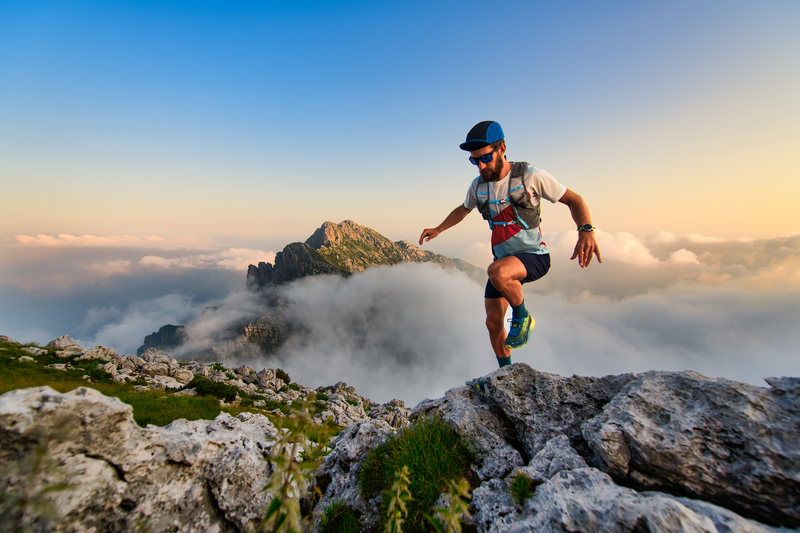
94% of researchers rate our articles as excellent or good
Learn more about the work of our research integrity team to safeguard the quality of each article we publish.
Find out more
REVIEW article
Front. Endocrinol. , 17 June 2022
Sec. Pediatric Endocrinology
Volume 13 - 2022 | https://doi.org/10.3389/fendo.2022.858832
This article is part of the Research Topic The Problem of Childhood Hypoglycemia View all 14 articles
Hypoglycemia results from an imbalance between glucose entering the blood compartment and glucose demand, caused by a defect in the mechanisms regulating postprandial glucose homeostasis. Hypoglycemia represents one of the most common metabolic emergencies in childhood, potentially leading to serious neurologic sequelae, including death. Therefore, appropriate investigation of its specific etiology is paramount to provide adequate diagnosis, specific therapy and prevent its recurrence. In the absence of critical samples for biochemical studies, etiological assessment of children with hypoglycemia may include dynamic methods, such as in vivo functional tests, and continuous glucose monitoring. By providing detailed information on actual glucose fluxes in vivo, proof-of-concept studies have illustrated the potential (clinical) application of dynamic stable isotope techniques to define biochemical and clinical phenotypes of inherited metabolic diseases associated with hypoglycemia. According to the textbooks, individuals with glycogen storage disease type I (GSD I) display the most severe hypoglycemia/fasting intolerance. In this review, three dynamic methods are discussed which may be considered during both diagnostic work-up and monitoring of children with hypoglycemia: 1) functional in vivo tests; 2) in vivo metabolic profiling by continuous glucose monitoring (CGM); 3) stable isotope techniques. Future applications and benefits of dynamic methods in children with hypoglycemia are also discussed.
Appropriate investigation of the etiology and simultaneous management in children with hypoglycemia is paramount to prevent (irreversible) brain injury or even death (1), although controversy remains on the definition (e.g., diagnostic plasma glucose threshold, definition of at–risk neonates) of childhood hypoglycemia (2–4). From a pathophysiological perspective, hypoglycemia is caused by an imbalance between the amount of glucose molecules entering the blood compartment and the glucose clearance. A continuous supply of glucose is crucial for the function and development of the brain, because of the absence of sufficient cerebral energy stores in the form of glycogen, protein or fat. Glucose oxidation is responsible for ~ 70% of the energy production in the brain, whereas lactate and ketone bodies can function as important alternative fuels. Hypoglycemia causes energy deficiency in brain cells ultimately leading to brain cell death due to secondary molecular mechanisms, such as activation of neuronal glutamate receptors, oxidative stress, neuronal zinc release and activation of poly-ADP-ribose polymerase-1 (5, 6). Clinically, untreated hypoglycemia can lead to serious neurologic morbidity (encephalopathy, convulsions, coma, developmental delay), and even mortality (7). In individuals with a possible, underlying disorder, unrecognized hypoglycemia may accelerate and aggravate the metabolic decompensation resulting in poor outcomes.
Multiple metabolic pathways cooperate to maintain normal blood glucose concentrations in the fed and fasted state, such as glycogenolysis, gluconeogenesis, mitochondrial fatty acid oxidation, ketogenesis and ketolysis, ensuring energy homeostasis. These pathways are tightly regulated by hormonal (e.g., insulin, glucagon, cortisol, and growth hormone) and autonomic (e.g., catecholamines) responses (8) to maintain normoglycemia. Besides acquired conditions (e.g., insufficient supply/increased loss of nutrients, dysmaturity, drugs, hypothermia, sepsis), hypoglycemia may be explained by a genetic defect of these mechanisms that normally guarantee glucose homeostasis during feeding and fasting. Hypoglycemia is an important feature of several inherited metabolic disorders (IMDs) characterized by a history of childhood fasting intolerance (9).
Compared to adults, children are at an increased risk of developing hypoglycemia, as they have smaller hepatic glycogen and muscle protein stores, together with an increased energy expenditure and higher brain/body weight ratio (10). Consequently, most young children are not able to maintain normoglycemia during more than 24 hours of fasting (11–16). Studies with stable isotope techniques have quantified endogenous glucose production (EGP) in newborns (17) and older children (18). Huidekoper et al. designed a non-linear regression model for EGP from infancy to adulthood (19). Table 1 illustrates that glucose homeostasis is tightly controlled in an age-dependent manner, with a small glucose pool in the blood compartment (row D) and high glucose turnover rate (row E).
The most used diagnostic approach to childhood hypoglycemia consists of pattern recognition by combining a variety of information, including medical history (e.g., age at onset, relation with food), physical examination (e.g., growth charts, liver size), and laboratory tests. If critical samples (i.e. samples collected during metabolic stress) of plasma and urine can be obtained, static or single-point concentrations of biomarkers (e.g., blood glucose, lactate, gases, free fatty acids, ketones, acylcarnitines, amino acids, insulin, cortisol, growth hormone, and urine organic acids) can provide crucial information to establish an etiological diagnosis (21). When critical samples are not available or inconclusive, next-generation sequencing may further direct the diagnostic process. Several diagnostic algorithms exist for the clinical problem of childhood hypoglycemia (1, 3), but these schemes are often not sufficiently specific. Moreover, they underestimate the complexity of the swiftly changing clinical signs and concentrations of different fuels in the blood compartment. Hence, many hypoglycemic children do not fit these textbook schemes, and additional investigation is often required. In the end, an important group of individuals is diagnosed with idiopathic ketotic hypoglycemia (22, 23).
In this narrative review, we illustrate several dynamic methods which may be considered in the diagnostic work-up and monitoring of children with hypoglycemia. Three methods are presented: 1) functional in vivo tests; 2) in vivo metabolic profiling by continuous glucose monitoring (CGM); 3) stable isotope tracing, focusing on glycogen storage disease type I (GSD I). Future applications and benefits of dynamic methods in children with hypoglycemia are also discussed.
For decades, functional in vivo tests have been used for the etiological clarification of fasting intolerance, unexplained hypoglycemia, and to diagnose specific IMDs (Table 2). In principle, these tests were particularly relevant as biochemical abnormalities might only appear intermittently in many IMDs. In times when diagnostic enzymatic studies and DNA studies were not routinely available, assessment of blood lactate curves after hexose (e.g. glucose, fructose and galactose) loading was used as a screening procedure in patients suspected of hepatic GSDs (24–26). The results of these tests combined with the assessment of response of blood glucose to glucagon injection provided major clues to identify the hepatic GSD subtype. As an example, the combination of increased blood lactate levels observed after oral glucose administration together with no response of blood glucose to intramuscular glucagon injection pointed to GSD III. Subsequently, the abnormally increasing lactate curves after ingestion of both fructose and galactose in GSD I patients has become one of the pillars, on which the current dietary recommendations are based. If performed, functional in vivo tests are conducted strictly adhering to a defined protocol to maximize the reliability and minimize the risks for patients. Functional in vivo tests should only be performed in experienced centers and under close medical supervision. Protocols for several functional in vivo (provocation and/or loading) tests can be found elsewhere (9, 27).
Table 2 Main in vivo functional tests used in children with hypoglycemia. Historical indications are shown.
In theory, evaluation of metabolic changes in response to an explorative or extended controlled fasting test may be helpful to reach the etiological diagnosis in children with hypoglycemia. Fasting induces several hormonal and metabolic responses to ensure an endogenous supply of energy after cessation of exogenous intake (28). Due to the complexity of the (patho)physiological response to fasting, complete hormonal and metabolic investigations should be performed before and at the end of the monitored fasting. Spare serum/urine samples should also be stored for any additional tests to be performed afterwards. Bedside monitoring of these laboratory parameters, and in particular during the extended controlled fasting test, vital parameter monitoring and CGM should also be performed. Under these circumstances, the maximal duration of the fasting is based on the clinical history (expected fasting tolerance) and on the child’s age (usually 12-16 hours at 6-12 months, 18 hours at 1-2 years, 20 hours at 2-7 years, 24 hours in children > 7 years). The fasting should be stopped at any time if the glucose concentration is below 2.6 mmol/L or the patient develops symptoms of hypoglycemia (21).
Even before the era of expanded newborn screening and next generation sequencing, the diagnostic yield of fasting studies has been limited. Morris et al. confirmed an endocrine disorder (n=15) or an IMD (n=15) in a group of 138 patients undergoing fasting studies (29). Bappal and Mula-Abed diagnosed an endocrine disorder (n=21) or an IMDs (n=31) in 96 patients subjected to diagnostic fasting tests (30). Both studies did not provide detailed information on confirmatory diagnostic testing in their patients. More recently Graves et al. showed that 4/91 fasting challenges performed in children with suspected or documented hypoglycemia resulted in an etiological diagnosis (i.e., hepatic GSD, partial ACTH deficiency, panhypopituitarism, isolated central hypothyroidism) (31).
Although in vivo functional tests have the advantage of triggering metabolic stress in a supervised setting, they are burdensome and inconvenient for young patients and their parents. If IMDs are not yet excluded, these tests can cause serious complications in unexperienced hands (e.g., worsening of hypoglycemia, accumulation of toxic metabolites). Therefore, psychological burden due to unexpected hypoglycemia for patients and their families should be balanced against the stress and risks associated with in vivo functional testing. Additionally, in vivo functional tests pose a significant organizational and logistical burden (e.g., need for a fully equipped laboratory and a dedicated medical team) by including several samples to be collected at specific time points (before and after the challenge), under strictly supervised conditions, and requiring hospital admission.
Based on these considerations and because indications have become more restricted, most of the in vivo functional tests have fallen into disuse. A few tests are nowadays even abandoned and considered obsolete (9). Historical indications for functional in vivo tests have been shifted with the increased awareness for rare diseases, the development of diagnostic algorithms and refined basal metabolic investigations (such as organic acid analysis, plasma acylcarnitines, specific enzyme analysis, in vitro metabolic flux analysis in cultured skin fibroblasts), introduction of expanded population newborn screening, and implementation of next-generation sequencing. Still, some functional in vivo tests -with slight modifications - may be useful to provide phenotypic information to support genetic variant classification, or to define outcome in clinical trials. In such situations, these tests remain the major (monitoring) methods allowing the assessment of metabolic changes in vivo in response to external factors (e.g., metabolic stress, treatment). In addition, controlled fasting test may guide the diagnostic process in suspected congenital hyperinsulinism when critical samples are not available (e.g., molecular genetic testing, 18F-DOPA positron emission tomography) (32, 33).
Continuous glucose monitoring (CGM) constitutes another dynamic method in the diagnostic work-up and monitoring of children with hypoglycemia. Progress has been made since CGM was firstly described in 1979 for a patient with insulinoma (34). Modern CGM devices consist of a subcutaneous sensor and a receiver. A wearable sensor is attached to the skin and measures glucose (with variable frequency) through a subcutaneous needle inserted in the interstitial fluid. Via an enzymatic technology (glucose oxidase), the sensor generates an electric current proportional to the glucose concentration in the interstitium. The electric current flows through a continuously shifting algorithm to generate a glucose value which is eventually transmitted to the receiver (receiving unit or compatible smartphone). The algorithm is finetuned via calibration by patient or factory to ensure accuracy over the recording periods (35). Major advantages of CGM are the availability of real-time data, continuous data (density), alarming function, at home use, and data-sharing with caregivers and health care providers, and it being a relatively non-invasive technique (no or fewer finger sticks).
Currently, CGM devices are only licensed for patients with diabetes mellitus (DM), for whom CGM-related outcome parameters (e.g., time-in-range and time-above-range) are defined to guide dietary changes and/or medication adjustments (36). Additionally, CGM has been used in clinical research to assess glucose metabolism in various conditions, including acute coronary syndrome (37) and individuals following an intermittent fasting dietary regimen (38). Evidence on its benefit is also accumulating in endocrine disorders (39, 40) and IMDs associated with hypoglycemia (41), particularly in hepatic GSDs (Table 3).
A significant correlation between CGM values and capillary glucose values has been found in patients with GSD I (42, 46). CGM can unveil both unrecognized (nocturnal) hypoglycemia and hyperglycemia and hence, provide information on the effect of the dietary regimen on glucose homeostasis in patients with hepatic GSDs (43). By providing visual glucose trends, CGM also has the advantage of generating dynamic, daily data compared to traditional single-point data. Thus, it can improve the home site monitoring (constituting an easier tool for patients compared to traditional methods) (44, 47) and the day-to-day healthcare for patients with hepatic GSDs (45, 46). CGM has also proven a reliable tool to assess the effect of novel/optimized dietary and/or medical treatments in patients with hepatic GSDs (48, 49). Additionally, it can assist the physicians in the assessment of children with hypoglycemia in combination with relevant information on personal and dietary history and appropriate biochemical investigations.
For patients with ketotic fasting intolerance (e.g., ketotic GSDs, idiopathic ketotic hypoglycemia, or any situation in which euglycemic ketonemia may occur, such as patients on ketogenic diets), parallel documentation of point-of-care capillary beta-hydroxybutyrate (BHB) concentrations by a portable ketone meter may provide important additional information. To the best of our knowledge, reference values for capillary BHB during the day are not available in the pediatric population, but information on BHB normal values after overnight fasting can be extrapolated from historical publications (Table 4). Most studies provide BHB concentrations after prolonged fasting, but recently, Parmar et al. demonstrated that serum point-of-care (POCT) BHB measurement using a precision Xtra meter are comparable to serum BHB concentrations in healthy children after an overnight fast (51). In 94 children (age range 6 months to 18.7 years) scheduled to undergo elective surgery, POCT BHB concentrations ranged from 0 to 1.1 mmol/l, but unfortunately, detailed information on the correlation between age and BHB concentrations was not provided in their study.
Table 4 Previous studies assessing ketones and glucose concentrations after prolonged fasting in healthy children.
Currently, only the BHB meters can be used for minimally invasive home monitoring to guide dietary management after a working diagnosis has been established. To our opinion, the combination of real-time CGM with alarm function and a portable ketone meter may be used in children undergoing parallel diagnostic and therapeutic metabolic profiling for hypoglycemic disorders [(52) and Table 5]. A hybrid protocol combining the exploration of fasting tolerance (by CGM) with so-called in vivo metabolic profiling (BHB and possibly additional biochemical parameters) may replace the historical approach in patients suspected with hepatic GSDs. Such approach will likely further decrease the use of diagnostic in vivo fasting tests. Indeed, the combination of information collected through different testing approaches will likely represent the future strategy to characterize childhood hypoglycemia, including both the diagnostic and monitoring phase. Additional factors (e.g., experience of the medical team, patients’ needs, available resources, insurance policy) may result in variations in local practice, even between specialized centers.
The flow of metabolites through a (disrupted) metabolic pathway is determined by both the rate of supply of substrates and the residual activities of the enzymes in the pathway. In patients with IMDs, changes in the distribution of fluxes occur secondary to enzyme defect(s) and/or altered substrate availability. By assessing the flow of metabolites through specific metabolic pathways in vivo, fluxomics can provide an overall description of tissue phenotypes in a relatively simple manner (53). (Radioactive or stable) isotope tracers have been used since 1930s to characterize such changes in cellular and whole-body metabolism (54). Stable isotopes techniques are well established methods in fundamental/translational research to assess metabolic fluxes/changes (55, 56). Proof-of-concept research has also shown the potential clinical (research and care) application of stable isotopes to characterize the biochemical and clinical phenotype of IMDs associated with hypoglycemia (57, 58). An overview on current evidence paving the path for future use of stable isotope techniques in diagnosis and monitoring of IMDs characterized by hypoglycemia is provided, with specific focus on GSD I.
The core assumption of isotopic methods is that a tracer [i.e., a substance that can be administered in order to follow a natural substance (tracee)] is metabolically indistinguishable from the tracee (59). Ideally, the amount of the administered tracer is sufficiently low to avoid any effect on the physiological process investigated (59). Flux calculations are often performed when the system is in a steady state (i.e., a condition in which the tracer is infused continuously and its dilution in the analyzed compartment (e.g., plasma) remains constant over time). In this condition, blood samples are collected before the tracer intravenous infusion (after a priming dose) and several times after achieving the steady state (60).
Two types of tracers can be used: those that contain radioisotopes or those that contain stable isotopes. Radioisotopes are isotopes which release radiation as a by-product of their decay. The energy released during the decay can be measured, following the path of the tracer from reactants to products. Although such tracers have been widely used up to the 1970s (61), the health risk associated with radioactive tracers in humans and the development of affordable mass spectrometers led to the greater use of stable isotope tracers (62). Stable isotopes do not emit radiation and can be easily detected by mass spectrometry. Stable isotope tracers employed to study carbohydrate metabolism usually contain a hydrogen isotope (e.g., D-[6,6-2H2]-glucose, or D-[1-2H]- galactose) or a carbon isotope (e.g., uniformly labelled glucose D-[U-13C]-glucose or [2-13C]- glycerol). Since tracer loss without net conversion to product, that may be due to recycling (for example in case of recycling of D-[2-2H2]-glucose by conversion of glucose-6-phosphate to fructose-6-phosphate and back), may occur at specific metabolic steps, appropriate selection of the tracer is crucial for accurate analysis (63). The use of D-[6,6-2H2]-glucose or D-[U-13C]-glucose may in part overcome the pitfalls associated with other tracers (64). Given that each stable isotope is naturally found in the environment and in the body at a low concentration, it is important to take into account its natural abundance in order to determine the degree of tracer enrichment (65). Also, it is necessary that the degree of enrichment is high enough to be measured. The enrichment is calculated as a fraction: [tracer]/([tracer]+[tracee]) and is usually expressed as mole percent excess (MPE) (63).
For glucose metabolism during fasting, at biological steady state the rate of appearance of glucose into the circulation (Ra) represents endogenous glucose production (EGP) in the absence of an additional input of glucose. Moreover, at this steady state Ra equals the rate of glucose disposal (Rd). Experimentally, Ra is estimated during a continuous infusion of a solution of labeled glucose from the measured dilution of stable isotope labeled glucose (for example D-[U-13C]-glucose) in the pool of plasma glucose at isotopic steady state. In general, the rate of infusion of labeled glucose (I) cannot be neglected in comparison with Ra. Therefore, during a stable isotope tracer infusion experiment the total rate of appearance of glucose (Rt) into the circulation is considered as, the sum of Ra and I: Rt = Ra + I = Rd. At isotopic steady state, the Rt can be calculated from the dilution of infused labelled glucose in the plasma glucose pool, as the ratio of fractional isotopologue enrichment of infusate over the fractional isotopologue enrichment of blood glucose, multiplied with the infusion rate. When D-[U-13C]-glucose, with 6 13C carbon atoms, is applied as a glucose label . Then Ra can be calculated as: . (59).
However, there are situations in which additional variables play a role and in which tracer enrichment can vary (non-steady state). For example, inflow of glucose (e.g., from the intestine in the post-absorptive state, or additional glucose infusion) or hormonal response (e.g., insulin, cortisol) drives glucose through different compartments before entering (and possibly re-entering) the bloodstream. Such complex conditions can still be simplified to a single-compartment model by including a pool volume of the total blood glucose pool and the changes in the [tracer]/([tracer]+[tracee]) enrichments over time in the calculations (66). Still, changes in the pool volume over time and equilibration rate of the tracer between compartments can result in aberrant results when using this approach. Frequent sampling and appropriate data fitting can minimize such errors (59). Alternatively, the pool volume can be measured (e.g., by infusing two or even three different isotopes), requiring more complex experimental designs (67).
Stable isotopes have been widely used to study the in vivo dynamics of glucose metabolism and conditions associated with hypoglycemia. As previously mentioned, Ra and Rd are the key variables when performing tracer studies. For blood glucose, Ra reflects the EGP in fasted conditions in the absence of any exogenous input [e.g. (e.g., glucose influx from the intestine, or additional unlabeled glucose infusion]. Physiologically, two main biochemical processes, namely gluconeogenesis and glycogenolysis, account for EGP (68). Conversely, Rd represents glucose uptake from the blood by the liver and other organs, and is determined by several metabolic pathways, including glycolysis and glycogen synthesis.
In 1977, Bier et al. investigated glucose turnover by using stable isotope tracers for the first time (20). Particularly, D-[6,6-2H2]-glucose intravenous infusion allowed quantification of EGP in children (with either hypoglycemia or normal glucose homeostasis) and (normoglycemic) adults, revealing that 1) physiologically EGP is higher in children than adults when normalized for body weight and 2) a linear correlation between EGP and brain weight exists in children (20). This study set the stage for further in-depth research on childhood hypoglycemia assessing glucose kinetics with stable isotope methods (69–71). D-[6,6-2H2]-glucose intravenous infusion has been used to investigate glucose kinetics in idiopathic ketotic hypoglycemia. Particularly, it has been clarified that this condition is due to impaired EGP (rather than increased glucose utilization) (72) secondary to insufficient increase in gluconeogenesis (71). In a single patient with congenital hyperinsulinism increased EGP and glycogenolysis after glucagon administration were demonstrated. Specifically, increased glucose Ra (assessed by D-[6,6-2H2]-glucose intravenous infusion) and decreased glycerol Ra [assessed by [2-13C]-glycerol intravenous infusion (73)] were noted. Additionally, various techniques to estimate in vivo rates of gluconeogenesis and/or glycogenolysis with stable isotopes have been developed (74).
As the liver accounts for ∼80% of EGP (with the rest accounted for mainly by the kidneys), a particular interest has developed to assess glucose kinetics with stable isotopes tracers in IMDs in which hypoglycemia is mainly due to the enzyme defect in the liver. A detailed report on the use of stable isotope methodology in IMDs goes beyond the scope of the present work [see for a review (75)]. Specific results on selected IMDs associated with hypoglycemia are presented here, with a special focus on GSD I.
Flux analysis in a mouse model of medium chain acyl-CoA dehydrogenase deficiency (MCADD) has shown that pharmacological inhibition of mitochondrial fatty acid oxidation does not affect EGP (76). Insufficient increase of gluconeogenesis upon hypoglycemia has been observed in long-chain acyl-CoA dehydrogenase-deficient mice (77). Observations on a patient with propionic acidemia showed elevated carbohydrate utilization both at rest and during exercise, suggesting that these patients rely more on carbohydrates as energy source than healthy volunteers (78). Research on hereditary fructose intolerance (HFI) showed that aldolase B contributes for around 50% of the fructose to glucose conversion in healthy subjects and that children with HFI exhibit a significantly lower conversion of fructose to glucose (79).
Several studies have made use of stable isotope tracer methodology to investigate glucose kinetics in GSD I, both in rodent models (80, 81) and humans (57, 82–88). GSD I (MIM# 232200) is an inherited disorder of glycogen metabolism due to a defect in the glucose 6-phosphatase (G6Pase) system. Defects either in the catalytic subunit (G6Pase-α, G6PC1) or in the microsomal glucose 6-phosphate transporter (G6PT, SLC37A4) cause GSD Ia and GSD Ib, respectively. Impaired glycogenolysis and gluconeogenesis result in fasting intolerance with hypoglycemia, elevated lactate, metabolic acidosis and secondary metabolic derangements. Uncooked cornstarch (UCCS) or extended-release cornstarch (Glycosade®) and/or continuous gastric drip-feeding is the cornerstone of the treatment (52).
Assessing EGP in GSD I has been one of the key research objectives for decades (57, 80, 82–88). After evidence on residual EGP was first provided in two patients with GSD I (89), several studies have made use of stable isotope methods to quantify glucose production in GSD I patients (57, 82–88). Those studies showed that, despite G6Pase deficiency, a limited (up to ∼60% of normal) capacity of EGP could be found in GSD Ia. Yet, estimated EGP varied considerably among patients. In some of these studies EGP was likely overestimated due to the contribution of dietary glucose or intravenous/intragastric glucose infusions, which caused total glucose Ra to exceed EGP. The reason why there is still residual EGP in GSD I is still not clear. Various mechanisms have been proposed, including residual G6Pase activity (90), extra-hepatic/renal/intestinal EGP (91), increased debrancher enzyme (AGL) activity, and increased acid alpha glucosidase (GAA) activity (82). Studies on rodent models have contributed to further delineate the (patho)physiological basis of this process. Stable isotope tracer methodology to investigate glucose kinetics in animal models for GSD I was first applied in an acute model of GSD Ib in fasted rats using the pharmacological G6PT-inhibitor S4048. The method applied consisted of an infusion of stable isotopes D-[U-13C]-glucose, [2-13C]-glycerol, and D-[1-2H]-galactose, as well as paracetamol, to quantify hepatic glucose and glycogen metabolism (80). Later, this same methodology was applied to mice with hepatocyte-specific G6pc deficiency, a model for hepatic GSD Ia (81), which retained about 30% of EGP compared to their wild-type littermates. Primary hepatocytes from these animals showed that residual glucose production (~20% of wild-type littermates) was almost completely abolished upon treatment with an α-glucosidase inhibitor, which inhibits both AGL and GAA. This indicates that either increased glycogen debranching and/or lysosomal glycogen breakdown accounts for the production of free glucose by the GSD Ia liver and hence could explain the residual EGP in GSD I (81). Nonetheless, research assessing the utilization of (various types of) cornstarch in hepatic GSD patients (92, 93) supports the extensibility of stable isotopes methods also to human research.
Although the past years have witnessed major advances in understanding the pathophysiology of disorders associated with hypoglycemia, relevant limitations in assessment and monitoring strategies remain. The current diagnostic approach to children presenting with hypoglycemia includes, besides medical/dietary history and clinical examination, biochemical tests (performed on “critical samples”) and eventually genetic confirmation (21). However, critical samples or reliable biochemical data are not always available or may not provide conclusive information. Also, traditional biochemical markers are static parameters and do not always appear sufficient to explain the phenotypic variability in patients with IMDs associated with hypoglycemia (94). Furthermore, those biomarkers may not adequately reflect the dynamic pathophysiological processes taking place in response to external factors such as metabolic stressors or treatment. Thus, there remain a group of patients with unsolved diagnostic and/or monitoring questions, that may be addressed by dynamic methods, such as in vivo functional tests, CGM and stable isotope tracing.
Functional in vivo tests have the advantage of characterizing metabolic pathways, such as glycogenolysis, gluconeogenesis, mitochondrial fatty acid oxidation, ketogenesis, and ketolysis, in a dynamic fashion. Yet, their use is progressively decreasing. Therefore, we do not recommend performing such tests in unexperienced centers. Several functional in vivo tests (e.g., controlled fasting challenge) are currently designed to monitor management after novel, innovative treatments (NCT03517085, NCT03665636, NCT05095727, NCT NCT04538989, NCT04720859, NCT03761693) in centers of expertise. Fasting test may still play a role in the diagnostic process of congenital hyperinsulinism. Yet, the development of safer and simpler diagnostic and monitoring tools for clinical research/care remains a compelling need (95).
CGM constitutes a highly informative technique to (non-invasively) monitor glucose levels in a dynamic fashion in patients with hypoglycemia (39–41, 45). Although physicians working in centers of expertise have become experienced with the CGM technology, its use in less experienced centers remains limited. Therefore, knowledge dissemination on the CGM technology and data interpretation is paramount. In fact, given its relatively low organizational burden and invasiveness, CGM may constitute a valuable technique. Ideally, individual patients’ CGM results could be compared with (1) the patient’s historical CGM data before and after intervention, and CGM data from both (2) an age-matched disease cohort and (3) age-matched healthy controls. Shah et al. recently reported CGM profiles from 153 nonpregnant, healthy, nondiabetic children and adults (age ≥6 years) with nonobese body mass index, using a blinded Dexcom G6 CGM, with once-daily calibration, for up to 10 days (96). Advanced CGM data analysis represents a promising strategy for minimally invasive patient monitoring (48). However, several challenges constrain CGM use in patients with hypoglycemia. First, neither general agreement on relevant CGM-related outcome parameters nor reference values for such parameters exist for patients with IMDs. Ideally, individual patients’ CGM results would be comparable once these issues have been solved. Second, the lag time (i.e., the time delay between a change in plasma glucose and the reporting of this change by the CGM device) may affect CGM reliability in patients experiencing sudden drops in glucose concentrations, as in hepatic GSDs. Third, most CGM devices do not contain predictive alarms (i.e., predicting an upcoming low glucose event using trend information) and rely upon the user to identify hypoglycemia and make manual adjustments. Also, current predictive alarms are based on a linear function of glucose trends, which may not adequately reflect the exponential decrease observed in hypoglycemic GSD patients (97). Likely the progress in machine learning and deep learning approaches and artificial neural networks will develop reliable algorithms for hypoglycemia prediction in patients with IMDs (35). Fourth, some devices require fingerprick calibration, which currently prioritizes hyperglycemia over hypoglycemia, thus constituting a potential additional source of inaccuracy, particularly a in the low range of blood glucose (35). Notably, most recent devices such as Dexcom G6 and Freestyle Libre are factory-calibrated (98). Fifth, as no specific CGM device is formally licensed for patients with IMDs, several types of CGM devices are used (Table 3) presenting with different functionalities, advantages, and limitations. Additionally, data management applications currently vary, depending on the type of CGM device installed and interconnectivity between CGM software packages, and digital health records are lacking. Harmonization of data management applications as well as improved interconnectivity between CGM software packages and data health records are strongly warranted to facilitate data sharing among healthcare professionals and beyond towards citizen science applications. Sixth, while a 14-day data collection is recommended in DM patients (99), the number of measurements required to generate reliable CGM profiles in patients with IMDs is still unknown.
Stable isotope techniques appear attractive as they give access to the in vivo dynamics of hypoglycemia from a pathophysiological perspective. In principle, this may prevent a late diagnosis in patients presenting with milder phenotypes (100, 101). Yet, this technique has been explored experimentally in a limited number of conditions (e.g., idiopathic ketotic hypoglycemia, GSD I, congenital hyperinsulinism), but it is currently not clinically implemented, neither applied in clinical trials. Potential clinical benefit of this approach includes the definition of an easy and safe method to guide the diagnosis of patients with hypoglycemia and to improve patient monitoring, possibly constituting an additional tool to assess the effect of novel treatments (e.g., gene therapy, mRNA therapy). Given the safety of this methodology, it appears well suited for clinical trials in humans. Traditionally, venous blood samples are collected before the intravenous tracer infusion (after a priming dose) and several times after achieving the steady state (60). Such a procedure may potentially hamper the translation of this approach into clinical research/care in its current form. Previous research has shown the reliability of dried blood spot (DBS) sampling (compared to traditional venous sampling) in mice by tail bleeding to assess glucose kinetics following infusion of D-[U-13C]-glucose, D-[1-2H]-galactose, and [2-13C]-glycerol (102) or after an intraperitoneal injection of D-[6,6-2H]-glucose (58). Although these findings pave the way for possible oral (non-steady-state) administration of stable isotope tracers to humans (who are metabolically in a steady state, i.e. upon fasting without any unknown (unmeasured) and/or non-steady additional exogenous glucose input), the effect of this administration route on the tracer bioavailability remains unknown. Future studies exploring this approach in humans are warranted. Additionally, the use of stable isotope tracers in animal models can guide further dissection of pathophysiological derangements occurring in IMDs.
In addition to functional in vivo tests, metabolic profiling by CGM and stable isotope techniques, the microdialysis technique, at least in theory, would enable continuous sampling of multiple metabolites from the extracellular space from various tissues, such as blood, adipose tissue, or brain in experimental models (103, 104). The combination of stable isotope tracing and microdialysis in animal models appears intriguing (105). As microdialysis allows not only glucose monitoring (106, 107) but also sampling of other metabolites (e.g., glycerol, acylcarnitines) (108), it has a great potential for experimental research in patients with IMDs. Still, there are currently no commercial systems available.
AR, MGSR, MHO and TGJD wrote the first version of the manuscript. THvD, BMB, D-JR and TGJD critically reviewed the manuscript. AR drafted and wrote the final version of manuscript. TGJD critically reviewed the final version of the manuscript. All authors substantially contributed to the work and were involved in (a) conception and design of the study and/or analysis and interpretation of data, and (b) revising the article critically for important intellectual content. All authors approved the final manuscript as submitted and agree to be accountable for all aspects of the work. All authors confirm the absence of previous similar or simultaneous publications. All authors contributed to the article and approved the submitted version.
The authors declare that the research was conducted in the absence of any commercial or financial relationships that could be construed as a potential conflict of interest.
The handling editor declared a past co-collaboration with the authors.
All claims expressed in this article are solely those of the authors and do not necessarily represent those of their affiliated organizations, or those of the publisher, the editors and the reviewers. Any product that may be evaluated in this article, or claim that may be made by its manufacturer, is not guaranteed or endorsed by the publisher.
1. Gandhi K. Approach to Hypoglycemia in Infants and Children. Transl Pediatr (2017) 6(4):408–20. doi: 10.21037/tp.2017.10.05
2. Cornblath M, Hawdon JM, Williams AF, Aynsley-Green A, Ward-Platt MP, Schwartz R, et al. Controversies Regarding Definition of Neonatal Hypoglycemia: Suggested Operational Thresholds. Pediatrics (2000) 105(5):1141–5. doi: 10.1542/peds.105.5.1141
3. Thornton PS, Stanley CA, De Leon DD, Harris D, Haymond MW, Hussain K, et al. Recommendations From the Pediatric Endocrine Society for Evaluation and Management of Persistent Hypoglycemia in Neonates, Infants, and Children. J Pediatr (2015) 167(2):238–45. doi: 10.1016/j.jpeds.2015.03.057
4. Kallem VR, Pandita A, Gupta G. Hypoglycemia: When to Treat? Clin Med Insights Pediatr (2017) 11:1179556517748913. doi: 10.1177/1179556517748913
5. Suh SW, Hamby AM, Swanson RA. Hypoglycemia, Brain Energetics, and Hypoglycemic Neuronal Death. Glia (2007) 55(12):1280–6. doi: 10.1002/glia.20440
6. Suh SW, Aoyama K, Chen Y, Garnier P, Matsumori Y, Gum E, et al. Hypoglycemic Neuronal Death and Cognitive Impairment are Prevented by Poly(ADP-Ribose) Polymerase Inhibitors Administered After Hypoglycemia. J Neurosci (2003) 23(33):10681–90. doi: 10.1523/JNEUROSCI.23-33-10681.2003
7. McKinlay CJ, Alsweiler JM, Ansell JM, Anstice NS, Chase JG, Gamble GD, et al. Neonatal Glycemia and Neurodevelopmental Outcomes at 2 Years. N Engl J Med (2015) 373(16):1507–18. doi: 10.1056/NEJMoa1504909
8. Tas E, Garibaldi L, Muzumdar R. Glucose Homeostasis in Newborns: An Endocrinology Perspective. Neoreviews (2020) 21(1):e14–29. doi: 10.1542/neo.21-1-e14
9. Saudubray JM, van den Berghe V, Walter J. (2016). Inherited Metabolic Diseases. 6th Edition. (Berlin, Germany: Springer).
10. Ghosh A, Banerjee I, Morris AAM. Recognition, Assessment and Management of Hypoglycaemia in Childhood. Arch Dis Child (2016) 101(6):575–80. doi: 10.1136/archdischild-2015-308337
11. Chaussain JL, Georges P, Calzada L, Job JC. Glycemic Response to 24-Hour Fast in Normal Children: III. Influence of Age. J Pediatr (1977) 91(5):711–4. doi: 10.1016/s0022-3476(77)81020-2
12. Haymond MW, Karl IE, Clarke WL, Pagliara AS, Santiago JV. Differences in Circulating Gluconeogenic Substrates During Short-Term Fasting in Men, Women, and Children. Metabolism (1982) 31(1):33–42. doi: 10.1016/0026-0495(82)90024-5
13. Lamers KJ, Doesburg WH, Gabreëls FJ, Romsom AC, Renier WO, Wevers RA, et al. Reference Values of Blood Components Related to Fuel Metabolism in Children After an Overnight Fast. Clin Chim Acta (1985) 145(1):17–26. doi: 10.1016/0009-8981(85)90015-4
14. Lamers KJ, Doesburg WH, Gabreëls FJ, Lemmens WA, Romsom AC, Wevers RA, et al. The Concentration of Blood Components Related to Fuel Metabolism During Prolonged Fasting in Children. Clin Chim Acta (1985) 152(1-2):155–63. doi: 10.1016/0009-8981(85)90186-X
15. Bonnefont JP, Specola NB, Vassault A, Lombes A, Ogier H, de Klerk JB, et al. The Fasting Test in Paediatrics: Application to the Diagnosis of Pathological Hypo- and Hyperketotic States. Eur J Pediatr (1990) 150(2):80–5. doi: 10.1007/BF02072043
16. van Veen MR, van Hasselt PM, de Sain-van der Velden MG, Verhoeven N, Hofstede FC, de Koning TJ, et al. Metabolic Profiles in Children During Fasting. Pediatrics (2011) 127(4):e1021–7. doi: 10.1542/peds.2010-1706
17. Sunehag A, Ewald U, Larsson A, Gustafsson J. Glucose Production Rate in Extremely Immature Neonates (< 28 Weeks) Studied by Use of Deuterated Glucose. Pediatr Res (1993) 33(2):97–100. doi: 10.1203/00006450-199302000-00001
18. Bier DM, Leake RD, Haymond MW, Arnold KJ, Gruenke LD, Sperling MA, et al. Measurement of “True” Glucose Production Rates in Infancy and Childhood With 6,6-Dideuteroglucose. Diabetes (1977) 26(11):1016–23. doi: 10.2337/diab.26.11.1016
19. Huidekoper HH, Ackermans MT, Ruiter A, Sauerwein HP, Wijburg FA. Endogenous Glucose Production From Infancy to Adulthood: A non-Linear Regression Model. Arch Dis Child (2014) 99(12):1098–102. doi: 10.1136/archdischild-2013-305718
20. Bougnères PF, Artavia-Loria E, Henry S, Basdevant A, Castaño L. Increased Basal Glucose Production and Utilization in Children With Recent Obesity Versus Adults With Long-Term Obesity. Diabetes (1989) 38(4):477–83. doi: 10.2337/diab.38.4.477
21. Casertano A, Rossi A, Fecarotta S, Rosanio FM, Moracas C, Di Candia F, et al. An Overview of Hypoglycemia in Children Including a Comprehensive Practical Diagnostic Flowchart for Clinical Use. Front Endocrinol (Lausanne) (2021) 12:684011. doi: 10.3389/fendo.2021.684011
22. Haymond MW, Pagliara AS. Ketotic Hypoglycaemia. Clin Endocrinol Metab (1983) 12(2):447–62. doi: 10.1016/S0300-595X(83)80051-6
23. Drachmann D, Hoffmann E, Carrigg A, Davis-Yates B, Weaver V, Thornton P, et al. Towards Enhanced Understanding of Idiopathic Ketotic Hypoglycemia: A Literature Review and Introduction of the Patient Organization, Ketotic Hypoglycemia International. Orphan J Rare Dis (2021) 16(1):173. doi: 10.1186/s13023-021-01797-2
24. Fernandes J, Huijing F, van de Kamer JH. A Screening Method for Liver Glycogen Diseases. Arch Dis Child (1969) 44(235):311–7. doi: 10.1136/adc.44.235.311
25. Fernandes J, Van De Kamer JH. Studies on the Utilization of Hexoses in Liver Glycogen Disease. Pediatrics (1965) 35:470–7. doi: 10.1542/peds.35.3.470
26. Fernandes J, van de Kamer JH. Hexose and Protein Tolerance Tests in Children With Liver Glycogenosis Caused by a Deficiency of the Debranching Enzyme System. Pediatrics (1968) 41(5):935–44. doi: 10.1542/peds.41.5.935
27. Blau N, Dionisi-Vici C, Ferreira CR, Vianey-Saban C, van Karnebeek CDM eds. Physician’s Guide to the Diagnosis, Treatment, and Follow-Up of Inherited Metabolic Diseases. Berlin Heidelberg: Springer-Verlag (2022).
29. Morris AA, Thekekara A, Wilks Z, Clayton PT, Leonard JV, Aynsley-Green A. Evaluation of Fasts for Investigating Hypoglycaemia or Suspected Metabolic Disease. Arch Dis Child (1996) 75(2):115–9. doi: 10.1136/adc.75.2.115
30. Bappal B, Mula-Abed WA. Evaluation of Diagnostic Fasting in the Investigation of Hypoglycemia in Children Omani Experience. Oman Med J (2007) 22(3):36–41.
31. Graves LE, Stewart K, Ambler GR, Bhattacharya K, Srinivasan S. Investigating Paediatric Hypoglycaemia: Dynamic Studies at a Tertiary Paediatric Hospital. J Paediatr Child Health (2021) 57(6):888–93. doi: 10.1111/jpc.15349
32. Demirbilek H, Hussain K. Congenital Hyperinsulinism: Diagnosis and Treatment Update. J Clin Res Pediatr Endocrinol (2017) 9(Suppl 2):69–87. doi: 10.4274/jcrpe.2017.S007
33. Rosenfeld E, Ganguly A, De Leon DD. Congenital Hyperinsulinism Disorders: Genetic and Clinical Characteristics. Am J Med Genet C Semin Med Genet (2019) 181(4):682–92. doi: 10.1002/ajmg.c.31737
34. Karam JH, Lorenzi M, Young CW, Burns AD, Prosser PR, Grodsky GM, et al. Feedback-Controlled Dextrose Infusion During Surgical Management of Insulinomas. Am J Med (1979) 66(4):675–80. doi: 10.1016/0002-9343(79)91183-5
35. Worth C, Dunne M, Ghosh A, Harper S, Banerjee I. Continuous Glucose Monitoring for Hypoglycaemia in Children: Perspectives in 2020. Pediatr Diab (2020) 21(5):697–706. doi: 10.1111/pedi.13029
36. American Diabetes Association. Standards of Medical Care in Diabetes. Diabetes Care (2020) 43:S1–2. doi: 10.2337/dc20-S002
37. Takahashi H, Iwahashi N, Kirigaya J, Kataoka S, Minamimoto Y, Gohbara M, et al. Glycemic Variability Determined With a Continuous Glucose Monitoring System can Predict Prognosis After Acute Coronary Syndrome. Cardiovasc Diabetol (2018) 17(1):116. doi: 10.1186/s12933-018-0761-5
38. Jamshed H, Beyl RA, Della Manna DL, Yang ES, Ravussin E, Peterson CM. Early Time-Restricted Feeding Improves 24-Hour Glucose Levels and Affects Markers of the Circadian Clock, Aging, and Autophagy in Humans. Nutrients (2019) 11(6):1234. doi: 10.3390/nu11061234
39. Cambiaso P, Schiaffini R, Pontrelli G, Carducci C, Ubertini G, Crea F, et al. Nocturnal Hypoglycaemia in ACTH and GH Deficient Children: Role of Continuous Glucose Monitoring. Clin Endocrinol (Oxf) (2013) 79(2):232–7. doi: 10.1111/cen.12123
40. Alsaffar H, Turner L, Yung Z, Didi M, Senniappan S. Continuous Flash Glucose Monitoring in Children With Congenital Hyperinsulinism; First Report on Accuracy and Patient Experience. Int J Pediatr Endocrinol (2018) 2018:3. doi: 10.1186/s13633-018-0057-2
41. Morales-Alvarez MC, Ricardo-Silgado ML, Lemus HN, González-Devia D, Mendivil CO. Fructosuria and Recurrent Hypoglycemia in a Patient With a Novel c.1693T>A Variant in the 3’ Untranslated Region of the Aldolase B Gene. SAGE Open Med Case Rep (2019) 7:2050313X18823098. doi: 10.1177/2050313X18823098
42. Hershkovitz E, Rachmel A, Ben-Zaken H, Phillip M. Continuous Glucose Monitoring in Children With Glycogen Storage Disease Type I. J Inherit Metab Dis (2001) 24(8):863–9. doi: 10.1023/A:1013996325720
43. Maran A, Crepaldi C, Avogaro A, Catuogno S, Burlina A, Poscia A, et al. Continuous Glucose Monitoring in Conditions Other Than Diabetes. Diabetes Metab Res Rev (2004) 20:S50–5. doi: 10.1002/dmrr.518
44. White F, Jones SA. The Use of Continuous Glucose Monitoring in the Practical Management of Glycogen Storage Disorders. J Inherit Metab Dis (2011) 34:631–642.25. doi: 10.1007/s10545-011-9335-3
45. Kasapkara ÇS, Cinasal Demir G, Hasanoglu A, Tümer L. Continuous Glucose Monitoring in Children With Glycogen Storage Disease Type I. Eur J Clin Nutr (2014) 68:101–5. doi: 10.1038/ejcn.2013.186
46. Herbert M, Pendyal S, Rairikar MR, Halaby C, Benjamin RW, Kishnani PS. Role of Continuous Glucose Monitoring in the Management of Glycogen Storage Dis-Orders. J Inherit Metab Dis (2018) 41:917–27. doi: 10.1007/s10545-018-0200-5
47. Kaiser N, Gautschi M, Bosanska L, Meienberg F, Baumgartner MR, Spinas GA, et al. Glycemic Control and Complications in Glycogen Storage Disease Type I: Results From the Swiss Registry. Mol Gen Metab (2019) 126:355–61. doi: 10.1016/j.ymgme.2019.02.008
48. Peeks F, Hoogeveen IJ, Feldbrugge RL, Burghard R, de Boer F, Fokkert-Wilts MJ, et al. A Retrospective in-Depth Analysis of Continuous Glucose Monitoring Datasets for Patients With Hepatic Glycogen Storage Disease: Recommended Outcome Parameters for Glucose Management. J Inherit Metab Dis (2021) 44(5):1136–50. doi: 10.1002/jimd.12383
49. Rossi A, Miele E, Fecarotta S, Veiga-da-Cunha M, Martinelli M, Mollica C, et al. Crohn Disease-Like Enterocolitis Remission After Empagliflozin Treatment in a Child With Glycogen Storage Disease Type Ib: A Case Report. Ital J Pediatr (2021) 47(1):149. doi: 10.1186/s13052-021-01100-w
50. Wolfsdorf JI, Sadeghi-Nejad A, Senior B. Fat-Derived Fuels During a 24-Hour Fast in Children. Eur J Pediatr (1982) 138(2):141–4. doi: 10.1007/BF00441141
51. Parmar K, Mosha M, Weinstein DA, Riba-Wolman R. Method Comparison of Beta-Hydroxybutyrate Point-of-Care Testing to Serum in Healthy Children. JIMD Rep (2021) 62(1):85–90. doi: 10.1002/jmd2.12245
52. Weinstein DA, Steuerwald U, De Souza CFM, Derks TGJ. Inborn Errors of Metabolism With Hypoglycemia: Glycogen Storage Diseases and Inherited Disorders of Gluconeogenesis. Pediatr Clin North Am (2018) 65(2):247–65. doi: 10.1016/j.pcl.2017.11.005
53. Klein S, Heinzle E. Isotope Labeling Experiments in Metabolomics and Fluxomics. Wil Interdiscip Rev Syst Biol Med (2012) 4(3):261–72. doi: 10.1002/wsbm.1167
54. Schoenheimer R, Rittenberg D. The APPLICATION of ISOTOPES to THE Study OF Intermediary Metabolism. Science (1938) 87(2254):221–6. doi: 10.1126/science.87.2254.221
55. Wilkinson DJ. Historical and Contemporary Stable Isotope Tracer Approaches to Studying Mammalian Protein Metabolism. Mass Spectrom Rev (2018) 37(1):57–80. doi: 10.1002/mas.21507
56. Yuan M, Kremer DM, Huang H, Breitkopf SB, Ben-Sahra I, Manning BD, et al. Ex Vivo and In Vivo Stable Isotope Labelling of Central Carbon Metabolism and Related Pathways With Analysis by LC-MS/MS. Nat Protoc (2019) 14(2):313–30. doi: 10.1038/s41596-018-0102-x
57. Huidekoper HH, Visser G, Ackermans MT, Sauerwein HP, Wijburg FA. A Potential Role for Muscle in Glucose Homeostasis: In Vivo Kinetic Studies in Glycogen Storage Disease Type 1a and fructose-1,6-bisphosphatase Deficiency. J Inherit Metab Dis (2010) 33(1):25–31. doi: 10.1007/s10545-009-9030-9
58. van Dijk TH, Laskewitz AJ, Grefhorst A, Boer TS, Bloks VW, Kuipers F, et al. A Novel Approach to Monitor Glucose Metabolism Using Stable Isotopically Labelled Glucose in Longitudinal Studies in Mice. Lab Anim (2013) 47(2):79–88. doi: 10.1177/0023677212473714
59. Cobelli C, Foster DM, Toffolo GM. Tracer Kinetics in Biomedical Research. New York: Kluwer Academic/Plenum Publishers (2000).
60. Kim IY, Suh SH, Lee IK, Wolfe RR. Applications of Stable, Nonradioactive Isotope Tracers in In Vivo Human Metabolic Research. Exp Mol Med (2016) 48(1):e203. doi: 10.1038/emm.2015.97
61. Wolfe RR. Radioactive and Stable Isotope Tracers in Biomedicine. 1st ed. New York, NY, USA: Wiley-Liss, Inc (1992).
62. Umpleby M, Fielding BA. Stable Isotopes in Nutrition Research. Nutr Res Methodol (2015) 2015:250–64. doi: 10.1002/9781119180425.ch17
63. Wolfe RR, Chinkes DL. Isotopic Tracers in Metabolic Research. In: Principles and Practice of Kinetic Analysis. Hoboken, NJ: Wiley-Liss (2005).
64. Wajngot A, Chandramouli V, Schumann WC, Kumaran K, Efendić S, Landau BR. Testing of the Assumptions Made in Estimating the Extent of Futile Cycling. Am J Physiol (1989) 256(5 Pt 1):E668–75. doi: 10.1152/ajpendo.1989.256.5.E668
65. Davies PSW. Stable Isotopes: Their Use and Safety in Human Nutrition Studies. Eur J Clin Nutr (2020) 74(3):362–5. doi: 10.1038/s41430-020-0580-0
66. Vella A, Rizza RA. Application of Isotopic Techniques Using Constant Specific Activity or Enrichment to the Study of Carbohydrate Metabolism. Diabetes (2009) 58(10):2168–74. doi: 10.2337/db09-0318
67. Finegood DT, Bergman RN, Vranic M. Estimation of Endogenous Glucose Production During Hyperinsulinemic-Euglycemic Glucose Clamps. Comparison of Unlabeled and Labeled Exogenous Glucose Infusates. Diabetes (1987) 36(8):914–24. doi: 10.2337/diab.36.8.914
68. Sharabi K, Tavares CD, Rines AK, Puigserver P. Molecular Pathophysiology of Hepatic Glucose Production. Mol Aspects Med (2015) 46:21–33. doi: 10.1016/j.mam.2015.09.003
69. Schwartz NS, Clutter WE, Shah SD, Cryer PE. Glycemic Thresholds for Activation of Glucose Counterregulatory Systems are Higher Than the Threshold for Symptoms. J Clin Invest (1987) 79(3):777–81. doi: 10.1172/JCI112884
70. Hawdon JM, Aynsley-Green A, Bartlett K, Ward Platt MP. The Role of Pancreatic Insulin Secretion in Neonatal Glucoregulation. II. Infants With Disordered Blood Glucose Homoeostasis. Arch Dis Child (1993) 68(3):280–5. doi: 10.1136/adc.68.3_spec_no.280
71. Huidekoper HH, Duran M, Turkenburg M, Ackermans MT, Sauerwein HP, Wijburg FA. Fasting Adaptation in Idiopathic Ketotic Hypoglycemia: A Mismatch Between Glucose Production and Demand. Eur J Pediatr (2008) 167(8):859–65. doi: 10.1007/s00431-007-0598-5
72. Bodamer OA, Hussein K, Morris AA, Langhans CD, Rating D, Mayatepek E, et al. Glucose and Leucine Kinetics in Idiopathic Ketotic Hypoglycaemia. Arch Dis Child (2006) 91(6):483–6. doi: 10.1136/adc.2005.089425
73. Cederblad F, Ewald U, Gustafsson J. Effect of Glucagon on Glucose Production, Lipolysis, and Gluconeogenesis in Familial Hyperinsulinism. Horm Res (1998) 50(2):94–8. doi: 10.1159/000023242
74. Chung ST, Chacko SK, Sunehag AL, Haymond MW. Measurements of Gluconeogenesis and Glycogenolysis: A Methodological Review. Diabetes (2015) 64(12):3996–4010. doi: 10.2337/db15-0640
75. Reijngoud DJ. Flux Analysis of Inborn Errors of Metabolism. J Inherit Metab Dis (2018) 41(3):309–28. doi: 10.1007/s10545-017-0124-5
76. Derks TG, van Dijk TH, Grefhorst A, Rake JP, Smit GP, Kuipers F, et al. Inhibition of Mitochondrial Fatty Acid Oxidation In Vivo Only Slightly Suppresses Gluconeogenesis But Enhances Clearance of Glucose in Mice. Hepatology (2008) 47(3):1032–42. doi: 10.1002/hep.22101
77. Houten SM, Herrema H, Te Brinke H, Denis S, Ruiter JP, van Dijk TH, et al. Impaired Amino Acid Metabolism Contributes to Fasting-Induced Hypoglycemia in Fatty Acid Oxidation Defects. Hum Mol Genet (2013) 22(25):5249–61. doi: 10.1093/hmg/ddt382
78. Storgaard JH, Madsen KL, Løkken N, Vissing J, van Hall G, Lund AM, et al. Impaired Lipolysis in Propionic Acidemia: A New Metabolic Myopathy? JIMD Rep (2020) 53(1):16–21. doi: 10.1002/jmd2.12113
79. Gopher A, Vaisman N, Mandel H, Lapidot A. Determination of Fructose Metabolic Pathways in Normal and Fructose-Intolerant Children: A 13C NMR Study Using [U-13C]Fructose. Proc Natl Acad Sci USA (1990) 87(14):5449–53. doi: 10.1073/pnas.87.14.5449
80. van Dijk TH, van der Sluijs FH, Wiegman CH, Baller JF, Gustafson LA, Burger HJ, et al. Acute Inhibition of Hepatic glucose-6-phosphatase Does Not Affect Gluconeogenesis But Directs Gluconeogenic Flux Toward Glycogen in Fasted Rats. A Pharmacological Study With the Chlorogenic Acid Derivative S4048. J Biol Chem (2001) 276(28):25727–35. doi: 10.1074/jbc.M101223200
81. Hijmans BS, Boss A, van Dijk TH, Soty M, Wolters H, Mutel E, et al. Hepatocytes Contribute to Residual Glucose Production in a Mouse Model for Glycogen Storage Disease Type Ia. Hepatology (2017) 66(6):2042–54. doi: 10.1002/hep.29389
82. Tsalikian E, Simmons P, Gerich JE, Howard C, Haymond MW. Glucose Production and Utilization in Children With Glycogen Storage Disease Type I. Am J Physiol (1984) 247(4 Pt 1):513. doi: 10.1152/ajpendo.1984.247.4.E513
83. Schwenk WF, Haymond MW. Optimal Rate of Enteral Glucose Administration in Children With Glycogen Storage Disease Type I. N Engl J Med (1986) 314(11):682–5. doi: 10.1056/NEJM198603133141104
84. Kalderon B, Korman SH, Gutman A, Lapidot A. Glucose Recycling and Production in Glycogenosis Type I and III: Stable Isotope Technique Study. Am J Physiol Endocrinol Metab (1989) 257(3):E346–53. doi: 10.1152/ajpendo.1989.257.3.E346
85. Kalderon B, Korman SH, Gutman A, Lapidot A. Estimation of Glucose Carbon Recycling in Children With Glycogen Storage Disease: A13c NMR Study Using [U-13C]Glucose. Proc Natl Acad Sci USA (1989) 86:4690–4. doi: 10.1073/pnas.86.12.4690
86. Collins JE, Bartlett K, Leonard JV, Aynsley-Green A. Glucose Production Rates in Type 1 Glycogen Storage Disease. J Inherit Metab Dis (1990) 13(2):195–206. doi: 10.1007/BF01799686
87. Rother KI, Schwenk WF. Glucose Production in Glycogen Storage Disease I is Not Associated With Increased Cycling Through Hepatic Glycogen. Am J Physiol (1995) 269(4 Pt 1):774. doi: 10.1152/ajpendo.1995.269.4.E774
88. Weghuber D, Mandl M, Krssák M, Roden M, Nowotny P, Brehm A, et al. Characterization of Hepatic and Brain Metabolism in Young Adults With Glycogen Storage Disease Type 1: A Magnetic Resonance Spectroscopy Study. Am J Physiol Endocrinol Metab (2007) 293(5):E1378–84. doi: 10.1152/ajpendo.00658.2006
89. Powell RC, Wentworth SM, Brandt IK. Endogenous Glucose Production in Type I Glycogen Storage Disease. Metabolism (1981) 30(5):443–50. doi: 10.1016/0026-0495(81)90178-5
90. Spencer-Peet J, Norman ME, Lake BD, McNamara J, Patrick AD. Hepatic Glycogen Storage Disease. Clin Lab Findings 23 Cases Q J Med (1971) 40(157):95–114.
91. Shieh JJ, Pan CJ, Mansfield BC, Chou JY. A glucose-6-phosphate Hydrolase, Widely Expressed Outside the Liver, can Explain Age-Dependent Resolution of Hypoglycemia in Glycogen Storage Disease Type Ia. J Biol Chem (2003) 278(47):47098–103. doi: 10.1074/jbc.M309472200
92. Bodamer OA, Feillet F, Lane RE, Lee PJ, Dixon MA, Halliday D, et al. Utilization of Cornstarch in Glycogen Storage Disease Type Ia. Eur J Gastroenterol Hepatol (2002) 14(11):1251–6. doi: 10.1097/00042737-200211000-00014
93. Bhattacharya K, Orton RC, Qi X, Mundy H, Morley DW, Champion MP, et al. A Novel Starch for the Treatment of Glycogen Storage Diseases. J Inherit Metab Dis (2007) 30(3):350–7. doi: 10.1007/s10545-007-0479-0
94. Peeks F, Steunenberg TAH, de Boer F, Rubio-Gozalbo ME, Williams M, Burghard R, et al. Clinical and Biochemical Heterogeneity Between Patients With Glycogen Storage Disease Type IA: The Added Value of CUSUM for Metabolic Control. J Inherit Metab Dis (2017) 40(5):695–702. doi: 10.1007/s10545-017-0039-1
95. Derks TGJ, Rodriguez-Buritica DF, Ahmad A, de Boer F, Couce ML, Grünert SC, et al. Glycogen Storage Disease Type Ia: Current Management Options, Burden and Unmet Needs. Nutrients (2021) 13(11):3828. doi: 10.3390/nu13113828
96. Shah VN, DuBose SN, Li Z, Beck RW, Peters AL, Weinstock RS, et al. Continuous Glucose Monitoring Profiles in Healthy Nondiabetic Participants: A Multicenter Prospective Study. J Clin Endocrinol Metab (2019) 104(10):4356–64. doi: 10.1210/jc.2018-02763
97. Rodbard D. Continuous Glucose Monitoring: A Review of Successes, Challenges, and Opportunities. Diabetes Technol Ther (2016) 18(Suppl 2):S3–S13. doi: 10.1089/dia.2015.0417
98. Dexcom, Inc. Make Knowledge Your Superpower With Thedexcom G6®CGM System. San Diego, CA: Dexcom, Inc (2019). Available at: https://www.dexcom.com/g6-cgm-system.
99. Ajjan R, Slattery D, Wright E. Continuous Glucose Monitoring: A Brief Review for Primary Care Practitioners. Adv Ther (2019) 36(3):579–96. doi: 10.1007/s12325-019-0870-x
100. Cassiman D, Libbrecht L, Verslype C, Meersseman W, Troisi R, Zucman-Rossi J, et al. An Adult Male Patient With Multiple Adenomas and a Hepatocellular Carcinoma: Mild Glycogen Storage Disease Type Ia. J Hepatol (2010) 53(1):213–7. doi: 10.1016/j.jhep.2010.03.002
101. Moest W, van der Deure W, Koster T, Spee-Dropková M, Swart-Busscher L, de Haas RJ, et al. Glycogen Storage Disease Type Ia: Adult Presentation With Microcytic Anemia and Liver Adenomas. Hepatology (2018) 68(2):780–2. doi: 10.1002/hep.29858
102. van Dijk TH, Boer TS, Havinga R, Stellaard F, Kuipers F, Reijngoud DJ. Quantification of Hepatic Carbohydrate Metabolism in Conscious Mice Using Serial Blood and Urine Spots. Anal Biochem (2003) 322(1):1–13. doi: 10.1016/j.ab.2003.07.008
103. Arner P, Bolinder J. Microdialysis of Adipose Tissue. J Intern Med (1991) 230(4):381–6. doi: 10.1111/j.1365-2796.1991.tb00461.x
104. Young B, Kalanuria A, Kumar M, Burke K, Balu R, Amendolia O, et al. Cerebral Microdialysis. Crit Care Nurs Clin North Am (2016) 28(1):109–24. doi: 10.1016/j.cnc.2015.09.005
105. Hershey ND, Kennedy RT. In Vivo Calibration of Microdialysis Using Infusion of Stable-Isotope Labeled Neurotransmitters. ACS Chem Neurosci (2013) 4(5):729–36. doi: 10.1021/cn300199m
106. Baumeister FA, Rolinski B, Busch R, Emmrich P. Glucose Monitoring With Long-Term Subcutaneous Microdialysis in Neonates. Pediatrics (2001) 108(5):1187–92. doi: 10.1542/peds.108.5.1187
107. Hage C, Mellbin L, Rydén L, Wernerman J. Glucose Monitoring by Means of an Intravenous Microdialysis Catheter Technique. Diabetes Technol Ther (2010) 12(4):291–5. doi: 10.1089/dia.2009.0150
Keywords: hypoglycemia, children, hepatic glycogen storage diseases, functional tests, fasting challenge, continuous glucose monitoring, stable isotopes
Citation: Rossi A, Rutten MGS, van Dijk TH, Bakker BM, Reijngoud D-J, Oosterveer MH and Derks TGJ (2022) Dynamic Methods for Childhood Hypoglycemia Phenotyping: A Narrative Review. Front. Endocrinol. 13:858832. doi: 10.3389/fendo.2022.858832
Received: 20 January 2022; Accepted: 27 April 2022;
Published: 17 June 2022.
Edited by:
Indraneel (Indi) Banerjee, The University of Manchester, United KingdomReviewed by:
Tohru Yorifuji, Osaka City General Hospital, JapanCopyright © 2022 Rossi, Rutten, van Dijk, Bakker, Reijngoud, Oosterveer and Derks. This is an open-access article distributed under the terms of the Creative Commons Attribution License (CC BY). The use, distribution or reproduction in other forums is permitted, provided the original author(s) and the copyright owner(s) are credited and that the original publication in this journal is cited, in accordance with accepted academic practice. No use, distribution or reproduction is permitted which does not comply with these terms.
*Correspondence: Terry G. J. Derks, dC5nLmouZGVya3NAdW1jZy5ubA==
Disclaimer: All claims expressed in this article are solely those of the authors and do not necessarily represent those of their affiliated organizations, or those of the publisher, the editors and the reviewers. Any product that may be evaluated in this article or claim that may be made by its manufacturer is not guaranteed or endorsed by the publisher.
Research integrity at Frontiers
Learn more about the work of our research integrity team to safeguard the quality of each article we publish.