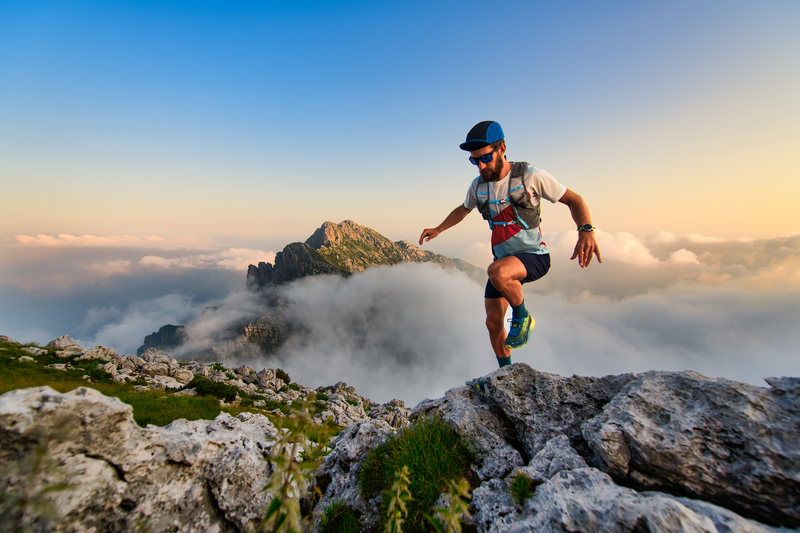
95% of researchers rate our articles as excellent or good
Learn more about the work of our research integrity team to safeguard the quality of each article we publish.
Find out more
ORIGINAL RESEARCH article
Front. Endocrinol. , 28 March 2022
Sec. Clinical Diabetes
Volume 13 - 2022 | https://doi.org/10.3389/fendo.2022.852395
This article is part of the Research Topic Cardiovascular Diseases Related to Diabetes and Obesity View all 13 articles
Objective: We aimed to investigate the impact of insulin resistance (IR), as determined by the homeostasis model assessment of insulin resistance (HOMA-IR), on cardiometabolic risk factors (CMRFs), and develop an anthropometry-based predictive nomogram for IR among adolescents in China.
Design: Data were acquired from a cross-sectional study with a stratified cluster sampling method, conducted among adolescents in Northeast China.
Participants: A total of 882 adolescents (aged 12–16 years, 468 boys) were included.
Measurements: All participants underwent anthropometric and biochemical examinations. The thresholds of IR included the 90th percentile of the HOMA-IR for adolescents with a normal body mass index (BMI) and fasting plasma glucose (FPG) level within each sex group (Cutoff A), and the 75th percentile for all participants of the same sex (Cutoff B).
Results: The HOMA-IR was associated with CMRFs. IR, as defined by both cutoffs A and B, was significantly associated with most CMRFs, except decreased HDL-C levels. Excellent concordance (κ = 0.825) was found between these two criteria in diagnosing IR. However, IR using cutoff A, was more closely associated with cardiometabolic risk. The incidence of IR, as defined by cutoff A, was 18.93% and increased from 10.99% to 43.87% based on the different BMI categories. Further, an anthropometry-based predictive model for IR, incorporating sex, age, waist-to-hip ratio, weight and BMI, was developed and presented as a nomogram.
Conclusions: IR among adolescents is strongly related to cardiometabolic risk. We developed an anthropometry-based predictive nomogram for IR among adolescents, which may facilitate health counselling and self-risk assessments.
Insulin resistance (IR) is often associated with the cluster of metabolically related cardiovascular risk factors [cardiometabolic risk factors (CMRFs)] that lead to morbidity and mortality worldwide (1–3). Owing to the global epidemic of obesity among children and adolescents, IR is also present in early life and contributes to metabolic disorders in childhood that tend to persist into adulthood (1). It is difficult to directly measure the incidence of IR due to the practical, ethical and economic issues involved with using the hyperinsulinemic-euglycemic clamp, which is considered the gold standard for measuring insulin sensitivity (4). The homeostasis model assessment of IR (HOMA-IR) has been proposed as a robust surrogate method for classifying IR in epidemiological studies (5, 6), and has been found to be more reliable than the fasting plasma glucose-to-insulin ratio and quantitative insulin sensitivity check index for quantifying the degree of IR in children with obesity (7). Additionally, puberty has also been associated with marked IR, and is known as “physiological IR” (1, 8). Among adults, the universally accepted HOMA-IR cutoff value for IR is 2.5 (5). However, among adolescents, a specific cutoff criterion for defining IR is still urgently needed to avoid under- or over-diagnosis. Here, using the data from a population-based study among adolescents in China, we aimed to classify IR in adolescents based on specific criteria, prevalence, and the extent of influences on CMRFs.
Owing to the use of anthropometric methods being convenient, noninvasive, and inexpensive, the importance of using these for the clinical diagnosis of obesity-related health risks is emphasized (9, 10). Overweight and obesity are frequently associated with IR (1, 2, 11). Weight, body mass index (BMI), waist circumference (WC), waist-to-hip ratio (WHR) and waist-to-height ratio (WHtR) are commonly used anthropometric indices for assessing global or local obesity in individuals. When identifying adolescents at risk of IR, anthropometric methods may be effective if the diagnostic values are appropriately specified in a statistical model. The nomogram is a simple and personalized visualization tool, that has been extensively used in the diagnosis of diseases. Here, we further aimed to develop an anthropometry-based predictive nomogram for early detection of IR among adolescents in China.
Data were acquired from a cross-sectional study that used stratified cluster sampling to include a total of 933 adolescents from the urban district of Liaoyang, a moderately sized city in northeast China. A total of 936 participants without known metabolic syndrome (MetS) were recruited, and 3 of which with HbA1c of ≥ 6.5% or FPG of ≥ 7.0 mmol/L were excluded because of the possibility of a diagnosis of diabetes. Ethical permission from the hospital’s Ethics Committee and written informed consent from the students and their parents were obtained prior to commencing the original study.
The following data were analyzed for the current study: age, sex, weight, height, WC, hip circumference (HC), systolic blood pressure (SBP), diastolic blood pressure (DBP), hemoglobin A1c (HbA1c), fasting plasma glucose (FPG), fasting plasma insulin (FINS), serum uric acid (SUA), total cholesterol (TC), triglycerides (TG), high-density lipoprotein cholesterol (HDL-C), low-density lipoprotein cholesterol (LDL-C), and a family history of type 2 diabetes mellitus (T2DM, defined as having a first- or second-degree relative with T2DM). A total of 882 participants (aged 12–16 years, 468 boys), without missing data on the variables described above, were included in the current analysis.
Physical examinations were conducted by trained physicians. Anthropometric indices were measured in a standing position while lightly clothed and without shoes. Weight was measured to the nearest 0.1 kg and height was measured to the nearest 0.1 cm. WC and HC were measured at the narrowest point between the iliac crest and the lowest rib during minimal respiration and at the largest girth, respectively. SBP and DBP were measured in the sitting position after a 10-minute rest, and calculated as the mean value of two repeated measurements. BMI was calculated as weight divided by height squared (kg/m2). WHR and WHtR were calculated as the quotient of the circumference of the waist to that of the hips and WC divided by height, respectively.
Blood samples were collected after an overnight fast (≥10 h). FPG, TG, TC, HDL-C and SUA were measured using an Olympus AU system (Olympus 400, Olympus Optical Company, Japan) in the laboratory at Liaoyang Diabetes Hospital. We further estimated LDL-C using Friedwald’s formula (12). HbA1c levels were measured using high performance liquid chromatography with a D-10 Hemoglobin Testing System (Bio-Rad Laboratories, Inc., Hercules, CA) in the central laboratory at Shengjing Hospital of China Medical University. HbA1c was standardized to the Diabetes Complications and Control Trial method. FINS was determined by radioimmunoassay (China Institute of Atomic Energy, Beijing, China). HOMA-IR was calculated as FINS (µU/mL) × FPG (mmol/L)/22.5 (5).
Obesity was defined as a BMI ≥95th percentile, and overweight was defined as a BMI ≥85th percentile and <95th percentile for children and adolescents of the same age and sex in China (13). Although IR is affected by pubertal status (8), Tanner stage data were not available for use in our study. Boys aged 12-18 years and girls aged 11-18 years are usually regarded as adolescents in China, because pubertal spurt is between ages 12 and 14 in boys and 11 and 13 in girls (14–18); thus, the participants (aged 12–16 years) in our study were all considered to be adolescents. Currently, there is no universally accepted method for defining the optimal threshold for IR among adolescents. Therefore, we adopted the 90th percentile of the HOMA-IR for adolescents with a normal BMI and FPG level within each sex group (Cutoff A) (7, 19), and the 75th percentile for all participants of the same sex (Cutoff B), as the thresholds of IR (20, 21). IR was identified when the HOMA-IR was above the following cutoff points: A: 5.64 (boy), 5.73 (girl) and B: 5.24 (boy), 5.20 (girl). According to the unified definition of pediatric metabolic syndrome (MetS) by the International Diabetes Federation (IDF) (22), MetS was identified in adolescents with abdominal obesity (defined as a WC ≥90th percentile in children and adolescents of the same age and sex in China) (23) and at least two of the following four criteria: TG levels of ≥1.70 mmol/L; HDL-C levels of <1.03 mmol/L for individuals aged 10–15 years and boys aged ≥16 years, or < 1.29 mmol/L for girls aged ≥16 years; an SBP of ≥130 mmHg and/or a DBP of ≥85 mmHg; and a FPG level of ≥5.6 mmol/L or T2DM.
Abdominal obesity, hypertension, increased FPG levels, dyslipidemia and hyperuricemia were assessed as CMRFs in this study. We used the definition of MetS (IDF) as a reference, which includes abdominal obesity, hypertension, increased FPG levels, increased TG levels and decreased HDL-C levels. Since neither the IDF nor the recommendations in China included threshold values for TC and LDL-C in children; we adopted the threshold values of a TC level of ≥5.2 mmol/L and a LDL-C level of ≥3.4 mmol/L as proposed by the American Academy of Pediatrics (24). Dyslipidemia was defined as the presence of at least one of the following: hypercholesterolemia, hypertriglyceridemia, hypo-HDL-C, and hyper-LDL-C. Due to the lack of universally accepted clinical diagnostic criteria for hyperuricemia in adolescents, we defined hyperuricemia as a SUA level of ≥357 µmol/L, which is consistent with similar previous studies (25). Clustering of CMRFs was defined as the presence of two or more CMRFs (26).
The Shapiro–Wilk and Levene tests were used to assess the normal distribution and homogeneity of each variable, respectively. Quantitative variables are expressed as the mean ± standard deviation or median (interquartile range) depending on the normality of their distributions, whereas qualitative variables are presented as counts and percentages. Quantitative data were analyzed using the Student’s t-test or Mann–Whitney U-test, and qualitative data were analyzed using the Chi-square test. Significance tests for differences in proportions were conducted using the two-tailed two-sample test approach. A partial correlation analysis was used to determine the relationship between HOMA-IR and CMRFs following adjustments for age and sex. We performed binary logistic regression analysis to determine the influence of IR on CMRFs. The kappa coefficient (κ) was calculated to determine the degree of agreement between the different definitions of IR. The prevalence of IR was calculated while considering BMI stratification. A least absolute shrinkage and selection operator (LASSO) regression analysis was used to select the most predictive anthropometric indices for IR (27, 28). Subsequently, a logistic regression analysis was performed to establish a prediction model by introducing the variables based on the LASSO regression, and the nomogram was constructed to achieve individual predictions. To validate the classification abilities of the nomogram, a bootstrapping calibration approach was used and randomly repeated 1000 times with replacement. Decision curve analysis and clinical impact curves were used to determine clinical usefulness. Data processing and statistical analyses were performed using R software (version 4.0.3; R project for Statistical Computing) and P-values <0.05 were considered statistically significant.
Among the 882 adolescents (468 boys), the median age was 14 (12–15) years, and 69 had MetS. The demographic data, physical examination results, and biochemical characteristics of the study participants according to gender are presented in Table 1. Boys had higher values for weight, BMI, WC, WHR, WHtR, SBP, FPG, SUA and HbA1c. Girls had higher values for TC, DBP and insulin levels, while there were no significant differences in values of HOMA-IR or other indices evaluated. Adolescent males were more likely to have abdominal obesity and hyperglycemia, contributing to a higher incidence of MetS.
The HOMA-IR was more significantly correlated with WC, FPG, TG, and SUA (r > 2, P <0.001), compared with other CMRFs evaluated (Figure 1). We analyzed the influence of IR, as defined by the different HOMA-IR thresholds (Cutoffs A and B), on CMRFs using a logistic regression analysis (Table 2). Whether adjusted for age and sex or not, IR as defined by both cutoffs A and B, was significantly associated with most CMRFs, except decreased HDL-C levels. More specifically, IR, as defined by cutoff B, was not correlated with hypercholesterolemia, and was associated with dyslipidemia only after adjustments for sex and age. Among participants with IR, the cardiometabolic risk increased by 3.132- and 2.662-fold according to cutoffs A and B, respectively. Excellent concordance (κ = 0.825, P <0.001) was found between these two criteria in diagnosing IR among adolescents in the Northeast of China (Table 3). Owing to the higher odds ratios for CMRFs, IR as defined by cutoff A was chosen for subsequent analyses.
Figure 1 Scatterplots of the homeostasis model assessment of insulin resistance against cardiometabolic risk factors (n = 882). Partial correlation coefficient of the HOMA-IR with WC, SBP, DBP, FPG, TC, TG, HDL, LDL, and SUA (A–I), adjusted for age and sex. Darker red denotes higher density. The black line is a smoothed condition mean, denoting the mean value of the cardiometabolic risk factor at a given HOMA-IR. DBP, diastolic blood pressure; FPG, fasting plasma glucose; HDL-C, high-density lipoprotein cholesterol; LDL-C, low-density lipoprotein cholesterol; SBP, systolic blood pressure; SUA, serum uric acid; TC, total cholesterol; TG, triglycerides; WC, waist circumference.
Table 2 The influence of insulin resistance on cardiometabolic risk factors according to different definitions of insulin resistance.
Table 3 Concordance between the different homeostasis model assessment of insulin resistance cutoff values used in diagnosing insulin resistance.
A total of 167 adolescents were classified as having IR based on cutoff A (90th percentile of the HOMA-IR for adolescents with a normal BMI and FPG level within each sex group), with a prevalence of 18.93%. The prevalence of IR varied among different BMI categories (Figure 2), and was highest in adolescents with obesity (43.87%) and lowest in adolescents with a BMI of <85th percentile (10.99%). There were no significant differences in the prevalence of IR between boys and girls either in total or in each subgroup alone.
Overweight and obesity are often associated with IR. Weight, BMI, WC, WHR and WHtR are common anthropometric indices used to assess global or local obesity in individuals. Based on the LASSO regression analysis, WHR, weight, and BMI had nonzero coefficients in addition to sex and age, with the greatest predictive power for IR (Figure 3A). A model incorporating sex, age, WHR, weight, and BMI was developed based on logistic regression analyses and presented as a nomogram (Figure 3B). The probability of IR was accurately predicted using the calibration curve (Figure 3C). The clinical impact and decision curves (Figures 3D, E) revealed that our model demonstrated a positive net benefit without increasing the number of false positives.
Figure 3 Development of an anthropometry-based nomogram for predicting insulin resistance among adolescents in China. (A) The LASSO coefficient profiles of eight variables. The coefficient profile plot was produced against the log(lambda) sequence. Five features with nonzero coefficients were selected by optimal lambda (0.006259), including sex, age, waist-to-hip ratio (WHR), weight and body mass index (BMI). (B) An anthropometry-based nomogram for the prediction of insulin resistance (IR) among adolescents in China, that includes sex, age, WHR, weight and BMI. (C) The calibration analysis. The x- and y-axes represents the nomogram-predicted probability of IR and the actual rate of IR, respectively. The solid line represents the performance of the nomogram. A more favorable performance is indicated by a closer fit to the ideal calibration line (the diagonal dotted line). (D) The clinical impact curve for evaluating clinical use. (E) The decision curve for evaluating clinical use. (F) Dynamic nomogram. An adolescent was randomly selected from the population, and the risk of IR was predicted using the five anthropometric indices of the nomogram.
A dynamic nomogram using a random participant was established to predict the risk of IR (Figure 3F). The participant’s characteristics were as follows: 12-year-old boy, WHR = 1.08, weight = 81.5 kg and BMI = 32.65 kg/m2. The total points were 213 and the probability of IR was 67.1%. Therefore, this tool provides an individualized estimate of the risk of IR and should be useful to patients and healthcare providers in the long-term management of adolescents with cardiometabolic risk. We also developed a dynamic nomogram using online software (https://doctordu.shinyapps.io/InsulinResistanceRiskNomogram/).
In China, the prevalence of overweight and obesity among children and adolescents has dramatically increased over the decades and in 2019, was 11.1% and 7.9%, respectively (29). With the earlier onset of obesity, it is also likely that the prevalence of IR has increased among children and adolescents, which may explain the concomitant earlier onset of pediatric metabolic disorders (2, 3, 30). Although IR has been extensively studied among adults (31, 32), there remains a lack of population-based studies on classifying IR in adolescents using specific criteria, prevalence, or the extent of influences on CMRFs.
Owing to the influence of pubertal development, it is imperative that the reference ranges used for diagnosis of IR in adolescents are appropriate for the relevant population. Currently, there are no universally agreed upon reference ranges that can be used to diagnose IR in adolescents. We summarized the main cut-off values of HOMA-IR for defining insulin resistance in children and adolescents in recent literatures (Table 4). Researchers in Turkey calculated the cut-off values for HOMA-IR by receiver operating characteristic (ROC) analysis, upon the IR criterion of a total blood insulin level exceeding 300 μU/mL during a standard oral glucose tolerance test (7, 38, 39). However, this method is not applicable for use in population-wide screening, owing to the relatively high cost and complexity. It is also inappropriate to apply their cutoff values directly in other races. Consequently, cutoff values for the diagnosis of IR have been defined based on the HOMA-IR distribution of a healthy reference population or the study population itself (19, 21, 33–37). The cutoff values of the HOMA-IR used for defining IR in adolescents varies widely according to ethnicity, age, gender, and screening methods, and ranges from 2.4 to 5.22 (19, 34, 36, 39). Due to the subjectivity and limitation in population studies of adding the Tanner criteria in identifying IR, there is a proposal to use cutoff values for HOMA-IR according to gender and pubertal staging (39). Here, we defined IR using the threshold of the 90th percentile of the HOMA-IR for adolescents with a normal BMI and FPG level within each sex group (5.64 for boys and 5.73 for girls), and the 75th percentile for adolescents of the same sex (5.24 for boys and 5.20 for girls), namely cutoffs A and B. A previous study evaluated the frequencies of dyslipidemia and hyperglycemia in children with IR assessed by cutoff points of HOMA-IR; the frequencies of increased TC, LDL-C and TG, decreased HDL-C were all above 50% (35). Another study demonstrated that in a sample of Korean children and adolescents, metabolic syndrome increased 18.4-fold and each MetS component (including central obesity, hypertriglyceridemia, hypertension, low HDL-C, and hyperglycemia) increased 2.12–7.42-fold among participants with IR (40). However, they used different cutoff values of HOMA-IR for the diagnosis of IR. Our study focused on more CMRFs and the clustering of CMRFs, and was not limited to only MetS components (36, 41). The partial correlation analysis revealed a significant association between the HOMA-IR and CMRFs, and HOMA-IR was more significantly correlated with WC, FPG, TG, and SUA compared with other CMRFs evaluated. The logistic regression, showed that IR was significantly associated with most CMRFs, except decreased HDL-C levels. Although, the direction of causality among IR and CMRFs could not be determined owing to the cross-sectional nature of this study, adolescents with IR have a 3.132- and 2.662-fold increased risk of clustering of CMRFs based on cutoffs A and B, respectively. Therefore, the early identification of IR is critical for early intervention and prevention of cardiometabolic disorders. The kappa coefficient (κ) suggested excellent agreement between cutoffs A and B for determining IR. However, based on cutoff B, IR was not correlated with hypercholesterolemia, and was associated with dyslipidemia only after adjustments for sex and age. Moreover, IR, as defined by cutoff A, was more closely associated with the clustering of CMRFs than IR as defined by cutoff B. Overall, the results suggested that cutoff A is the optimal indicator for clinical application. Using cutoff A, we further assessed the incidence of IR, which was 18.93% in this study, and increased from 10.99% to 43.87% based on the different BMI categories. This finding was similar to that of previous population-based studies (36, 37). Several studies have reported widely varying prevalence rates of IR among adolescents with obesity in different countries, such as the United States (52.1%) (33) and Brazil (29.1%) (42). Similar to other studies, there were no obvious differences based on sex in the frequency of IR (35, 40, 41).
Table 4 Main cut-off values of HOMA-IR for defining IR in children and adolescents in recent literatures.
Although several studies have been conducted to identify the anthropometric indices for IR among adolescents (42–44), few studies have integrated multiple anthropometric indices in a prediction model or scoring system to predict the individual risk of IR among adolescents. Nomograms, which allow for the seamless incorporation of risk prediction into clinical decision making, are widely used as personalized risk-prediction tools. According to the LASSO analysis, age, gender, BMI, weight, and WHR are important predictors of IR in adolescents. Our study quantified the above factors in a statistical prediction model, which we converted to a clinically usable nomogram that potentially illustrates the extent to which individual risk indicators contribute to the risk of IR. Its classifying power and clinical use were supported by the calibration, clinical impact and decision curves. As depicted in our example, the probability of a 12-year-old boy with a WHR of 1.08, weight of 81.5 kg, and BMI of 32.65 kg/m2, having IR is 67.1%. This was the first study to develop an anthropometry-based nomogram for predicting individualized probabilities of IR among adolescents in China.
This study has some limitations. The Tanner stage data, which may be necessary for accurately classifying adolescence, were not included. Owing to the limited sample size, we were unable to stratify the analyses by age and pubertal stage (early, mid, and late puberty). Further research focused on the association of IR with other CMRFs (such as nonalcoholic fatty liver disease) among adolescents are awaited to make the results more valuable for reference. Our study included a single-center cohort, which was not representative of the entire adolescent population in China. Further evaluation in large-scaled, multicenter-study populations is imperative to generalize these findings.
In conclusions, IR, as defined by the HOMA-IR, was significantly associated with an increased cardiometabolic risk. For defining IR among adolescents in China, the optimal thresholds, using the HOMA-IR, were above 5.64 for boys and 5.73 for girls. The incidence of IR increases with an increased BMI, and is highest among adolescents with obesity. Additionally, we developed an anthropometry-based nomogram for predicting IR among adolescents, that incorporated sex, age, WHR, weight and BMI, and which may facilitate health counselling, self-risk assessments and early detection of IR.
The processed data that support the findings of this study are available from the corresponding author upon reasonable request.
The studies involving human participants were reviewed and approved by Ethics Committee of Liaoyang Diabetes Hospital. Written informed consent to participate in this study was provided by the participants’ legal guardian/next of kin.
RD and YW conceptualized the study, carried out the initial analyses, and drafted the initial manuscript. PL and LL designed the data collection instruments, collected data, and revised the manuscript. All authors have read and agreed to the published version of the manuscript.
The study was supported by the scientific and technological planning project of Liaoning Province (Grant 2008225009-21).
The authors declare that the research was conducted in the absence of any commercial or financial relationships that could be construed as a potential conflict of interest.
All claims expressed in this article are solely those of the authors and do not necessarily represent those of their affiliated organizations, or those of the publisher, the editors and the reviewers. Any product that may be evaluated in this article, or claim that may be made by its manufacturer, is not guaranteed or endorsed by the publisher.
The authors would like to thank all the nurses and physicians at Liaoyang Diabetes Hospital for assisting in the acquisition of data.
1. Drozdz D, Alvarez-Pitti J, Wojcik M, Borghi C, Gabbianelli R, Mazur A, et al. Obesity and Cardiometabolic Risk Factors: From Childhood to Adulthood. Nutrients (2021) 13:4176. doi: 10.3390/nu13114176
2. Morandi A, Di Sessa A, Zusi C, Umano GR, El Mazloum D, Fornari E, et al. Nonalcoholic Fatty Liver Disease and Estimated Insulin Resistance in Obese Youth: A Mendelian Randomization Analysis. J Clin Endocrinol Metab (2020) 105:1175–83. doi: 10.1210/clinem/dgaa583
3. Di Bonito P, Pacifico L, Licenziati MR, Maffeis C, Morandi A, Manco M, et al. Elevated Blood Pressure, Cardiometabolic Risk and Target Organ Damage in Youth With Overweight and Obesity. Nutr Metab Cardiovasc Dis (2020) 30:1840–7. doi: 10.1016/j.numecd.2020.05.024
4. Tam CS, Xie W, Johnson WD, Cefalu WT, Redman LM, Ravussin E. Defining Insulin Resistance From Hyperinsulinemic-Euglycemic Clamps. Diabetes Care (2012) 35:1605–10. doi: 10.2337/dc11-2339
5. Matthews DR, Hosker JP, Rudenski AS, Naylor BA, Treacher DF, Turner RC. Homeostasis Model Assessment: Insulin Resistance and Beta-Cell Function From Fasting Plasma Glucose and Insulin Concentrations in Man. Diabetologia (1985) 28:412–9. doi: 10.1007/bf00280883
6. Szurkowska M, Szafraniec K, Gilis-Januszewska A, Szybinski Z, Huszno B. Insulin Resistance Indices in Population-Based Study and Their Predictive Value in Defining Metabolic Syndrome. Przegl Epidemiol (2005) 59:743–51.
7. Keskin M, Kurtoglu S, Kendirci M, Atabek ME, Yazici C. Homeostasis Model Assessment is More Reliable Than the Fasting Glucose/Insulin Ratio and Quantitative Insulin Sensitivity Check Index for Assessing Insulin Resistance Among Obese Children and Adolescents. Pediatrics (2005) 115:e500–503. doi: 10.1542/peds.2004-1921
8. Tobisch B, Blatniczky L, Barkai L. Cardiometabolic Risk Factors and Insulin Resistance in Obese Children and Adolescents: Relation to Puberty. Pediatr Obes (2015) 10:37–44. doi: 10.1111/j.2047-6310.2013.00202.x
9. Malavazos AE, Capitanio G, Milani V, Ambrogi F, Matelloni IA, Basilico S, et al. Tri-Ponderal Mass Index vs Body Mass Index in Discriminating Central Obesity and Hypertension in Adolescents With Overweight. Nutr Metab Cardiovasc Dis (2021) 31:1613–21. doi: 10.1016/j.numecd.2021.02.013
10. Munoz-Hernando J, Escribano J, Ferre N, Closa-Monasterolo R, Grote V, Koletzko B, et al. Usefulness of the Waist-to-Height Ratio for Predicting Cardiometabolic Risk in Children and its Suggested Boundary Values. Clin Nutr (2022) 41:508–16. doi: 10.1016/j.clnu.2021.12.008
11. Romualdo MC, Nobrega FJ, Escrivao MA. Insulin Resistance in Obese Children and Adolescents. J Pediatr (Rio J) (2014) 90:600–7. doi: 10.1016/j.jped.2014.03.005
12. Friedewald WT, Levy RI, Fredrickson DS. Estimation of the Concentration of Low-Density Lipoprotein Cholesterol in Plasma, Without Use of the Preparative Ultracentrifuge. Clin Chem (1972) 18:499–502. doi: 10.1093/clinchem/18.6.499
13. Li H, Ji CY, Zong XN, Zhang YQ. Body Mass Index Growth Curves for Chinese Children and Adolescents Aged 0 to 18 Years. Zhonghua Er Ke Za Zhi (2009) 47:493–8.
14. Song Y, Ma J, Li LB, Dong B, Wang Z, Agardh A. Secular Trends for Age at Spermarche Among Chinese Boys From 11 Ethnic Minorities, 1995-2010: A Multiple Cross-Sectional Study. BMJ Open (2016) 6:e010518. doi: 10.1136/bmjopen-2015-010518
15. Song Y, Ma J, Hu PJ, Zhang B. Study of Geographic Distribution and 10 Years' Change of Spermarche in Chinese Boys of Han-Group Aged 11 to 18. Zhonghua Yu Fang Yi Xue Za Zhi (2011) 45:522–6.
16. Lian Q, Mao Y, Luo S, Zhang S, Tu X, Zuo X, et al. Puberty Timing Associated With Obesity and Central Obesity in Chinese Han Girls. BMC Pediatr (2019) 19:1. doi: 10.1186/s12887-018-1376-4
17. Song Y, Ma J, Agardh A, Lau PW, Hu P, Zhang B. Secular Trends in Age at Menarche Among Chinese Girls From 24 Ethnic Minorities, 1985 to 2010. Glob Health Action (2015) 8:26929. doi: 10.3402/gha.v8.26929
18. Song Y, Ma J, Wang HJ, Wang Z, Hu P, Zhang B, et al. Trends of Age at Menarche and Association With Body Mass Index in Chinese School-Aged Girls, 1985-2010. J Pediatr (2014) 165:1172–7.e1171. doi: 10.1016/j.jpeds.2014.08.013
19. Barja S, Arnaiz P, Dominguez A, Villarroel L, Cassis B, Castillo O, et al. Normal Plasma Insulin and HOMA Values Among Chilean Children and Adolescents. Rev Med Chil (2011) 139:1435–43. doi: 10.4067/S0034-98872011001100007
20. Beck-Nielsen H. General Characteristics of the Insulin Resistance Syndrome: Prevalence and Heritability. European Group for the Study of Insulin Resistance (EGIR). Drugs (1999) 58(Suppl 1):7–10; discussion 75-82. doi: 10.2165/00003495-199958001-00003
21. Song P, Yu J, Chang X, Wang M, An L. Prevalence and Correlates of Metabolic Syndrome in Chinese Children: The China Health and Nutrition Survey. Nutrients (2017) 9:79. doi: 10.3390/nu9010079
22. Zimmet P, Alberti G, Kaufman F, Tajima N, Silink M, Arslanian S, et al. The Metabolic Syndrome in Children and Adolescents. Lancet (2007) 369:2059–61. doi: 10.1016/S0140-6736(07)60958-1
23. Ma GS, Ji CY, Ma J, Mi J, Sung R, Xiong F, et al. Waist Circumference Reference Values for Screening Cardiovascular Risk Factors in Chinese Children and Adolescents Aged 7 - 18 Years. Zhonghua Liu Xing Bing Xue Za Zhi (2010) 31:609–15.
24. American Academy of Pediatrics, Committee on Nutrition. American Academy of Pediatrics. Committee on Nutrition. Cholesterol in Childhood. Pediatrics (1998) 101:141–7. doi: 10.1542/peds.101.1.141
25. Ford ES, Li C, Cook S, Choi HK. Serum Concentrations of Uric Acid and the Metabolic Syndrome Among US Children and Adolescents. Circulation (2007) 115:2526–32. doi: 10.1161/CIRCULATIONAHA.106.657627
26. Shah AS, Dolan LM, Gao Z, Kimball TR, Urbina EM. Clustering of Risk Factors: A Simple Method of Detecting Cardiovascular Disease in Youth. Pediatrics (2011) 127:e312–318. doi: 10.1542/peds.2010-1125
27. Friedman J, Hastie T, Tibshirani R. Regularization Paths for Generalized Linear Models via Coordinate Descent. J Stat Softw (2010) 33:1–22. doi: 10.18637/jss.v033.i01
28. D'Addabbo A, Latiano A, Palmieri O, Maglietta R, Annese V, Ancona N. Regularized Least Squares Classifiers may Predict Crohn's Disease From Profiles of Single Nucleotide Polymorphisms. Ann Hum Genet (2007) 71:537–49. doi: 10.1111/j.1469-1809.2007.00349.x
29. Pan XF, Wang L, Pan A. Epidemiology and Determinants of Obesity in China. Lancet Diabetes Endocrinol (2021) 9:373–92. doi: 10.1016/S2213-8587(21)00045-0
30. Di Bonito P, Valerio G, Licenziati MR, Miraglia Del Giudice E, Baroni MG, Morandi A, et al. High Uric Acid, Reduced Glomerular Filtration Rate and non-Alcoholic Fatty Liver in Young People With Obesity. J Endocrinol Invest (2020) 43:461–8. doi: 10.1007/s40618-019-01130-6
31. Hill MA, Yang Y, Zhang L, Sun Z, Jia G, Parrish AR, et al. Insulin Resistance, Cardiovascular Stiffening and Cardiovascular Disease. Metabolism (2021) 119:154766. doi: 10.1016/j.metabol.2021.154766
32. Banerjee D, Biggs ML, Mercer L, Mukamal K, Kaplan R, Barzilay J, et al. Insulin Resistance and Risk of Incident Heart Failure: Cardiovascular Health Study. Circ Heart Fail (2013) 6:364–70. doi: 10.1161/CIRCHEARTFAILURE.112.000022
33. Lee JM, Okumura MJ, Davis MM, Herman WH, Gurney JG. Prevalence and Determinants of Insulin Resistance Among U.S. Adolescents: A Population-Based Study. Diabetes Care (2006) 29:2427–32. doi: 10.2337/dc06-0709
34. Ballerini MG, Bergada I, Rodriguez ME, Keselman A, Bengolea VS, Pipman V, et al. Insulin Level and Insulin Sensitivity Indices Among Healthy Children and Adolescents. Arch Argent Pediatr (2016) 114:329–36. doi: 10.5546/aap.2016.32910.5546/aap.2016.eng.329
35. Nogueira-de-Almeida CA, de Mello ED. Different Criteria for the Definition of Insulin Resistance and Its Relation With Dyslipidemia in Overweight and Obese Children and Adolescents. Pediatr Gastroenterol Hepatol Nutr (2018) 21:59–67. doi: 10.5223/pghn.2018.21.1.59
36. Yin J, Li M, Xu L, Wang Y, Cheng H, Zhao X, et al. Insulin Resistance Determined by Homeostasis Model Assessment (HOMA) and Associations With Metabolic Syndrome Among Chinese Children and Teenagers. Diabetol Metab Syndr (2013) 5:71. doi: 10.1186/1758-5996-5-71
37. Yi KH, Hwang JS, Kim EY, Lee SH, Kim DH, Lim JS. Prevalence of Insulin Resistance and Cardiometabolic Risk in Korean Children and Adolescents: A Population-Based Study. Diabetes Res Clin Pract (2014) 103:106–13. doi: 10.1016/j.diabres.2013.10.021
38. Atabek ME, Pirgon O. Assessment of Insulin Sensitivity From Measurements in Fasting State and During an Oral Glucose Tolerance Test in Obese Children. J Pediatr Endocrinol Metab (2007) 20:187–95. doi: 10.1515/jpem.2007.20.2.187
39. Kurtoglu S, Hatipoglu N, Mazicioglu M, Kendirici M, Keskin M, Kondolot M. Insulin Resistance in Obese Children and Adolescents: HOMA-IR Cut-Off Levels in the Prepubertal and Pubertal Periods. J Clin Res Pediatr Endocrinol (2010) 2:100–6. doi: 10.4274/jcrpe.v2i3.100
40. Lee SH, Ahn MB, Choi YJ, Kim SK, Kim SH, Cho WK, et al. Comparison of Different Criteria for the Definition of Insulin Resistance and its Relationship to Metabolic Risk in Children and Adolescents. Ann Pediatr Endocrinol Metab (2020) 25:227–33. doi: 10.6065/apem.2040002.001
41. Juarez-Lopez C, Klunder-Klunder M, Medina-Bravo P, Madrigal-Azcarate A, Mass-Diaz E, Flores-Huerta S. Insulin Resistance and its Association With the Components of the Metabolic Syndrome Among Obese Children and Adolescents. BMC Public Health (2010) 10:318. doi: 10.1186/1471-2458-10-318
42. Gobato AO, Vasques AC, Zambon MP, Barros Filho Ade A, Hessel G. Metabolic Syndrome and Insulin Resistance in Obese Adolescents. Rev Paul Pediatr (2014) 32:55–62. doi: 10.1590/s0103-05822014000100010
43. Morais PR, Sousa AL, Jardim Tde S, Nascente FM, Mendonca KL, Povoa TI, et al. Correlation of Insulin Resistance With Anthropometric Measures and Blood Pressure in Adolescents. Arq Bras Cardiol (2016) 106:319–26. doi: 10.5935/abc.20160041
Keywords: adolescent, anthropometry, cardiometabolic risk factors, China, homeostasis model assessment, insulin resistance, nomogram
Citation: Du R, Li L, Li P and Wang Y (2022) Impact of Insulin Resistance on Cardiometabolic Risk Factors and an Anthropometry-Based Predictive Nomogram for Insulin Resistance Among Adolescents in China. Front. Endocrinol. 13:852395. doi: 10.3389/fendo.2022.852395
Received: 11 January 2022; Accepted: 28 February 2022;
Published: 28 March 2022.
Edited by:
Jamie Lynn Young, University of Louisville, United StatesReviewed by:
Maurizio Delvecchio, Giovanni XXIII Children’s Hospital, ItalyCopyright © 2022 Du, Li, Li and Wang. This is an open-access article distributed under the terms of the Creative Commons Attribution License (CC BY). The use, distribution or reproduction in other forums is permitted, provided the original author(s) and the copyright owner(s) are credited and that the original publication in this journal is cited, in accordance with accepted academic practice. No use, distribution or reproduction is permitted which does not comply with these terms.
*Correspondence: Yanjun Wang, d2FuZ3lqc3lAMTYzLmNvbQ==
Disclaimer: All claims expressed in this article are solely those of the authors and do not necessarily represent those of their affiliated organizations, or those of the publisher, the editors and the reviewers. Any product that may be evaluated in this article or claim that may be made by its manufacturer is not guaranteed or endorsed by the publisher.
Research integrity at Frontiers
Learn more about the work of our research integrity team to safeguard the quality of each article we publish.