).
Results
Causal Effect of PUFA on BMD
After verification, the final data of SNPs enrolled in our analysis are shown in Supplementary Tables S2–8. We evaluated the causal effect of PUFA, which includes omega-3 FAs, omega-6 FAs, and the ratio of omega-6 FAs to omega-3 FAs on LS-BMD, FN-BMD, FA-BMD, and TB-BMD in the two-sample MR analysis. The scatter plots are displayed in Supplementary Figures S13–24. The results are displayed in Table 1 and Figure 1. Based on the IVW analysis, omega-6 fatty acids were proven to be negatively related to the TB-BMD (beta-estimate: −0.0515; 95% confidence interval [CI]: −0.0911 to −0.0119; standard error [SE]: 0.0201; p-value: 0.0106), which indicated that a 1-SD decrease in omega-6 fatty acids was associated with the improvement in TB-BMD levels by 0.0515 g/cm2. The result was further validated by maximum likelihood method (beta-estimate: −0.0517; 95% CI: −0.0915 to −0.0120; SE: 0.0202; p-value: 0.0106). Moreover, no significant correlations were found between omega-6 FAs and site-specific BMD (LS-BMD, FN-BMD, and FA-BMD) according to the statistical analysis results of IVW method, MR-Egger regression, weighted median method, and maximum likelihood analysis.
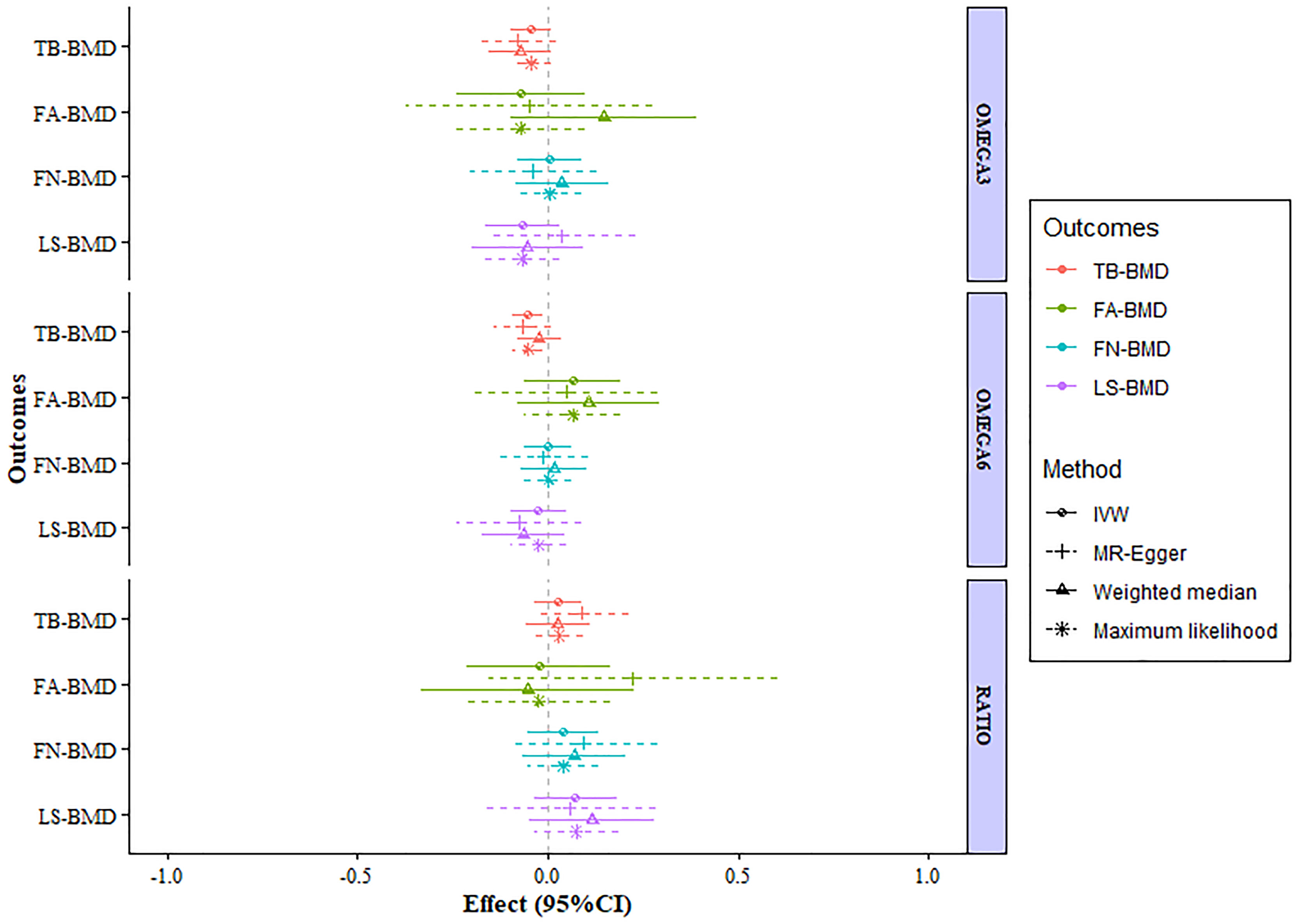
Figure 1 MR estimates of the associations between PUFAs and BMD. The x-axis is the effects of PUFAs on BMD values. The vertical dashed line is the reference at effect = 0. The y-axis presents different BMD types, which are highlighted in different colors. Different MR methods are displayed with different line types. MR, Mendelian randomization; BMD, bone mineral density; FN-BMD, femoral neck BMD; LS-BMD, lumbar spine BMD; FA-BMD, forearm BMD; TB-BMD, total body BMD; SNPs, single nucleotide polymorphisms; IVW, inverse variance weighted; SE, standard error; CI, confidence interval.
A higher ratio of omega-6 FAs to omega-3 FAs was proven to be poorly related to the improved BMD of the lumbar spine (beta-estimate: 0.0726; 95% CI: −0.0376 to 0.1829; SE: 0.0562; p-value: 0.1966) (Table 1) according to the result of the IVW analysis. Furthermore, this conclusion from IVW approach was in accordance with those of the other three statistical models. Moreover, no significant correlations were found between the ratio of FAs and FN-BMD or FA-BMD according to the statistical analysis results of IVW method, MR-Egger regression, weighted median method, and maximum likelihood analysis.
Analogously, omega-3 fatty acids also demonstrated no positive correlation to LS-BMD (beta-estimate: −0.0671; 95% CI: −0.1650 to 0.0307; SE: 0.0499; p-value: 0.1789), FN-BMD (beta-estimate: 0.0041; 95% CI: −0.0802 to 0.0885; SE: 0.0430; p-value: 0.9237), FA-BMD (beta-estimate: −0.0722; 95% CI: −0.2408 to 0.0963; SE: 0.0860; p-value: 0.4011), and TB-BMD (beta-estimate: −0.0438; 95% CI: −0.0958 to 0.0082; SE: 0.0265; p-value: 0.0989) (Table 1). These conclusions above were also further validated by the MR-Egger analysis, weighted median analysis, and maximum likelihood analysis.
Heterogeneity and Sensitivity Analyses
We analyzed heterogeneity through IVW analysis and applied the MR-Egger regression to analyze the pleiotropy. No heterogeneity for the causal effect of PUFAs on BMD was found in our statistical analysis (e.g., as for the causal effect of omega-3 FAs on LS-BMD: Q=20.5023; heterogeneity p-value=0.4896) (Table 1). According to the intercept values from the MR-Egger regression, no directional pleiotropy was detected for the causal effect of PUFAs on BMD (e.g., omega-6 FAs to LS-BMD: intercept=0.0033, intercept p-value=0.4814; for FN-BMD: intercept=0.0008, intercept p-value=0.8101; for FA-BMD: intercept=0.0011, intercept p-value=0.8722) (Table 1). The MR-PRESSO global test further validated that both outlier and horizontal pleiotropy were not observed in our MR analyses (e.g., omega-6 FAs: p-value=0.083 to LS-BMD; p-value=0.992 to FN-BMD; p-value=0.783 to FA-BMD) (Table 2).
Among the instrumental variables, MR-PRESSO did not identify any outlier for the causal effect between PUFAs and BMD.
MVMR and Bidirectional MR
As shown in the result of MVMR (Table 3), no statistically significant correlations between PUFAs and BMD were observed after adjusting the interactions between metabolites.
As shown in Tables 4, 5, TB-BMD was proven to be negatively related to the omega-6 fatty acids based on the MR-Egger method (beta-estimate: −0.0699; 95% CI: −0.1304 to −0.0095; SE: 0.0308; p-value: 0.0265). No other reverse causations were observed between BMD and PUFAs.
Discussion
The PUFAs contain two main acid types: omega-3 and omega-6 FAs. Omega-3 PUFAs are a group of fatty acids mainly synthesized in the body and maintained through diet, which predominantly include eicosapentaenoic acid (EPA), alpha-linolenic acid (ALA), and docosahexaenoic acid (DHA). Correspondingly, omega-6 fatty acids that are mainly found in various vegetable oils always come from linoleic acid (LA).Recently, several observational studies reported conflicting and discrepant conclusions on the association between PUFAs and BMD. Therein, omega-3 FAs were validated to positively affect bone remodeling via many different processes, including inhibiting osteoclast and promoting osteoblast activities. On the contrary, omega-6 FAs were always thought to be proinflammatory and pernicious to the maintenance of bone health. Accumulating animal experiments have revealed that supplementation of omega-3 FAs could enhance bone density and improve bone quality by various mechanisms. Acting as the specific ligand of peroxisome proliferator-activated receptor γ (PPAR γ), PUFAs could bind to the PPAR γ and induce the differentiation of adipocytes and fatty acids metabolism, which in turn affect the metabolism of the bone tissue (13). In addition, PUFAs could also modulate the formation of inflammatory cytokines to regulate the balance between formation and resorption of the bone via acting on the biosynthetic pathway of prostaglandin E2 (PGE2). Previous studies revealed that PUFAs could regulate the expression or the enzyme activity of cyclooxygenase (COX)-2, which is a rate-limiting enzyme in the synthesis of PGE2. The dual effect of PUFAs is that omega-3 FAs favor the downregulation of COX-2, which leads to the decrease in the production of PGE2 and, furthermore, enhance the formation of the bone. As for omega-6 FAs, it produced the entire opposite effect. PUFAs could also affect the bone marrow microcirculation to reduce the metabolic capacity of the bone. The effect of promoting uptaking of calcium from diet has also been reported. Inconsistent with the conclusion drawn from animal models, the observations from clinical trials still remain controversial.
To the best of our knowledge, this is the first time that the causal association between PUFAs and BMD through a two-sample MR analysis is investigated. Our analysis involved 53,236 individuals of European decent for the association with site-specific BMD, 66,628 individuals for TB-BMD, and 114,999 individuals for PUFAs. Our analytical studies demonstrated that omega-6 fatty acids were proven to be negatively related to the TB-BMD. Moreover, reverse causation was also observed between them. However, after adjusting the interactions between metabolites, no cause and effect association was shown based on the MVMR result. This may suggest that the associations between PUFAs and BMD are likely contributed by other confounding risk factors or the interactions between FAs. To ensure the consistency and reliability of the analysis, our research employed multiple statistical process to check the heterogeneity and control the pleiotropy. We also selected the IVs (F-statistics>10) from the large GWAS data to better represent PUFAs and BMD. In general, our two-sample MR study possessed adequate precision and stability to support the conclusion.
As far as we know, the previous observational studies were always limited to the effect of some specific types of PUFAs on bone health or some particular subtypes of the population, such as post-menopausal women and older people. Furthermore, the intake of dietary fatty acids was usually retrospectively estimated using some questionnaires (40, 41). Thus, the inherent methodological limitation of evaluating the supplementation of fatty acids is unavoidable (42). Due to this, it is not surprising that the previous studies are controversial while still puzzling. Most of the observational studies found that BMD was positively correlated with the supplementation of omega-3 PUFA or fish oil. According to the Women’s Health Initiative Study, positive associations between hip fractures and omega-3 FAs were shown; however, inverse associations were observed between omega-6 FAs, MUFAs, and PUFAs (43). Similarly, in a study of 76,000 women and 45,000 men enrolled, the fracture risk was negatively correlated with the consumption of omega-6 FAs and PUFAs (40). In contrast, a few researchers reported no statistically significant relationship between consumption of PUFAs and BMD or the incidence of fracture (44, 45). Other studies observed completely different findings in which a higher intake of PUFAs may deteriorate bone loss (43). In a recent meta-analysis that enrolled 28 RCTs (7,288 participants), the experimenter reported that the increased supplementation of omega-3 FAs may exert a low magnitude to the increase in BMD of the lumbar spine by 2.6% and femoral neck by 4.1%; however, the grade of evidence was insufficient (46). Another interesting finding that emerged from the analysis is that the increasing intake of total PUFAs may have little to no effect on BMD (46). Recently, in a single-center study of postmenopausal Spanish women, a high level of plasma omega-3 FAs was an independent risk factor of bone health (47).
The vast discrepancy of various studies may be attributed to multiple complex confounders such as sex and gender, etc. One notable confounder is that the consumption of cod liver oil rich in vitamins A and D was likely to exert influence to some degree on bone health (48, 49). Some researchers attempt to explain this phenomenon with more objective and more profound mechanisms, such as circulating fatty acids. Based on the Framingham Osteoporosis Study, which included 765 participants, a negative trend was observed between arachidonic acid (AA) and risk of hip fracture (50). Another cross-sectional study indicated that greater red blood cell omega-3 FAs were beneficial to decrease the risk of hip fracture (51). One important finding is that the influence of fatty acids on BMD may vary dynamically over time, beyond possible sex differences (42).
In accumulating animal experiments, the mechanism of PUFAs affecting bone health could be better elaborated. Deep down to the microlevel, the benefits of fish oil is closely linked to the presence of allelic variants in some genes such as PPAR γ, according to a comparative study on mice with polymorphisms in the PPAR γ gene (6T) (52). On the contrary, no effect of consumption of PUFAs on bone structure or metabolism was found in healthy mice. In another study conducted in ovariectomized rats, the level of PUFAs and ratio of omega-6/omega-3 PUFAs could be the essential important factors for maintaining BMD and bone turnover markers (53). The dietary ratio of 5:1 significantly elevated the amount of DHA in the bone tissues of the femur. This conclusion was also supported by some observational population study; in an investigated population with a higher intake ratio of omega-3 FAs to omega-6 FAs, such as the Japanese population, a lower ratio of osteoporosis was reported (54). In addition, different dietary sources of omega-3 FAs exhibited significant disparities in biochemistry and metabolism. Rozner et al. found that flaxseed oil was effective in ameliorating the micro-architecture, and fish oil could improve BMD, in which the core mechanism may be the alteration of peripheral clock in bone cells (55).
Some study limitations should be noted, although the rationale of MR analyses made it superior to conventional observational studies in excluding the existence of confounders. First, we only focused on the causal associations between a specific type of PUFA and BMD and did not take into consideration some other nutrients that might interact with PUFAs and cause bias. The potential limitation might contribute to the implausible casual relationship between PUFAs and BMD to some extent. Therefore, we conducted MR-Egger and MR-PRESSO methods to exclude the potential pleiotropy. Furthermore, the PhenoScanner tool was adopted to screen and remove the SNPs associated with confounders. Hence, the conclusion of this study should be creditable. Second, the samples were not further substratified according to gender and age, which were believed to be important risk factors of BMD based on previous studies. However, the effect on our analyses could be small due to the strength of the large sample size. Lastly, the exact mechanism underlying the causality between them was not explored in-depth. Therefore, a mechanistic research should be carried out in the future.
Conclusion
This two-sample MR analysis produced strong and new genomic evidence that there was causal relationship between omega-6 FAs and BMD. However, a further validation by MVMR and bidirectional MR suggested that the association between them may be caused by the interactions of metabolites and reverse causality. Further investigations are still required to elucidate the potential mechanism.
Data Availability Statement
The original contributions presented in the study are included in the article/Supplementary Material, further inquiries can be directed to the corresponding author/s.
Author Contributions
LW and XL conducted study design. LW, CZ, and HL conducted data collection and statistical analysis. LW, NZ, TH, and ZZ conducted data interpretation, manuscript preparation, and literature search. LW and XL conducted funds collection. All authors contributed to the article and approved the submitted version.
Funding
This study was funded by the National Natural Science Foundation of China (81873998).
Conflict of Interest
The authors declare that the research was conducted in the absence of any commercial or financial relationships that could be construed as a potential conflict of interest.
Publisher’s Note
All claims expressed in this article are solely those of the authors and do not necessarily represent those of their affiliated organizations, or those of the publisher, the editors and the reviewers. Any product that may be evaluated in this article, or claim that may be made by its manufacturer, is not guaranteed or endorsed by the publisher.
Supplementary Material
The Supplementary Material for this article can be found online at: https://www.frontiersin.org/articles/10.3389/fendo.2022.858851/full#supplementary-material
References
1. Compston JE, McClung MR, Leslie WD. Osteoporosis. Lancet (London England) (2019) 393:364–76. doi: 10.1016/s0140-6736(18)32112-3
2. Ensrud KE, Crandall CJ. Osteoporosis. Ann Internal Med (2017) 167:Itc17–itc32. doi: 10.7326/aitc201708010
3. Burge R, Dawson-Hughes B, Solomon DH, Wong JB, King A, Tosteson A. Incidence and Economic Burden of Osteoporosis-Related Fractures in the United States, 2005-2025. J Bone Miner Res Off J Am Soc Bone Miner Res (2007) 22:465–75. doi: 10.1359/jbmr.061113
4. Harvey N, Dennison E, Cooper C. Osteoporosis: Impact on Health and Economics. Nat Rev Rheumatol (2010) 6:99–105. doi: 10.1038/nrrheum.2009.260
5. Kanis JA, Melton LJ 3rd, Christiansen C, Johnston CC, Khaltaev N. The Diagnosis of Osteoporosis. J Bone Miner Res Off J Am Soc Bone Miner Res (1994) 9:1137–41. doi: 10.1002/jbmr.5650090802
6. WHO Study GroupAssessment of Fracture Risk and its Application to Screening for Postmenopausal Osteoporosis. Report of a WHO Study Group Vol. 843. World Health Organization technical report series (1994) p. 1–129.
7. Morris JA, Kemp JP, Youlten SE, Laurent L, Logan JG, Chai RC, et al. An Atlas of Genetic Influences on Osteoporosis in Humans and Mice. Nat Genet (2019) 51:258–66. doi: 10.1038/s41588-018-0302-x
8. Richards JB, Zheng HF, Spector TD. Genetics of Osteoporosis From Genome-Wide Association Studies: Advances and Challenges. Nat Rev Genet (2012) 13:576–88. doi: 10.1038/nrg3228
9. Yang TL, Shen H, Liu A, Dong SS, Zhang L, Deng FY, et al. A Road Map for Understanding Molecular and Genetic Determinants of Osteoporosis. Nat Rev Endocrinol (2020) 16:91–103. doi: 10.1038/s41574-019-0282-7
10. Alghadir AH, Gabr SA, Al-Eisa ES, Alghadir MH. Correlation Between Bone Mineral Density and Serum Trace Elements in Response to Supervised Aerobic Training in Older Adults. Clin Interventions Aging (2016) 11:265–73. doi: 10.2147/cia.S100566
11. Bhattarai T, Bhattacharya K, Chaudhuri P, Sengupta P. Correlation of Common Biochemical Markers for Bone Turnover, Serum Calcium, and Alkaline Phosphatase in Post-Menopausal Women. Malaysian J Med Sci (2014) 21(1):58–61.
12. Maggio D, Polidori MC, Barabani M, Tufi A, Ruggiero C, Cecchetti R, et al. Low Levels of Carotenoids and Retinol in Involutional Osteoporosis. Bone (2006) 38:244–8. doi: 10.1016/j.bone.2005.08.003
13. Wauquier F, Léotoing L, Philippe C, Spilmont M, Coxam V, Wittrant Y. Pros and Cons of Fatty Acids in Bone Biology. Prog Lipid Res (2015) 58:121–45. doi: 10.1016/j.plipres.2015.03.001
14. Bao M, Zhang K, Wei Y, Hua W, Gao Y, Li X, et al. Therapeutic Potentials and Modulatory Mechanisms of Fatty Acids in Bone. Cell Prolif (2020) 53:e12735. doi: 10.1111/cpr.12735
15. Longo AB, Ward WE. PUFAs, Bone Mineral Density, and Fragility Fracture: Findings From Human Studies. Adv Nutr (Bethesda Md.) (2016) 7:299–312. doi: 10.3945/an.115.009472
16. Lipina C, Hundal HS. Lipid Modulation of Skeletal Muscle Mass and Function. J Cachexia Sarcopenia Muscle (2017) 8:190–201. doi: 10.1002/jcsm.12144
17. Mangano KM, Sahni S, Kerstetter JE, Kenny AM, Hannan MT. Polyunsaturated Fatty Acids and Their Relation With Bone and Muscle Health in Adults. Curr Osteoporosis Rep (2013) 11:203–12. doi: 10.1007/s11914-013-0149-0
18. Tachtsis B, Camera D, Lacham-Kaplan O. Potential Roles of N-3 PUFAs During Skeletal Muscle Growth and Regeneration. Nutrients (2018) 10:309. doi: 10.3390/nu10030309
19. Haag M, Magada ON, Claassen N, Böhmer LH, Kruger MC. Omega-3 Fatty Acids Modulate ATPases Involved in Duodenal Ca Absorption. Prostaglandins Leukotrienes Essential Fatty Acids (2003) 68:423–9. doi: 10.1016/s0952-3278(03)00067-x
20. Maurin AC, Chavassieux PM, Meunier PJ. Expression of PPARgamma and Beta/Delta in Human Primary Osteoblastic Cells: Influence of Polyunsaturated Fatty Acids. Calcified Tissue Int (2005) 76:385–92. doi: 10.1007/s00223-004-0108-y
21. Emdin C, Khera A, Kathiresan S. Mendelian Randomization. JAMA (2017) 318:1925–6. doi: 10.1001/JAMA.2017.17219
22. Lawlor DA, Harbord RM, Sterne JA, Timpson N, Davey Smith G. Mendelian Randomization: Using Genes as Instruments for Making Causal Inferences in Epidemiology. Stat Med (2008) 27:1133–63. doi: 10.1002/sim.3034
23. Hemani G, Zheng J, Elsworth B, Wade KH, Haberland V, Baird D, et al. The MR-Base Platform Supports Systematic Causal Inference Across the Human Phenome. eLife (2018) 7:e34408. doi: 10.7554/eLife.34408
24. Abecasis GR, Altshuler D, Auton A, Brooks LD, Durbin RM, Gibbs RA, et al. Map of Human Genome Variation From Population-Scale Sequencing. Nature (2010) 467:1061–73. doi: 10.1038/nature09534
25. Kamat MA, Blackshaw JA, Young R, Surendran P, Burgess S, Danesh J, et al. PhenoScanner V2: An Expanded Tool for Searching Human Genotype-Phenotype Associations. Bioinf (Oxford England) (2019) 35:4851–3. doi: 10.1093/bioinformatics/btz469
26. Staley JR, Blackshaw J, Kamat MA, Ellis S, Surendran P, Sun BB, et al. PhenoScanner: A Database of Human Genotype-Phenotype Associations. Bioinf (Oxford England) (2016) 32:3207–9. doi: 10.1093/bioinformatics/btw373
27. Burgess S, Small DS, Thompson SGA. Review of Instrumental Variable Estimators for Mendelian Randomization. Stat Methods Med Res (2017) 26:2333–55. doi: 10.1177/0962280215597579
28. Burgess S, Thompson SG. Avoiding Bias From Weak Instruments in Mendelian Randomization Studies. Int J Epidemiol (2011) 40:755–64. doi: 10.1093/ije/dyr036
29. Sudlow C, Gallacher J, Allen N, Beral V, Burton P, Danesh J, et al. UK Biobank: An Open Access Resource for Identifying the Causes of a Wide Range of Complex Diseases of Middle and Old Age. PLoS Med (2015) 12:e1001779. doi: 10.1371/journal.pmed.1001779
30. Julkunen H, Cichońska A, Slagboom PE, Würtz P. Metabolic Biomarker Profiling for Identification of Susceptibility to Severe Pneumonia and COVID-19 in the General Population. eLife (2021) 10:e63033. doi: 10.7554/eLife.63033
31. Zheng HF, Forgetta V, Hsu YH, Estrada K, Rosello-Diez A, Leo PJ, et al. Whole-Genome Sequencing Identifies EN1 as a Determinant of Bone Density and Fracture. Nature (2015) 526:112–7. doi: 10.1038/nature14878
32. Medina-Gomez C, Kemp JP, Trajanoska K, Luan J, Chesi A, Ahluwalia TS, et al. Life-Course Genome-Wide Association Study Meta-Analysis of Total Body BMD and Assessment of Age-Specific Effects. Am J Hum Genet (2018) 102:88–102. doi: 10.1016/j.ajhg.2017.12.005
33. Burgess S, Butterworth A, Thompson SG. Mendelian Randomization Analysis With Multiple Genetic Variants Using Summarized Data. Genet Epidemiol (2013) 37:658–65. doi: 10.1002/gepi.21758
34. Bowden J, Del Greco MF, Minelli C, Davey Smith G, Sheehan NA, Thompson JR. Assessing the Suitability of Summary Data for Two-Sample Mendelian Randomization Analyses Using MR-Egger Regression: The Role of the I2 Statistic. Int J Epidemiol (2016) 45:1961–74. doi: 10.1093/ije/dyw220
35. Burgess S, Thompson SG. Interpreting Findings From Mendelian Randomization Using the MR-Egger Method. Eur J Epidemiol (2017) 32:377–89. doi: 10.1007/s10654-017-0255-x
36. Bowden J, Davey Smith G, Haycock PC, Burgess S. Consistent Estimation in Mendelian Randomization With Some Invalid Instruments Using a Weighted Median Estimator. Genet Epidemiol (2016) 40:304–14. doi: 10.1002/gepi.21965
37. Pierce BL, Burgess S. Efficient Design for Mendelian Randomization Studies: Subsample and 2-Sample Instrumental Variable Estimators. Am J Epidemiol (2013) 178:1177–84. doi: 10.1093/aje/kwt084
38. Verbanck M, Chen CY, Neale B, Do R. Detection of Widespread Horizontal Pleiotropy in Causal Relationships Inferred From Mendelian Randomization Between Complex Traits and Diseases. Nat Genet (2018) 50:693–8. doi: 10.1038/s41588-018-0099-7
39. Hemani G, Tilling K, Davey Smith G. Orienting the Causal Relationship Between Imprecisely Measured Traits Using GWAS Summary Data. PLoS Genet (2017) 13:e1007081. doi: 10.1371/journal.pgen.1007081
40. Virtanen JK, Mozaffarian D, Willett WC, Feskanich D. Dietary Intake of Polyunsaturated Fatty Acids and Risk of Hip Fracture in Men and Women. Osteoporosis Int J Established as Result Cooperation Between Eur Foundation Osteoporosis Natl Osteoporosis Foundation USA (2012) 23:2615–24. doi: 10.1007/s00198-012-1903-3
41. Järvinen R, Tuppurainen M, Erkkilä AT, Penttinen P, Kärkkäinen M, Salovaara K, et al. Associations of Dietary Polyunsaturated Fatty Acids With Bone Mineral Density in Elderly Women. Eur J Clin Nutr (2012) 66:496–503. doi: 10.1038/ejcn.2011.188
42. Harris TB, Song X, Reinders I, Lang TF, Garcia ME, Siggeirsdottir K, et al. Plasma Phospholipid Fatty Acids and Fish-Oil Consumption in Relation to Osteoporotic Fracture Risk in Older Adults: The Age, Gene/Environment Susceptibility Study. Am J Clin Nutr (2015) 101:947–55. doi: 10.3945/ajcn.114.087502
43. Orchard TS, Cauley JA, Frank GC, Neuhouser ML, Robinson JG, Snetselaar L, et al. Fatty Acid Consumption and Risk of Fracture in the Women's Health Initiative. Am J Clin Nutr (2010) 92:1452–60. doi: 10.3945/ajcn.2010.29955
44. Salari Sharif P, Asalforoush M, Ameri F, Larijani B, Abdollahi M. The Effect of N-3 Fatty Acids on Bone Biomarkers in Iranian Postmenopausal Osteoporotic Women: A Randomized Clinical Trial. Age (Dordrecht Netherlands) (2010) 32:179–86. doi: 10.1007/s11357-009-9122-3
45. Virtanen JK, Mozaffarian D, Cauley JA, Mukamal KJ, Robbins J, Siscovick DS. Fish Consumption, Bone Mineral Density, and Risk of Hip Fracture Among Older Adults: The Cardiovascular Health Study. J Bone Miner Res Off J Am Soc Bone Miner Res (2010) 25:1972–9. doi: 10.1002/jbmr.87
46. Abdelhamid A, Hooper L, Sivakaran R, Hayhoe RPG, Welch A. The Relationship Between Omega-3, Omega-6 and Total Polyunsaturated Fat and Musculoskeletal Health and Functional Status in Adults: A Systematic Review and Meta-Analysis of RCTs. Calcified Tissue Int (2019) 105:353–72. doi: 10.1007/s00223-019-00584-3
47. Roncero-Martín R, Aliaga I, Moran JM, Puerto-Parejo LM, Rey-Sánchez P, de la Luz Canal-Macías M, et al. Plasma Fatty Acids and Quantitative Ultrasound, DXA and pQCT Derived Parameters in Postmenopausal Spanish Women. Nutrients (2021) 13:1454. doi: 10.3390/nu13051454
48. Bischoff-Ferrari HA, Willett WC, Wong JB, Giovannucci E, Dietrich T, Dawson-Hughes B. Fracture Prevention With Vitamin D Supplementation: A Meta-Analysis of Randomized Controlled Trials. JAMA (2005) 293:2257–64. doi: 10.1001/JAMA.293.18.2257
49. Feskanich D, Singh V, Willett WC, Colditz GA. Vitamin A Intake and Hip Fractures Among Postmenopausal Women. JAMA (2002) 287:47–54. doi: 10.1001/JAMA.287.1.47
50. Farina EK, Kiel DP, Roubenoff R, Schaefer EJ, Cupples LA, Tucker KL. Plasma Phosphatidylcholine Concentrations of Polyunsaturated Fatty Acids are Differentially Associated With Hip Bone Mineral Density and Hip Fracture in Older Adults: The Framingham Osteoporosis Study. J Bone Miner Res Off J Am Soc Bone Miner Res (2012) 27:1222–30. doi: 10.1002/jbmr.1581
51. Orchard TS, Ing SW, Lu B, Belury MA, Johnson K, Wactawski-Wende J, et al. The Association of Red Blood Cell N-3 and N-6 Fatty Acids With Bone Mineral Density and Hip Fracture Risk in the Women's Health Initiative. J Bone Miner Res Off J Am Soc Bone Miner Res (2013) 28:505–15. doi: 10.1002/jbmr.1772
52. Bonnet N, Somm E, Rosen CJ. Diet and Gene Interactions Influence the Skeletal Response to Polyunsaturated Fatty Acids. Bone (2014) 68:100–7. doi: 10.1016/j.bone.2014.07.024
53. Watkins BA, Li Y, Seifert MF. Dietary Ratio of N-6/N-3 PUFAs and Docosahexaenoic Acid: Actions on Bone Mineral and Serum Biomarkers in Ovariectomized Rats. J Nutr Biochem (2006) 17:282–9. doi: 10.1016/j.jnutbio.2005.05.012
54. Paunescu AC, Dewailly E, Dodin S, Nieboer E, Ayotte P. Dioxin-Like Compounds and Bone Quality in Cree Women of Eastern James Bay (Canada): A Cross-Sectional Study. Environ Health Global Access Sci Source (2013) 12:54. doi: 10.1186/1476-069x-12-54
Keywords: polyunsaturated fatty acids - PUFA, bone mineral density—BMD, mendelian randomization, osteoporosis, omega - 3 fatty acids
Citation: Wang L, Zhang C, Liang H, Zhou N, Huang T, Zhao Z and Luo X (2022) Polyunsaturated Fatty Acids Level and Bone Mineral Density: A Two-Sample Mendelian Randomization Study. Front. Endocrinol. 13:858851. doi: 10.3389/fendo.2022.858851
Received: 20 January 2022; Accepted: 31 May 2022;
Published: 08 July 2022.
Edited by:
Alberto Falchetti, Italian Auxological Institute (IRCCS), ItalyReviewed by:
Maria Lucia Fleiuss Farias, Federal University of Rio de Janeiro, BrazilPing Zeng, Xuzhou Medical University, China
Copyright © 2022 Wang, Zhang, Liang, Zhou, Huang, Zhao and Luo. This is an open-access article distributed under the terms of the Creative Commons Attribution License (CC BY). The use, distribution or reproduction in other forums is permitted, provided the original author(s) and the copyright owner(s) are credited and that the original publication in this journal is cited, in accordance with accepted academic practice. No use, distribution or reproduction is permitted which does not comply with these terms.
*Correspondence: Xiaoji Luo, Y3kyOTgyQDE2My5jb20=