- 1Department of Pediatrics, The Second Affiliated Hospital of Xi’an Jiao Tong University, Xi’an, China
- 2Department of Joint Surgery, HongHui Hospital, Xi’an Jiao Tong University, Xi’an, China
Menarche is the first occurrence of menstrual bleeding and one of the most important events of female puberty. Alarmingly, over the last several decades, the mean age at menarche (AAM) has decreased. Environmental endocrine disruptors (EEDs) are chemicals that may interfere with the endocrine system, resulting in adverse developmental, immunological, neurological, and reproductive effects in humans. Thus, the effects of EEDs on fertility and reproduction are growing concerns in modern societies. In this study, we aimed to determine the influence of genetic and environmental factors on AAM. We used data from an AAM genome-wide association study of 329,345 women to conduct a transcriptome-wide association study (TWAS) with FUSION software. As references, we determined the gene-expression levels in the hypothalamus, pituitary gland, ovaries, uterus, and whole blood. We performed Gene Ontology and Kyoto Encyclopedia of Genes and Genomes enrichment analyses using the significantly dysregulated genes identified by the TWAS. Using the STRING database, we also generated a protein–protein-interaction network to analyze common AAM-specific genes identified by the TWAS with different tissues. We performed chemical-related gene set enrichment analysis (CGSEA) and identified significant TWAS genes to uncover relationships between different chemicals and AAM. The TWAS identified 9,848 genes; among these, 1580 genes were significant (P < 0.05), and 11 genes were significant among the hypothalamus, pituitary, ovary, uterus, and whole blood. CGSEA identified 1,634 chemicals, including 120 chemicals significantly correlated with AAM. In summary, we performed a TWAS (for genetic factors) and CGSEA (for environmental factors) focusing on AAM and identified several AAM-associated genes and EEDs. The results of this study expand our understanding of genetic and environmental factors related to the onset of female puberty.
Introduction
Puberty is a complex process occurring between childhood and adulthood, producing internal and external physical changes that promote the development of primary and secondary sexual characteristics important for sexual reproduction (1). Sex hormones are responsible for the physical manifestations of female puberty, including thelarche, pubarche, and menarche (2). Menarche is the first menstrual bleeding and one of the most important events of female puberty. Over the last several decades, the mean age at menarche (AAM) has declined, which is concerning (3). Epidemiological evidence suggests that the onset of puberty is advancing in humans through undetermined mechanisms (4).
Environmental endocrine disruptors (EEDs) are chemicals that interfere with the endocrine system and evoke adverse developmental, immunological, neurological, and reproductive effects in humans (5). EEDs are common in human living environments and include pesticides, plasticizers, industrial by-products, drugs, and some naturally occurring phytochemicals (6). These exogenous chemicals can interfere with the complex endocrine system, causing adverse health effects, such as reproductive disorders, metabolic diseases, and various cancers (7). Chronic exposure to EEDs may play a role in accelerating or delaying the onset of menarche, and extensive research has shown that pesticides, phenols, polycyclic aromatic hydrocarbons, phthalates, and some heavy metals are responsible for hormone metabolism disorders occurring during puberty (8). Consequently, the effects of EEDs on fertility and reproduction are a growing concern in modern societies (9).
Recent genome-wide association studies (GWASs) have identified thousands of genetic variants associated with complex phenotypes and have provided insights into their genetic architectures. GWASs are also extremely well-suited for identifying common single-nucleotide polymorphism (SNP)-based variants (10). GWAS analysis has been conducted to research early puberty and identify the genetic characteristics of idiopathic central precocious puberty and validate the polygenic risk for early puberty (11). Felix R Day et al. have identified 389 independent, genome-wide significant signals for AAM, which explained ~7.4% of the population variance in AAM and corresponded to ~25% of the estimated heritability (11).
Genetic loci cause trait variations, ranging from growth and fitness in simple organisms to disease in humans. Determining the genetics of gene-expression differences has emerged as a key approach for linking DNA-sequence variations to phenotypes (12). Transcriptome-wide association study (TWAS) analysis has been used to identify significant expression-trait associations by integrating genotypes, gene-expression levels, and phenotypes in order to gain insights into the genetic basis of complex traits (13). In a recent study, a TWAS was performed to discover transcriptome differences that affect the age of natural menopause (ANM), and 34 ANM-associated genes were reported (14). The hypothalamic–pituitary–gonadal axis controls puberty and reproduction (15). Therefore, investigating the associated gene-regulation relationships may help identify important genes that are co-expressed in all tissue types during AAM.
Here, we aimed to determine the influences of genetic and environmental factors on AAM by performing a large-scale TWAS for AAM based on a GWAS data set. We investigated gene-expression levels in the hypothalamus, pituitary gland, ovaries, uterus, and whole blood. We also reevaluated the expression of TWAS-identified genes, functionally explored the genes, and identified AAM-associated EEDs.
Methods
Summary of the AAM GWAS Data Used in This Study
We used published GWAS summary data for female AAM (16). Briefly, Day et al. performed a meta-analysis of pooled GWAS data from multiple studies of 329,345 women of European ancestry, including 40 studies from the ReproGen consortium (N = 179,117), 23andMe (N = 76,831), and the United Kingdom Biobank (N = 73,397). The results were grouped into the relatively sparse HapMap 2 reference panel or attributed to the 1000 Genomes Project reference panel using gene-centric arrays. In each study, the associations of SNPs with AAM were based on a two-tailed additive linear-regression model and several factors, including the age at the study visit and other study-specific covariates. Day et al. performed an expanded genomic analysis of AAM in women that was nearly three times larger and used denser genomic data than previous studies. Information on the subjects, genotypes, responsibilities, and quality control were detailed in the published study (16). In addition, ethical approval was not applicable for this study as publicly available data were used for the analysis.
TWAS Analysis
We used FUSION (13) software (http://gusevlab.org/projects/fusion/) to analyze the GWAS summary data for the previous meta-analysis of AAM. The most popular TWAS methods, such as PrediXcan, TWAS-Fusion, and SMR, test causal relationships between gene-expression levels and complex traits (17), among which, the TWAS-Fusion method is used more often. Briefly, Bayesian sparse linear-mixed models (18) were used to calculate SNP expression weights for specific genes at the 1-Mb cis position and estimate the association of predicted expression levels with AAM using the following formula: Ztwas = w + Z/(w × [Lw]1/2) (13), where w denotes the weight, Z denotes the Z-score, and L denotes the SNP correlation matrix (definition, LD). We used the gene-expression weights for the hypothalamus (N samples = 108; N features = 2,315), pituitary (N samples = 157; N features = 4,402), ovary (N samples = 122; N features = 2,809), uterus (N samples = 101; N features = 2,135), and whole blood (N samples = 1,264; N features = 4,701) as references, which are available for download from the FUSION website (http://gusevlab.org/projects/fusion/). We estimated the transcriptome-wide significance as P = 5.08 × 10−6 (0.05/9,848) using the Bonferroni correction (14). Manhattan plot was made by “CMplot”(v. 3.6.2) in R package.
Functional Exploration of Genes
We performed Kyoto Encyclopedia of Genes and Genomes (KEGG) (19) and Gene Ontology (GO) (20) enrichment analyses to identify and confirm related biological processes. KEGG and GO enrichment were performed using the R packages “org.Hs.eg.db” and “clusterProfiler” (R Foundation for Statistical Computing, Vienna, Austria. https://www.R-project.org/).
Interaction-Network Analysis
We generated a protein–protein-interaction (PPI) network using the STRING database, v11.5 (STRING, https://string-db.org), requiring a confidence score of 0.15 and “active interaction sources” based on a previous study (21). Cytoscape (22) was used to visualize all interaction networks, and the Molecular Complex Detection (MCODE) plugin (23) was used for module analysis.
Chemical Gene Expression Annotation Data Set
The chemical-related gene-expression annotation data set used in this study was downloaded from the Comparative Toxicology Genomics Database (CTD) (http://ctdbase.org/downloads/). The CTD mainly provides four data sets, including a chemical–gene-interaction function, a chemical–disease association, a genetic disease association, and a chemical element-phenotypic association. The CTD integrates the four data sets to automatically construct a hypothetical chemical–gene phenotype disease network to illustrate the molecular mechanisms underlying diseases that affect the environment (24). Cheng et al. downloaded and used 1,788,149 chemical–gene pair annotation terms for humans and mice, generating 11,190 chemical substance-related gene sets (25). We also used that data set to perform our chemical-related gene set enrichment analysis (CGSEA).
CGSEA
CGSEA is a flexible tool for assessing associations between chemicals and complex diseases. Briefly, the software uses genome-wide summary data (e.g., a summary of the TWAS data and messenger RNA [mRNA]-expression profiles) to explore functional relationships among chemical substances and diseases from a genomics perspective, for many complex diseases and characteristics. We used the CTD Chemical Gene Interaction Network and TWAS Expression Association to test the AAM statistics and weighted Kolmogorov–Smirnov running sum statistics to explore the relationships between chemicals and AAM, as described previously in greater detail (26). Specifically, in this study, we performed 10,000 permutations to obtain the empirical distribution of the gene set enrichment analysis (GSEA) statistical data for each chemical and then calculated the P-value of each chemical based on the empirical distribution of the CGSEA statistical data. Based on previous findings, we excluded gene sets containing <10 or >200 genes to limit the influence of gene set sizes on the results (27). To avoid deviations from expression correlations between genes, a package of lme4qtl software (28) was used to adapt the mixed-model regression of the TWAS Z-score based on the number of members in each gene set, in order to consider the correlation of the Z-score between genes caused by LD (28). A detailed description of the analytical method used was provided previously (25).
Results
TWAS Analysis of AAM
TWAS analysis identified 9,848 genes from the GWAS summary data and of those 1,580 genes expression was associated with AAM (P <0.05) while 64 showed a significant association (P <5.08 × 10−6); 2,289 genes tested in hypothalamus and of 322 genes expression was associated with AAM (P <0.05) while 8 showed a significant association (P <5.08 × 10-6; Figure 1A), 4,362 genes tested in pituitary and of 568 genes expression was associated with AAM (P <0.05) while 26 showed a significant association (P <5.08 × 10−6; Figure 1B), 2,768 genes tested in ovary and of 366 genes expression was associated with AAM (P <0.05) while 14 showed a significant association (P <5.08 × 10−6; Figure 1C), 2,104 genes tested in uterus and of 253 genes expression was associated with AAM (P <0.05) while 7 showed a significant association (P <5.08 × 10−6; Figure 1D), and 4,671 genes tested in whole blood and of 604 genes expression was associated with AAM (P <0.05) while 9 showed a significant association (P <5.08 × 10−6; Figure 1E), respectively (Supplementary Information).
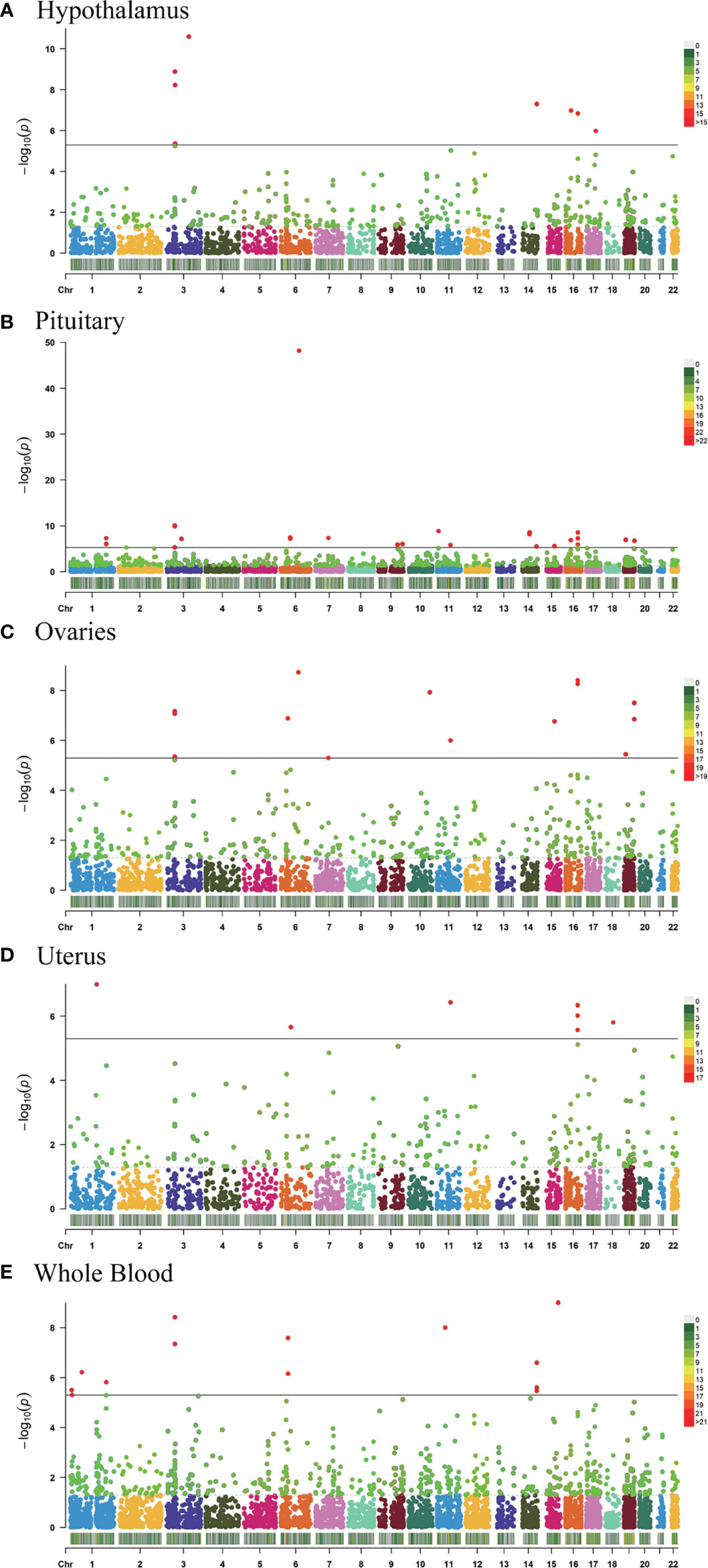
Figure 1 Manhattan plots of the association results from the AAM TWAS. The dashed horizontal lines represent P = 5.00 × 10−2. The solid horizontal lines represent P = 5.00 × 10−6 (Bonferroni correction). Each dot represents the genetically predicted expression of one specific gene in the hypothalamus, pituitary gland, ovary, uterus, and whole blood tissues. The X axis represents the chromosome (Chr) encoding the corresponding gene, and the Y axis represents the negative logarithm of the association P TWAS value. (A) Gene-expression weights for the hypothalamus. (B) Gene-expression weights for the pituitary gland. (C) Gene-expression weights for the ovaries. (D) Gene-expression weights for the uterus. (E) Gene-expression weights for whole blood.
Functional Exploration of TWAS-Identified Genes Associated With AAM
Tissues have unique gene-expression profiles. Thus, we performed an overlap analysis of the significant genes in different tissues to identify the most representative genes. Figure 2A shows the resulting Venn diagram, which indicates the number of genes expressed in one or more tissues. Overall, 163 TWAS-identified significant AAM-specific genes were associated with the hypothalamus: 38 significant genes were associated with the hypothalamus and pituitary gland; 7 significant genes were associated with the hypothalamus, pituitary, and ovary; and 11 significant genes were associated with the hypothalamus, pituitary, ovary, uterus, and whole blood. The 11 novel TWAS-significant AAM-susceptible genes identified in all five tissues were RBM6 (RNA-binding motif protein 6; chromosome 3), PILRB (paired immunoglobin-like type 2 receptor beta; chromosome 7), CPSF1 (cleavage and polyadenylation-specific factor 1; chromosome 8), INPP5E (inositol polyphosphate-5-phosphatase E; chromosome 9), MRPL43 (mitochondrial ribosomal protein L43; chromosome 10), HSD17B12 (hydroxysteroid-(17-β)-dehydrogenase 12; chromosome 11), TIPIN (TIMELESS-interacting protein; chromosome 15), FLYWCH1 (FLYWCH-type zinc finger 1; chromosome 16), EXOSC6 (exosome component 6; chromosome 16), ADORA2B (adenosine A2b receptor; chromosome 17), and SPATA20 (spermatogenesis-associated 20; chromosome 17). Table 1 presents detailed information regarding these 11 genes, including the rsIDs of the most significant (i.e., best) GWAS SNPs in the locus (i.e., BEST.GWAS.ID) and the TWAS P-value (i.e., P TWAS).
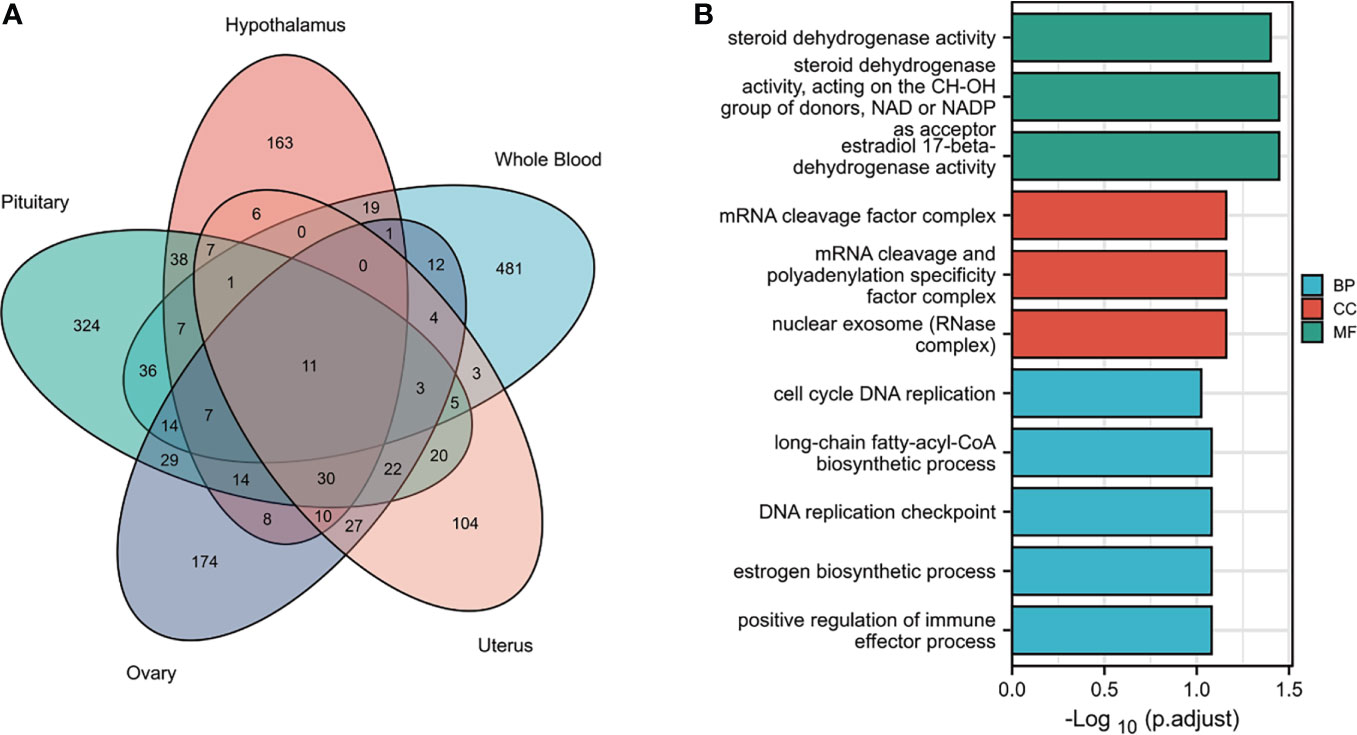
Figure 2 Functional exploration of the TWAS-identified genes associated with AAM. (A) Venn diagram revealing the overlap of TWAS-significant genes in different tissues. Red, hypothalamus; green, pituitary gland; purple, ovaries orange, uterus; blue, whole blood. (B) Bar plot of enriched GO terms for the overlapping genes.
We subjected the TWAS-identified genes to GO analysis (Figure 2B). Five enriched GO terms belonged to the biological process (BP) category, including cell cycle DNA replication, long-chain fatty-acyl-CoA metabolic process, DNA replication checkpoints, estrogen biosynthetic processes, and positive regulation of immune effector processes. Three significantly enriched GO terms belonged to the cellular component (CC) category, including the mRNA cleavage factor complex, mRNA cleavage and polyadenylation specificity factor complex, and nuclear exosome (RNase complex). In terms of the molecular function (MF) category, the enriched GO terms primarily involved estrogen metabolism (such as steroid dehydrogenase activity or steroid dehydrogenase activity acting on the CH-OH group of donors), nicotinamide adenine dinucleotide or nicotinamide adenine dinucleotide phosphate as an acceptor, and estradiol 17-beta-dehydrogenase activity.
PPI Network of the TWAS-Identified Genes
We used 1,580 TWAS-significant AAM-associated genes for PPI analysis and successfully transformed 1,056 protein-coding genes (Figure 3A). To effectively find densely connected regions of the PPI network, we formed seven MCODE clusters with the PPI network genes (Figures 3B–H). The hub genes identified using the MCODE plugin were further analyzed for functional exploration. MCODE cluster 1 (MCODE1) was related to ribosome biogenesis, female pregnancy, and blastocyst development. MCODE2 was characterized by genes in the human leukocyte antigen (HLA) family associated with the immune process. MCODE3, MCODE4, and MCODE5 were related to tRNA, energy metabolism processes, and biosynthetic processes. MCODE6 was associated with lipid oxidation and maternal processes involved in female pregnancy. MCODE7 contained some important significantly enriched terms, including fatty acid metabolic, gonadotropin-releasing hormone (GnRH), estrogen, oxytocin, and PI3K-Akt signaling pathways and breast cancer.
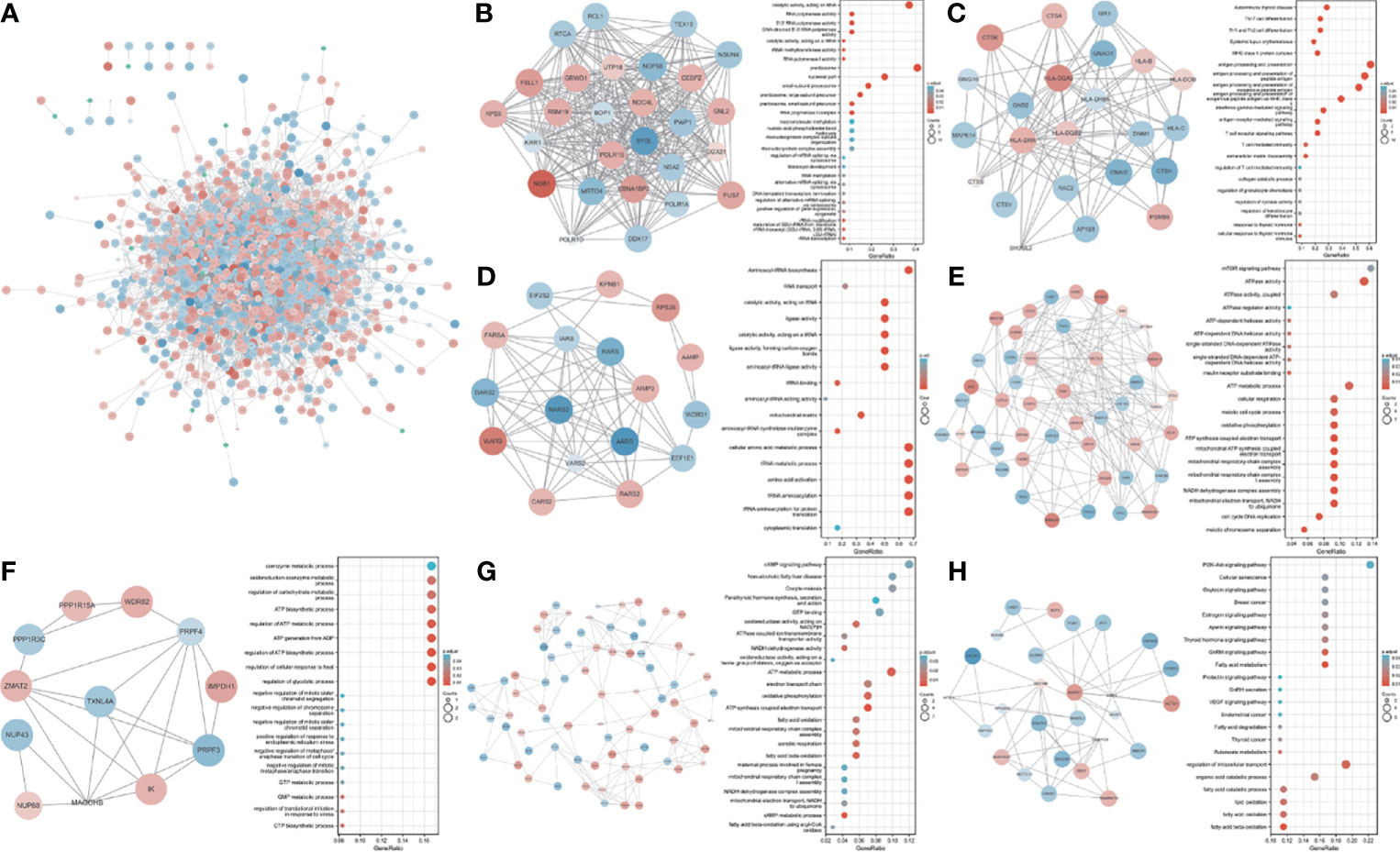
Figure 3 PPI network and significant modules. Red and blue circles indicate upregulated and downregulated TWAS-identified genes. (A) PPI network of the TWAS-identified genes. (B-H) Significant modules 1 to 7 of the PPI network and their functional exploration. GeneRatio: the ratio of the number of genes associated with a term linked to an enriched gene to the total number of enriched genes.
CGSEA of the TWAS-Identified Genes
We performed CGSEA to investigate environmental factors that influence the onset of puberty, which identified 1,634 chemicals, including 120 chemicals that correlated significantly with AAM. These significant chemicals included some drugs (e.g., fluoxetine), pesticides (e.g., ametryne), plant extracts (e.g., isoflavones), nutrients (e.g., cholesterol), phenols (e.g., cannabidiol), phthalates (e.g., monobutyl phthalate), heavy metals (e.g., uranium), and atmospheric pollutants (e.g., phosgene). Figure 4 illustrates our constructed network of EEDs and their target genes based on the TWAS-identified genes. Chemical with absolute normalize enrichment score (NES) values of >1 were considered significantly enriched according to GSEA. We identified 77 significantly enriched chemicals with an |NES| value of >1 and a P-value of <0.05 (Table 2).
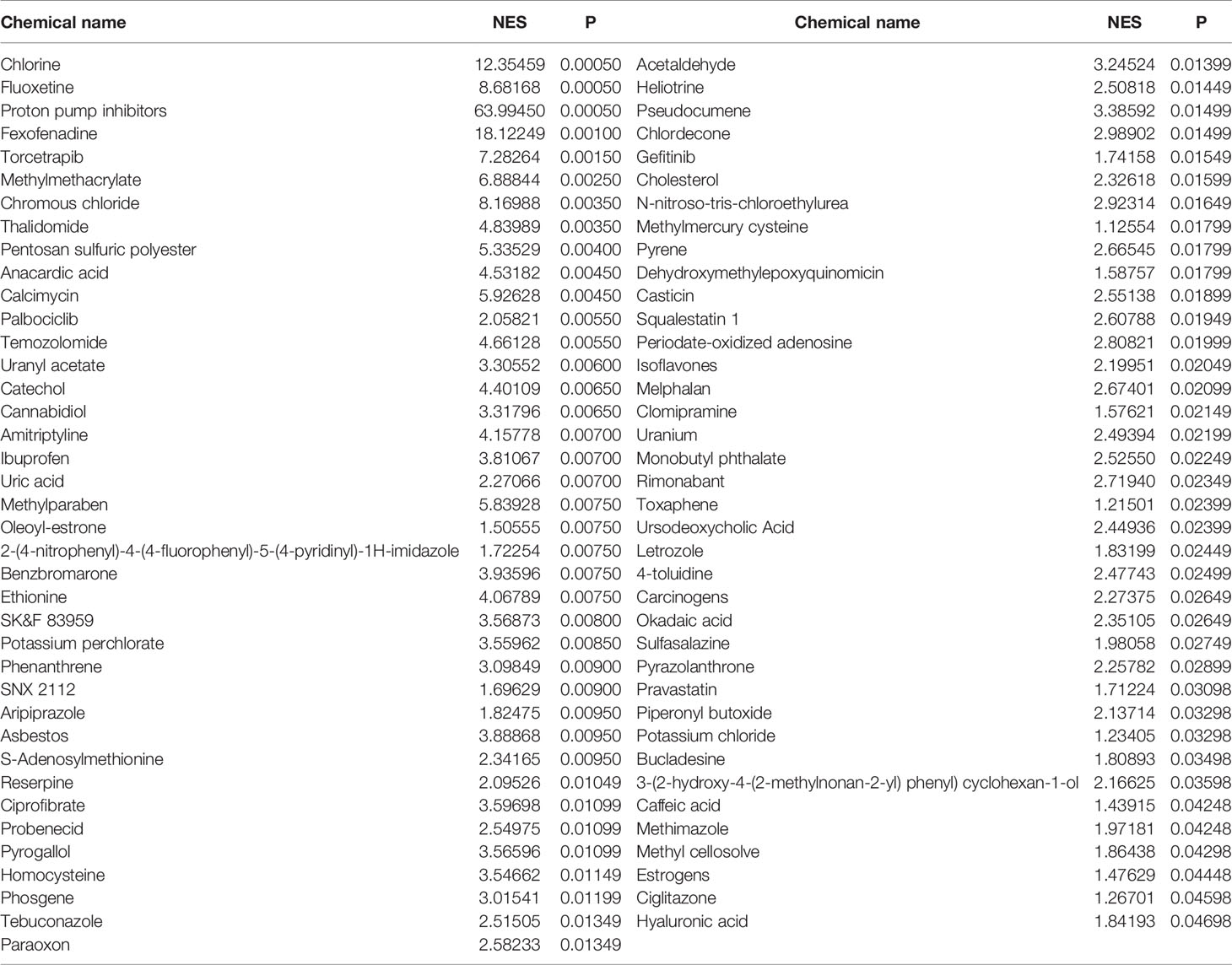
Table 2 Significant chemicals identified by CGSEA of the significant TWAS-identified AAM-associated genes.
Discussion
Puberty is a developmental period affecting the body and behavior of children developing secondary sexual characteristics (29). Pubertal hormones play important roles in the adrenal, gonadal, and growth axes (30). Pubertal timing is determined by the activation of pulsatile hypothalamic GnRH secretion when pituitary gonadotropin secretion and downstream gonadal maturation are also initiated (31). Thus, various tissue types are affected by AAM, including the hypothalamus, pituitary gland, ovaries, uterus, and whole blood.
Up to now, previous studies have identified several genes associated with AAM, these results are similar to our studies (Table 3). Here, we performed a comprehensive TWAS to evaluate the relationship between AAM and predicted genes found in the hypothalamus, pituitary gland, ovaries, uterus, and whole blood. We identified 11 genes whose genetically predicted expression was associated with AAM (P TWAS ≤ 0.05), including 10 novel genes (RBM6, PILRB, CPSF1, INPP5E, MRPL43, TIPIN, FLYWCH1, EXOSC6, ADORA2B, and SPATA20) and one gene (HSD17B12) linked to AAM in a previous GWAS. The 11 protein-coding genes identified in our study have been implicated in estrogen metabolism. We also identified enriched fatty acid-metabolism pathways, similar to the findings of a recent study (36), indicating that different types of fatty acids may influence puberty timing. Additionally, individual fatty acids might have different physiological and metabolic effects, including the progression of pubertal development. Therefore, our study provides new information that improves our understanding of the genetics and etiology of AAM.
HSD17B12 encodes 17β-hydroxysteroid dehydrogenase (17β-HSD), which is crucial for converting estrone into estradiol and fatty acid elongation. Our results support those of Kemiläinen et al. (37), who found that HSD17B12 played a vital role in female fertility through arachidonic acid metabolism. Specifically, an investigation of the 17β-HSD enzyme expression in human and mouse ovaries revealed that female HSD17B+/- mice more often had frequent dysfunctional oogenesis and ovulation, leading to less frequent births. Under extreme circumstances, haploinsufficiency of the HSD17B12 gene in female mice resulted in subfertility. HSD17B12 can affect fatty acid elongation and ceramide accumulation in the serum (38). Previous data showed that central ceramides participate in the timing of female puberty (39), suggesting that HSD17B12 could be a candidate gene for female puberty. Notably, HSD17B12 was also related to endometrial (40), ovarian (41), and breast (42) cancers.
Previous results have shown that INPP5E is concentrated at the cilia base, where it helps control phosphoinositide metabolism (43). Primary cilia are present on virtually all cell types. Cilia appear to exert a crucial modulatory role in appropriate axonal wiring due to INPP5E-dependent activation of the PI3K–AKT signaling pathway, triggering an axonal Ca2+ wave (44). GnRH neurons in adults are multi-ciliated, and the percentage of GnRH neurons possessing multiple Kiss1r-positive cilia increases during puberty, correlating with sexual maturation (45). ADORA2B encodes an adenosine receptor and is involved in axon elongation. A previous report showed that ADORA2B transcripts were significantly downregulated in GnRH neurons during proestrus (46), while it has not been studied in pituitary. Therefore, these newly defined genes may influence the electrophysiology of puberty in hypothalamus and pituitary gland.
TIPIN is part of the replisome complex and binds the replication fork-protection complex TIMELESS, which is involved in circadian rhythm regulation (47). Circadian rhythms are well known to play key roles in animal reproduction (48). The existing body of research suggests that during the chronotype and the circadian timekeeping system change during the puberty, including endogenous rhythm period and sensitivity to environmental time cues (49). The results of several studies have established that melatonin promotes follicle-stimulating hormone in the pituitary gland and increases serum estrogen levels, thereby accelerating the onset of puberty (50). Those studies indirectly support a potential role of TIPIN in AAM.
CPSF recognizes the AAUAAA signal in pre-mRNA and interacts with other factors to facilitate RNA cleavage and the poly(A) addition. CPSF1 is the largest subunit of the CPSF complex. Previous data established that CPSF1 may promote ovarian cancer (51), cell proliferation, and triple-negative breast cancer (52). However, women have a higher risk for developing autoimmune diseases than men, which is attributed to sweeping endocrinological changes during puberty that considerably affect the immune system (53). CPSF1 was previously found to have a high affinity for HLA molecules (54). Thus, CPSF1 may be linked to the immune response in adolescent girls.
We extended the well-established GSEA approach to detect associations between environmental chemicals and AAM using published GWAS summary data sets and identified 120 chemicals, including drugs, organic compounds, inorganic compounds, plant extracts, nutrients, phenols, plasticizers, pesticides, herbicides, pollutants, and heavy metals. Aromatase is a rate-limiting enzyme in the conversion of androgens to estrogens, and letrozole is a selective aromatase inhibitor (55). Letrozole has been used to treat McCune–Albright syndrome, which has been associated with precocious puberty in girls (56) and delayed growth and puberty in boys (57). Further, fluoxetine is drug that is commonly used to treat adults with depressive disorders (58). The effect of fluoxetine on puberty has been a controversial issue. The results of one study demonstrated that fluoxetine exposure in utero delays puberty onset in female rats (59), whereas other data suggested that fluoxetine exposure during gestation did not alter plasma estrogen concentrations in peripubertal offspring (60).
Some plant extracts act as EEDs, as they are among a group of secondary metabolites with chemical structures similar to those of endogenous hormones. Isoflavones are phytoestrogens that are mainly produced in soybeans and can promote advancement of the vaginal opening in female rats after exogenous supplementation (61). Galangin is a naturally occurring flavonoid that inhibits the effects of flavonoids on human cytochrome P450 (62). Galangin was found to inhibit the aryl hydrocarbon receptor and is considered a potential drug for treating breast cancer (63, 64). Notable health benefits have also been reported for galangin (65), and previous data suggest that galangin is useful for treating precocious puberty. Zearalenone (ZEN) is a non-steroidal mycoestrogen that can exert adverse endocrine effects in mammals (66). To date, there is little agreement regarding the effect of ZEN on puberty. One group found that ZEN might trigger central precocious puberty (CPP) development in girls (67), whereas another group found an association between ZEN and normal pubertal development in adolescent girls (68).
A growing body of literature has associated being overweight or obese with early puberty (69); overweight and obese girls undergo menarche earlier than normal-weight girls (70). Several cross-sectional data have suggested that a substantial proportion of girls with CPP have hypertriglyceridemia (71). Cholesterol is an endogenous ligand of estrogen-related receptor alpha (72) that plays a role in activating estrogen receptors (73). Based on these data, we can infer that a high-cholesterol diet may also be a risk factor for precocious puberty. Plasticizers are additives used to produce or promote plasticity and flexibility in plastics, which are commonly used in everyday life. Further, parabens have been added to personal care products as antimicrobial preservatives (74), and methylparaben exposure increases glandular tissue sizes during critical developmental windows (75). A large survey showed that elevated phthalate metabolite levels pose potentially high health risks to children (76). In addition, monobutyl phthalate exposure might be associated with a risk for sexual precocity in girls (77). Animal experiments have demonstrated that two alkylphenols (4-nonylphenol and 4-tert-octylphenol) modify hormone biosynthesis and delay the onset of puberty (78).
This study has some limitations. First, the pooled GWAS data were obtained from the United Kingdom Biobank, and the study subjects were predominantly from European populations; these aspects affect the extrapolation of the results. Therefore, our results should be used cautiously when studying AAM in other populations. Second, although some of the genes we screened have been confirmed in other studies, our enrichment analysis used genes that did not pass correction for multiple testing (P <0.05), which may introduce some false positives so it requires caution when using our results. And some genes related to AAM susceptibility identified here have not been verified via molecular biology experiments, which should be performed in future studies. Further, some EEDs identified in this study were previously demonstrated to play a role in AAM, whereas others are not yet validated, which will require more clinical observations and cohort studies. However, to the best of our knowledge, this is the first large study using CGSEA to identify candidate EEDs related to AAM. Our TWAS analysis detected AAM-associated genes at the DNA level, and our CGSEA extended the classic GSEA approach to detect associations between environmental chemicals and AAM.
Conclusion
In this study, we aimed to determine the influences of genetic and environmental factors on AAM. Therefore, we performed a TWAS and CGSEA related to AAM and identified multiple AAM-associated genes and EEDs. The results of this study expand our understanding of the genetic and environmental factors affecting the timing of female puberty.
Data Availability Statement
The original contributions presented in the study are included in the article/Supplementary Material. Further inquiries can be directed to the corresponding author.
Author Contributions
Author ML and RF collected and processed the data, as well as wrote this article. YQ and HD provided language help and writing assistance. BL proofreaded the article. CY and YX designed the study. All authors contributed to the article and approved the submitted version.
Conflict of Interest
The authors declare that the research was conducted in the absence of any commercial or financial relationships that could be construed as a potential conflict of interest.
Publisher’s Note
All claims expressed in this article are solely those of the authors and do not necessarily represent those of their affiliated organizations, or those of the publisher, the editors and the reviewers. Any product that may be evaluated in this article, or claim that may be made by its manufacturer, is not guaranteed or endorsed by the publisher.
Acknowledgments
We are indebted to all individuals who participated in, or helped with, our research.
Supplementary Material
The Supplementary Material for this article can be found online at: https://www.frontiersin.org/articles/10.3389/fendo.2022.836527/full#supplementary-material
References
1. Herting MM, Sowell ER. Puberty and Structural Brain Development in Humans. Front Neuroendocrinol (2017) 44:122–37. doi: 10.1016/j.yfrne.2016.12.003
2. Villamor E, Jansen EC. Nutritional Determinants of the Timing of Puberty. Annu Rev Public Health (2016) 37:33–46. doi: 10.1146/annurev-publhealth-031914-122606
3. Dvornyk V, Waqar ul H. Genetics of Age at Menarche: A Systematic Review. Hum Reprod Update (2012) 18(2):198–210. doi: 10.1093/humupd/dmr050
4. Avendano MS, Vazquez MJ, Tena-Sempere M. Disentangling Puberty: Novel Neuroendocrine Pathways and Mechanisms for the Control of Mammalian Puberty. Hum Reprod Update (2017) 23(6):737–63. doi: 10.1093/humupd/dmx025
5. Marks KJ, Howards PP, Smarr MM, Flanders WD, Northstone K, Daniel JH, et al. Prenatal Exposure to Mixtures of Persistent Endocrine Disrupting Chemicals and Early Menarche in a Population-Based Cohort of British Girls. Environ Pollut (2021) 276:116705. doi: 10.1016/j.envpol.2021.116705
6. Lopez-Rodriguez D, Franssen D, Bakker J, Lomniczi A, Parent AS. Cellular and Molecular Features of EDC Exposure: Consequences for the GnRH Network. Nat Rev Endocrinol (2021) 17(2):83–96. doi: 10.1038/s41574-020-00436-3
7. La Merrill MA, Vandenberg LN, Smith MT, Goodson W, Browne P, Patisaul HB, et al. Consensus on the Key Characteristics of Endocrine-Disrupting Chemicals as a Basis for Hazard Identification. Nat Rev Endocrinol (2020) 16(1):45–57. doi: 10.1038/s41574-019-0273-8
8. Oskar S, Wolff MS, Teitelbaum SL, Stingone JA. Identifying Environmental Exposure Profiles Associated With Timing of Menarche: A Two-Step Machine Learning Approach to Examine Multiple Environmental Exposures. Environ Res (2021) 195:110524. doi: 10.1016/j.envres.2020.110524
9. Lopez-Rodriguez D, Aylwin CF, Delli V, Sevrin E, Campanile M, Martin M, et al. Multi- and Transgenerational Outcomes of an Exposure to a Mixture of Endocrine-Disrupting Chemicals (EDCs) on Puberty and Maternal Behavior in the Female Rat. Environ Health Perspect (2021) 129(8):87003. doi: 10.1289/EHP8795
10. Wu C, Tan S, Liu L, Cheng S, Li P, Li W, et al. Transcriptome-Wide Association Study Identifies Susceptibility Genes for Rheumatoid Arthritis. Arthritis Res Ther (2021) 23(1):38. doi: 10.1186/s13075-021-02419-9
11. Lin WD, Cheng CF, Wang CH, Liang WM, Chen CH, Hsieh AR, et al. Genetic Factors of Idiopathic Central Precocious Puberty and Their Polygenic Risk in Early Puberty. Eur J Endocrinol (2021) 185(4):441–51. doi: 10.1530/EJE-21-0424
12. Albert FW, Kruglyak L. The Role of Regulatory Variation in Complex Traits and Disease. Nat Rev Genet (2015) 16(4):197–212. doi: 10.1038/nrg3891
13. Gusev A, Ko A, Shi H, Bhatia G, Chung W, Penninx BW, et al. Integrative Approaches for Large-Scale Transcriptome-Wide Association Studies. Nat Genet (2016) 48(3):245–52. doi: 10.1038/ng.3506
14. Shi J, Wu L, Li B, Lu Y, Guo X, Cai Q, et al. Transcriptome-Wide Association Study Identifies Susceptibility Loci and Genes for Age at Natural Menopause. Reprod Sci (2019) 26(4):496–502. doi: 10.1177/1933719118776788
15. Abreu AP, Kaiser UB. Pubertal Development and Regulation. Lancet Diabetes Endocrinol (2016) 4(3):254–64. doi: 10.1016/S2213-8587(15)00418-0
16. Day FR, Thompson DJ, Helgason H, Chasman DI, Finucane H, Sulem P, et al. Genomic Analyses Identify Hundreds of Variants Associated With Age at Menarche and Support a Role for Puberty Timing in Cancer Risk. Nat Genet (2017) 49(6):834–41.
17. Zhang Y, Quick C, Yu K, Barbeira A, Consortium GT, Luca F, et al. PTWAS: Investigating Tissue-Relevant Causal Molecular Mechanisms of Complex Traits Using Probabilistic TWAS Analysis. Genome Biol (2020) 21(1):232. doi: 10.1186/s13059-020-02026-y
18. Zhou X, Carbonetto P, Stephens M. Polygenic Modeling With Bayesian Sparse Linear Mixed Models. PLoS Genet (2013) 9(2):e1003264. doi: 10.1371/journal.pgen.1003264
19. Kanehisa M, Goto S. KEGG: Kyoto Encyclopedia of Genes and Genomes. Nucleic Acids Res (2000) 28(1):27–30. doi: 10.1093/nar/28.1.27
20. Hill DP, Blake JA, Richardson JE, Ringwald M. Extension and Integration of the Gene Ontology (GO): Combining GO Vocabularies With External Vocabularies. Genome Res (2002) 12(12):1982–91. doi: 10.1101/gr.580102
21. Jensen LJ, Kuhn M, Stark M, Chaffron S, Creevey C, Muller J, et al. STRING 8–a Global View on Proteins and Their Functional Interactions in 630 Organisms. Nucleic Acids Res (2009) 37(Database issue):D412–6. doi: 10.1093/nar/gkn760
22. Shannon P, Markiel A, Ozier O, Baliga NS, Wang JT, Ramage D, et al. Cytoscape: A Software Environment for Integrated Models of Biomolecular Interaction Networks. Genome Res (2003) 13(11):2498–504. doi: 10.1101/gr.1239303
23. Bader GD, Hogue CW. An Automated Method for Finding Molecular Complexes in Large Protein Interaction Networks. BMC Bioinf (2003) 4:2. doi: 10.1186/1471-2105-4-2
24. Mattingly CJ, Colby GT, Rosenstein MC, Forrest JN Jr, Boyer JL. Promoting Comparative Molecular Studies in Environmental Health Research: An Overview of the Comparative Toxicogenomics Database (CTD). Pharmacogenomics J (2004) 4(1):5–8. doi: 10.1038/sj.tpj.6500225
25. Cheng S, Ma M, Zhang L, Liu L, Cheng B, Qi X, et al. CGSEA: A Flexible Tool for Evaluating the Associations of Chemicals With Complex Diseases. G3 (Bethesda) (2020) 10(3):945–9. doi: 10.1534/g3.119.400945
26. Wang K, Li M, Bucan M. Pathway-Based Approaches for Analysis of Genomewide Association Studies. Am J Hum Genet (2007) 81(6):1278–83. doi: 10.1086/522374
27. Mooney MA, Wilmot B. Gene Set Analysis: A Step-by-Step Guide. Am J Med Genet B Neuropsychiatr Genet (2015) 168(7):517–27. doi: 10.1002/ajmg.b.32328
28. Ziyatdinov A, Vázquez-Santiago M, Brunel H, Martinez-Perez A, Aschard H, Soria JM. Lme4qtl: Linear Mixed Models With Flexible Covariance Structure for Genetic Studies of Related Individuals. BMC Bioinf (2018) 19(1):68. doi: 10.1186/s12859-018-2057-x
29. Stamou MI, Balasubramanian R. Hypothalamic Ceramides and the Ovarian Sympathetic System: At the Crossroads of Obesity and Puberty. Cell Metab (2021) 33(1):6–8. doi: 10.1016/j.cmet.2020.11.012
30. Gracia-Tabuenca Z, Moreno MB, Barrios FA, Alcauter S. Development of the Brain Functional Connectome Follows Puberty-Dependent Nonlinear Trajectories. Neuroimage (2021) 229:117769. doi: 10.1016/j.neuroimage.2021.117769
31. Navarro VM. Metabolic Regulation of Kisspeptin - the Link Between Energy Balance and Reproduction. Nat Rev Endocrinol (2020) 16(8):407–20. doi: 10.1038/s41574-020-0363-7
32. Perry JR, Day F, Elks CE, Sulem P, Thompson DJ, Ferreira T, et al. Parent-Of-Origin-Specific Allelic Associations Among 106 Genomic Loci for Age at Menarche. Nature (2014) 514(7520):92–7.
33. Cousminer DL, Berry DJ, Timpson NJ, Ang W, Thiering E, Byrne EM, et al. Genome-Wide Association and Longitudinal Analyses Reveal Genetic Loci Linking Pubertal Height Growth, Pubertal Timing and Childhood Adiposity. Hum Mol Genet (2013) 22(13):2735–47. doi: 10.1093/hmg/ddt104
34. Elks CE, Perry JRB, Sulem P, Chasman DI, Franceschini N, He C, et al. Thirty New Loci for Age at Menarche Identified by a Meta-Analysis of Genome-Wide Association Studies. Nat Genet (2010) 42(12):1077–85.
35. Mancuso N, Shi H, Goddard P, Kichaev G, Gusev A, Pasaniuc B. Integrating Gene Expression With Summary Association Statistics to Identify Genes Associated With 30 Complex Traits. Am J Hum Genet (2017) 100(3):473–87. doi: 10.1016/j.ajhg.2017.01.031
36. Cheng TS, Day FR, Perry JRB, Luan J, Langenberg C, Forouhi NG, et al. Prepubertal Dietary and Plasma Phospholipid Fatty Acids Related to Puberty Timing: Longitudinal Cohort and Mendelian Randomization Analyses. Nutrients (2021) 13(6). doi: 10.3390/nu13061868
37. Kemiläinen H, Adam M, Mäki-Jouppila J, Damdimopoulou P, Damdimopoulos AE, Kere J, et al. The Hydroxysteroid (17β) Dehydrogenase Family Gene HSD17B12 Is Involved in the Prostaglandin Synthesis Pathway, the Ovarian Function, and Regulation of Fertility. Endocrinology (2016) 157(10):3719–30. doi: 10.1210/en.2016-1252
38. Heikelä H, Ruohonen ST, Adam M, Viitanen R, Liljenbäck H, Eskola O, et al. Hydroxysteroid (17β) Dehydrogenase 12 is Essential for Metabolic Homeostasis in Adult Mice. Am J Physiol Endocrinol Metab (2020) 319(3):E494–e508. doi: 10.1152/ajpendo.00042.2020
39. Heras V, Castellano JM, Fernandois D, Velasco I, Rodríguez-Vazquez E, Roa J, et al. Central Ceramide Signaling Mediates Obesity-Induced Precocious Puberty. Cell Metab (2020) 32(6):951–966.e8. doi: 10.1016/j.cmet.2020.10.001
40. Hevir-Kene N, Rižner TL. The Endometrial Cancer Cell Lines Ishikawa and HEC-1A, and the Control Cell Line HIEEC, Differ in Expression of Estrogen Biosynthetic and Metabolic Genes, and in Androstenedione and Estrone-Sulfate Metabolism. Chem Biol Interact (2015) 234:309–19. doi: 10.1016/j.cbi.2014.11.015
41. Szajnik M, Szczepanski MJ, Elishaev E, Visus C, Lenzner D, Zabel M, et al. 17β Hydroxysteroid Dehydrogenase Type 12 (HSD17B12) is a Marker of Poor Prognosis in Ovarian Carcinoma. Gynecol Oncol (2012) 127(3):587–94. doi: 10.1016/j.ygyno.2012.08.010
42. Haynes BP, Straume AH, Geisler J, A'Hern R, Helle H, Smith IE, et al. Intratumoral Estrogen Disposition in Breast Cancer. Clin Cancer Res (2010) 16(6):1790–801. doi: 10.1158/1078-0432.CCR-09-2481
43. Conduit SE, Davies EM, Fulcher AJ, Oorschot V, Mitchell CA. Superresolution Microscopy Reveals Distinct Phosphoinositide Subdomains Within the Cilia Transition Zone. Front Cell Dev Biol (2021) 9:634649. doi: 10.3389/fcell.2021.634649
44. Guo J, Otis JM, Suciu SK, Catalano C, Xing L, Constable S, et al. Primary Cilia Signaling Promotes Axonal Tract Development and Is Disrupted in Joubert Syndrome-Related Disorders Models. Dev Cell (2019) 51(6):759–74.e5. doi: 10.1016/j.devcel.2019.11.005
45. Koemeter-Cox AI, Sherwood TW, Green JA, Steiner RA, Berbari NF, Yoder BK, et al. Primary Cilia Enhance Kisspeptin Receptor Signaling on Gonadotropin-Releasing Hormone Neurons. Proc Natl Acad Sci U S A (2014) 111(28):10335–40. doi: 10.1073/pnas.1403286111
46. Rodolosse A, Solymosi N, Liposits Z. Altered Expression of Genes Encoding Neurotransmitter Receptors in GnRH Neurons of Proestrous Mice. Front Cell Neurosci (2016) 10:230. doi: 10.3389/fncel.2016.00230
47. Kondratov RV, Antoch MP. Circadian Proteins in the Regulation of Cell Cycle and Genotoxic Stress Responses. Trends Cell Biol (2007) 17(7):311–7. doi: 10.1016/j.tcb.2007.07.001
48. Basili D, Gioacchini G, Todisco V, Candelma M, Marisaldi L, Pappalardo L, et al. Opsins and Gonadal Circadian Rhythm in the Swordfish (Xiphias Gladius) Ovary: Their Potential Roles in Puberty and Reproductive Seasonality. Gen Comp Endocrinol (2021) 303:113707. doi: 10.1016/j.ygcen.2020.113707
49. Hagenauer MH, Lee TM. The Neuroendocrine Control of the Circadian System: Adolescent Chronotype. Front Neuroendocrinol (2012) 33(3):211–29. doi: 10.1016/j.yfrne.2012.04.003
50. Yang C, Ran Z, Liu G, Hou R, He C, Liu Q, et al. Melatonin Administration Accelerates Puberty Onset in Mice by Promoting FSH Synthesis. Molecules (2021) 26(5). doi: 10.3390/molecules26051474
51. Zhang B, Liu Y, Liu D, Yang L. Targeting Cleavage and Polyadenylation Specific Factor 1 via shRNA Inhibits Cell Proliferation in Human Ovarian Cancer. J Biosci (2017) 42(3):417–25. doi: 10.1007/s12038-017-9701-x
52. Wang L, Lang GT, Xue MZ, Yang L, Chen L, Yao L, et al. Dissecting the Heterogeneity of the Alternative Polyadenylation Profiles in Triple-Negative Breast Cancers. Theranostics (2020) 10(23):10531–47. doi: 10.7150/thno.40944
53. Desai MK, Brinton RD. Autoimmune Disease in Women: Endocrine Transition and Risk Across the Lifespan. Front Endocrinol (Lausanne) (2019) 10:265. doi: 10.3389/fendo.2019.00265
54. Noblejas-López MDM, Nieto-Jiménez C, Morcillo García S, Pérez-Peña J, Nuncia-Cantarero M, Andrés-Pretel F, et al. Expression of MHC Class I, HLA-A and HLA-B Identifies Immune-Activated Breast Tumors With Favorable Outcome. Oncoimmunology (2019) 8(10):e1629780. doi: 10.1080/2162402X.2019.1629780
55. Wit JM, Hero M, Nunez SB. Aromatase Inhibitors in Pediatrics. Nat Rev Endocrinol (2011) 8(3):135–47. doi: 10.1038/nrendo.2011.161
56. Estrada A, Boyce AM, Brillante BA, Guthrie LC, Gafni RI, Collins MT, et al. Long-Term Outcomes of Letrozole Treatment for Precocious Puberty in Girls With McCune-Albright Syndrome. Eur J Endocrinol (2016) 175(5):477–83. doi: 10.1530/EJE-16-0526
57. Kohva E, Varimo T, Huopio H, Tenhola S, Voutilainen R, Toppari J, et al. Anti-Müllerian Hormone and Letrozole Levels in Boys With Constitutional Delay of Growth and Puberty Treated With Letrozole or Testosterone. Hum Reprod (2020) 35(2):257–64. doi: 10.1093/humrep/dez231
58. Cipriani A, Furukawa TA, Salanti G, Chaimani A, Atkinson LZ, Ogawa Y, et al. Comparative Efficacy and Acceptability of 21 Antidepressant Drugs for the Acute Treatment of Adults With Major Depressive Disorder: A Systematic Review and Network Meta-Analysis. Lancet (2018) 391(10128):1357–66. doi: 10.1016/S0140-6736(17)32802-7
59. Dos Santos AH, Vieira ML, de Azevedo Camin N, Anselmo-Franci JA, Ceravolo GS, Pelosi GG, et al. In Utero and Lactational Exposure to Fluoxetine Delays Puberty Onset in Female Rats Offspring. Reprod Toxicol (2016) 62:1–8. doi: 10.1016/j.reprotox.2016.04.006
60. Barbosa MA, Veríssimo LF, Gerardin DCC, Pelosi GG, Ceravolo GS, Moreira EG. Maternal Exposure to Fluoxetine During Gestation and Lactation Does Not Alter Plasma Concentrations of Testosterone, Oestrogen or Corticosterone in Peripubertal Offspring. Reprod Fertil Dev (2019) 31(5):1002–8. doi: 10.1071/RD18279
61. Sleiman HK, de Oliveira JM, Langoni de Freitas GB. Isoflavones Alter Male and Female Fertility in Different Development Windows. BioMed Pharmacother (2021) 140:111448. doi: 10.1016/j.biopha.2021.111448
62. Kao YC, Zhou C, Sherman M, Laughton CA, Chen S. Molecular Basis of the Inhibition of Human Aromatase (Estrogen Synthetase) by Flavone and Isoflavone Phytoestrogens: A Site-Directed Mutagenesis Study. Environ Health Perspect (1998) 106(2):85–92. doi: 10.1289/ehp.9810685
63. Murray TJ, Yang X, Sherr DH. Growth of a Human Mammary Tumor Cell Line is Blocked by Galangin, a Naturally Occurring Bioflavonoid, and is Accompanied by Down-Regulation of Cyclins D3, E and A. Breast Cancer Res (2006) 8(2):R17. doi: 10.1186/bcr1391
64. Donovan MG, Selmin OI, Doetschman TC, Romagnolo DF. Epigenetic Activation of BRCA1 by Genistein In Vivo and Triple Negative Breast Cancer Cells Linked to Antagonism Toward Aryl Hydrocarbon Receptor. Nutrients (2019) 11(11). doi: 10.3390/nu11112559
65. Xuan H, Ou A, Hao S, Shi J, Jin X. Galangin Protects Against Symptoms of Dextran Sodium Sulfate-Induced Acute Colitis by Activating Autophagy and Modulating the Gut Microbiota. Nutrients (2020) 12(2). doi: 10.3390/nu12020347
66. Ali N, Degen GH. Urinary Biomarkers of Exposure to the Mycoestrogen Zearalenone and its Modified Forms in German Adults. Arch Toxicol (2018) 92(8):2691–700. doi: 10.1007/s00204-018-2261-5
67. Massart F, Meucci V, Saggese G, Soldani G. High Growth Rate of Girls With Precocious Puberty Exposed to Estrogenic Mycotoxins. J Pediatr (2008) 152(5):690–5, 695.e1. doi: 10.1016/j.jpeds.2007.10.020
68. Rivera-Núñez Z, Barrett ES, Szamreta EA, Shapses SA, Qin B, Lin Y, et al. Urinary Mycoestrogens and Age and Height at Menarche in New Jersey Girls. Environ Health (2019) 18(1):24. doi: 10.1186/s12940-019-0464-8
69. Brix N, Ernst A, Lauridsen LLB, Parner ET, Arah OA, Olsen J, et al. Childhood Overweight and Obesity and Timing of Puberty in Boys and Girls: Cohort and Sibling-Matched Analyses. Int J Epidemiol (2020) 49(3):834–44. doi: 10.1093/ije/dyaa056
70. Ortega MT, McGrath JA, Carlson L, Flores Poccia V, Larson G, Douglas C, et al. Longitudinal Investigation of Pubertal Milestones and Hormones as a Function of Body Fat in Girls. J Clin Endocrinol Metab (2021) 106(6):1668–83. doi: 10.1210/clinem/dgab092
71. Zurita-Cruz JN, Villasís-Keever MA, Manuel-Apolinar L, Damasio-Santana L, Gutierrez-Gonzalez A, Wakida-Kusunoki G, et al. Altered Cardiometabolic Profile in Girls With Central Precocious Puberty and Adipokines: A Propensity Score Matching Analysis. Cytokine (2021) 148:155660. doi: 10.1016/j.cyto.2021.155660
72. Wei W, Schwaid AG, Wang X, Wang X, Chen S, Chu Q, et al. Ligand Activation of Errα by Cholesterol Mediates Statin and Bisphosphonate Effects. Cell Metab (2016) 23(3):479–91. doi: 10.1016/j.cmet.2015.12.010
73. Li D, Cai Y, Teng D, Li W, Tang Y, Liu G. Computational Insights Into the Interaction Mechanisms of Estrogen-Related Receptor Alpha With Endogenous Ligand Cholesterol. Chem Biol Drug Des (2019) 94(1):1316–29. doi: 10.1111/cbdd.13506
74. Jensen TK, Andersson AM, Main KM, Johannsen TH, Andersen MS, Kyhl HB, et al. Prenatal Paraben Exposure and Anogenital Distance and Reproductive Hormones During Mini-Puberty: A Study From the Odense Child Cohort. Sci Total Environ (2021) 769:145119. doi: 10.1016/j.scitotenv.2021.145119
75. Gopalakrishnan K, Teitelbaum SL, Lambertini L, Wetmur J, Manservisi F, Falcioni L, et al. Changes in Mammary Histology and Transcriptome Profiles by Low-Dose Exposure to Environmental Phenols at Critical Windows of Development. Environ Res (2017) 152:233–43. doi: 10.1016/j.envres.2016.10.021
76. Yao Y, Chen D, Wu Y, Zhou L, Cheng J, Li Y, et al. Urinary Phthalate Metabolites in Primary School Starters in Pearl River Delta, China: Occurrences, Risks and Possible Sources. Environ Res (2019) 179(Pt B):108853. doi: 10.1016/j.envres.2019.108853
77. Wen Y, Liu SD, Lei X, Ling YS, Luo Y, Liu Q, et al. Association of PAEs With Precocious Puberty in Children: A Systematic Review and Meta-Analysis. Int J Environ Res Public Health (2015) 12(12):15254–68. doi: 10.3390/ijerph121214974
Keywords: menarche, puberty, environmental endocrine disruptor, GWAS, TWAS, CGSEA
Citation: Lu M, Feng R, Qin Y, Deng H, Lian B, Yin C and Xiao Y (2022) Identifying Environmental Endocrine Disruptors Associated With the Age at Menarche by Integrating a Transcriptome-Wide Association Study With Chemical-Gene-Interaction Analysis. Front. Endocrinol. 13:836527. doi: 10.3389/fendo.2022.836527
Received: 15 December 2021; Accepted: 03 February 2022;
Published: 24 February 2022.
Edited by:
Renata Marino Romano, State University of Midwest Paraná, BrazilReviewed by:
Sally Mortlock, The University of Queensland, AustraliaMaochun Wang, The Affiliated Hospital of Nanjing University Medical School, China
Paula Bargi-Souza, Federal University of Minas Gerais, Brazil
Copyright © 2022 Lu, Feng, Qin, Deng, Lian, Yin and Xiao. This is an open-access article distributed under the terms of the Creative Commons Attribution License (CC BY). The use, distribution or reproduction in other forums is permitted, provided the original author(s) and the copyright owner(s) are credited and that the original publication in this journal is cited, in accordance with accepted academic practice. No use, distribution or reproduction is permitted which does not comply with these terms.
*Correspondence: Yanfeng Xiao, eGlhb3lhbmZlbmdncm91cEBzaW5hLmNvbQ==; Chunyan Yin, Mjg2ODk2MTczQHFxLmNvbQ==
†These authors share senior authorship