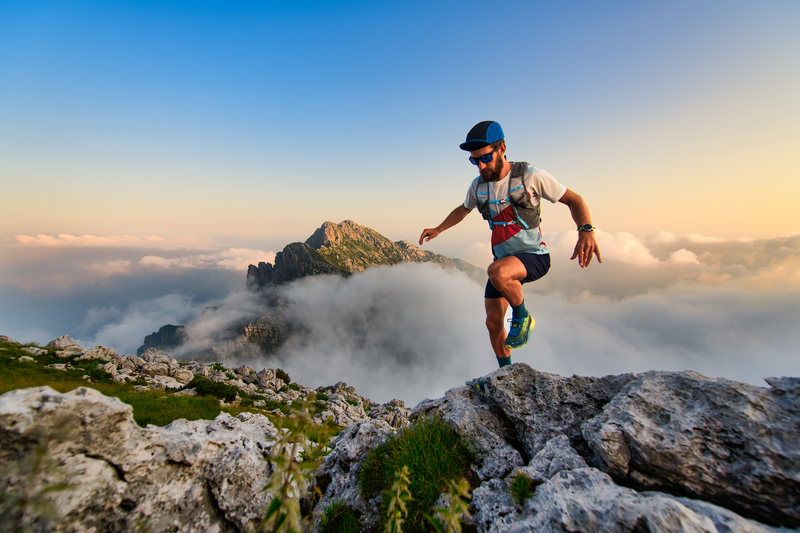
94% of researchers rate our articles as excellent or good
Learn more about the work of our research integrity team to safeguard the quality of each article we publish.
Find out more
ORIGINAL RESEARCH article
Front. Endocrinol. , 28 February 2022
Sec. Reproduction
Volume 13 - 2022 | https://doi.org/10.3389/fendo.2022.825934
This article is part of the Research Topic Omics in Biology of Reproduction View all 7 articles
The outcomes of in vitro fertilization (IVF) for endometriotic women are significantly worse than for patients without ovarian endometriosis (OEM), as shown by fewer retrieved oocytes. However, the exact pathophysiological mechanism is still unknown. Thus, we conducted a prospective study that analyzed mRNA and lncRNA transcriptome between granulosa cells (GCs) from patients with fewer retrieved oocytes due to OEM and GCs from controls with male factor (MF) infertility using an RNA sequencing approach. We found a group of significantly differentially expressed genes (DEGs), including NR5A2, MAP3K5, PGRMC2, PRKAR2A, DEPTOR, ITGAV, KPNB1, GPC6, EIF3A, and SMC5, which were validated to be upregulated and negatively correlated with retrieved oocyte numbers in GCs of patients with OEM, while DUSP1 demonstrated the opposite. The molecular functions of these DEGs were mainly enriched in pathways involving mitogen-activated protein kinase (MAPK) signaling, Wnt signaling, steroid hormone response, apoptosis, and cell junction. Furthermore, we performed lncRNA analysis and identified a group of differentially expressed known/novel lncRNAs that were co-expressed with the validated DEGs and correlated with retrieved oocyte numbers. Co-expression networks were constructed between the DEGs and known/novel lncRNAs. These distinctive molecular signatures uncovered in this study are involved in the pathological regulation of ovarian reserve dysfunction in OEM patients.
Endometriosis is defined as the presence of endometrial-like tissue outside of the uterine cavity, commonly proliferating onto the peritoneum and abdominal organs. This causes chronic inflammation with the formation of adhesions and is associated with pelvic pain and infertility (1, 2). Ovarian endometriosis (endometrioma, OEM), as one subtype of endometriosis, is formed by an intraovarian hematoma surrounded by ovarian cortex caused by recurrent ectopic endometrial hemorrhages and is present in up to 17%–44% of patients with endometriosis (3–5). Repeated and periodic bleeding of endometriotic lesions causes severe fibrosis and adhesions around the endometriotic cysts, leading to the destruction of the normal ovarian structure and reduction in the ovarian reserve (6).
In vitro fertilization (IVF) is recommended as an effective approach for infertility associated with endometriosis (7). However, the outcomes of IVF cycles for endometriotic women are significantly worse than for patients without this condition, as shown by the reduced numbers of oocytes retrieved (8–10) and attenuated oocyte quality (11, 12). Fewer mature follicles and retrieved oocytes reflect impaired folliculogenesis, which is the primary dilemma for women with OEM in attempting to achieve pregnancy. However, the exact pathophysiological mechanism of endometrioma related to lower numbers of retrieved oocytes is still unknown.
The GCs play an important role in regulating the development, maturation, and function of thecal cells and oocytes via signal transduction or direct cell-to-cell interactions, eventually affecting the whole process of follicle development, maturation, and atresia. Long noncoding RNAs (lncRNAs) are defined as transcripts that are longer than 200 nucleotides without significant protein coding potential (13, 14). The lncRNA and messenger RNAs (mRNAs) expression patterns were studied between eutopic and normal endometrium in the proliferative phase of OEM patients, which provided new resources for EM diagnosis and treatment (15–18). However, further analysis of mRNA and lncRNA profile and regulatory mechanism at GCs level has not been reported, nor has it been associated with infertility and IVF outcomes.
RNA sequencing (RNA-seq) allows the investigation of transcriptomes, including mRNA and lncRNA, at unsurpassed resolution of biological processes. RNA-seq analysis from oocytes and GCs during the stepwise follicular development reveals the transcriptomic landscape of folliculogenesis (19). To characterize the cellular heterogeneity of GCs and provide insights into the complex transcriptomes of GCs from patients with OEM, here, we aimed to identify the mRNA and lncRNA transcriptional regulation landscape of GCs from patients with OEM using an RNA-seq approach.
GCs collected from infertile women due to OEM were put in the OEM group, while GCs from women in couples with male factor (MF) infertility were regarded as control. The clinical characteristics of GC samples for RNA-seq, detailed in Table 1, showed that the only significantly different parameter between the two groups was the number of retrieved oocytes (p < 0.05).
The quality control statistics for the RNA-sequencing is presented in Supplementary Table S1. The RNA quality detection data of GCs for RNA-seq samples are presented in Supplementary Table S2 and Supplementary Figures S1A–C. The expression of cell-type-specific markers for GCs is shown in Supplementary Figures S1D–O. There were 15,890 mRNAs detected in total, of which 459 significantly DEGs (396 upregulated and 63 downregulated; Figure 1A and Supplementary Table S3) were found in the GCs by comparing the two groups. Z-score normalized fragments per kilobase of transcript per million mapped reads (FPKM) of differentially expressed genes were used as inputs for two-dimensional principal component analysis (PCA) by using R package Stats (Figure 1B). There was a clear separation between the distribution of the GC mRNA datasets from the OEM and MF group (p < 0.05).
Figure 1 mRNA profiling of granulosa cells (GCs) from patients with infertility associated with ovarian endometriosis (OEM). (A) The x-axis of the volcano map represents log2(fold change (FC)) value of total detected genes, while the y-axis represents their −log10(p value) value. The significant FC_up differentially expressed genes (DEGs) are presented in the upper right quadrant (red), and the significant FC_down DEGs are shown in upper left quadrant (blue). (B) Two-dimensional principal component analysis (PCA) of DEGs from the transcriptomes of RNA-seq data. The PC1 separates the two groups (MF versus OEM). The PC2 separates the samples according to the gene expression pattern. Green color indicates samples from the male factor (MF) group, and red color indicates samples from the OEM group. (C) Correlation heatmap between DEGs and the clinical characteristics of RNA-seq GC samples. The ordinate represents DEGs, and the abscissa is clinical characteristics (*p < 0.05, **p < 0.01, ***p < 0.001) (NMF = 6, NOEM = 5).
To clarify the correlation between the expression of DEGs and the clinical characteristics of samples, we constructed their correlation heatmap (Figure 1C). The results showed that most of the DEGs were significantly correlated with the number of retrieved oocytes, followed by 2PN zygote numbers, cleavage stage embryo numbers, and AMH level.
Functional enrichment analysis of these DEGs helped to identify the pathways that affected the function of GCs from patients with OEM. GO analysis demonstrated that these DEGs were mainly involved in the following terms: cell junction, MAPK cascade, oocyte maturation, responses to FSH and LH, and cell cycle (Figure 2A). By using KEGG analysis, we found that these DEGs were involved in several pathways, including adhesion junction, Wnt signaling, MAPK signaling, FoxO signaling, cAMP signaling, and estrogen signaling pathway (Figure 2B).
Figure 2 Functional enrichment analysis. (A) Gene Ontology (GO) bubble chart for DEG enrichment. (B) Kyoto Encyclopedia of Genes and Genomes (KEGG) bubble chart indicating the enrichment of DEGs. Rich factor is defined as S gene number divided by B gene number. “S” was annotated as the number of genes with significant difference in specific GO/KEGG categories; “B” was the number of genes for a specific GO/KEGG (NMF = 6, NOEM = 5).
In order to screen more potential DEGs for validation, we included the mRNA with threshold of |log2(fold change (FC))| ≥ 1, p < 0.08, and FPKM >0.8 in all samples; then, 77 mRNA were selected. Among them, 60 genes were upregulated, and 17 genes were downregulated simultaneously. To validate the transcriptomic sequencing results, genes that were previously reported related to GCs/oocyte/follicular development, genes that intersected with reported original transcriptomic sequencing data for GCs (19) and OEM-affected oocytes (20), and genes that were involved in significantly enriched GO functions of this study were selected from the significant 77 DEGs and tested on more clinical GC samples (Figure 3A and Supplementary Table S4). These included up- and downregulated genes demonstrated in Figure 3B.
Figure 3 DEGs selection for validation. (A) Purple circle represents DEGs with |log2(FC)| ≥ 1, p < 0.08 and FPKM > 0.8 in all samples from this study, yellow circle represents reported genes from the DEGs that are related to GCs/oocyte/follicular development, green circle represents genes from the DEGs are intersected with reported original transcriptomic sequencing data of GCs and OEM-affected oocytes. (B) The log2(FC) value of selected DEGs for validation (NMF = 6, NOEM = 5).
The clinical characteristics of patients’ cohort for validation are listed in Table 2. The average AMH, basal antral follicle count, retrieved oocytes number, growing follicle number, 2PN zygote number, cleavage stage embryo number, high qualified embryo number, and 2PN fertilization rates of OEM group were also significantly lower than those in MF group, while basal FSH level was also higher. These clinical manifestations are consistent with the phenomenon that OEM leads to the ovarian reserve dysfunction and compromised oocyte quality.
The quantitative PCR (qPCR) results of GCs for validation showed that the relative expressions of NR5A2 (Figure 4A), MAP3K5 (Figure 4B), PGRMC2 (Figure 4C), DEPTOR (Figure 4D), ITGAV (Figure 4E), KPNB1 (Figure 4F), PRKAR2A (Figure 4G), GPC6 (Figure 4H), EIF3A (Figure 4I), and SMC5 (Figure 4J) were indeed significantly higher in OEM group when compared with the MF group, while DUSP1 (Figure 4K) was lower expressed in OEM group.
Figure 4 OEM-associated DEGs validation and correlation analysis with retrieved oocyte numbers. (A–K) Validation of selected DEGs by expanding clinical samples through real-time quantitative polymerase chain reaction (qPCR) amplification demonstrate significant differences between the OEM and MF groups. (N, sample size). (L–U) Pearson correlation analyses between relative expression levels of the validated DEGs and the retrieved oocyte numbers (r, Pearson correlation coefficient). (*p < 0.05, **p < 0.01, ***p < 0.001).
We analyzed the correlations of the qPCR value with the IVF clinical outcomes, including the numbers of retrieved oocytes, 2PN zygote, cleavage stage embryo on day 3 of culture (developed from 2PN zygotes), and growing follicles (diameter ≥ 14 mm on the day of hCG triggering), in validation cohort. Our results demonstrated that, among the validated DEGs, the number of retrieved oocytes was negatively correlated with the relative gene expression levels of NR5A2 (Figure 4L), PGRMC2 (Figure 4M), DEPTOR (Figure 4N), ITGAV (Figure 4O), KPNB1 (Figure 4P), PRKAR2A (Figure 4Q), GPC6 (Figure 4R), EIF3A (Figure 4S), and SMC5 (Figure 4T) and positively correlated with the relative expression of DUSP1 (Figure 4U). In addition, the number of 2PN zygote (Supplementary Figures S2A–J), cleavage stage embryo (Supplementary Figures S3A–J), and growing follicles (Supplementary Figures S4A–G) were correlated with the relative gene expression levels of the validated DEGs in this study.
Through RNA-seq, we detected 47,414 non-protein coding transcripts from these GC samples. Among these transcripts, there were 14,379 known lncRNA annotated in the genome, and 33,035 novel lncRNAs were predicted. Differential expression analysis demonstrated that 35 known lncRNAs were significantly upregulated including PTPRG-AS1, LINC01278, MEG8, LERFS, PRKCQ-AS1, and SNHG17, and 24 known lncRNAs were significantly downregulated including MALAT1, FTX, BDNF-AS, DANCR, and GASAL1 (Figure 5A and Supplementary Table S3). The correlation heatmap showed that most of the differentially expressed known lncRNA were also related to the number of retrieved oocytes, followed by 2PN zygote numbers and cleavage stage embryo numbers (Figure 5B). Interestingly, the correlation network diagram showed that most of significantly differentially expressed known lncRNAs were negatively correlated with these clinical indicators. For example, MRPL20-AS1 and MIF-AS1 were all negatively correlated with the number of retrieved oocytes, 2PN zygote numbers, and cleavage stage embryo numbers (Figure 5C).
Figure 5 LncRNA profiling of GCs from patients with infertility associated with OEM. (A) Volcano map analysis of differentially expressed known lncRNA indicates significantly upregulated lncRNAs (red), downregulated significantly lncRNAs (blue), and non-significant lncRNAs (gray). (B) Correlation heatmap between differentially expressed known lncRNA and the clinical characteristics of RNA-seq samples. The ordinate represents known lncRNA, and the abscissa is clinical characteristics. (C) Co-expression network of clinical characteristics and known lncRNAs through Weighted Gene Co-expression Network Analysis (WGCNA); Cytoscape was used to visualize the network diagram (|correlation coefficient| > 0.5, p < 0.05). (D) Volcano map analysis of differentially expressed novel lncRNA. (E) Correlation heatmap between differentially expressed novel lncRNA and the clinical characteristics of RNA-seq samples. (F) Co-expression network of clinical characteristics and novel lncRNAs through WGCNA; Cytoscape was used to visualize the network diagram (|correlation coefficient| > 0.5, p < 0.05) (*p < 0.05, **p < 0.01, ***p < 0.001) (NMF = 6, NOEM = 5).
For the predicted novel lncRNA, 360 of them were significantly upregulated, and 51 were significantly downregulated (Figure 5D and Supplementary Table S3). The correlation heatmap also showed that most of the differentially expressed novel lncRNAs were also related to the number of retrieved oocytes, followed by 2PN zygote numbers and cleavage stage embryo numbers (Figure 5E). Meanwhile, through screening, in the novel lncRNA whose average FPKM of all samples was ≥1, we found that most of these lncRNA were negatively correlated with these clinical characteristics. Novel lncRNA, such as MSTRG.47475.1, MSTRG.51840.1, MSTRG.47454.1, and MSTRG.14101.4 were all negatively expressed with the number of retrieved oocytes, 2PN zygote numbers, and cleavage stage embryo numbers (Figure 5F).
Establishing mRNA–lncRNA regulatory networks is useful for revealing interactions between mRNAs and co-expressed lncRNAs. We identified 210 significant mRNA–lncRNA(known) groups with correlation coefficient threshold |abs_rho| > 0.5 and p < 0.05. Most of them were (208/210) positively associated, and only two of the groups were negatively associated. The validated DEGs, including NR5A2, MAP3K5, PGRMC2, ITGAV, KPNB1, PRKAR2A, GPC6, EIF3A, and SMC5 co-expressed with known lncRNAs under the correlation coefficient threshold |abs_rho| > 0.9 and p < 0.05 (Figure 6A). Among them, EIF3A, PGRMC2, ITGAV, SMC5, and PRKAR2A had over 10 paired co-expressed known lncRNA (Supplementary Table S5).
Figure 6 Co-expression analysis of mRNA and lncRNA. (A) Co-expression network from validated DEGs and known lncRNAs through WGCNA; Cytoscape was used to visualize the network diagram (|correlation coefficient| > 0.9, p < 0.05). (B) Co-expression network from validated DEGs and novel lncRNAs through WGCNA; Cytoscape was used to visualize the network diagram (|correlation coefficient| > 0.9, p < 0.05). (C) Venn diagram of co-expressed known lncRNAs with clinical characteristics and validated DEGs. Purple circle represents co-expressed known lncRNA with clinical characteristics, while yellow circle means co-expressed known lncRNA with all validated DEGs (|correlation coefficient| > 0.5, p < 0.05). (D) Venn diagram of co-expressed novel lncRNAs with clinical characteristics and validated DEGs. Purple circle represents co-expressed novel lncRNA with clinical characteristics, while yellow circle means co-expressed novel lncRNA with all validated DEGs (|correlation coefficient| > 0.5, p < 0.05) (NMF = 6, NOEM = 5).
Simultaneously, we identified 750 mRNA–lncRNA(novel) groups with correlation coefficient threshold |abs_rho| > 0.5 and p < 0.05 from the novel lncRNA with average FPKM value of all samples ≥ 1. Most of them were (744/750) positively associated, while only six of the groups were negatively associated. Identically, under the correlation coefficient threshold |abs_rho| > 0.9 and p < 0.05, validated DEGs including NR5A2, MAP3K5, PGRMC2, ITGAV, KPNB1, PRKAR2A, GPC6, EIF3A, and SMC5 had co-expressed novel lncRNAs, while DEPTOR and DUSP1 had not (Figure 6B). Among them, GPC6, NR5A2, EIF3A, and MAP3K5 had over 20 pairs of co-expressed novel lncRNA (Supplementary Table S5).
The Venn diagrams demonstrated that MIF-AS1, LERFS, AC123912, LINC00662, MUC20-OT1, and FP671120 were co-expressed known lncRNAs, which both correlated with clinical characteristics and validated DEGs (Figure 6C). Novel lncRNAs, such as MSTRG.15872.5, MSTRG.47475.1, MSTRG.19364.1, MSTRG.14101.4, and MSTRG.42454.1, were all associated with clinical characteristics and validated DEGs (Figure 6D).
Oocytes, granulosa cells (GC), and follicular fluid are the main components of follicles. GCs grow closely around the oocyte, constitute the microenvironment for oocyte survival, and play an important role in follicle and oocyte health (21). The direct exchanging of small molecules via gap junction channels and bidirectional paracrine signaling between oocytes and GCs are critical to synchronize follicle development and oocyte maturation, ovulation, and atresia (22). On the other hand, redundant GCs would be discarded in the process of oocyte retrieval; their acquisition is non-invasive to human body nor to oocytes. Furthermore, many studies have correlated GCs gene expression with different outcome measures such as oocyte maturity, fertilization, embryo development, implantation, and pregnancy (23–25). GCs transcriptome has also been studied to identify gamete quality (26) and explore the potential of clinical implications for the treatment of infertility (27). Therefore, we choose GCs as a noninvasive indicator of oocytes competence and ovarian function.
In the clinical characteristics of the validation cohort in Table 2, the mean age of patients in OEM group was 3 years older than that in the MF group, although the average age of both groups was below 35 years old. On the one hand, for these patients enrolled in the MF group, the female partners are generally healthy, they do not need extra surgical treatment before getting pregnant. However, for these patients with OEM, all of them underwent previous laparoscopic surgery. Thus, the time of IVF treatment is delayed. Due to the poor ovarian reserve, the number of IVF treatment cycles is much more than that of the MF group. On the other hand, the clinical indexes about ovarian reserve, such as AMH level, were dramatically decreased in OEM group, which was far more than the fecundity drops caused by advancing age from 30 to 33 years old. There are two main possible reasons for the significant decline of ovarian reserve in OEM patients, rather than age in our cohort. One is the space occupation of OEM cyst, and subsequent ovarian tissue fibrosis reduces the functional ovarian tissue (6, 28). The other is that inevitable removal of unaffected ovarian tissue during the surgical excision of endometrioma cyst wall aggravates the reduction in ovarian reserve function (3, 29). Therefore, it is the dual factors of OEM itself and laparoscopic surgery that lead to the significant decrease in ovarian reserve.
GCs act under steroid hormonal control and play key roles in folliculogenesis and corpus luteum formation after ovulation. The DEGs we identified in this study, including NR5A2 and PGRMC2, are involved in steroid hormone receptor activity and steroid hormone-mediated signaling pathways. NR5A2 plays pivotal roles in the regulation of steroidogenic enzymes (30) and is essential for progesterone synthesis (31) and luteinization (32). The high expression of NR5A2 in our study indicates the increased ability of GCs from patients with OEM to synthesize and secrete progesterone, with possible premature luteinization of follicles and follicle atresia (33) and reflecting reduced ovarian reserve (34, 35). Moreover, PGRMC2—coding for progesterone receptor membrane component 2—was reported to be involved in ovarian folliculogenesis (36–38). An increased expression of PGRMC2 in patients was reported to be associated with diminished ovarian reserve (39).
Follicular atresia is mostly indicated by GC apoptosis (33). MAP3K5, a significant DEG validated in this study, is a mediator of the MAPK pathway, also known as apoptosis signal regulating kinase 1 (ASK1) (40). MAP3K5 was reported to suppress healthy rabbit GC functions, including promoting apoptosis, inhibiting proliferation, and altering progesterone release (41). Moreover, MAP3K5 is involved in intrinsic apoptotic signaling pathway in response to oxidative stress through GO evaluation. Excessive oxidative stress is reported to occur in GCs (12, 42), and in follicular fluid (43), from patients with OEM and is associated with infertility. In addition, another validated DEG, KPNB1, interacts with MAP3K5 in response to stress (44) and plays a role in mouse oogenesis and folliculogenesis (45). The upregulated expression of MAP3K5 and KPNB1 suggests that they may be involved in the apoptosis of GCs, which is consistent with the study that GCs from patients with endometriosis show increased levels of apoptosis (46).
The delicate interactions between GCs and oocytes through cell junctions are essential for follicular development and in ensuring oocyte competence. Abnormal GC function can reflect incompetent oocytes. PRKAR2A plays an important role in the maturation of GCs (47) and also has critical functions in meiotic arrest and meiotic maturation in mammalian oocytes (48, 49). SMC5, together with SMC6, functions in DNA repair (50) and in successful meiotic divisions (51, 52). Thus, the upregulated expression levels of PRKAR2A and SMC5 in GCs from patients with OEM in this study are likely to reflect failure of meiotic resumption.
Inhibition of DUSP1 in cumulus cells caused abnormal cell cycle progression (53). RNA-seq of oocytes from OEM patients revealed that DUSP1 might be a potential biomarker to diagnose oocyte quality due to their association with decreased oocyte competence (20). In our study, DUSP1 was downregulated in OEM group, and its relative expression levels were positively correlated with retrieved oocyte number. This suggests that DUSP1 may play a role in the process of OEM affecting follicular development.
LncRNAs in GCs are not only largely involved in normal folliculogenesis, but their function and expression will change accordingly under pathological conditions (54). LncRNA MALAT1 is one of the studied lncRNAs in endometriosis. It mediates hypoxia-induced pro-survival autophagy of endometrial stromal cells in endometriosis (55), inhibits apoptosis of endometrial stromal cells by activating PI3K-AKT pathway (56), and involves in the E2-mediated epithelial–mesenchymal transition process in endometriosis (57). Our results are consistent with the reported study, which demonstrated that lncRNA MALAT1 was significantly downregulated in GCs from OEM patients (58). The downregulation of lncRNA MALAT1 in GCs may have an adverse effect on the growth and development of oocytes by inhibiting GC proliferation through MAPK pathway. Interestingly, two of these predicted upregulated novel lncRNAs, namely,
MSTRG.13411.12 and MSTRG.13411.14 are near MALAT1 locus, suggesting that MALAT1 is involved in the pathological regulation of GCs from OEM-related infertility patients.
The known lncRNA, Z83843, an autophagy-related lncRNA (59), co-expresses with NR5A2 and PGRMC2. PRKAR2A, SMC5, ITGAV, PGRMC2, and EIF3A have more than eight overlapped co-expressed known lncRNA, including MEG8, Z83843, AC092683, MUC20-OT1, FTX, SNHG5, AL136169, and LINC01275. In our study, MEG8 is one of the most significantly upregulated lncRNAs, while FTX is significantly downregulated in GCs from OEM patients. EIF3A also correlated with LINC00662 and LINC01278, which are reported to be involved in many biology functions, such as cell proliferation (60), carcinoma metastasis (61), and M2 macrophage polarization via activating Wnt/β-catenin signaling (62).
Furthermore, LINC00662 is correlated with oocyte-retrieved number and the expression of EIF3A. LINC00662 is reported to be involved in regulating oxidative stress (63); also, it plays a role in spermatogenesis and male infertility (64). MUC20-OT1 is highly correlated with 2PN zygote number and the expression of PRKAR2A, GPC6, SMC5, EIF3A, ITGAV, and PGRMC2. AC123912 is highly correlated with oocyte-retrieved number and the expression of SMC5, EIF3A, PRKAR2A, ITGAV, and PGRMC2. LERFS is a known lncRNA that is highly correlated with oocyte-retrieved number and the expression of SMC5, PRKAR2A, ITGAV, and PGRMC2. The function of these known lncRNAs were mainly studied in cancer research (62, 65). On the other hand, many of the novel lncRNAs that are associated with clinical characteristics and validated DEGs, such as MSTRG.47489.1, MSTRG.31476.1, and MSTRG.58466.1, are located near LSAMP locus, which indicates that these novel lncRNAs may play a role in transcriptional regulation by targeting at LSAMP.
This study creatively demonstrated the transcriptional regulation profile of GCs related to OEM, screened out the mRNAs and lncRNAs that correlated with the low retrieved oocyte number due to OEM, and mapped their co-expression network. It provides predictive value for oocyte competence through GC gene diagnosis. However, the limitation of this study is lacking of in-depth mechanism research of those mRNAs and lncRNAs, which deserve to be further studied on their specific biological functions in our future work. In addition, the sample size of each group for RNA-seq is <10 cases, which might inevitably lead to incompleteness of gene screening and bias of data analysis.
In conclusion, our results have given a better understanding for OEM in the transcriptomic level. The major findings are as follows (1): we show a differential mRNA and lncRNA expression profiling of GCs from OEM patients and report significant differentially expressed mRNAs, known and novel lncRNAs; (2) we have identified a group of mRNAs and lncRNAs that likely to be involved in regulating ovarian reserve and are closely correlated to IVF clinical outcomes; and (3) we have constructed mRNA–lncRNA co-expression networks that display abnormal and complex regulatory mechanisms of GCs from infertility patients due to OEM at transcriptional level. These findings demonstrate that the distinctive molecular signatures are involved in the pathological process of ovarian reserve dysfunction in OEM patients, and the potential regulatory relationship between mRNA and lncRNA in GCs from OEM patients make some novel lncRNAs as diagnostic or therapeutic targets.
The use of human GC samples for this study was approved by the Institutional Review Board of Sir Run Run Shaw Hospital, Zhejiang University School of Medicine (ethical approval no. 20200423-44), and signed informed consent was obtained from all participants before inclusion.
GCs were collected from infertile women who underwent IVF due to unilateral or bilateral OEM or from women in couples with MF infertility (henceforth “MF group”) from May 2018 to April 2019. The inclusion criteria for both groups of women included an age of 20–42 years, regular menstrual cycles of 25–35 days, body mass index (BMI) of 18–25 kg/m2, and normal hormone profile in the early proliferative phase (days 2–5 of the menstrual cycle). Furthermore, patients from the MF group did not show signs of endometriosis or any other female infertility problems. For these patients, the main reason of infertility was diagnosed low sperm quantity and quality, abnormal chromosome, or sexual dysfunction of their husband. Simultaneously, patients from the OEM group underwent previous laparoscopic surgery for unilateral or bilateral ovarian endometriomas, with clear pathological diagnosis, and were classified as stage II–IV according to the World Endometriosis Society consensus on the classification of endometriosis (2). The detailed information about the distribution (bilaterally or unilaterally), location, and size of lesions of all OEM patients included in this study is listed in Supplementary Table S6. In addition, patients with chromosomal abnormalities, acute inflammation, malignant tumors of the reproductive system, previous endometrial neoplasias, or other anatomical endometrial pathologies were excluded.
All patients included in this study underwent controlled ovarian stimulation with gonadotropins. Oocyte retrieval was scheduled 36 h later after human chorionic gonadotropin (hCG, Livzon, Guangdong, China) was administered. When preovulatory follicles were aspirated, the oocyte–cumulus complexes were pulled from the follicle wall with the fluid. Under a microscope, all the oocyte–cumulus complexes from one single patient were identified and picked out and then washed in Gemmate medium (Cook Medical, Indianapolis, IN, USA). Before oocytes were removed for further culture prior to IVF, oocytes and cumulus cells were separated from the oocyte–cumulus complexes with syringe needles. The rest of the cumulus cells, as a subtype of GCs, were pooled and collected for RNA extraction.
Total RNA was extracted using Trizol reagent (Invitrogen, Carlsbad, CA, USA) following the manufacturer’s procedure. The total RNA quantity and purity were analyzed using an Agilent Bioanalyzer 2100 and RNA 1000 Nano LabChip kits (Agilent Technologies, Santa Clara, CA, USA) with an RNA integrity number >7.0. An RNA-seq library was constructed following the manufacturer’s instructions using SMARTer® Stranded Total RNA-Seq kits v. 2 (Takara Bio USA, Inc., Mountain View, CA, USA). The first-strand cDNA was synthesized from 10 ng of diluted RNA. Then, Illumina adapters and barcodes were added to the cDNA fragments. After purification of the RNA-Seq library with AMPure beads and depletion of ribosomal cDNA with ZapR v. 2 and R-Probes v. 2, the final RNA-Seq library was amplificated and enriched. The mean insert size for the paired-end libraries was 300 bp (± 50 bp).
Paired-end sequencing was performed on an Illumina X10 sequencing system with a constructed human GC RNA-seq library at LC-Bio Technology Co. Ltd. (Hangzhou, China). The average sequencing depth is 10.98 G. After quality control for reads, 5.81 G was left.
We aligned reads to the UCSC Homo sapiens reference genome GRCh38 (http://genome.ucsc.edu/) using the HISAT package (http://daehwankimlab.github.io/hisat2/). HISAT allows multiple alignments per read (up to 20 by default) and a maximum of two mismatches when mapping the reads to the reference genome.
The mapped reads of each sample were assembled using StringTie (https://ccb.jhu.edu/software/stringtie/). Then, all transcriptomes from the samples were merged to reconstruct a comprehensive transcriptome using gffcompare (github.com/gpertea/gffcompare/). StringTie (66) was used to calculate the expression levels for mRNAs and lncRNAs by calculating FPKM. The differentially expressed mRNAs and lncRNAs were selected with |log2(FC)| ≥ 1 and with statistical significance (p < 0.05) using R package edgeR (67).
To identify novel lncRNA, transcripts that overlapped with known mRNAs and transcripts shorter than 200 bp were discarded. Then, we utilized CPC0.9-r2 (68) (cpc2.cbi.pku.edu.cn/) with default parameters (cpc2 -i novel.fa -o cpc2.out) and CNCI2.0 (69) (www.bioinfo.org/software/cnci) with default parameters (CNCI.py -f novel.fa -o CNCI.result -p 1 -m ve -g novel.gtf -d genome.fa) to predict the coding potential for transcripts. All transcripts with CPC score <−1 and CNCI score <0 were removed, and the remained transcripts were considered as novel lncRNAs.
All the significantly DEGs, including 396 upregulated and 63 downregulated genes, were subjected to Kyoto Encyclopedia of Genes and Genomes (KEGG) enrichment and Gene Ontology (GO) evaluation. “S” was the number of genes with significant difference in specific GO/KEGG categories, “TS” represented the number of genes with significant difference, “B” was the number of genes for a specific GO/KEGG, and “TB” was the total number of genes. KEGG pathways enriched and presented downregulated and upregulated genes with similar biological function. The enrichment of down- and upregulated genes for the same GO function was also demonstrated. The higher rich factor (S gene number divided by B gene number) represents the higher enrichment degree. The calculation formula of p-values of enrichment analysis was: . The R package Ggplot2 (70) was used to visualize the data of analysis.
Selected DEGs were corroborated through real-time quantitative polymerase chain reaction (qPCR) as described previously (6). Primers are shown in Supplementary Table S7. The validation of primers for qPCR was estimated through the melting curve of qPCR procedure. Glyceraldehyde 3-phosphate dehydrogenase (GAPDH) was used as the endogenous control. For each sample, the average cycle threshold (Ct) was calculated from triplicate wells that varied by <0.5 Ct. All Ct values were normalized against the average Ct values of the GC samples from the MF patient group.
The Weighted Gene Co-expression Network Analysis (WGCNA) (71) was used to obtain the network relationship. According to the relationship, Cytoscape was used to draw the network diagram. Correlation network was performed using the OmicStudio tools at https://www.omicstudio.cn/tool. A Pearson’s correlation analysis was subsequently performed to cluster samples and detect outliers. The threshold of co-expression network between known/novel lncRNAs and clinical characteristics was with |correlation coefficient| > 0.5, p < 0.05. The threshold of co-expression network between known/novel lncRNAs and validated DEGs was with |correlation coefficient| > 0.9, p < 0.05.
Linear regression was applied to assess the correlation between validated DEGs and IVF clinical outcomes. Spearman correlation analysis was used to construct correlation heatmap between clinical characteristics and the expression of mRNAs and lncRNAs. Pearson correlation analysis was utilized to analyze co-expression relationships between mRNAs and lncRNAs. p < 0.05 is considered statistically significant. The data were analyzed using IBM SPSS Statistics v. 20.0 for Mac (IBM Corp., Armonk, NY, USA). Venn’s diagrams were schematized by using https://bioinfogp.cnb.csic.es/tools/venny/index.html.
The datasets presented in this study can be found in online repositories. The names of the repository/repositories and accession number(s) can be found below: https://bigd.big.ac.cn/gsa-human/, HRA000391.
The studies involving human participants were reviewed and approved by the Institutional Review Board of Sir Run Run Shaw Hospital, Zhejiang University School of Medicine. The patients/participants provided their written informed consent to participate in this study.
LS made substantial contributions to conception and design and interpretation of data, and wrote and edited the manuscript. XW was involved in experimental execution and sample collection. BW and CY mainly analyzed RNA-seq data and revised the manuscript critically for important intellectual content. CL and FZ were involved in sample collection and acquisition of clinical data. YD executed qPCR to validate gene expression. JC was involved in the selection and recruitment of patients with OEM and MF. XL contributed to RNA extraction and reverse transcription. SZ devised and supervised the study and made final approval of the version to be published. All authors contributed to the article and approved the submitted version.
This research was funded by grants from the National Natural Science Foundation of China (grant numbers 81971358, U20A20349, and 81871135), Medical Science and Technology Project Foundation of Zhejiang Province (2018KY486, 2018KY465, 2019KY411, and 2020KY606), and National Key Research and Development Program grant 2018YFC1004800.
The authors declare that the research was conducted in the absence of any commercial or financial relationships that could be construed as a potential conflict of interest.
All claims expressed in this article are solely those of the authors and do not necessarily represent those of their affiliated organizations, or those of the publisher, the editors and the reviewers. Any product that may be evaluated in this article, or claim that may be made by its manufacturer, is not guaranteed or endorsed by the publisher.
The authors sincerely appreciate all the study participants who made this work possible and the medical staff from the Assisted Reproduction Unit of Sir Run Run Shaw Hospital for their cooperation and assistance in obtaining samples and clinical data. The authors are grateful to Haiyan Zhao for the graphic abstract drawing.
The Supplementary Material for this article can be found online at: https://www.frontiersin.org/articles/10.3389/fendo.2022.825934/full#supplementary-material
1. Bulun SE, Yilmaz BD, Sison C, Miyazaki K, Bernardi L, Liu S, et al. Endometriosis. Endocr Rev (2019) 40(4):1048–79. doi: 10.1210/er.2018-00242
2. Johnson NP, Hummelshoj L, Adamson GD, Keckstein J, Taylor HS, Abrao MS, et al. World Endometriosis Society Consensus on the Classification of Endometriosis. Hum Reprod (2017) 32(2):315–24. doi: 10.1093/humrep/dew293
3. Younis JS, Shapso N, Fleming R, Ben-Shlomo I, Izhaki I. Impact of Unilateral Versus Bilateral Ovarian Endometriotic Cystectomy on Ovarian Reserve: A Systematic Review and Meta-Analysis. Hum Reprod Update (2019) 25(3):375–91. doi: 10.1093/humupd/dmy049
4. Benaglia L, Busnelli A, Biancardi R, Vegetti W, Reschini M, Vercellini P, et al. Oocyte Retrieval Difficulties in Women With Ovarian Endometriomas. Reprod BioMed Online (2018) 37(1):77–84. doi: 10.1016/j.rbmo.2018.03.020
5. Muzii L, Di Tucci C, Di Feliciantonio M, Galati G, Verrelli L, Donato VD, et al. Management of Endometriomas. Semin Reprod Med (2017) 35(1):25–30. doi: 10.1055/s-0036-1597126
6. Shi LB, Zhou F, Zhu HY, Huang D, Jin XY, Li C, et al. Transforming Growth Factor Beta1 From Endometriomas Promotes Fibrosis in Surrounding Ovarian Tissues via Smad2/3 Signaling. Biol Reprod (2017) 97(6):873–82. doi: 10.1093/biolre/iox140
7. Chapron C, Marcellin L, Borghese B, Santulli P. Rethinking Mechanisms, Diagnosis and Management of Endometriosis. Nat Rev Endocrinol (2019) 15(11):666–82. doi: 10.1038/s41574-019-0245-z
8. Nicolaus K, Brauer D, Sczesny R, Jimenez-Cruz J, Buhler K, Hoppe I, et al. Endometriosis Reduces Ovarian Response in Controlled Ovarian Hyperstimulation Independent of AMH, AFC, and Women's Age Measured by Follicular Output Rate (FORT) and Number of Oocytes Retrieved. Arch Gynecol Obstet (2019) 300(6):1759–65. doi: 10.1007/s00404-019-05337-z
9. Li Y, Li R, Ouyang N, Dai K, Yuan P, Zheng L, et al. Investigating the Impact of Local Inflammation on Granulosa Cells and Follicular Development in Women With Ovarian Endometriosis. Fertil Steril (2019) 112(5):882–91.e1. doi: 10.1016/j.fertnstert.2019.07.007
10. Gonzalez-Foruria I, Soldevila PB, Rodriguez I, Rodriguez-Purata J, Pardos C, Garcia S, et al. Do Ovarian Endometriomas Affect Ovarian Response to Ovarian Stimulation for IVF/ICSI? Reprod BioMed Online (2020) 41(1):37–43. doi: 10.1016/j.rbmo.2020.03.013
11. Orazov MR, Radzinsky VY, Ivanov II, Khamoshina MB, Shustova VB. Oocyte Quality in Women With Infertility Associated Endometriosis. Gynecol Endocrinol (2019) 35(sup1):24–6. doi: 10.1080/09513590.2019.1632088
12. Sanchez AM, Vanni VS, Bartiromo L, Papaleo E, Zilberberg E, Candiani M, et al. Is the Oocyte Quality Affected by Endometriosis? A Rev Lit J Ovarian Res (2017) 10(1):43. doi: 10.1186/s13048-017-0341-4
13. Choi SW, Kim HW, Nam JW. The Small Peptide World in Long Noncoding RNAs. Brief Bioinform (2019) 20(5):1853–64. doi: 10.1093/bib/bby055
14. Kopp F, Mendell JT. Functional Classification and Experimental Dissection of Long Noncoding RNAs. Cell (2018) 172(3):393–407. doi: 10.1016/j.cell.2018.01.011
15. Cui D, Ma J, Liu Y, Lin K, Jiang X, Qu Y, et al. Analysis of Long Non-Coding RNA Expression Profiles Using RNA Sequencing in Ovarian Endometriosis. Gene (2018) 673:140–8. doi: 10.1016/j.gene.2018.06.046
16. Ghafouri-Fard S, Shoorei H, Taheri M. Role of Non-Coding RNAs in the Pathogenesis of Endometriosis. Front Oncol (2020) 10:1370. doi: 10.3389/fonc.2020.01370
17. Panir K, Schjenken JE, Robertson SA, Hull ML. Non-Coding RNAs in Endometriosis: A Narrative Review. Hum Reprod Update (2018) 24(4):497–515. doi: 10.1093/humupd/dmy014
18. Bai J, Wang B, Wang T, Ren W. Identification of Functional lncRNAs Associated With Ovarian Endometriosis Based on a ceRNA Network. Front Genet (2021) 12:534054. doi: 10.3389/fgene.2021.534054
19. Zhang Y, Yan Z, Qin Q, Nisenblat V, Chang HM, Yu Y, et al. Transcriptome Landscape of Human Folliculogenesis Reveals Oocyte and Granulosa Cell Interactions. Mol Cell (2018) 72(6):1021–1034 e4. doi: 10.1016/j.molcel.2018.10.029
20. Ferrero H, Corachan A, Aguilar A, Quinonero A, Carbajo-Garcia MC, Alama P, et al. Single-Cell RNA Sequencing of Oocytes From Ovarian Endometriosis Patients Reveals a Differential Transcriptomic Profile Associated With Lower Quality. Hum Reprod (2019) 34(7):1302–12. doi: 10.1093/humrep/dez053
21. Dumesic DA, Meldrum DR, Katz-Jaffe MG, Krisher RL, Schoolcraft WB. Oocyte Environment: Follicular Fluid and Cumulus Cells Are Critical for Oocyte Health. Fertil Steril (2015) 103(2):303–16. doi: 10.1016/j.fertnstert.2014.11.015
22. Clarke HJ. Regulation of Germ Cell Development by Intercellular Signaling in the Mammalian Ovarian Follicle. Wiley Interdiscip Rev Dev Biol (2018) 7(1):1–22. doi: 10.1002/wdev.294
23. Li SH, Lin MH, Hwu YM, Lu CH, Yeh LY, Chen YJ, et al. Correlation of Cumulus Gene Expression of GJA1, PRSS35, PTX3, and SERPINE2 With Oocyte Maturation, Fertilization, and Embryo Development. Reprod Biol Endocrinol (2015) 13:93. doi: 10.1186/s12958-015-0091-3
24. Assidi M, Montag M, Sirard MA. Use of Both Cumulus Cells' Transcriptomic Markers and Zona Pellucida Birefringence to Select Developmentally Competent Oocytes in Human Assisted Reproductive Technologies. BMC Genomics (2015) 16 Suppl:1, S9. doi: 10.1186/1471-2164-16-S1-S9
25. Kahraman S, Cetinkaya CP, Cetinkaya M, Tufekci MA, Ekmekci CG, Montag M. Is There a Correlation Between Follicle Size and Gene Expression in Cumulus Cells and Is Gene Expression an Indicator of Embryo Development? Reprod Biol Endocrinol (2018) 16(1):69. doi: 10.1186/s12958-018-0388-0
26. Wyse BA, Fuchs Weizman N, Kadish S, Balakier H, Sangaralingam M, Librach CL. Transcriptomics of Cumulus Cells - A Window Into Oocyte Maturation in Humans. J Ovarian Res (2020) 13(1):93. doi: 10.1186/s13048-020-00696-7
27. Fragouli E, Lalioti MD, Wells D. The Transcriptome of Follicular Cells: Biological Insights and Clinical Implications for the Treatment of Infertility. Hum Reprod Update (2014) 20(1):1–11. doi: 10.1093/humupd/dmt044
28. Kasapoglu I, Ata B, Uyaniklar O, Seyhan A, Orhan A, Yildiz Oguz S, et al. Endometrioma-Related Reduction in Ovarian Reserve (ERROR): A Prospective Longitudinal Study. Fertil Steril (2018) 110(1):122–7. doi: 10.1016/j.fertnstert.2018.03.015
29. Nankali A, Kazeminia M, Jamshidi PK, Shohaimi S, Salari N, Mohammadi M, et al. The Effect of Unilateral and Bilateral Laparoscopic Surgery for Endometriosis on Anti-Mullerian Hormone (AMH) Level After 3 and 6 Months: A Systematic Review and Meta-Analysis. Health Qual Life Outcomes (2020) 18(1):314. doi: 10.1186/s12955-020-01561-3
30. Ju Y, Mizutani T, Imamichi Y, Yazawa T, Matsumura T, Kawabe S, et al. Nuclear Receptor 5A (NR5A) Family Regulates 5-Aminolevulinic Acid Synthase 1 (ALAS1) Gene Expression in Steroidogenic Cells. Endocrinology (2012) 153(11):5522–34. doi: 10.1210/en.2012-1334
31. Guo R, Chen F, Shi Z. Suppression of Notch Signaling Stimulates Progesterone Synthesis by Enhancing the Expression of NR5A2 and NR2F2 in Porcine Granulosa Cells. Genes (Basel) (2020) 11(2):1–12. doi: 10.3390/genes11020120
32. Bertolin K, Gossen J, Schoonjans K, Murphy BD. The Orphan Nuclear Receptor Nr5a2 Is Essential for Luteinization in the Female Mouse Ovary. Endocrinology (2014) 155(5):1931–43. doi: 10.1210/en.2013-1765
33. Zhang J, Xu Y, Liu H, Pan Z. MicroRNAs in Ovarian Follicular Atresia and Granulosa Cell Apoptosis. Reprod Biol Endocrinol (2019) 17(1):9. doi: 10.1186/s12958-018-0450-y
34. Younis JS, Matilsky M, Radin O, Ben-Ami M. Increased Progesterone/Estradiol Ratio in the Late Follicular Phase Could Be Related to Low Ovarian Reserve in In Vitro Fertilization-Embryo Transfer Cycles With a Long Gonadotropin-Releasing Hormone Agonist. Fertil Steril (2001) 76(2):294–9. doi: 10.1016/s0015-0282(01)01918-5
35. Younis JS. The Role of Progesterone/Estradiol Ratio in Exploring the Mechanism of Late Follicular Progesterone Elevation in Low Ovarian Reserve Women. Med Hypotheses (2019) 125:126–8. doi: 10.1016/j.mehy.2019.02.047
36. Griffin D, Liu X, Pru C, Pru JK, Peluso JJ. Expression of Progesterone Receptor Membrane Component-2 Within the Immature Rat Ovary and Its Role in Regulating Mitosis and Apoptosis of Spontaneously Immortalized Granulosa Cells. Biol Reprod (2014) 91(2):36. doi: 10.1095/biolreprod.114.117481
37. Peluso JJ, Pru CA, Liu X, Kelp NC, Pru JK. Progesterone Receptor Membrane Component 1 and 2 Regulate Granulosa Cell Mitosis and Survival Through a NFKappaB-Dependent Mechanismdagger. Biol Reprod (2019) 100(6):1571–80. doi: 10.1093/biolre/ioz043
38. Sueldo C, Liu X, Peluso JJ. Progestin and AdipoQ Receptor 7, Progesterone Membrane Receptor Component 1 (PGRMC1), and PGRMC2 and Their Role in Regulating Progesterone's Ability to Suppress Human Granulosa/Luteal Cells From Entering Into the Cell Cycle. Biol Reprod (2015) 93(3):63. doi: 10.1095/biolreprod.115.131508
39. Skiadas CC, Duan S, Correll M, Rubio R, Karaca N, Ginsburg ES, et al. Ovarian Reserve Status in Young Women Is Associated With Altered Gene Expression in Membrana Granulosa Cells. Mol Hum Reprod (2012) 18(7):362–71. doi: 10.1093/molehr/gas008
40. Eapen MS, Kota A, Vindin H, McAlinden KD, Xenaki D, Oliver BG, et al. Apoptosis Signal-Regulating Kinase 1 Inhibition Attenuates Human Airway Smooth Muscle Growth and Migration in Chronic Obstructive Pulmonary Disease. Clin Sci (Lond) (2018) 132(14):1615–27. doi: 10.1042/CS20180398
41. Sirotkin AV, Petrak J, Alwasel S, Harrath AH. Apoptosis Signal-Regulating Kinase (ASK1) and Transcription Factor Tumor Suppressor Protein TP53 Suppress Rabbit Ovarian Granulosa Cell Functions. Anim Reprod Sci (2019) 204:140–51. doi: 10.1016/j.anireprosci.2019.03.018
42. Lin X, Dai Y, Tong X, Xu W, Huang Q, Jin X, et al. Excessive Oxidative Stress in Cumulus Granulosa Cells Induced Cell Senescence Contributes to Endometriosis-Associated Infertility. Redox Biol (2020) 30:101431. doi: 10.1016/j.redox.2020.101431
43. Da Broi MG, de Albuquerque FO, de Andrade AZ, Cardoso RL, Jordao Junior AA, Navarro PA. Increased Concentration of 8-Hydroxy-2'-Deoxyguanosine in Follicular Fluid of Infertile Women With Endometriosis. Cell Tissue Res (2016) 366(1):231–42. doi: 10.1007/s00441-016-2428-4
44. Sturchler E, Feurstein D, Chen W, McDonald P, Duckett D. Stress-Induced Nuclear Import of Apoptosis Signal-Regulating Kinase 1 Is Mediated by Karyopherin Alpha2/Beta1 Heterodimer. Biochim Biophys Acta (2013) 1833(3):583–92. doi: 10.1016/j.bbamcr.2012.10.023
45. Mihalas BP, Western PS, Loveland KL, McLaughlin EA, Holt JE. Changing Expression and Subcellular Distribution of Karyopherins During Murine Oogenesis. Reproduction (2015) 150(6):485–96. doi: 10.1530/REP-14-0585
46. Sanchez AM, Vigano P, Quattrone F, Pagliardini L, Papaleo E, Candiani M, et al. The WNT/Beta-Catenin Signaling Pathway and Expression of Survival Promoting Genes in Luteinized Granulosa Cells: Endometriosis as a Paradigm for a Dysregulated Apoptosis Pathway. Fertil Steril (2014) 101(6):1688–96. doi: 10.1016/j.fertnstert.2014.02.040
47. Carr DW, DeManno DA, Atwood A, Hunzicker-Dunn M, Scott JD. Follicle-Stimulating Hormone Regulation of A-Kinase Anchoring Proteins in Granulosa Cells. J Biol Chem (1993) 268(28):20729–32. doi: 10.1016/S0021-9258(19)36841-3
48. Nishimura T, Sugiura K, Naito K. A-Kinase Anchor Protein 1 (AKAP1) Regulates cAMP-Dependent Protein Kinase (PKA) Localization and Is Involved in Meiotic Maturation of Porcine Oocytes. Biol Reprod (2013) 88(4):85. doi: 10.1095/biolreprod.112.106351
49. Nishimura T, Fujii W, Sugiura K, Naito K. Cytoplasmic Anchoring of cAMP-Dependent Protein Kinase (PKA) by A-Kinase Anchor Proteins (AKAPs) Is Required for Meiotic Arrest of Porcine Full-Grown and Growing Oocytes. Biol Reprod (2014) 90(3):58. doi: 10.1095/biolreprod.113.114736
50. Aragon L. The Smc5/6 Complex: New and Old Functions of the Enigmatic Long-Distance Relative. Annu Rev Genet (2018) 52:89–107. doi: 10.1146/annurev-genet-120417-031353
51. Farmer S, San-Segundo PA, Aragon L. The Smc5-Smc6 Complex Is Required to Remove Chromosome Junctions in Meiosis. PloS One (2011) 6(6):e20948. doi: 10.1371/journal.pone.0020948
52. Hwang G, Sun F, O'Brien M, Eppig JJ, Handel MA, Jordan PW. SMC5/6 Is Required for the Formation of Segregation-Competent Bivalent Chromosomes During Meiosis I in Mouse Oocytes. Development (2017) 144(9):1648–60. doi: 10.1242/dev.145607
53. Fu XH, Chen CZ, Li S, Han DX, Wang YJ, Yuan B, et al. Dual-Specificity Phosphatase 1 Regulates Cell Cycle Progression and Apoptosis in Cumulus Cells by Affecting Mitochondrial Function, Oxidative Stress, and Autophagy. Am J Physiol Cell Physiol (2019) 317(6):C1183–C93. doi: 10.1152/ajpcell.00012.2019
54. Tu J, Chen Y, Li Z, Yang H, Chen H, Yu Z. Long Non-Coding RNAs in Ovarian Granulosa Cells. J Ovarian Res (2020) 13(1):63. doi: 10.1186/s13048-020-00663-2
55. Liu H, Zhang Z, Xiong W, Zhang L, Du Y, Liu Y, et al. Long non-Coding RNA MALAT1 Mediates Hypoxia-Induced Pro-Survival Autophagy of Endometrial Stromal Cells in Endometriosis. J Cell Mol Med (2019) 23(1):439–52. doi: 10.1111/jcmm.13947
56. Feng Y, Tan BZ. LncRNA MALAT1 Inhibits Apoptosis of Endometrial Stromal Cells Through miR-126-5p-CREB1 Axis by Activating PI3K-AKT Pathway. Mol Cell Biochem (2020) 475(1-2):185–94. doi: 10.1007/s11010-020-03871-y
57. Du Y, Zhang Z, Xiong W, Li N, Liu H, He H, et al. Estradiol Promotes EMT in Endometriosis via MALAT1/miR200s Sponge Function. Reproduction (2019) 157(2):179–88. doi: 10.1530/REP-18-0424
58. Li Y, Liu YD, Chen SL, Chen X, Ye DS, Zhou XY, et al. Down-Regulation of Long non-Coding RNA MALAT1 Inhibits Granulosa Cell Proliferation in Endometriosis by Up-Regulating P21 via Activation of the ERK/MAPK Pathway. Mol Hum Reprod (2019) 25(1):17–29. doi: 10.1093/molehr/gay045
59. Sun Z, Jing C, Xiao C, Li T. An Autophagy-Related Long Non-Coding RNA Prognostic Signature Accurately Predicts Survival Outcomes in Bladder Urothelial Carcinoma Patients. Aging (Albany NY) (2020) 12(15):15624–37. doi: 10.18632/aging.103718
60. Xia XQ, Lu WL, Ye YY, Chen J. LINC00662 Promotes Cell Proliferation, Migration and Invasion of Melanoma by Sponging miR-890 to Upregulate ELK3. Eur Rev Med Pharmacol Sci (2020) 24(16):8429–38. doi: 10.26355/eurrev_202008_22640
61. Huang WJ, Tian XP, Bi SX, Zhang SR, He TS, Song LY, et al. The Beta-Catenin/TCF-4-LINC01278-miR-1258-Smad2/3 Axis Promotes Hepatocellular Carcinoma Metastasis. Oncogene (2020) 39(23):4538–50. doi: 10.1038/s41388-020-1307-3
62. Tian X, Wu Y, Yang Y, Wang J, Niu M, Gao S, et al. Long Noncoding RNA LINC00662 Promotes M2 Macrophage Polarization and Hepatocellular Carcinoma Progression via Activating Wnt/beta-Catenin Signaling. Mol Oncol (2020) 14(2):462–83. doi: 10.1002/1878-0261.12606
63. Tao LM, Gong YF, Yang HM, Pei JH, Zhao XJ, Liu SS. LINC00662 Promotes Glycolysis and Cell Survival by Regulating miR- 375/HIF-1alpha Axis in Ovarian Cancer. J Biol Regul Homeost Agents (2020) 34(3):467–77. doi: 10.23812/19-300-A-18
64. Joshi M, Rajender S. Long non-Coding RNAs (lncRNAs) in Spermatogenesis and Male Infertility. Reprod Biol Endocrinol (2020) 18(1):103. doi: 10.1186/s12958-020-00660-6
65. Cheng B, Rong A, Zhou Q, Li W. LncRNA LINC00662 Promotes Colon Cancer Tumor Growth and Metastasis by Competitively Binding With miR-340-5p to Regulate CLDN8/IL22 Co-Expression and Activating ERK Signaling Pathway. J Exp Clin Cancer Res (2020) 39(1):5. doi: 10.1186/s13046-019-1510-7
66. Pertea M, Pertea GM, Antonescu CM, Chang TC, Mendell JT, Salzberg SL. StringTie Enables Improved Reconstruction of a Transcriptome From RNA-Seq Reads. Nat Biotechnol (2015) 33(3):290–5. doi: 10.1038/nbt.3122
67. Robinson MD, McCarthy DJ, Smyth GK. Edger: A Bioconductor Package for Differential Expression Analysis of Digital Gene Expression Data. Bioinformatics (2010) 26(1):139–40. doi: 10.1093/bioinformatics/btp616
68. Kong L, Zhang Y, Ye ZQ, Liu XQ, Zhao SQ, Wei L, et al. CPC: Assess the Protein-Coding Potential of Transcripts Using Sequence Features and Support Vector Machine. Nucleic Acids Res (2007) 35(Web Server issue):W345–9. doi: 10.1093/nar/gkm391
69. Sun L, Luo H, Bu D, Zhao G, Yu K, Zhang C, et al. Utilizing Sequence Intrinsic Composition to Classify Protein-Coding and Long Non-Coding Transcripts. Nucleic Acids Res (2013) 41(17):e166. doi: 10.1093/nar/gkt646
70. Walter W, Sanchez-Cabo F, Ricote M. GOplot: An R Package for Visually Combining Expression Data With Functional Analysis. Bioinformatics (2015) 31(17):2912–4. doi: 10.1093/bioinformatics/btv300
Keywords: RNA sequencing, mRNA, lncRNA, ovarian endometriosis, granulosa cells, retrieved oocyte numbers, female infertility
Citation: Shi L, Wei X, Wu B, Yuan C, Li C, Dai Y, Chen J, Zhou F, Lin X and Zhang S (2022) Molecular Signatures Correlated With Poor IVF Outcomes: Insights From the mRNA and lncRNA Expression of Endometriotic Granulosa Cells. Front. Endocrinol. 13:825934. doi: 10.3389/fendo.2022.825934
Received: 30 November 2021; Accepted: 01 February 2022;
Published: 28 February 2022.
Edited by:
Aniel Sanchez Puente, Lund University, SwedenReviewed by:
Shin Matsubara, Suntory Foundation for Life Sciences, JapanCopyright © 2022 Shi, Wei, Wu, Yuan, Li, Dai, Chen, Zhou, Lin and Zhang. This is an open-access article distributed under the terms of the Creative Commons Attribution License (CC BY). The use, distribution or reproduction in other forums is permitted, provided the original author(s) and the copyright owner(s) are credited and that the original publication in this journal is cited, in accordance with accepted academic practice. No use, distribution or reproduction is permitted which does not comply with these terms.
*Correspondence: Songying Zhang, emhhbmdzb25neWluZ0B6anUuZWR1LmNu
Disclaimer: All claims expressed in this article are solely those of the authors and do not necessarily represent those of their affiliated organizations, or those of the publisher, the editors and the reviewers. Any product that may be evaluated in this article or claim that may be made by its manufacturer is not guaranteed or endorsed by the publisher.
Research integrity at Frontiers
Learn more about the work of our research integrity team to safeguard the quality of each article we publish.