- 1Laboratory of Experimental Medicine and Pediatrics and Member of the Infla-Med Centre of Excellence, Faculty of Medicine and Health Sciences, University of Antwerp, Antwerp, Belgium
- 2Department of Pediatrics, Antwerp University Hospital, Edegem, Belgium
- 3Zeepreventorium VZW, De Haan, Belgium
- 4Department of Developmental, Personality and Social Psychology, Ghent University, Ghent, Belgium
- 5Department of Developmental Psychology, Radboud University, Nijmegen, Netherlands
- 6Department of Pediatric Cardiology, Amsterdam University Medical Centers, Amsterdam, Netherlands
Background: Inpatient pediatric obesity treatments are highly effective, although dropouts and weight regain threaten long-term results. Preliminary data indicate that leptin, adiponectin, and cardiometabolic comorbidities might predict treatment outcomes. Previous studies have mainly focused on the individual role of adipokines and comorbidities, which is counterintuitive, as these risk factors tend to cluster. This study aimed to predict the dropouts and treatment outcomes by pre-treatment patient characteristics extended with cardiometabolic comorbidities (individually and in total), leptin, and adiponectin.
Methods: Children aged 8–18 years were assessed before, immediately after and 6 months after a 12-month inpatient obesity treatment. Anthropometric data were collected at each visit. Pre-treatment lipid profiles; glucose, insulin, leptin, and adiponectin levels; and blood pressure were measured. The treatment outcome was evaluated by the change in body mass index (BMI) standard deviation score (SDS) corrected for age and sex.
Results: We recruited 144 children with a mean age of 14.3 ± 2.2 years and a mean BMI of 36.7 ± 6.2 kg/m2 corresponding to 2.7 ± 0.4 BMI SDS. The 57 patients who dropped out during treatment and the 44 patients who dropped out during aftercare had a higher pre-treatment BMI compared to the patients who completed the treatment (mean BMI, 38.3 ± 6.8 kg/m2 vs 35.7 ± 5.5 kg/m2) and those who completed aftercare (mean BMI, 34.6 ± 5.3 kg/m2 vs 37.7 ± 6.3 kg/m2) (all p<0.05). Additionally, aftercare attenders were younger than non-attenders (mean age, 13.4 ± 2.3 years vs 14.9 ± 2.0, p<0.05).
Patients lost on average 1.0 ± 0.4 SDS during treatment and regained 0.4 ± 0.3 SDS post-treatment corresponding to regain of 43 ± 27% (calculated as the increase in BMI SDS post-treatment over the BMI SDS lost during treatment). A higher BMI and more comorbidities inversely predicted BMI SDS reduction in linear regression (all p<0.05).
The absolute BMI SDS increase after returning home was predicted by pre-treatment leptin and systolic blood pressure, whereas the post-treatment BMI SDS regain was predicted by pre-treatment age, leptin, and adiponectin levels (all p<0.05) in multivariate linear regressions.
Conclusion: Patients who need treatment the most are at increased risk for dropouts and weight regain, emphasizing the urgent need for interventions to reduce dropout and support inpatients after discharge. Furthermore, this study is the first to report that pre-treatment leptin and adiponectin levels predict post-treatment BMI SDS regain, requiring further research.
1 Introduction
The worldwide obesity pandemic poses an important threat to global healthcare due to the coincidence of medical comorbidities and socioeconomic repercussions from obesity-related healthcare costs (1, 2). Obesity tends to persist from childhood to adulthood (3). Therefore, it is crucial to treat excess weight at a young age to tackle the growing obesity burden. Highly successful residential treatment programs are available for children (4). However, inpatient (pediatric) obesity treatment programs face two major challenges. The first challenge is to retain patients within the treatment program, as early dropout is often reported (4, 5), and premature treatment cessation results in less chance of achieving or maintaining weight reduction (6, 7). Research on predictors of dropout in children undergoing obesity treatment varies among the predictors studied, and the findings between different studies are inconsistent. It is uncertain whether these inconsistent findings result from population or treatment differences or from another definition or measurement of dropouts (8). The second challenge is the problem of regaining weight after discharge from the rehabilitation center (9). A previous trial in the Netherlands found no significant difference in body mass index (BMI) standard deviation score (SDS) 2 years after follow-up between a group of obese children treated in residential care versus a group treated by an outpatient lifestyle intervention, even though the inpatient group lost significantly more weight initially, for example, a BMI reduction of 18% in the inpatient group compared to 10.5% in the outpatient group (10). As many studies regarding inpatient treatment outcomes focus on predicting (short-term) weight loss during treatment rather than exploring what happens after treatment cessation, evidence on predictive factors for long-term outcomes is rather scarce.
Besides standard patient characteristics, such as age, sex, and BMI, some studies indicate a role for certain metabolic comorbidities as predictors of treatment outcomes. The effect of insulin resistance has mostly been described (11–13), and a single study has broadened the research question to the predictive value of all metabolic components in children with obesity (14). However, to the best of our knowledge, no studies have investigated whether the cumulative number of cardiometabolic comorbidities influences the treatment outcomes in children with obesity.
Some adipocyte hormones, such as leptin and adiponectin, might also play a role in weight regulation and treatment outcome (15–17). Leptin is involved in regulating appetite and satiety as well as food intake, energy homeostasis, and body fat regulation due to its central-acting properties in the hypothalamus (18). Individuals with obesity have higher circulating leptin levels (19, 20) and some may be leptin-resistant, similar to the more widely known insulin-resistant state. Nevertheless, the exact underlying mechanism responsible for leptin resistance is not completely understood (21). In contrast to leptin levels, adiponectin levels are reduced in obesity (22, 23). Adiponectin is produced by the adipose tissue itself and generally exerts health-protective effects. It has antidiabetic, anti-inflammatory, antiatherogenic, and cardioprotective properties (24), and some evidence also indicates the role of adiponectin in regulating energy homeostasis, with adiponectin exerting both peripheral and central effects, increasing the body’s energy expenditure (24–26). Previous studies have demonstrated the predictive potential of leptin and adiponectin with regard to future BMI change in children aged 9–10 years (15) and short-term weight loss during treatment in overweight and obese children (16, 17).
Interestingly, previous studies have focused on metabolic comorbidities and adipokines as stand-alone factors that might affect treatment outcomes, however these risk factors often tend to cluster (27). Therefore, in this study, we determined the predictive value of baseline patient characteristics (age, sex, and adiposity) extended with cardiometabolic comorbidities, leptin, and adiponectin in early (during treatment) and late (during aftercare) dropouts and short- and long-term treatment outcomes in children with obesity treated in an inpatient pediatric obesity treatment program.
2 Materials and Methods
2.1 Study Design
Patients were consecutively recruited between July 2017 and January 2018 upon participation in a 12-month inpatient weight loss program at “Het Zeepreventorium,” a pediatric rehabilitation center in De Haan, Belgium. This program is described in more detail in Section 2.3 (28).
A pre-treatment baseline assessment was planned for all patients, after which two follow-up visits were planned: a first follow-up visit at the end of residential treatment (corresponding to 12 months from baseline) and a second follow-up visit at 6 months after discharge from the rehabilitation center (corresponding to 18 months from baseline).
This study was approved by the Ethics Committee of Ghent University Hospital (EC n°B670201731779), and written informed consent was obtained from the children and their parents or legal representatives. The data used in this study were collected as part of a previous randomized controlled trial registered in the International Standard Randomised Controlled Trial Number (ISRCTN) registry (ISRCTN14722584).
2.2 Patients
Participants were eligible for inclusion if they fulfilled the following criteria:
- Aged 8–18 years
- Obesity defined by the International Obesity Task Force (IOTF) criteria based on the Flemish growth curves (29, 30)
Patients were excluded if they had an active, chronic disease precluding them from optimal treatment participation (such as impairment in the motor or sensory functions of the lower extremities), use of weight-loss antagonizing medications, or an endogenous (hormonal or genetic) etiology of their obesity.
2.3 Multidisciplinary Obesity Treatment Program
The treatment program consisted of a 12-month inpatient weight loss program, focusing on dietary modifications, physical activity, and psychological counseling, individually or in groups.
Regarding physical activity, the inpatient residents were required to engage in physical activities in groups (games or supervised team sports) for at least 1 hour/day. This was supplemented with an individual, monitored exercise program for 3 hours/week, which was led by a physiotherapist.
The dietary program focused on acquiring a structured and healthy eating pattern. Three main meals and three pieces of fruit were offered daily. Dietitians determined a minimal and a maximal portion for each main meal requiring all necessary components of a healthy diet (10% protein, 50–55% carbohydrate, and 30–35% fat).
Parents were actively involved throughout the program and invited to participate in psychoeducation classes to enhance the implementation of a healthy lifestyle at home and learn and practice new parenting skills.
A more detailed description of the program has been published previously (28).
2.4 Clinical Evaluation
2.4.1 Anthropometry
Height was measured to the nearest 0.1 cm using a calibrated yard stick and weight was recorded to the nearest 0.05 kg using a standardized weighing scale (Seca Ltd., Birmingham, England). BMI (kg/m2) was calculated and further analyzed as BMI SDS, which was corrected for age and sex, using the Flemish growth charts as a reference population (29). Waist circumference was measured at the midpoint between the lowest rib and iliac crest, hip circumference was measured at the maximal circumference around the buttock, and waist-to-hip ratio was calculated.
2.4.2 Blood Pressure
Blood pressure was measured thrice with the patient in the supine position. The average systolic and diastolic blood pressures were calculated and further analyzed using the corresponding SDS adjusted for age, sex, and height (31).
2.4.3 Body Composition
The BCM (Body Composition Monitor (Fresenius Medical Care, St. Wendel, Germany)), a device based on the principles of bioimpedance spectroscopy, was used to determine fat and fat-free mass (expressed in kg and %). Measurements were obtained with the patient in a fasting state, lying supine with electrodes attached following the wrist-ankle approach, as described previously (32, 33). The measurement quality calculated using the BCM device was greater than 80% for all measurements.
2.4.4 Metabolic Profile
A fasting venous blood sample was drawn before the start of the inpatient treatment program to examine metabolic health by determining the lipid profile (triglycerides, total cholesterol, high density lipoprotein (HDL)-cholesterol, and low density lipoprotein (LDL)-cholesterol), pro-inflammatory status (high sensitivity C-reactive protein(hs-CRP)), glucose metabolism (fasting glucose, fasting insulin, homeostatic model assessment of insulin resistance (HOMA-IR [calculated as glucose × insulin/405])), and liver transaminases (aspartate aminotransferase (AST), alanine aminotransferase (ALT), AST/ALT ratio). All determinations were performed in the central laboratory of Antwerp University Hospital using standardized techniques.
To determine the influence of the cumulative number of comorbidities, we have categorized each of the five components of the metabolic syndrome, that is, abdominal obesity (waist circumference), hypertriglyceridemia, low HDL-cholesterol and high fasting glucose and arterial hypertension, as “present” or “not present,” and added up the number of comorbidities present, resulting in a score ranging from 0 to 5 for each patient.
Cutoff values were determined as follows:
- Triglyceride level ≥ 130 mg/dL (34)
- HDL-cholesterol level < 40 mg/dL (34)
- Waist circumference ≥ 90th percentile (35)
- Fasting glucose level ≥ 100 mg/dL (36)
- Arterial hypertension: systolic or diastolic blood pressure ≥ 90th percentile (31)
2.4.5 Adipokines
Leptin and total adiponectin were determined by a human adiponectin and leptin enzyme-linked immunosorbent assay according to the manufacturer’s guidelines (Invitrogen, Life Technologies, Waltham, Massachussets, USA) on a fasting venous blood sample drawn before the start of treatment. The lower limits of detection of the assay were 15.6 pg/mL for leptin and 0.5 ng/mL for adiponectin. The interassay coefficient of variation was 14.2% for leptin and 10.5% for adiponectin. The intra-assay coefficient of variation was <10% for all samples.
2.4.6 Sleep Evaluation
Patients underwent polygraphy using the portable screening device Apnealink Air® (Apnealink, Resmed, Basel, Switzerland) to screen for the presence of sleep apnea (37). The respiratory airflow was measured using a nasal pressure cannula (detecting -10 hPa to +10hPa), the saturation was measured by a pulse oximeter, and the respiratory effort was measured by a pressure sensor attached around the thorax (sampling rate of 10 Hz, detecting -6 hPa to +6 HPa). A minimum of 4 h of good-quality signal was required. Parameters of interest were the obstructive apnea-hypopnea index and oxygen desaturation index.
2.4.7 Endothelial Function
Endothelial function was assessed at the microvascular level by the Endo-PAT 2000® (Endo-PAT, Itamar Medical, Caesarea, Israel) following recommendations in children as described previously (38). The patients were placed in the supine position with pneumatic probes at both index fingers to measure pulsatile pressure changes in the small arteries. A blood pressure cuff was placed on the non-dominant arm of the patient, and the other arm served as a control. After a 5-min baseline assessment, an occlusion period followed in which the blood pressure cuff was inflated to 60 mmHg suprasystolic pressure for 5 min. Afterward, the cuff was deflated, and a period of reactive hyperemia occurred, which was recorded for another 5 min. The parameters of interest were maximal dilatation and the time to maximal dilatation during the hyperemia period.
2.4.8 Evaluation of Treatment Outcome
The following endpoints to assess treatment outcome were defined:
- BMI SDS decrease during inpatient treatment
- Absolute BMI SDS change after discharge from the inpatient treatment center to the six-month follow-up
- Relative increase (regain), expressed as the percentage of lost BMI SDS points regained, 6 months after treatment: This relative increase was calculated as follows: (BMI in SDS at the second follow-up – BMI in SDS at the end of treatment)/(BMI in SDS at baseline – BMI in SDS at the end of treatment).
However, the BMI SDS has some limitations in children and adolescents with severe obesity. Therefore, it is recommended to report more BMI-derived measures (39); therefore, supplementary analyses were performed with BMI (in kg/m2) as the outcome variable.
2.4.9 Statistical Analysis
First, data were explored for normality using a Kolmogorov–Smirnov test and/or histogram (depending on the sample size) and further analyzed as appropriate. Normally distributed data are presented as mean and standard deviation, whereas skewed data are reported as median, followed by minimum and maximum values. Associations between baseline data were explored by correlations and independent samples’ t-tests. To assess the longitudinal evolution of the patients’ weight, BMI, BMI SDS, and body composition, a linear mixed model was used with Bonferroni post hoc test to allow pairwise comparisons.
Second, the baseline patient characteristics of the patients who dropped out prematurely were compared to the group that completed the entire treatment program using the Welch or Mann–Whitney U test for continuous data or chi-square test for categorical data. The independent predictive values of these variables were tested using backward logistic regression. The same approach was applied to compare the baseline patient characteristics of the dropout cases during aftercare to aftercare completers.
Third, possible baseline predictors (patient characteristics, metabolic comorbidities, and adipokines) of BMI SDS reduction during treatment were identified by including each predictor as an independent variable in a univariate model (including the intercept) and the difference between baseline and end-of-treatment BMI SDS as the dependent variable. Significant associations are shown, and non-significant data are mentioned but not presented in a Table. Subsequently, all significant univariate predictors (p<0.05) were combined into a multivariate linear regression model to identify independent predictors. Multicollinearity was checked by examining the variance inflation factor. The same statistical approach was used to determine the baseline predictors of post-treatment outcomes (absolute and relative post-treatment BMI SDS re-increase) at the last follow-up visit, planned 6 months after patients had returned home.
For all analyses, a p-value of ≤0.05 was considered significant. When possible (based on the presence of a symmetrical distribution), two-tailed significance was used. All statistical analyses were performed using Statistical Package for Social Sciences (SPSS, version 27, IBM Inc., Armonk, New York, USA).
3 Results
3.1 Study Population
A total of 141 patients were included with a mean age of 14.3 ± 2.2 years and mean BMI of 36.7 ± 6.2 kg/m2, corresponding to a mean BMI SDS of 2.7 ± 0.4. The baseline patient characteristics are depicted in Table 1.
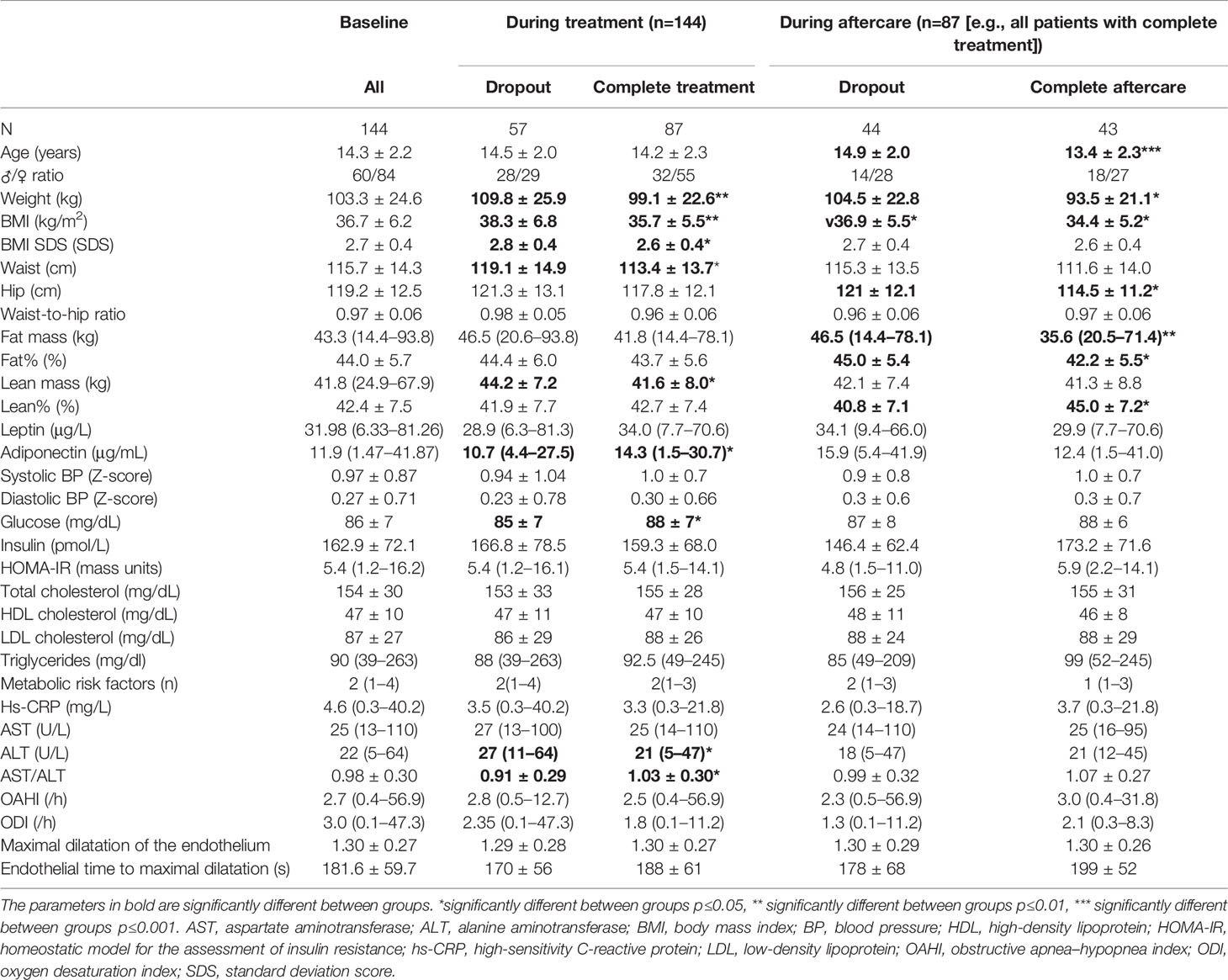
Table 1 Baseline characteristics and comparison between patients that have completed the obesity treatment program and follow-up and those who have not.
Patients had a median of two metabolic risk factors (range, 1–4) based on the cutoff values described in the methodology. All patients had a high waist circumference, 46 were hypertensive, 36 had low HDL-cholesterol, four had impaired glucose tolerance (based on fasting glucose), and 30 patients fulfilled the criteria of hypertriglyceridema. The number of metabolic comorbidities was associated with baseline weight (r=0.274, p=0.002), BMI (r=0.298, p<0.001), and BMI SDS (r=0.304, p<0.001) but not with age (p=0.40).
Pre-treatment age was significantly associated with baseline weight (r=0.61, p<0.001), BMI (r=0.53, p<0.001), and BMI SDS (r=0.40, p<0.001). Boys had a higher weight than girls (109.9 ± 28.2 kg vs 98.6 ± 20.2 kg, p=0.009), but no sex difference was found in BMI or BMI SDS (p>0.05).
3.2 Premature Dropout During Inpatient Treatment
Of the 144 patients included at baseline, 87 completed the 12-month inpatient treatment program, corresponding to a dropout rate of 40% (every patient who did not complete the entire treatment was considered a dropout, independent of their duration of stay). Patients who prematurely left the treatment center had a higher weight, BMI, BMI SDS, and waist circumference at baseline than those who completed the entire program as shown in Table 1. Additionally, a higher ALT (median, 27 U/L (11–64) vs 21 U/L (5–47); p=0.017), lower AST/ALT ratio (mean, 0.91 ± 0.29 vs 1.03 ± 0.30; p=0.021), and lower adiponectin levels (median, 10.7 (4.4–27.5) μg/mL vs median, 14.3 (1.5–30.7) μg/mL; p=0.021) were found in the group that dropped out before treatment was completed.
A logistic regression predicting dropout was built, including weight, AST/ALT ratio, fasting glucose, and adiponectin. As a representation of the anthropometric variables, weight was included as a predictor in the model, as this anthropometric variable most significantly differed between completers and dropouts based on the p-value and model fit (Nagelkerke R Square). For the same reason, the AST/ALT ratio was included in the model instead of AST or ALT values. After backward elimination of non-significant terms, only weight and adiponectin were retained with a p-value of 0.04 and 0.006, respectively (Nagelkerke R2 0.15).
3.3 Predictors of BMI Reduction During Inpatient Treatment
The 87 patients who completed the entire treatment program reduced their BMI by 1.0 ± 0.4 SDS, p<0.001. The evolution of the other anthropometric variables is presented in Table 2. Of all patients completing inpatient treatment, 43 (49%) remained obese, 21 (24%) achieved reduction to overweight, and 23 (26%) achieved reduction to a healthy BMI, based on the Flemish growth charts.
The BMI SDS decrease during inpatient treatment was inversely associated with baseline weight status, cumulative number of metabolic risk factors (see Section 2.4.4 for the cutoff values), and AST/ALT ratio (Table 3). No sex differences were found (p=0.7), and there was no association with pre-treatment age (p=0.3) or other individual baseline cardiometabolic risk factors, sleep or endothelial parameters (all p>0.05), leptin, or adiponectin (p=0.9 and p=0.5).
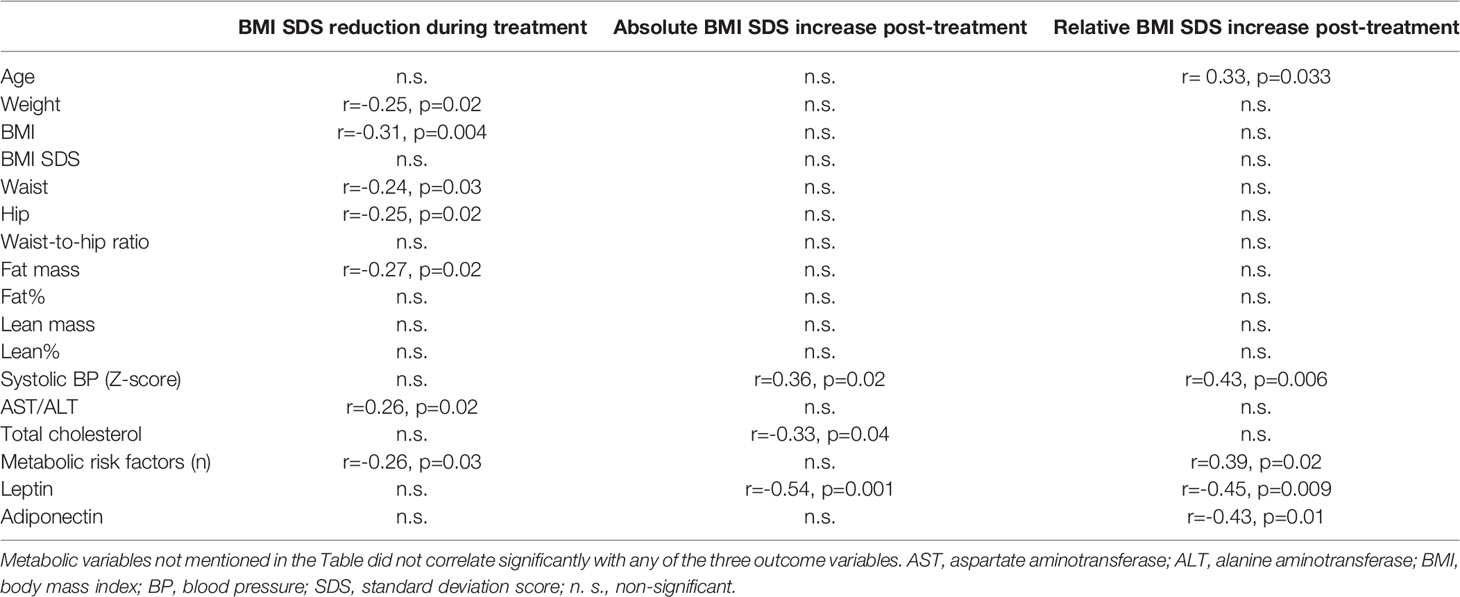
Table 3 Partial correlations from univariate linear regressions between baseline variables and treatment outcome.
A linear regression model was created with pre-treatment BMI, number of metabolic risk factors, and AST/ALT ratio as independent predictors. After the removal of non-significant terms, only BMI and the number of metabolic risk factors were retained as significant contributors to the BMI decrease during inpatient treatment (Table 4), resulting in an explained proportion of variance of 12.0%.

Table 4 Linear regression identifying pre-treatment factors predictive for BMI SDS loss during inpatient treatment after removal of non-significant terms (n=87).
3.4 Predictors of Dropout During Aftercare
Six months after discharge from the inpatient treatment center, 43 of the 87 patients participated in the follow-up visit (49.4%). The patients who attended the follow-up visit were generally younger than those who did not attend the last visit (13.4 ± 2.3 years vs 14.9 ± 2.0 years respectively; p=0.001) and had a lower weight and BMI before treatment initiation (Table 1).
There were no differences in sex (p=0.7) or in any metabolic, endothelial, or sleep-related parameters, including adipokines, between those attending aftercare and those who did not (all p > 0.05).
In a logistic regression predicting dropout during aftercare by pre-treatment age and weight, only age was significant (p=0.012 and Nagelkerke R2 0.15).
3.5 Predictors of Post-Treatment BMI Change
The 43 patients attending the 6-month follow-up visit regained 0.4 ± 0.3 SDS compared to the end of treatment (p < 0.001) (Figure 1). When controlling for lost weight during treatment, a relative BMI regain of 43 ± 27% was found. Nevertheless, there was high interindividual variability ranging from an additional loss of 27% to a complete regain of 102% relative to the previous BMI SDS reduction. More specifically, two patients had further reduced their BMI by more than 0.1 SDS, two patients had kept their BMI stable with an increase < 0.1 SDS, and the remaining 39 patients had increased BMI. Of all patients attending the last study visit, 20 remained obese (46%), 17 (40%) achieved reduction to overweight, and 6 (14%) achieved reduction to a healthy BMI based on the Flemish growth charts. On average, patients had lost 0.6 ± 0.4 SDS from baseline.
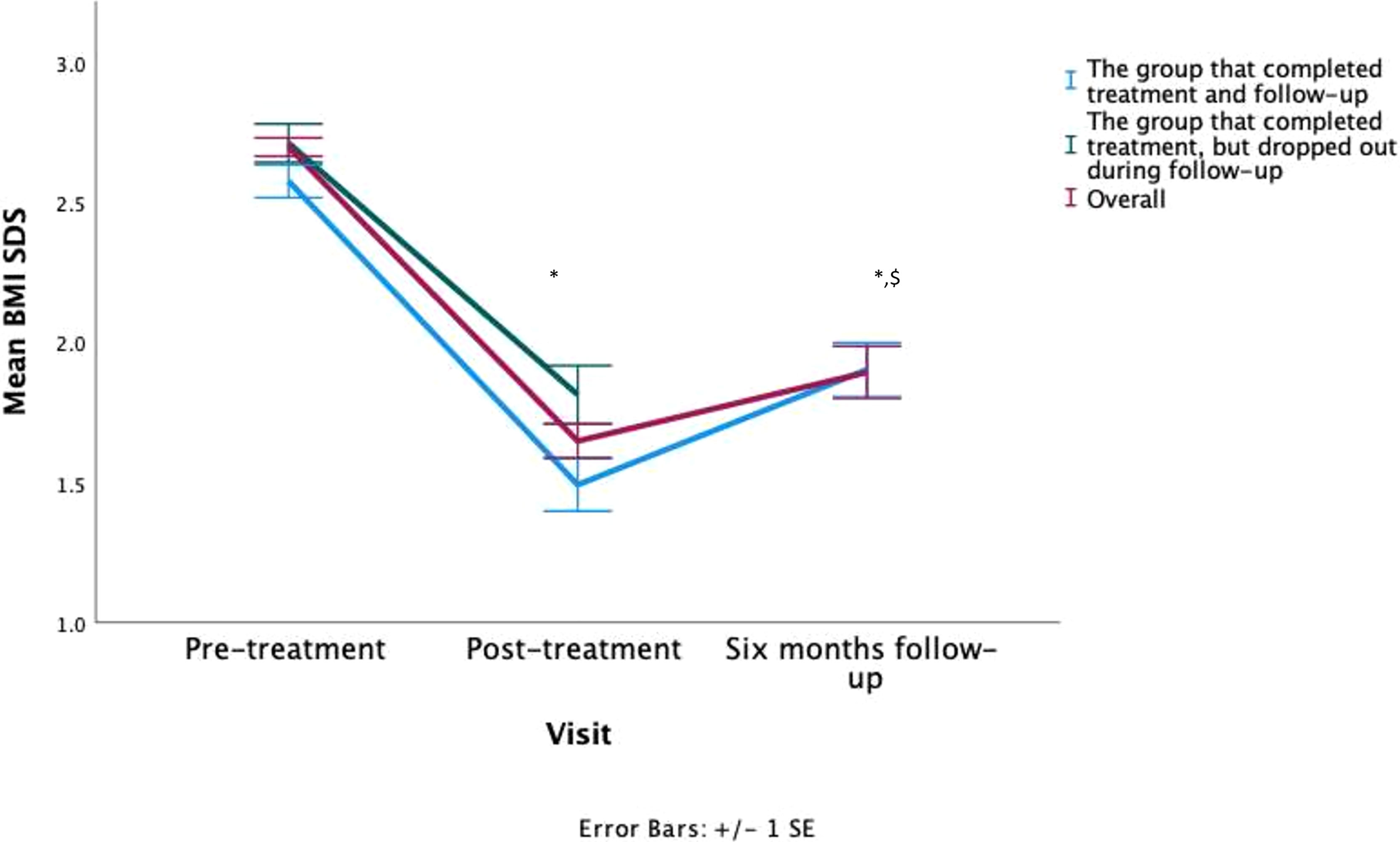
Figure 1 Visual representation of the patients their BMI SDS evolution, * = statistically significant from baseline, $ = statistically significant from post-treatment. Both were p<0.001.
The absolute change in BMI (in case of most patients, an increase) after inpatient treatment was not associated with baseline characteristics such as age or any anthropometric variables, and no differences in sex were found. Regarding metabolic profile (including sleep and endothelial function), no significant associations were found, except for a positive association between post-treatment BMI SDS increase and systolic blood pressure and an inverse association with baseline leptin and total cholesterol (Table 3). Linear regression was fitted, including systolic blood pressure, total cholesterol, and leptin. After the removal of total cholesterol because of non-significance, the model revealed a significant effect of leptin level (p=0.02) and systolic blood pressure (p=0.01), which explained 38% of the total variance (Table 5).
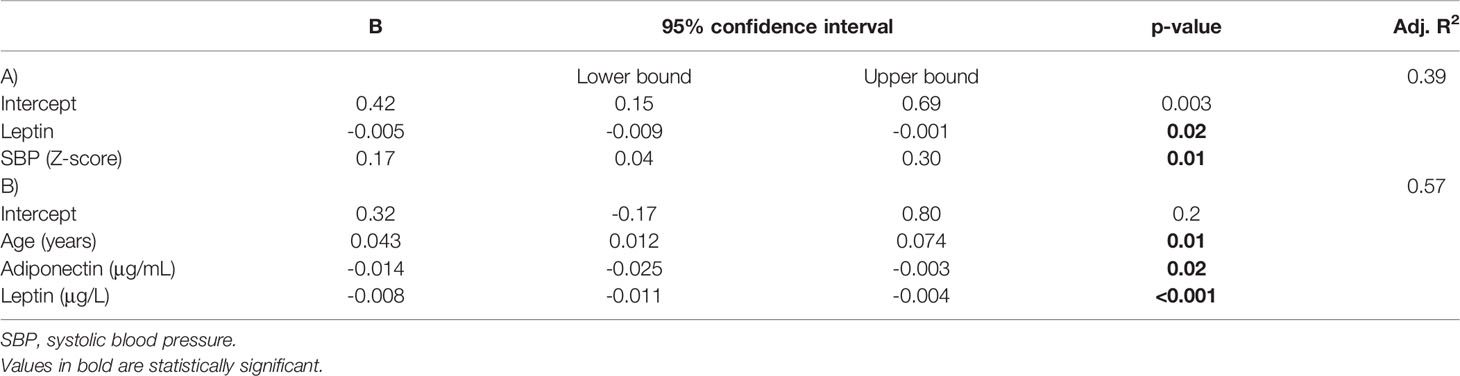
Table 5 Linear regression identifying pre-treatment predictive factors for absolute BMI SDS change (for most patients an increase) (A) and BMI SDS regain (B) after inpatient treatment (n=43).
Subsequently, to correct for the previous BMI SDS reduction, we explored the determinants of the amount of BMI SDS regained in relation to the preceding BMI SDS decrease. The BMI SDS regained 6 months after treatment was associated with age, systolic blood pressure, number of metabolic comorbidities (based on Section 2.4.4), leptin level, and adiponectin level (Table 3) but not with any anthropometric or other metabolic variables (including sleep and endothelial function). There were no sex differences in the amount of BMI SDS regained (p=0.5). A linear regression model was created to predict the relative BMI increase after treatment, including age, number of metabolic risk factors, leptin, and adiponectin. Systolic blood pressure was not included, as this variable was also incorporated into the number of metabolic comorbidities, and the latter correlated more strongly and significantly. After removing the number of metabolic risk factors because of non-significance, the model indicated age, leptin, and adiponectin as significant predictors of this relative BMI increase, which resulted in an explained proportion of the variance of 57% (Table 5). Figure 2 provides an overview of our findings.
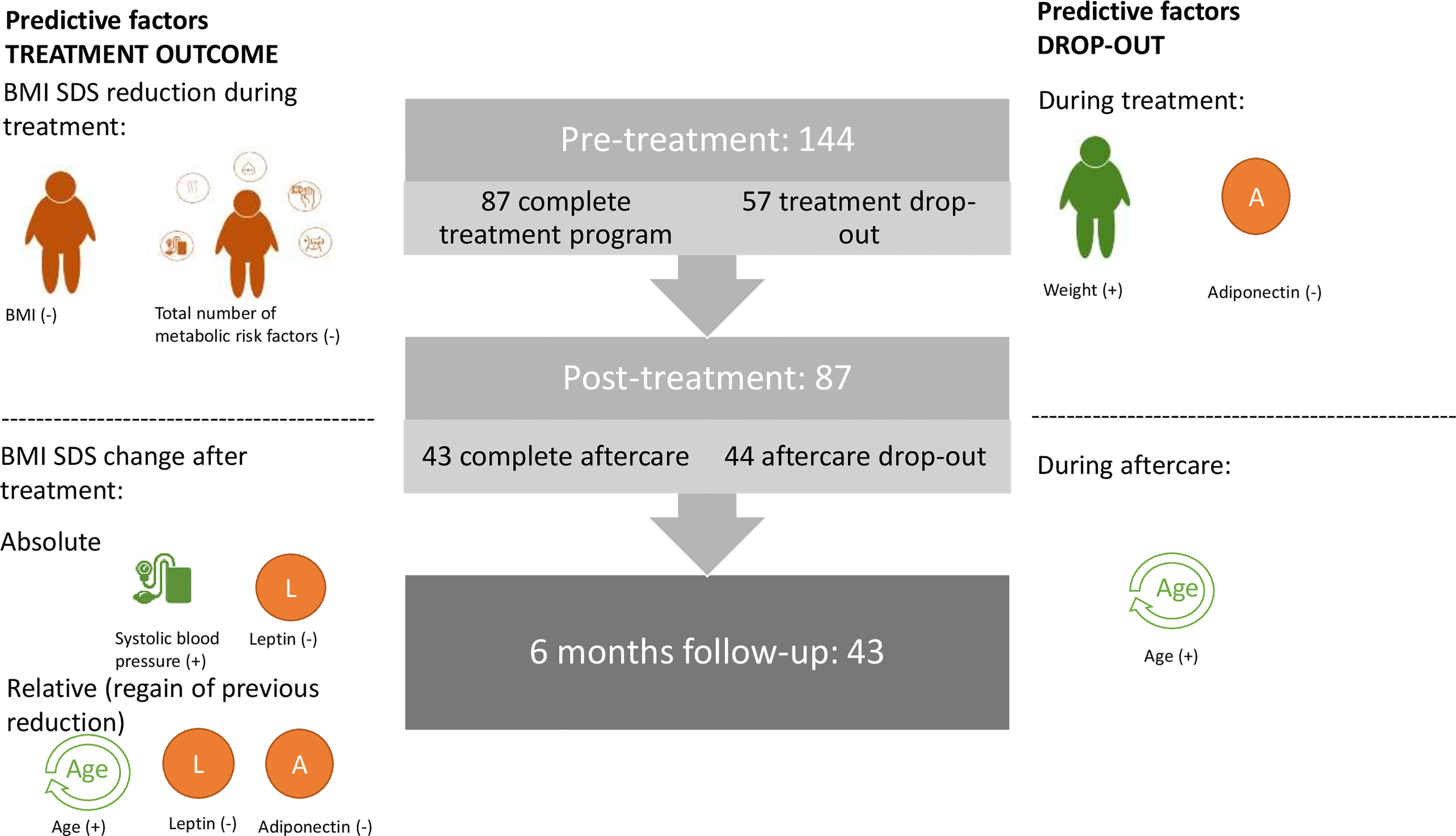
Figure 2 Visual overview of factors predictive for dropout and treatment outcome.The middle panel represents the number of study patients at every visit. The left side depicts the factors predictive of the treatment outcomes. Pre-treatment BMI and total number of metabolic risk factors inversely affected the BMI SDS reduction during treatment. Post-treatment absolute BMI SDS change was predicted by systolic blood pressure (+), expressed as Z-score and baseline leptin (-), whereas post-treatment BMI SDS regain was predicted by age (+), leptin (-), and adiponectin (-). On the right side, factors predictive of dropout during and after treatment were identified. During treatment, higher weight and lower adiponectin levels were risk factors for premature treatment cessation, whereas during aftercare, only those at older age (which coincides with a higher BMI) were found to be at increased risk for dropout. + or – indicates whether the factor is positively or inversely predictive of the dependent variable, that is, treatment outcome or dropout.
4 Discussion
The present study explored the predictive value of baseline patient characteristics, comorbidities, and adipokines regarding treatment dropout and short- and long-term treatment outcome in a large cohort of Belgian children with obesity previously treated for their obesity in a pediatric rehabilitation center.
First, our study showed that children with more severe obesity accompanied by a higher weight, lower adiponectin level, and older age were at higher risk of early (during residential treatment) and late (during aftercare) dropout, consistent with previous studies (40, 41). As premature treatment cessation and dropout during aftercare are risk factors for poorer treatment outcomes (4, 6, 7, 42, 43), our results indicate a pessimistic future for patients with the most severe obesity. As a first step to improving future outcomes, strategies focused on retaining at-risk individuals during treatment and aftercare should be urgently developed. However, there is a lack of studies on the reasons (e.g., the child giving up) behind dropout during inpatient treatment. Other circumstances were parents requesting a return home, expulsion from the program, requirement of psychiatric aid before starting the program, and social reasons. Furthermore, no studies on preventive interventions to retain individuals during inpatient treatment have been conducted, resulting in a lack of knowledge of effective strategies that could be used. Similarly, with regard to dropout during aftercare, no specific interventions have been studied, but here it might be interesting for future research to look at successful interventions in outpatient care (44), such as implementing reminder phone calls, offering an orientation session before treatment start, or sending goal-setting text messages (45–47). Furthermore, due to COVID-19, online healthcare is rapidly evolving and might overcome commonly experienced barriers related to physical distance, (travel) time, or missed work/school (48). Positive results with online care in the context of pediatric obesity have been previously reported for communities facing difficulties with healthcare access (49, 50). As the inpatient treatment center in our study was situated in a remote corner of the country, online interventions might improve aftercare attendance in this setting and are currently being used due to the COVID pandemic.
Second, we identified factors predictive of changes in BMI SDS during and after treatment. This is the first study to report on the influence of the total number of weight-related comorbidities on BMI reduction during treatment. Furthermore, our data indicate that a worse metabolic profile (higher systolic blood pressure, more metabolic risk factors) relates to more post-treatment BMI SDS regain, supporting a previous study reporting individual negative associations between metabolic syndrome markers and treatment outcome (14). Regarding BMI, some studies have confirmed that a higher initial BMI inversely impacts weight loss (51–53), whereas other studies have reported an opposite association (54–58). A possible explanation for these conflicting findings is the observed dropout rate of the most severely obese patients, creating a bias in the population available for analysis. Otherwise, it should be noted that in discrepant studies other BMI metrics were used, such as BMI (expressed in kg/m2 instead of BMI SDS) and adjusted BMI (calculated as [measured BMI of the patient]/[BMI corresponding to percentile 50 for age and sex] × 100), which might change the observed findings, as pointed out previously (39). Indeed, when analyzing the data on BMI (expressed in kg/m2) (instead of BMI SDS), the exact opposite was found (more BMI loss for those with a higher BMI), as shown in Supplementary Table 1, which contributes to the ongoing discussion on which outcome measures should be used. Besides BMI and metabolic comorbidities, age was found to be an independent positive predictor of post-treatment BMI SDS regain. One hypothesis could be the confounding effect of physical activity. Better cardiorespiratory fitness has been positively associated with weight loss during treatment (51), but an inverse relation has been observed between age and physical activity (59–61). These findings again point to the pessimistic prognosis of those participants in the highest need for treatment, even if they complete the entire inpatient treatment and aftercare, and emphasize the importance of guiding these patients and their families to make and maintain changes in the home environment.
Finally, we studied the predictive ability of leptin and adiponectin on weight outcomes during and after obesity treatment. Neither leptin nor adiponectin levels predicted weight loss during inpatient treatment. However, leptin and adiponectin levels were both inversely predictive of weight gain during aftercare. The finding that a high baseline leptin level was associated with less weight regain was unexpected when considering leptin resistance. Here, it should be noted that we only gathered follow-up data from a limited sample of children that were generally younger and less obese. Therefore, these children might have not yet developed resistance to the actions of leptin, as was previously hypothesized by Murer et al. who studied baseline leptin level and its changes in relation to weight loss in a similar population of children with obesity participating in an inpatient treatment program (62). As a result, a higher leptin level promotes satiety, thereby counteracting weight regain. A higher adiponectin level was also found to be predictive of less weight regain after treatment. Adiponectin enhances the body’s insulin sensitivity in peripheral tissues by stimulating glucose transport and fatty acid oxidation (24) and subsequently promoting weight loss (25). Centrally, it acts on the body’s energy expenditure by increasing oxygen consumption and thermogenesis, again promoting weight loss (26). Through these mechanisms, adiponectin may counteract weight regain. An alternative explanation for the inverse association between adiponectin and weight gain can be found in the adipose tissue. Adiponectin production tends to be lower in the visceral abdominal adipose tissue (VAT) compared to the subcutaneous adipose tissue (SAT) (63). Furthermore, a negative correlation between BMI, waist circumference, estimated VAT percentage, and VAT secretion of adiponectin was documented, whereas the state of obesity did not influence the production of adiponectin in the SAT (64). Therefore, it could be hypothesized that obese participants with lower adiponectin levels had more visceral adipose tissue than those with higher adiponectin levels. As weight loss and weight regain tend to alter visceral adipose stores favorably, participants with a low adiponectin level (hence more visceral adipose tissue) would therefore be expected to lose weight more easily but consequently also be more vulnerable to weight regain (65). Thus, a lower adiponectin level could also reflect a higher obesity severity and fit into the previously discussed worse prognosis for the youngsters with the highest initial weight and BMI. As this is one of the first studies to describe the predictive potential of leptin and adiponectin regarding post-treatment weight gain in children with obesity, further research is required to confirm these findings.
Despite adding a new perspective, our results should be considered with the following limitations. The first limitation of our study is that the group attending the last follow-up visit consisted of a limited number of patients, which should be considered when interpreting the results regarding long-term weight regain. However, this limitation provided us with a sufficiently large group for the analysis of dropout cases. Second, we focused on the BMI SDS as an outcome variable, whereas in obesity treatment, the focus was on improving health. When focusing on the health benefits of treatment, this might change the point of view, as the health benefits obtained in residential treatment remain present for a longer period despite weight regain (66, 67). Nevertheless, this state of metabolic healthy obesity is a rather temporal stage before obesity-related comorbidities develop (68). As residential treatment centers are not universally available, most children with obesity seek treatment in outpatient care, where other factors such as travel distance to the center, number of contact hours, and family structure might affect treatment outcomes. Therefore, the current results should not be applied to an outpatient setting but should encourage further research.
In conclusion, children with more severe obesity and more metabolic comorbidities have an increased risk of early dropout in an inpatient obesity treatment program, are more likely to decline participation in aftercare sessions, have an unfavorable outcome during treatment, and regain their lost weight after returning home. Therefore, successful strategies to reduce dropouts during and after treatment and prevent post-treatment weight regain are urgently needed. With the knowledge that younger, less obese patients have better long-term results after inpatient treatment, physicians should consider early referral of these patients to an inpatient treatment setting when outpatient results are insufficient. Additionally, this study identified baseline leptin and adiponectin levels as independent predictors of post-treatment weight gain in children with (previous) obesity. Further research on how adipokines mediate the relationship between adipose tissue and weight is required.
Data Availability Statement
The raw data supporting the conclusions of this article will be made available by the authors, without undue reservation on reviewers’ request.
Ethics Statement
The studies involving human participants were reviewed and approved by Ethics Committee of the Ghent University Hospital (EC n°B670201731779). Written informed consent to participate in this study was provided by the participants’ legal guardian/next of kin.
Author Contributions
The current study was conceptualized by AE, SV, KH, BW, LB, CB, TN, LV and AT. AE, SV, KH, BW and MY designed the methodology. The formal analysis was done by EV. The experiments were performed by MY, AE, SM and EV. AG, MH and AT provided the patients and conducted the clinical follow-up. The original draft was prepared by EV and AE. SV, KM, KH, BW, LB, SM, MY, AG, MH and AT reviewed the original version. AE supervised the whole research activity together with SV, KH, KM and BW. The project administration was executed by AE. The funding for this research project was obtained by LB, SV and BW. All authors contributed to the article and approved the submitted version.
Funding
Funding was received by the Research Foundation – Flanders: FWO TBM project n°150179 and by the Ir. Jozef and Maria de Swerts scholarship of the Royal Academy for Medicine Belgium, but these organizations had no conflict of interest with the results, nor a role in study design, the collection, analysis and interpretation of the data, the writing of the report or the decision to submit the article for publication.
Conflict of Interest
The authors declare that the research was conducted in the absence of any commercial or financial relationships that could be construed as a potential conflict of interest.
Publisher’s Note
All claims expressed in this article are solely those of the authors and do not necessarily represent those of their affiliated organizations, or those of the publisher, the editors and the reviewers. Any product that may be evaluated in this article, or claim that may be made by its manufacturer, is not guaranteed or endorsed by the publisher.
Acknowledgments
We would like to thank the Research Foundation – Flanders and the Royal Academy for Medicine Belgium to provide us with financial support to conduct this research. Furthermore, we would like to thank the staff of ‘Het Zeepreventorium’ and Eddy Basslé specifically to provide a venue to conduct our measurements. Furthermore, I would like to express my thanks to our lab technicians for conducting the ELISAs and we would like to thank Editage (www.editage.com) for English language editing. Lastly, we thank all the children for their participation in this research project.
Supplementary Material
The Supplementary Material for this article can be found online at: https://www.frontiersin.org/articles/10.3389/fendo.2022.822962/full#supplementary-material
References
1. Kent S, Fusco F, Gray A, Jebb SA, Cairns BJ, Mihaylova B. Body Mass Index and Healthcare Costs: A Systematic Literature Review of Individual Participant Data Studies. Obes Rev (2017) 18(8):869–79. doi: 10.1111/obr.12560
2. Karnik S, Kanekar A. Childhood Obesity: A Global Public Health Crisis. Int J Prev Med (2012) 3(1):1–7.
3. Simmonds M, Llewellyn A, Owen CG, Woolacott N. Predicting Adult Obesity From Childhood Obesity: A Systematic Review and Meta-Analysis. Obes Rev (2016) 17(2):95–107. doi: 10.1111/obr.12334
4. Kelly KP, Kirschenbaum DS. Immersion Treatment of Childhood and Adolescent Obesity: The First Review of a Promising Intervention. Obes Rev (2011) 12:37–49. doi: 10.1111/j.1467-789X.2009.00710.x
5. Skelton JA, Beech BM. Attrition in Paediatric Weight Management: A Review of the Literature and New Directions. Obes Rev (2011) 12(5):e273–81. doi: 10.1111/j.1467-789X.2010.00803.x
6. Kalarchian MA, Levine MD, Arslanian SA, Ewing LJ, Houck PR, Cheng Y, et al. Family-Based Treatment of Severe Pediatric Obesity: Randomized, Controlled Trial. Pediatrics (2009) 124(4):1060–8. doi: 10.1542/peds.2008-3727
7. Baum JG, Clark HB, Sandler J. Preventing Relapse in Obesity Through Posttreatment Maintenance Systems: Comparing the Relative Efficacy of Two Levels of Therapist Support. J Behav Med (1991) 14(3):287–302. doi: 10.1007/BF00845456
8. Miller BM, Brennan L. Measuring and Reporting Attrition From Obesity Treatment Programs: A Call to Action! Obes Res Clin Pract (2015) 9(3):187–202. doi: 10.1016/j.orcp.2014.08.007
9. Rank M, Wilks DC, Foley L, Jiang Y, Langhof H, Siegrist M, et al. Health-Related Quality of Life and Physical Activity in Children and Adolescents 2 Years After an Inpatient Weight-Loss Program. J Pediatr (2014) 165(4):732–37.e2. doi: 10.1016/j.jpeds.2014.05.045
10. Van Der Baan-Slootweg O, Benninga MA, Beelen A, van der Palen J, Tamminga-Smeulders C, Tijssen JGP, et al. Inpatient Treatment of Children and Adolescents With Severe Obesity in the Netherlands: A Randomized Clinical Trial. JAMA Pediatr (2014) 168(9):807–14. doi: 10.1001/jamapediatrics.2014.521
11. Cummings D, Henes S, Kolasa K, Olsson J, Collier D. Insulin Resistance Status: Predicting Weight Response in Overweight Children. Arch Pediatr Adolesc Med (2008) 162(8):764–8. doi: 10.1001/archpedi.162.8.764
12. Pinhas-Hamiel O, Lerner-Geva L, Copperman N, Jacobson M. Insulin Resistance and Parental Obesity as Predictors to Response to Therapeutic Life Style Change in Obese Children and Adolescents 10-18 Years Old. J Adolesc Health (2008) 43(5):437–43. doi: 10.1016/j.jadohealth.2008.03.002
13. Madsen K, Garber A, Mietus-Snyder M, Orrell-Valente J, Tran C, Wlasiuk L, et al. A Clinic-Based Lifestyle Intervention for Pediatric Obesity: Efficacy and Behavioral and Biochemical Predictors of Response. J Pediatr Endocrinol Metab (2009) 22(9):805–14. doi: 10.1515/JPEM.2009.22.9.805
14. Uysal Y, Wolters B, Knop C, Reinehr T. Components of the Metabolic Syndrome are Negative Predictors of Weight Loss in Obese Children With Lifestyle Intervention. Clin Nutr (2014) 33(4):620–5. doi: 10.1016/j.clnu.2013.09.007
15. Nishimura R, Sano H, Matsudaira T, Morimoto A, Miyashita Y, Shirasawa T, et al. Changes in Body Mass Index, Leptin and Adiponectin in Japanese Children During a Three-Year Follow-Up Period: A Population-Based Cohort Study. Cardiovasc Diabetol (2009) 8:30. doi: 10.1186/1475-2840-8-30
16. Pejsova H, Hubacek JA, Zemankova P, Zlatohlavek L. Baseline Leptin/Adiponectin Ratio is a Significant Predictor of BMI Changes in Children/Adolescents After Intensive Lifestyle Intervention. Exp Clin Endocrinol Diab (2019) 127(10):691–6. doi: 10.1055/a-0859-7041
17. Reinehr T, Kleber M, De Sousa G, Andler W. Leptin Concentrations are a Predictor of Overweight Reduction in a Lifestyle Intervention. Int J Pediatr Obes (2009) 4(4):215–23. doi: 10.3109/17477160902952464
18. Kelesidis T, Kelesidis I, Chou S, Mantzoros CS. Narrative Review: The Role of Leptin in Human Physiology: Emerging Clinical Applications. Ann Intern Med (2010) 152(2):93–100. doi: 10.7326/0003-4819-152-2-201001190-00008
19. Antunes H, Santos C, Carvalho S. Serum Leptin Levels in Overweight Children and Adolescents. Br J Nutr (2009) 101(8):1262–6. doi: 10.1017/S0007114508055682
20. Kelly AS, Metzig AM, Schwarzenberg SJ, Norris AL, Fox CK, Steinberger J. Hyperleptinemia and Hypoadiponectinemia in Extreme Pediatric Obesity. Metab Syndr Relat Disord (2012) 10(2):123–7. doi: 10.1089/met.2011.0086
21. Izquierdo AG, Crujeiras AB, Casanueva FF, Carreira MC. Leptin, Obesity, and Leptin Resistance: Where are We 25 Years Later? Nutrients (2019) 11(11):2704. doi: 10.3390/nu11112704
22. Klünder-Klünder M, Flores-Huerta S, García-Macedo R, Peralta-Romero J, Cruz M. Adiponectin in Eutrophic and Obese Children as a Biomarker to Predict Metabolic Syndrome and Each of its Components. BMC Public Health (2013) 13(1):88. doi: 10.1186/1471-2458-13-88
23. Stefan N, Bunt JC, Salbe AD, Funahashi T, Matsuzawa Y, Tataranni PA. Plasma Adiponectin Concentrations in Children: Relationships With Obesity and Insulinemia. J Clin Endocrinol Metab (2002) 87(10):4652–6. doi: 10.1210/jc.2002-020694
24. Nigro E, Scudiero O, Monaco ML, Palmieri A, Mazzarella G, Costagliola C, et al. New Insight Into Adiponectin Role in Obesity and Obesity-Related Diseases. BioMed Res Int (2014) 2014:658913. doi: 10.1155/2014/658913
25. Fruebis J. Proteolytic Cleavage Product of 30-kDa Adipocyte Complement-Related Protein Increases Fatty Acid Oxidation in Muscle and Causes Weight Loss in Mice. Proc Natl Acad Sci (2001) 98(4):2005–10. doi: 10.1073/pnas.98.4.2005
26. Qi Y, Takahashi N, Hileman SM, Patel HR, Berg AH, Pajvani UB, et al. Adiponectin Acts in the Brain to Decrease Body Weight. Nat Med (2004) 10(5):524–9. doi: 10.1038/nm1029
27. Magge SN, Goodman E, Armstrong SC, Daniels S, Corkins M, De Ferranti S, et al. The Metabolic Syndrome in Children and Adolescents: Shifting the Focus to Cardiometabolic Risk Factor Clustering. Pediatrics (2017) 140:e20171603. doi: 10.1542/peds.2017-1603
28. Tanghe A, Fondelli T, Van Helvoirt M, Van Roye L, Blanckaert J, Marlein N, et al. Inpatient Treatment of Children and Adolescents With Severe Obesity. Belgian J Pediatr (2020) 22(4):244–8.
29. Roelants M, Hauspie R, Hoppenbrouwers K. References for Growth and Pubertal Development From Birth to 21 Years in Flanders, Belgium. Ann Hum Biol (2009) 36(6):680–94. doi: 10.3109/03014460903049074
30. Cole TJ, Bellizzi MC, Flegal KM, Dietz WH. Establishing a Standard Definition for Child Overweight and Obesity Worldwide: International Survey. Br Med J (2000) 320(7244):1240–3. doi: 10.1136/bmj.320.7244.1240
31. Baker-Smith CM, Flinn SK, Flynn JT, Kaelber DC, Blowey D, Carroll AE, et al. Diagnosis, Evaluation, and Management of High Blood Pressure in Children and Adolescents. Pediatrics (2018) 142:e20182096. doi: 10.1542/peds.2018-2096
33. Vermeiren E, Ysebaert M, Van Hoorenbeeck K, Bruyndonckx L, Van Dessel K, Van Helvoirt M, et al. Comparison of Bioimpedance Spectroscopy and Dual Energy X-Ray Absorptiometry for Assessing Body Composition Changes in Obese Children During Weight Loss. Eur J Clin Nutr (2021) 75(1):73–84. doi: 10.1038/s41430-020-00738-9
34. Daniels S, Benuck I, Christakis D. Expert Panel on Integrated Guidelines for Cardiovascular Health and Risk Reduction in Children an Adolescents: Full Report, 2011. National Heart Lung and Blood Institute (2011).
35. Li C, Ford E, Mokdad A, Cook S. Recent Trends in Waist Circumference and Waist-Height Ratio Among US Children and Adolescents. Pediatrics (2006) 118(5):e1390–8. doi: 10.1542/peds.2006-1062
36. Magge SN, Silverstein J, Elder D, Nadeau K, Hannon TS. Evaluation and Treatment of Prediabetes in Youth. J Pediatr (2020) 219:11–22. doi: 10.1016/j.jpeds.2019.12.061
37. Erman MK, Stewart D, Einhorn D, Gordon N, Casal E. Validation of the ApneaLink for the Screening of Sleep Apnea: A Novel and Simple Single-Channel Recording Device. J Clin Sleep Med (2007) 3(4):387–92. doi: 10.5664/jcsm.26861
38. Bruyndonckx L, Radtke T, Eser P, Vrints CJ, Ramet J, Wilhelm M, et al. Methodological Considerations and Practical Recommendations for the Application of Peripheral Arterial Tonometry in Children and Adolescents. Int J Cardiol (2013) 168(4):3183–90. doi: 10.1016/j.ijcard.2013.07.236
39. Kelly AS, Daniels SR. Rethinking the Use of Body Mass Index Z-Score in Children and Adolescents With Severe Obesity: Time to Kick It to the Curb? J Pediatr (2017) 188:7–8. doi: 10.1016/j.jpeds.2017.05.003
40. Van De Maele K, De Schepper J, Vanbesien J, Van Helvoirt M, De Guchtenaere A, Gies I. Is Vitamin D Deficiency in Obese Youth a Risk Factor for Less Weight Loss During a Weight Loss Program? Endocr Connect (2019) 8(11):1468–73. doi: 10.1530/EC-19-0364
41. Warschburger P, Kröller K. Loss to Follow-Up in a Randomized Controlled Trial Study for Pediatric Weight Management (EPOC). BMC Pediatr (2016) 16(1):1–9. doi: 10.1186/s12887-016-0727-2
42. Adam S, Westenhoefer J, Rudolphi B, Kraaibeek H. Three- and Five-Year Follow-Up of a Combined Inpatient-Outpatient Treatment of Obese Children and Adolescents. Int J Pediatr (2013) 2013:856743. doi: 10.1155/2013/856743
43. Siegrist M, Rank M, Wolfarth B, Langhof H, Haller B, Koenig W, et al. Leptin, Adiponectin, and Short-Term and Long-Term Weight Loss After a Lifestyle Intervention in Obese Children. Nutrition (2013) 29(6):851–7. doi: 10.1016/j.nut.2012.12.011
44. Ball GDC, Sebastianski M, Wijesundera J, Keto-Lambert D, Ho J, Zenlea I, et al. Strategies to Reduce Attrition in Managing Paediatric Obesity: A Systematic Review. Pediatr Obes (2021) 16(4):e12733. doi: 10.1111/ijpo.12733
45. Geer B, Porter RM, Haemer M, Krajicek MJ. Increasing Patient Attendance in a Pediatric Obesity Clinic: A Quality Improvement Project. J Pediatr Nurs (2014) 29(6):528–35. doi: 10.1016/j.pedn.2014.09.001
46. Germann JN, Kirschenbaum DS, Rich BH. Use of an Orientation Session may Help Decrease Attrition in a Pediatric Weight Management Program for Low-Income Minority Adolescents. J Clin Psychol Med Settings (2006) 13:177–87. doi: 10.1007/s10880-006-9015-x
47. de Niet J, Timman R, Bauer S, van den Akker E, de Klerk C, Kordy H, et al. Short Message Service Reduces Dropout in Childhood Obesity Treatment: A Randomized Controlled Trial. Heal Psychol (2012) 31(6):797–805. doi: 10.1037/a0027498
48. Skelton JA, Martin S, Irby MB. Satisfaction and Attrition in Paediatric Weight Management. Clin Obes (2016) 6(2):143–53. doi: 10.1111/cob.12138
49. Irby MB, Boles KA, Jordan C, Skelton JA. TeleFIT: Adapting a Multidisciplinary, Tertiary-Care Pediatric Obesity Clinic to Rural Populations. Telemed e-Health (2012) 18(3):247–9. doi: 10.1089/tmj.2011.0117
50. Cohen GM, Irby MB, Boles K, Jordan C, Skelton JA. Telemedicine and Paediatric Obesity Treatment: Review of the Literature and Lessons Learnt. Clin Obes (2012) 2(3–4):103–11. doi: 10.1111/j.1758-8111.2012.00050.x
51. Mets G, Marissens G, Servayge J, Vandekerckhove K, Wurth B, De Guchtenaere A. Physical Determinants of Weight Loss During a Residential Rehabilitation Program for Adolescents With Obesity. Belgian J Pediatr (2020) 22(4):250–3.
52. Goldschmidt AB, Best JR, Stein RI, Saelens BE, Epstein LH, Wilfley DE. Predictors of Child Weight Loss and Maintenance Among Family-Based Treatment Completers. J Consult Clin Psychol (2014) 82(6):1140–50. doi: 10.1037/a0037169
53. Danielsson P, Kowalski J, Ekblom Ö, Marcus C. Response of Severely Obese Children and Adolescents to Behavioral Treatment. Arch Pediatr Adolesc Med (2012) 166(12):1103–8. doi: 10.1001/2013.jamapediatrics.319
54. Larnkjær A, Høj AR, Bendtsen KM, Mølgaard C, Michaelsen KF. Weight Loss and the Effect on Stature in Children During a Residential Intervention Program. Obesity (2008) 16(12):2652–7. doi: 10.1038/oby.2008.436
55. Reinelt T, Petermann F, Bauer F, Bauer CP. Emotion Regulation Strategies Predict Weight Loss During an Inpatient Obesity Treatment for Adolescents. Obes Sci Pract (2020) 6(3):293–9. doi: 10.1002/osp4.410
56. Goossens L, Braet C, Van Vlierberghe L, Mels S. Weight Parameters and Pathological Eating as Predictors of Obesity Treatment Outcome in Children and Adolescents. Eat Behav (2009) 10(1):71–3. doi: 10.1016/j.eatbeh.2008.10.008
57. Moens E, Braet C, Van Winckel Myriam M. An 8-Year Follow-Up of Treated Obese Children: Children’s, Process and Parental Predictors of Successful Outcome. Behav Res Ther (2010) 48(7):626–33. doi: 10.1016/j.brat.2010.03.015
58. Deforche B, Bourdeaudhuij I, Tanghe A, Debode P, Peter Hills A, Bouckaert J. Role of Physical Activity and Eating Behaviour in Weight Control After Treatment in Severely Obese Children and Adolescents. Acta Paediatr (2007) 94(4):464–70. doi: 10.1111/j.1651-2227.2005.tb01919.x
59. Sember V, Jurak G, Kovač M, Đurić S, Starc G. Decline of Physical Activity in Early Adolescence: A 3-Year Cohort Study. PloS One (2020) 15(3):e0229305. doi: 10.1371/journal.pone.0229305
60. Nader PR, Bradley RH, Houts RM, McRitchie SL, O’Brien M. Moderate-To-Vigorous Physical Activity From Ages 9 to 15 Years. JAMA - J Am Med Assoc (2008) 300(3):295–305. doi: 10.1001/jama.300.3.295
61. Bacil EDA, Mazzardo Júnior O, Rech CR, Legnani RFDS, de Campos W. Physical Activity and Biological Maturation: A Systematic Review. Rev Paul Pediatr (2015) 33(1):114–21. doi: 10.1016/j.rpped.2014.11.003
62. Murer SB, Knöpfli BH, Aeberli I, Jung A, Wildhaber J, Wildhaber-Brooks J, et al. Baseline Leptin and Leptin Reduction Predict Improvements in Metabolic Variables and Long-Term Fat Loss in Obese Children and Adolescents: A Prospective Study of an Inpatient Weight-Loss Program. Am J Clin Nutr (2011) 93(4):695–702. doi: 10.3945/ajcn.110.002212
63. Lihn A, Bruun J, He G, Pedersen S, Jensen P, Richelsen B. Lower Expression of Adiponectin mRNA in Visceral Adipose Tissue in Lean and Obese Subjects. Mol Cell Endocrinol (2004) 219(1–2):9–15. doi: 10.1016/j.mce.2004.03.002
64. Reneau J, Goldblatt M, Gould J, Kindel T, Kastenmeier A, Higgins R, et al. Effect of Adiposity on Tissue-Specific Adiponectin Secretion. PloS One (2018) 13(6):e0198889. doi: 10.1371/journal.pone.0198889
65. Maclean PS, Higgins JA, Giles ED, Sherk VD, Jackman MR. The Role for Adipose Tissue in Weight Regain After Weight Loss. Obes Rev (2015) 16(S1):45–54. doi: 10.1111/obr.12255
66. Vermeiren E, Bruyndonckx L, De Winter B, Verhulst S, Van Eyck A, Van Hoorenbeeck K. The Effect of Weight Regain on Cardiometabolic Health in Children With Obesity: A Systematic Review of Clinical Studies. Nutr Metab Cardiovasc Dis (2021) 31(9):2575–86. doi: 10.1016/j.numecd.2021.05.020
67. Jacobs S, Mylemans E, Ysebaert M, Vermeiren E, De Guchtenaere A, Heuten H, et al. The Impact of Obstructive Sleep Apnea on Endothelial Function During Weight Loss in an Obese Pediatric Population. Sleep Med (2021) 86:48–55. doi: 10.1016/j.sleep.2021.08.002
Keywords: pediatric obesity, weight reduction programs, patient dropout, treatment outcome, adipokines
Citation: Vermeiren E, Van Eyck A, Van De Maele K, Ysebaert M, Makhout S, De Guchtenaere A, Van Helvoirt M, Tanghe A, Naets T, Vervoort L, Braet C, Bruyndonckx L, De Winter B, Verhulst S and Van Hoorenbeeck K (2022) The Predictive Value of Adipokines and Metabolic Risk Factors for Dropouts and Treatment Outcomes in Children With Obesity Treated in a Pediatric Rehabilitation Center. Front. Endocrinol. 13:822962. doi: 10.3389/fendo.2022.822962
Received: 26 November 2021; Accepted: 29 April 2022;
Published: 13 June 2022.
Edited by:
Luís Pedro Rato, Instituto Politécnico da Guarda, PortugalReviewed by:
Deborah Gustafson, SUNY Downstate Medical Center, United StatesDeborah Masquio, Centro Universitário São Camilo, Brazil
Copyright © 2022 Vermeiren, Van Eyck, Van De Maele, Ysebaert, Makhout, De Guchtenaere, Van Helvoirt, Tanghe, Naets, Vervoort, Braet, Bruyndonckx, De Winter, Verhulst and Van Hoorenbeeck. This is an open-access article distributed under the terms of the Creative Commons Attribution License (CC BY). The use, distribution or reproduction in other forums is permitted, provided the original author(s) and the copyright owner(s) are credited and that the original publication in this journal is cited, in accordance with accepted academic practice. No use, distribution or reproduction is permitted which does not comply with these terms.
*Correspondence: Kim Van Hoorenbeeck, a2ltLnZhbmhvb3JlbmJlZWNrQHV6YS5iZQ==