- 1Department of Ophthalmology, Union Hospital, Tongji Medical College, Huazhong University of Science and Technology, Wuhan, China
- 2Department of Endocrinology, Union Hospital, Tongji Medical College, Huazhong University of Science and Technology, Wuhan, China
- 3Department of Biostatistics, School of Public Health, Southern Medical University, Guangzhou, China
Purpose: This study aimed to investigate the causal association between unhealthy lifestyle factors and diabetic retinopathy (DR) risk and to determine better interventions targeting these modifiable unhealthy factors.
Design: Two-sample Mendelian randomization (MR) analysis was performed in this study. The inverse variance-weighted method was used as the primary method.
Method: Our study included 687 single-nucleotide polymorphisms associated with unhealthy lifestyle factors as instrumental variables. Aggregated data on individual-level genetic information were obtained from the corresponding studies and consortia. A total of 292,622,3 cases and 739,241,18 variants from four large consortia (MRC Integrative Epidemiology Unit [MRC-IEU], Genetic Investigation of Anthropometric Traits [GIANT], GWAS & Sequencing Consortium of Alcohol and Nicotine Use [GSCAN], and Neale Lab) were included.
Result: In the MR analysis, a higher body mass index (BMI) (odds ratio [OR], 95% confidence interval [CI] = 1.42, 1.30–1.54; P < 0.001] and cigarettes per day (OR, 95% CI = 1.16, 1.05–1.28; P = 0.003) were genetically predicted to be causally associated with an increased risk of DR, while patients with higher hip circumference (HC) had a lower risk of DR (OR, 95% CI = 0.85, 0.76–0.95; P = 0.004). In the analysis of subtypes of DR, the results of BMI and HC were similar to those of DR, whereas cigarettes per day were only related to proliferative DR (PDR) (OR, 95% CI = 1.18, 1.04–1.33; P = 0.009). In the MR-PRESSO analysis, a higher waist-to-hip ratio (WHR) was a risk factor for DR and PDR (OR, 95% CI = 1.24, 1.02–1.50, P = 0.041; OR, 95% CI = 1.32, 1.01–1.73, P = 0.049) after removing the outliers. Furthermore, no pleiotropy was observed in these exposures.
Conclusion: Our findings suggest that higher BMI, WHR, and smoking are likely to be causal factors in the development of DR, whereas genetically higher HC is associated with a lower risk of DR, providing insights into a better understanding of the etiology and prevention of DR.
1. Introduction
Diabetic retinopathy (DR), a common microvascular complication of diabetes mellitus (DM), remains a leading cause of acquired vision loss in adults of working age and is associated with an increased risk of life-threatening systemic vascular complications (1). Moreover, 35% of individuals with diabetes experience DR, and 10% are in the stage of vision threatening (2). To confront the growing threat,revealing the risk factors of DR is an essential part of implementing interventions for prevention.
Considering unhealthy lifestyle a modifiable factor, mounting observational epidemiological studies have focused on the association between unhealthy lifestyle and DR. However, based on the current studies, the results of some unhealthy lifestyle factors are controversial and inconsistent (3–7). According to a meta-analysis of prospective cohort studies, obesity was a risk factor for non-proliferative DR (5). However, another meta-analysis failed to find any correlation between obesity and DR risk (7). Regarding alcohol intake, different meta-analyses also present controversial results. Chen et al. demonstrated no significant association between alcohol intake and DR (6) whereas Zhu et al. found protective effects in the wine group (3). Due to potential methodological limitations in observational studies, the causal association between such factors and DR can be confused by reverse causality or ethnicity, sex, and age.
Mendelian randomization (MR) analysis utilizes genetic predictors as instrumental variables (IVs) to investigate the causal association between risk factors and diseases (8). Because genetic variants are randomly distributed at conception, each IV is considered an alternative to randomized controlled trials and can avoid various bias commonly presented in traditional observational studies (9). However, most previous MR studies have focused on modified factors and diabetes, and we did not find any study concern about unhealthy lifestyle factors and DR. In addition, the influence of these factors on the different types of DR is uncertain. Thus, based on publicly available data, we performed MR analysis to assess the causal associations between unhealthy lifestyle factors (including smoking, alcohol intake, and obesity) and the risk of DR, including its subtypes.
2. Materials and methods
2.1. Genetic variants associated with unhealthy lifestyle
In this study, smoking, alcohol intake, and obesity were selected as typical examples of unhealthy lifestyle habits. We selected body mass index (BMI) (ukb-a-248), waist circumference (ieu-a-67), hip circumference (HC, ieu-a-55), and waist-to-hip ratio (WHR, ieu-a-79) as indicators of obesity. Single nucleotide variants (single-nucleotide polymorphisms [SNPs]) for BMI were retrieved from the Neale Lab consortium, and the rest were retrieved from a published meta-analysis of genome-wide association studies (GWAS) datasets summarized by Shungin (10) (datasets: ukb-a-248, ieu-a-79, ieu-a-55, ieu-a-67). The SNPs for smoking were obtained from another published meta-analysis of GWAS datasets summarized by Liu (11) and the MRC Integrative Epidemiology Unit (MRC-IEU) consortium (datasets: ieu-b-24, ieu-b-25, ukb-b-20261), including three phenotypes measured by the heaviness of smoking: age of smoking initiation, cigarettes per day, and ever smoked. For alcohol intake, we selected the SNPs of alcoholic drinks per week and alcohol intake frequency from a published meta-analysis of GWAS datasets summarized by Liu (11) and the GWAS & Sequencing Consortium of Alcohol and Nicotine use consortium (datasets: ieu-b-73, ukb-b-5779). All SNPs associated with exposures that reached the GWAS threshold of statistical significance (P < 5 × 10−8) were extracted as IVs. To ensure that they were entirely independent, linkage disequilibrium analysis was performed using a threshold of r2 < 0.001. Additionally, we extracted the summary data and effects of each SNP and calculated the effect sizes and standard errors using the MR-Base platform (12). Details of the data sources are provided in Table 1.
2.2. GWAS summary data on diabetic retinopathy
Published GWAS summary data on DR were retrieved from the MRC-IEU (14,584 cases and 176,010 controls, European ancestry) (https://gwas.mrcieu.ac.uk/) (13). To evaluate potential heterogeneity in the causal effects, we further stratified the DR type for background DR (BDR) and PDR. BDR is an early stage of DR known as non-PDR. We used GWAS summary data of 2026 BDR cases and 8681 PDR cases from the MRC-IEU with 204,208 common controls (Table 1). For each selected SNP, we retrieved summary data (the effects on DR, effect sizes, standard errors, and effect alleles) for the MRC-IEU using the MR-Base platform (12, 14).
2.3. Statistical analyses
We used a two-sample MR approach to examine the potential causal association between unhealthy lifestyle and DR incidence. The MR method must satisfy three assumptions: (i) the IVs need to be robustly related to the exposures; (ii) the instruments influence the outcome only through the exposures of interest; and (iii) the IVs are independent of any confounders of the exposure–outcome association (Figure 1) (15). A flowchart of the statistical analyses is shown in Figure 2.
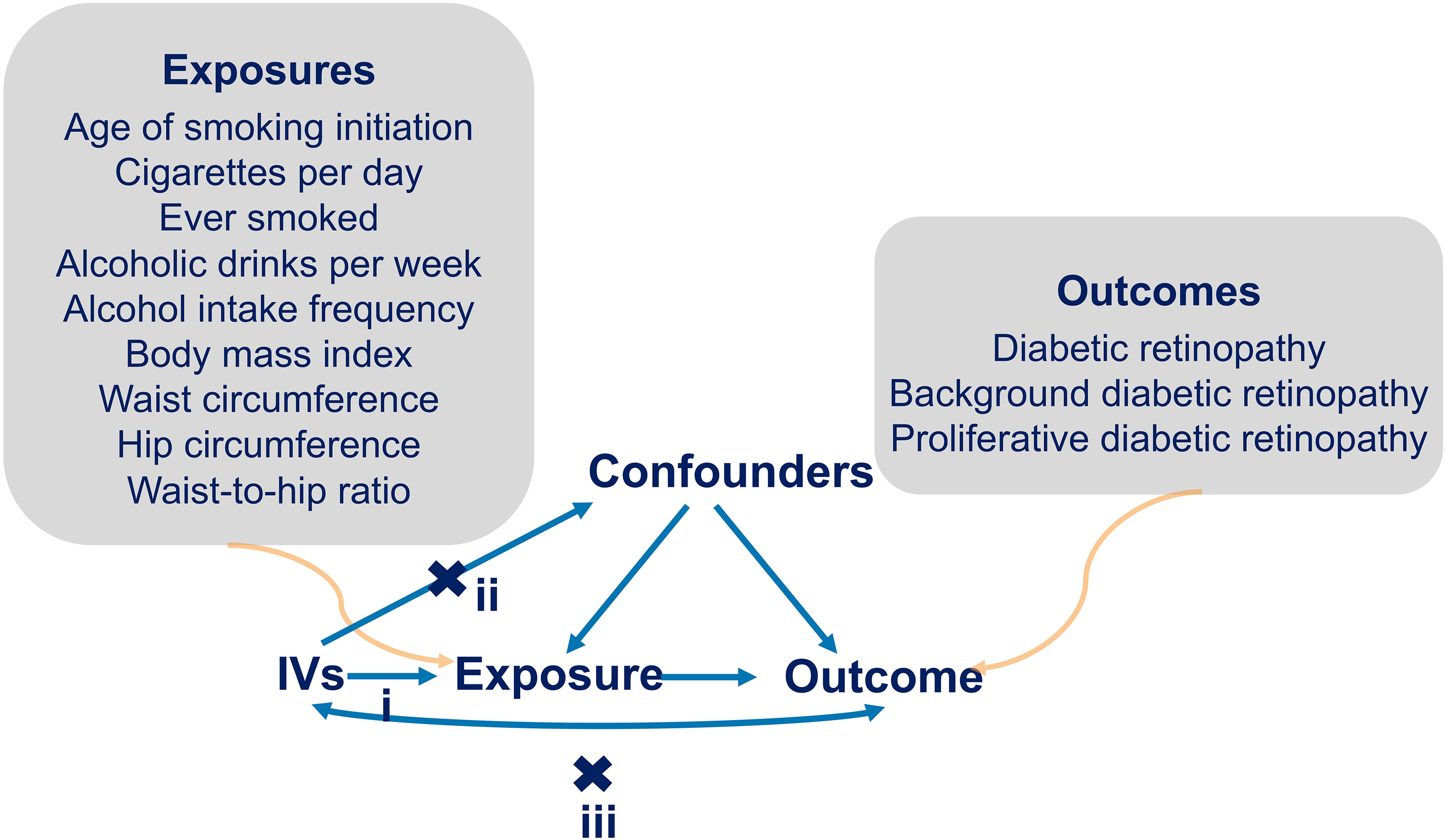
Figure 1 Diagram of MR assumptions. i): represents the IVs need to be related with the exposures robustly. ii): represents the IVs influence the outcome only through the exposures of interest. iii): represents the IVs are independent of any confounders of the exposure-outcome relation. Arrows interpret the causal relationships among variables.
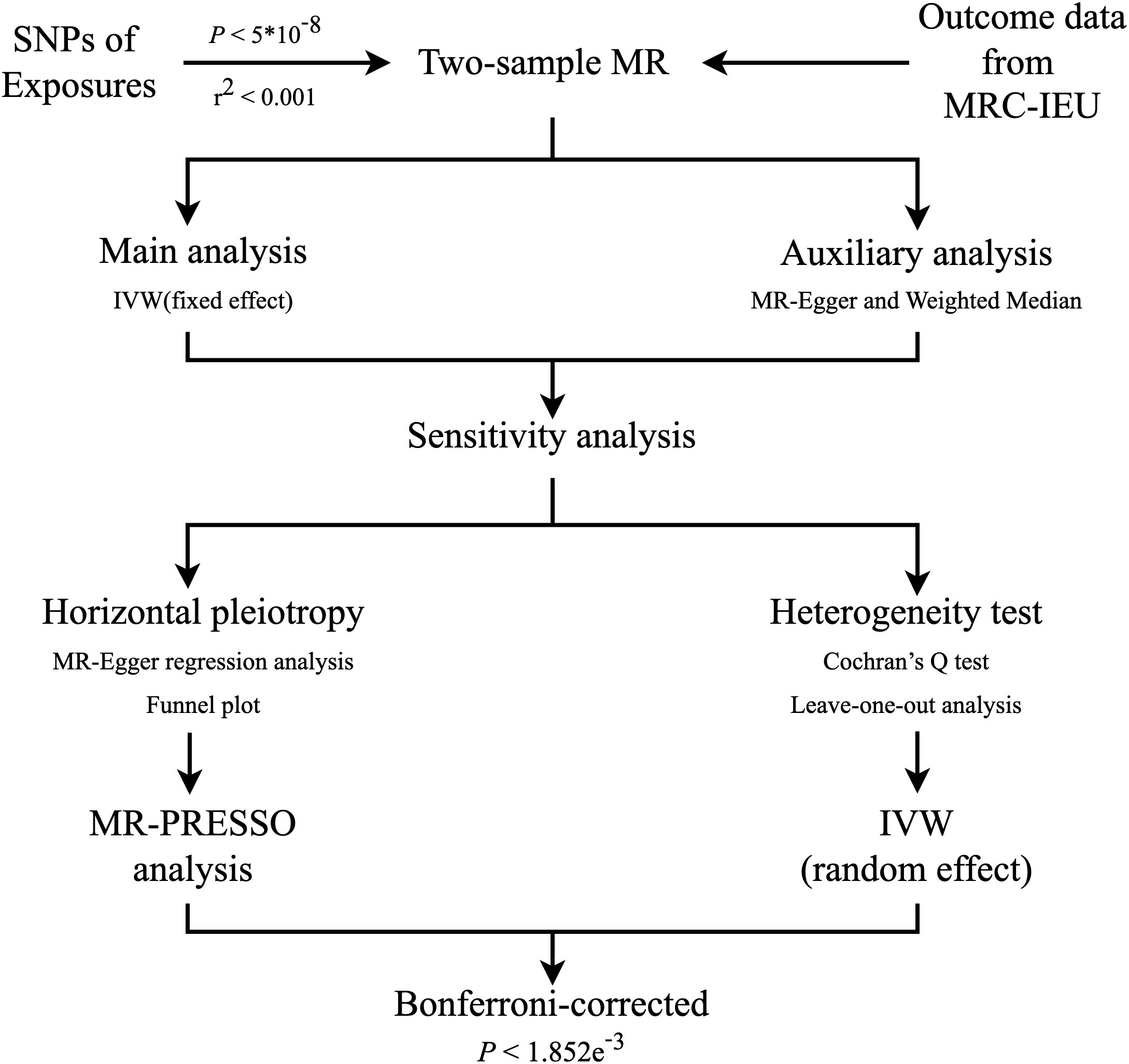
Figure 2 Flowchart of the statistical analyses, outlining the different analyses performed at each stage of the study. SNP, single-nucleotide polymorphism; MR, Mendelian randomization; MRC-IEU, MRC Integrative Epidemiology Unit; IVW, inverse‐variance weighted.
In the MR analyses, the inverse-variance weighted (IVW) model was the main method used in this study. The MR-Egger regression and weighted median (WM) models were also used to test the coherence of the causal estimates. The IVW method treated each SNP as a valid natural experiment, assessing the causal effects of each SNP on the outcome, and used the outcome as weights for meta-analysis to evaluate the combined causal effect. The IVW (fixed-effect) method provides an unbiased estimate in the absence of horizontal pleiotropy or when horizontal pleiotropy is balanced (16). If heterogeneity was present, the multiplicative random-effects IVW model was used and provided valid estimates under the assumption of balanced pleiotropy (17, 18).
In sensitivity analyses, the MR-Egger-intercept test was used to determine pleiotropy. We also applied the MR pleiotropy residual sum and outlier (MR-PRESSO) method to identify outlying SNPs and examine whether the causal effect would change after removing these outliers (19). Cochran’s Q statistic was used to determine the heterogeneity test between individual genetic variants (20). Leave-one-out analysis was performed to evaluate whether MR estimates were driven or biased by a single SNP and to calculate the meta-effect of the remaining SNPs. The asymmetry in funnel plots may indicate that the second assumption is violated by horizontal pleiotropy.
The results were shown as odds ratios (ORs) with their corresponding 95% confidence intervals (CIs). Because we included analyses of nine exposures with three outcomes, using a conservative approach, a Bonferroni-corrected P value < 0.05 divided by 27 (i.e., 1.852e–3) was considered a significant causal association to adjust for multiple testing. A P-value between 0.05 and 1.852e–3 was considered suggestive of a potential association. All statistical analyses were performed in R (version 4.1.0) using the R package “Two sample MR” (version 0.5.6) and “MR- PRESSO” (version 1.0) (12).
3. Results
3.1. Mendelian randomization analysis
3.1.1. Overview of the results
An overview of the MR analysis results is shown in Figure 3. More specific results from the MR analyses are summarized in Figure 4, Table 2, and Tables S2, S3. Detailed information on each SNP is provided in Table S1.
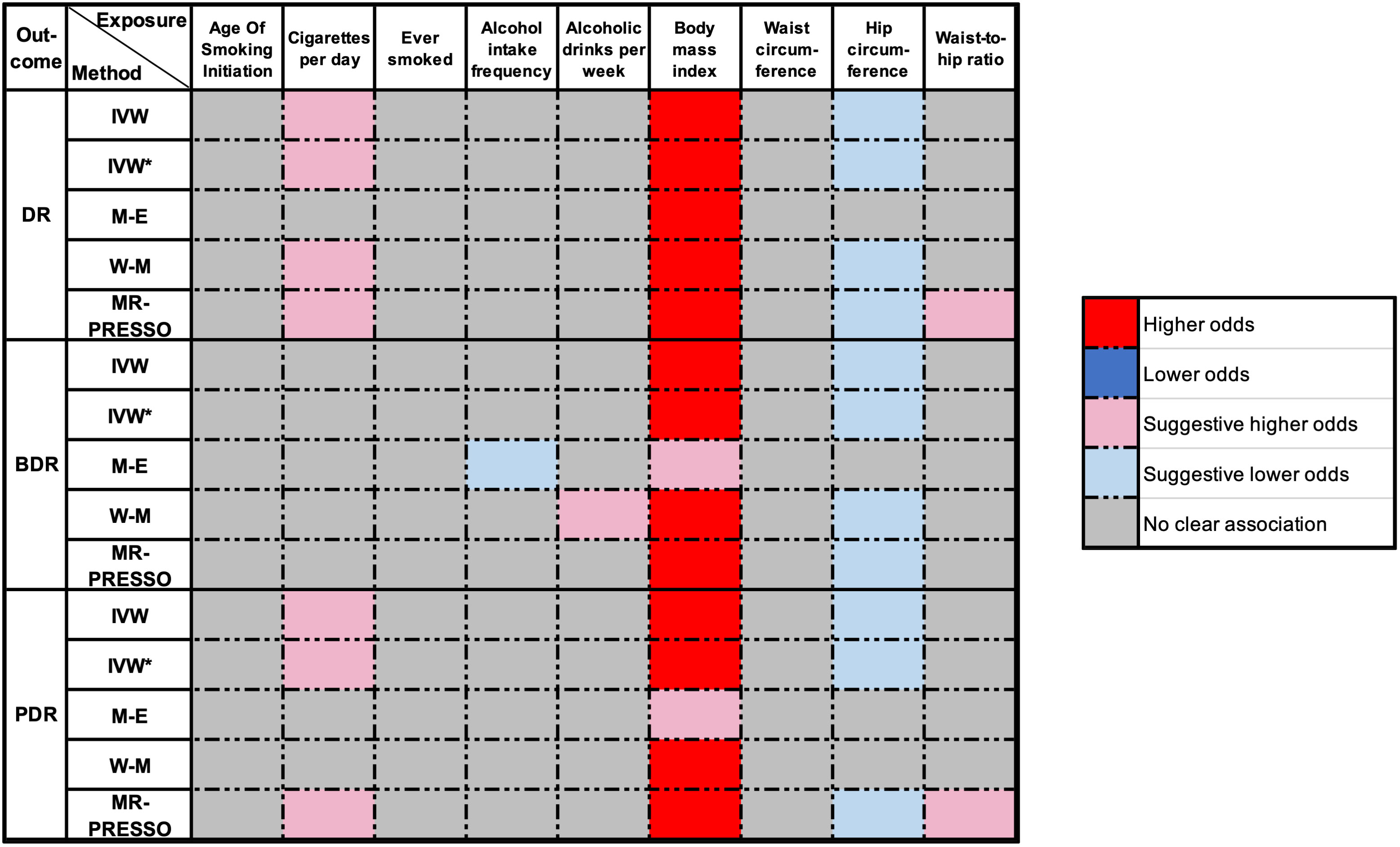
Figure 3 Overview of the main results of MR analyze. DR, diabetic retinopathy; BDR, background diabetic retinopathy; PDR, proliferative diabetic retinopathy; IVW, inverse‐variance weighted (fixed-effect); IVW*, Inverse variance weighted (multiplicative random-effects); MR, Mendelian randomization; M-E, MR Egger; W-M, weighted median; MR-PRESSO, MR Pleiotropy Residual Sum and Outlier. P-value < 1.852e-03 was regarded as a significant causal association, the P-value between 0.05 and 1.852e-03 was considered suggestive of a potential association.
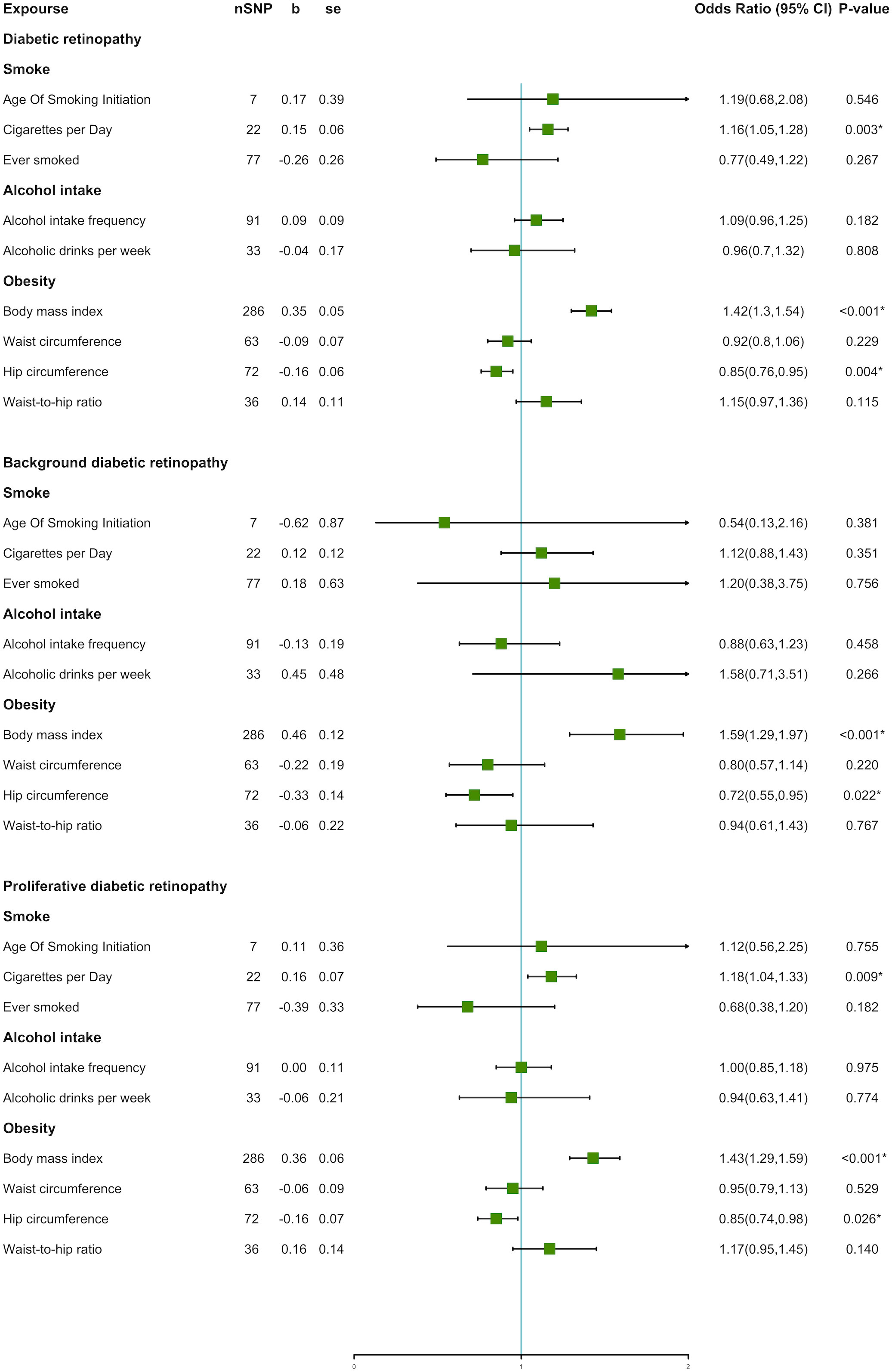
Figure 4 Forest plot of unhealthy lifestyle factors associated with the risk of DR and its subtypes.
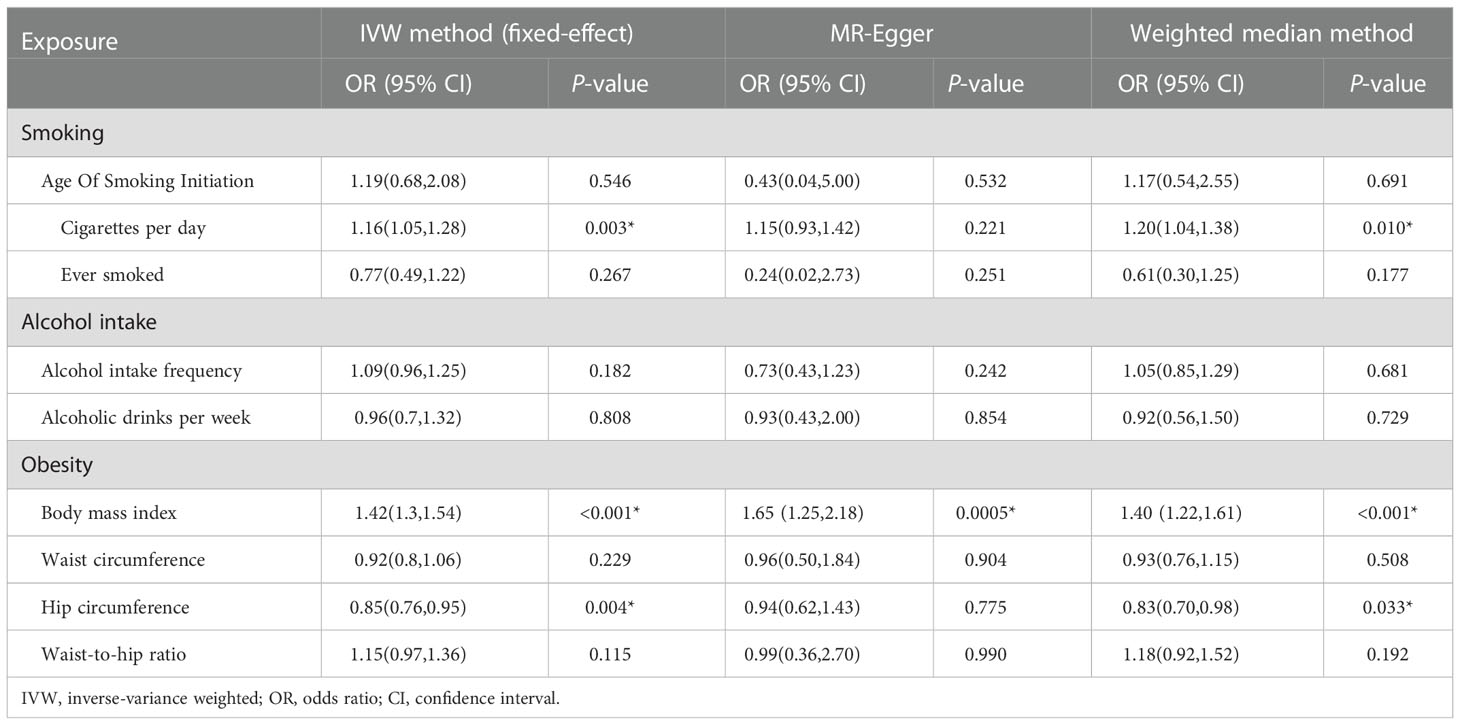
Table 2 Mendelian randomization estimates of the associations between unhealthy lifestyle and risk of DR.
3.1.2. Results of DR
The IVW method indicated an over 40% increased risk of DR incidence per standard deviation (SD) increase in BMI (OR = 1.42; 95% CI, 1.30–1.54; P < 0.001*) and an approximately 20% increase in the risk of cigarettes per day (OR = 1.16; 95% CI, 1.05–1.28; P = 0.003*). Interestingly, higher HC decreased 15% lower risk in DR incidence per SD (OR = 0.85; 95% CI, 0.76–0.95; P = 0.004*). A forest plot of SNPs associated with cigarettes per day, BMI, and HC is presented in Figure S1. The results obtained using the WM method were similar to those obtained using the IVW method (Table 2). In the MR-Egger model, BMI also showed a significant causal effect. However, the MR-Egger model showed a consistent effect direction in cigarettes per day, and HC was not statistically significant (Table 2). We did not observe any association between alcohol intake and DR (Figure 4). We further proceeded with Bonferroni correction, which indicated significant causal effects between BMI and DR risk (P = 9.389e–16).
3.1.3. Results of DR subtypes
In DR subtypes, a genetically predicted higher BMI increased the risk of BDR (OR = 1.59; 95% CI, 1.29–1.97; P < 0.001*) and PDR (OR = 1.43; 95% CI, 1.29–1.59; P < 0.001*). In the WM method and MR-Egger model, BMI also indicated an analogous causal effect. Higher HC was a protective factor in BDR (OR = 0.72; 95% CI, 0.55–0.95; P = 0.022*) and PDR (OR = 0.85; 95% CI, 0.74–0.98; P = 0.026*). The result of cigarettes per day indicated an increased risk of PDR (OR = 1.18; 95% CI, 1.04–1.33; P = 0.009*). The forest plot of SNPs associated with the above exposures and the risks of BDR and PDR are presented in Figure S2. The HC in the BDR using the WM method was also significant. The results of the MR-Egger model and other results of the WM method are presented in Tables S2, S3. We did not find causality in the other factors with BDR and PDR. After Bonferroni correction, a higher BMI still had a significant causal association with BDR and PDR risks (BDR, P = 1.687e–5; PDR, P = 4.085e–11).
3.2. Sensitivity analyses
3.2.1. Sensitivity analyses of DR
We assessed heterogeneity using Cochran’s Q test and horizontal pleiotropy from the MR-Egger regression analysis. There was no evidence of directional pleiotropy using MR-Egger regression (Table 3). Cochran’s Q test for BMI was statistically significant (P < 0.05). Hence, we used a multiplicative random-effects model to re-estimate the MR effect of BMI. The results showed a causal association and positive correlation between BMI and DR risk (P = 4.24e–13*).
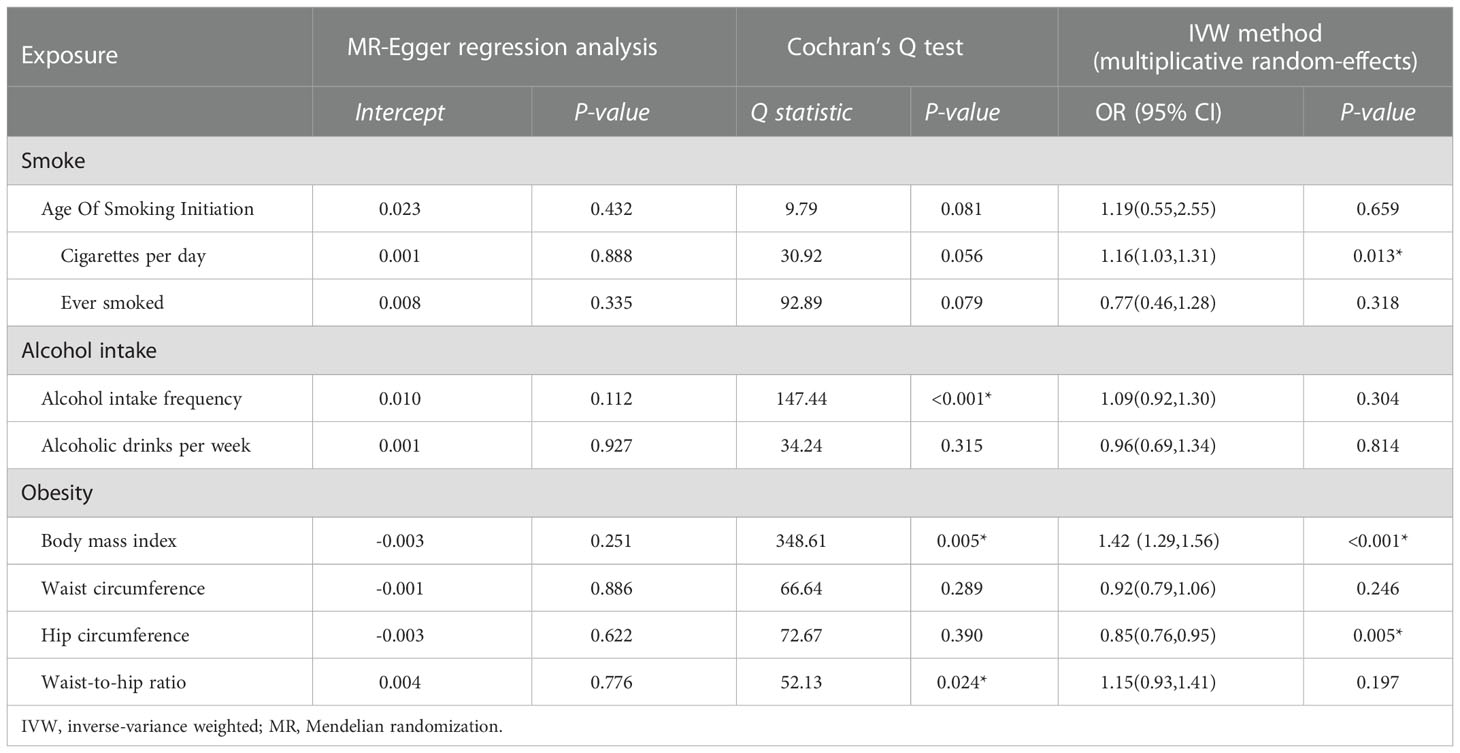
Table 3 Sensitivity test of Mendelian randomization analyze of the associations between unhealthy lifestyle and risk of DR.
3.2.2. Sensitivity analyses of DR subtypes
In DR subtypes, the heterogeneity analysis showed a similar result for BMI (P < 0.05). The results of multiplicative random effects showed that BMI was a causal risk factor for BDR (P = 1.06e–04) and PDR (P = 6.05e–09). Directional pleiotropy was found in alcohol intake frequency in PDR (P = 0.024*) using MR-Egger regression. No evidence of directional pleiotropy was found in the other exposures (Tables S4, S5).
3.2.3. MR-PRESSO analysis
We applied MR-PRESSO to recognize outlying SNPs that might cause horizontal pleiotropy effects. Several outliers were identified during the MR-PRESSO analysis. After removing these outliers, we found that a higher WHR indicated a higher risk of DR (OR = 1.24; 95% CI, 1.02–1.50; P = 0.041) and PDR (OR = 1.32; 95% CI, 1.01–1.73; P = 0.049). In the remaining cases, the results remained consistent with the original results after the removal of these outliers. The corrected results of the MR-PRESSO analysis are listed in Table S6.
3.2.4. Leave-one-out analysis and funnel plot
The leave-one-out analysis suggested that the risk estimates of cigarettes per day, BMI, and HC for DR, BDR, and PDR generally remained consistent after eliminating each single SNP at a time (Figures S3, S4, Table S7). In addition, the points were roughly symmetrical on both sides of the vertical line in funnel plot, which meant no horizontal pleiotropy in the SNPs for shown exposures (Figures S5, S6).
4. Discussion
The present study demonstrated a causal association among cigarettes per day, BMI, and WHR and an increased risk of DR, whereas HC was found to have a lower risk of DR. In subgroup analyses, we found that higher BMI increased the risk of all types of DR, especially BDR. Cigarettes per day was only associated with PDR. Additionally, HC decreased the risks of BDR and PDR. These results were contrary to those of several previous conventional observational studies (3–7). A possible explanation is that the small sample sizes and confounders might interfere with the results of traditional observational epidemiological studies. Here, MR analysis demonstrated an unbiased estimation of whether certain risk factors played a causal role in diseases, using data from published large-scale GWAS data, which made our results more reliable and convincing.
The mechanisms underlying the associations among cigarette smoking, BMI, HC, WHR, and DR remain unclear. In smoking patients, nicotine causes a vasoconstrictive effect. This reduces the blood flow of the retina and makes it difficult for the retinal blood vessels to autoregulate hyperoxia (21, 22). Additionally, smoking may increase carboxyhemoglobin levels (23), thereby reducing the retinal oxygen delivery and oxygen-carrying capacity of the blood. Moreover, mainstream cigarette smoke contains certain components (24) that can interact with plasma and extracellular matrix proteins. This leads to the formation of covalent adducts similar to advanced glycation end products (25, 26) and involved in diabetic end-organ complications.
For obesity, a higher BMI is often associated with dyslipidemia – a disease that may be responsible for the development of DR (27). Moreover, obese individuals with hyperleptinemia (28) are more likely to have higher blood pressure and oxidative stress levels which consequently, are possibly correlated with an increased risk of DR. Additionally, higher levels of the vascular endothelial growth factor found in obese individuals (29) were related to the pathogenesis of PDR (30). For WHR, abdominal obesity played a role in insulin resistance (31) and inflammation (32). Both of these have an association with the pathogenesis of DR (33–35). For HC, the protective effects of a higher HC might result from a larger muscle mass in the gluteofemoral region (36). Different fat deposits exhibit different metabolic properties; lower body fat could somewhat reverse the impact of abdominal fat and protect against insulin resistance (37). As suggested by previous research, for a certain amount of abdominal fat, higher peripheral fat accumulation in the hips and thighs might relate to a better metabolic state (38–42). Additionally, a larger HC might reduce the risk of DR by the contribution of active lipoprotein lipase and the low turnover of fatty acid in gluteofemoral adipose tissues.
The present study has several strengths. First, very few studies have comprehensively investigated the association between unhealthy lifestyles and DR incidence. To the best of our knowledge, our study is the first to investigate the potential causal association between unhealthy lifestyles and the risk of DR employing the MR approach and a large GWASs data. Second, due to the use of MR analysis, our findings were less likely to cause confounding and reverse causality, when compared to those of conventional observational studies. Third, the accuracy of our findings might be higher as we applied analysis of subtypes stratified by clinical classification to investigate the consistency of the pooled effects. Finally, the sample overlap only could account for up to 6.94%, and the exposure and outcome data were obtained from different databases, thereby reducing the bias of the estimate in the direction of observational association. These strengths of MR analysis, as a result, might increase the reliability of our findings.
However, certain limitations were found in our study. The SNPs were obtained from five large European populations-based consortia, which, therefore, might affect the generalization of our findings in other populations and regions. Otherwise, our study only performed MR analysis with summarized statistics. This could only allow us to make a preliminary conclusion on the causal association between unhealthy lifestyles and DR but fail to further investigate this association in terms of age, sex, type of diabetes, and DR severity. Additionally, it is hardly possible to remove all pleiotropy in our studies, especially the correlated horizontal pleiotropy. Consequently, some undetected confounders between exposures and outcomes may bias our results. Therefore, our findings should be cautiously interpreted and confirmed through further studies.
In conclusion, we demonstrate that smoking, BMI, and WHR are risk factors for DR. For future studies, informed by the work reported here, individual-level data and basic science approaches are required to investigate the further mechanism underlying the association between cigarette intake and obesity and DR development. In addition, due to the contrary results of WHR and HC, more emphasis should be placed on how different fat distributions relate to DR, which can disclose the underlying association between obesity and DR and strengthen the understanding of obesity. For clinical practice and public health strategies, the present study highlights the importance of lifestyle management in patients with diabetes and provides a reference for the future refined identification of high-risk individuals for DR. Health education can be strengthened to suggest avoiding unhealthy lifestyles. In addition, more frequent ocular examinations may be essential in patients with diabetes with such risk factors.
Data availability statement
The original contributions presented in the study are included in the article/Supplementary Material. Further inquiries can be directed to the corresponding authors.
Author contributions
FJ and XW were responsible for the concept and design of the study, interpretation of data, and drafting and writing of the article. ZS and ZW were contributed equally to the manuscript as joint first authors. The other authors were responsible for interpretation of data and revision of the intellectual content. All authors contributed to the article and approved the submitted version.
Funding
This work was supported by the National Natural Science Foundation of China (No.81900912). The funding organization had no role in the design or conduct of this study.
Acknowledgments
The authors acknowledge the efforts of the consortia in providing high-quality GWAS resources for researchers. Data and materials are available from the corresponding GWAS consortium. The authors would like to thank the editors and the reviewers for their valuable comments and suggestions to improve the quality of the paper.
Conflict of interest
The authors declare that the research was conducted in the absence of any commercial or financial relationships that could be construed as a potential conflict of interest.
Publisher’s note
All claims expressed in this article are solely those of the authors and do not necessarily represent those of their affiliated organizations, or those of the publisher, the editors and the reviewers. Any product that may be evaluated in this article, or claim that may be made by its manufacturer, is not guaranteed or endorsed by the publisher.
Supplementary material
The Supplementary Material for this article can be found online at: https://www.frontiersin.org/articles/10.3389/fendo.2022.1087965/full#supplementary-material
Abbreviations
AGEs, advanced glycation end-products; BMI, body mass index; BDR, background diabetic retinopathy; CI, confidence interval; DM, diabetes mellitus; DR, diabetic retinopathy; GIANT, Genetic Investigation of ANthropometric Traits; GWAS, genome-wide association studies; GSCAN, GWAS & Sequencing Consortium of Alcohol and Nicotine use; HC, hip circumference; IDF, International Diabetes Federation; IVs, instrumental variables; IVW, inverse-variance weighted; LD, linkage disequilibrium; MR, Mendelian randomization; MRC-IEU, MRC Integrative Epidemiology Unit; MR-PRESSO, MR Pleiotropy Residual Sum and Outlier; NPDR, non-proliferative diabetic retinopathy; OR, odds ratio; PDR, proliferative diabetic retinopathy; SNPs, single-nucleotide polymorphisms; WC, waist circumference; WHR, waist-to-hip ratio; WM, weighted median.
References
1. Cheung N, Mitchell P, Wong TY. Diabetic retinopathy. Lancet (London England). (2010) 376(9735):124–36. doi: 10.1016/S0140-6736(09)62124-3
2. Yau JW, Rogers SL, Kawasaki R, Lamoureux EL, Kowalski JW, Bek T, et al. Global prevalence and major risk factors of diabetic retinopathy. Diabetes Care (2012) 35(3):556–64. doi: 10.2337/dc11-1909
3. Zhu W, Meng YF, Wu Y, Xu M, Lu J. Association of alcohol intake with risk of diabetic retinopathy: a meta-analysis of observational studies. Sci Rep (2017) 7(1):4. doi: 10.1038/s41598-017-00034-w
4. Cai X, Chen Y, Yang W, Gao X, Han X, Ji L. The association of smoking and risk of diabetic retinopathy in patients with type 1 and type 2 diabetes: a meta-analysis. Endocrine (2018) 62(2):299–306. doi: 10.1007/s12020-018-1697-y
5. Zhu W, Wu Y, Meng YF, Xing Q, Tao JJ, Lu J. Association of obesity and risk of diabetic retinopathy in diabetes patients: A meta-analysis of prospective cohort studies. Medicine (2018) 97(32):e11807. doi: 10.1097/MD.0000000000011807
6. Chen C, Sun Z, Xu W, Tan J, Li D, Wu Y, et al. Associations between alcohol intake and diabetic retinopathy risk: a systematic review and meta-analysis. BMC Endocr Disord (2020) 20(1):106. doi: 10.1186/s12902-020-00588-3
7. Zhou Y, Zhang Y, Shi K, Wang C. Body mass index and risk of diabetic retinopathy: A meta-analysis and systematic review. Med (Baltimore). (2017) 96(22):e6754. doi: 10.1097/MD.0000000000006754
8. Davey Smith G, Ebrahim S. What can mendelian randomisation tell us about modifiable behavioural and environmental exposures? BMJ (Clinical Res ed) (2005) 330(7499):1076–9. doi: 10.1136/bmj.330.7499.1076
9. Su Z, Jiang Y, Li C, Zhong R, Wang R, Wen Y, et al. Relationship between lung function and lung cancer risk: a pooled analysis of cohorts plus mendelian randomization study. J Cancer Res Clin Oncol (2021) 147(10):2837–49. doi: 10.1007/s00432-021-03619-1
10. Shungin D, Winkler TW, Croteau-Chonka DC, Ferreira T, Locke AE, Mägi R, et al. New genetic loci link adipose and insulin biology to body fat distribution. Nature (2015) 518(7538):187–96. doi: 10.1038/nature14132
11. Liu M, Jiang Y, Wedow R, Li Y, Brazel DM, Chen F, et al. Association studies of up to 1.2 million individuals yield new insights into the genetic etiology of tobacco and alcohol use. Nat Genet (2019) 51(2):237–44.
12. Hemani G, Zheng J, Elsworth B, Wade KH, Haberland V, Baird D, et al. The MR-base platform supports systematic causal inference across the human phenome. eLife (2018) 7:e34408. doi: 10.7554/eLife.34408
13. Wang Y, McKay JD, Rafnar T, Wang Z, Timofeeva MN, Broderick P, et al. Rare variants of large effect in BRCA2 and CHEK2 affect risk of lung cancer. Nat Genet (2014) 46(7):736–41. doi: 10.1038/ng.3002
14. Elsworth B, Lyon M, Alexander T, Liu Y, Matthews P, Hallett J, et al. The MRC IEU OpenGWAS data infrastructure. bioRxiv (2020). doi: 10.1101/2020.08.10.244293
15. Boef AGC, Dekkers OM, le Cessie S. Mendelian randomization studies: a review of the approaches used and the quality of reporting. Int J Epidemiol. (2015) 44(2):496–511. doi: 10.1093/ije/dyv071
16. Hemani G, Bowden J, Davey Smith G. Evaluating the potential role of pleiotropy in mendelian randomization studies. Hum Mol Genet (2018) 27(R2):R195–r208. doi: 10.1093/hmg/ddy163
17. Bowden J, Spiller W, Del Greco MF, Sheehan N, Thompson J, Minelli C, et al. Improving the visualization, interpretation and analysis of two-sample summary data mendelian randomization via the radial plot and radial regression. Int J Epidemiol (2018) 47(4):1264–78. doi: 10.1093/ije/dyy101
18. Bowden J, Holmes MV. Meta-analysis and mendelian randomization: A review. Res Synth Methods (2019) 10(4):486–96. doi: 10.1002/jrsm.1346
19. Verbanck M, Chen C-Y, Neale B, Do R. Detection of widespread horizontal pleiotropy in causal relationships inferred from mendelian randomization between complex traits and diseases. Nat Genet (2018) 50(5):693–8. doi: 10.1038/s41588-018-0099-7
20. Greco M FD, Minelli C, Sheehan NA, Thompson JR. Detecting pleiotropy in mendelian randomisation studies with summary data and a continuous outcome. Stat Med (2015) 34(21):2926–40. doi: 10.1002/sim.6522
21. Morgado PB, Chen HC, Patel V, Herbert L, Kohner EM. The acute effect of smoking on retinal blood flow in subjects with and without diabetes. Ophthalmology (1994) 101(7):1220–6. doi: 10.1016/S0161-6420(94)31185-7
22. Costa F, Soares R. Nicotine: a pro-angiogenic factor. Life Sci (2009) 84(23-24):785–90. doi: 10.1016/j.lfs.2009.03.002
23. Leone A. Biochemical markers of cardiovascular damage from tobacco smoke. Curr Pharm Des (2005) 11(17):2199–208. doi: 10.2174/1381612054367391
24. Nicholl ID, Bucala R. Advanced glycation endproducts and cigarette smoking. Cell Mol Biol (Noisy-le-Grand France). (1998) 44(7):1025–33.
25. Brownlee M, Vlassara H, Cerami A. Nonenzymatic glycosylation and the pathogenesis of diabetic complications. Ann Internal Med (1984) 101(4):527–37. doi: 10.7326/0003-4819-101-4-527
26. Wautier JL, Guillausseau PJ. Advanced glycation end products, their receptors and diabetic angiopathy. Diabetes Metab (2001) 27(5 Pt 1):535–42.
27. Chew EY, Davis MD, Danis RP, Lovato JF, Perdue LH, Greven C, et al. The effects of medical management on the progression of diabetic retinopathy in persons with type 2 diabetes: the action to control cardiovascular risk in diabetes (ACCORD) eye study. Ophthalmology (2014) 121(12):2443–51. doi: 10.1016/j.ophtha.2014.07.019
28. Considine RV, Sinha MK, Heiman ML, Kriauciunas A, Stephens TW, Nyce MR, et al. Serum immunoreactive-leptin concentrations in normal-weight and obese humans. New Engl J Med (1996) 334(5):292–5. doi: 10.1056/NEJM199602013340503
29. Miyazawa-Hoshimoto S, Takahashi K, Bujo H, Hashimoto N, Saito Y. Elevated serum vascular endothelial growth factor is associated with visceral fat accumulation in human obese subjects. Diabetologia (2003) 46(11):1483–8. doi: 10.1007/s00125-003-1221-6
30. Aiello LP, Avery RL, Arrigg PG, Keyt BA, Jampel HD, Shah ST, et al. Vascular endothelial growth factor in ocular fluid of patients with diabetic retinopathy and other retinal disorders. New Engl J Med (1994) 331(22):1480–7. doi: 10.1056/NEJM199412013312203
31. Fujimoto WY, Abbate SL, Kahn SE, Hokanson JE, Brunzell JD. The visceral adiposity syndrome in Japanese-American men. Obes Res (1994) 2(4):364–71. doi: 10.1002/j.1550-8528.1994.tb00076.x
32. Panagiotakos DB, Pitsavos C, Yannakoulia M, Chrysohoou C, Stefanadis C. The implication of obesity and central fat on markers of chronic inflammation: The ATTICA study. Atherosclerosis (2005) 183(2):308–15. doi: 10.1016/j.atherosclerosis.2005.03.010
33. Anan F, Takayuki M, Takahashi N, Nakagawa M, Eshima N, Saikawa T, et al. Diabetic retinopathy is associated with insulin resistance and cardiovascular autonomic dysfunction in type 2 diabetic patients. Hypertens Res (2009) 32(4):299–305. doi: 10.1038/hr.2009.8
34. Maneschi F, Mashiter K, Kohner EM. Insulin resistance and insulin deficiency in diabetic retinopathy of non-insulin-dependent diabetes. Diabetes (1983) 32(1):82–7. doi: 10.2337/diab.32.1.82
35. Chaturvedi N, Sjoelie AK, Porta M, Aldington SJ, Fuller JH, Songini M, et al. Markers of insulin resistance are strong risk factors for retinopathy incidence in type 1 diabetes. Diabetes Care (2001) 24(2):284–9. doi: 10.2337/diacare.24.2.284
36. Manolopoulos KN, Karpe F, Frayn KN. Gluteofemoral body fat as a determinant of metabolic health. Int J Obes (Lond). (2010) 34(6):949–59. doi: 10.1038/ijo.2009.286
37. Snijder MB, Dekker JM, Visser M, Bouter LM, Stehouwer CD, Kostense PJ, et al. Associations of hip and thigh circumferences independent of waist circumference with the incidence of type 2 diabetes: the hoorn study. Am J Clin Nutr (2003) 77(5):1192–7. doi: 10.1093/ajcn/77.5.1192
38. Williams MJ, Hunter GR, Kekes-Szabo T, Snyder S, Treuth MS. Regional fat distribution in women and risk of cardiovascular disease. Am J Clin Nutr (1997) 65(3):855–60. doi: 10.1093/ajcn/65.3.855
39. Van Pelt RE, Evans EM, Schechtman KB, Ehsani AA, Kohrt WM. Contributions of total and regional fat mass to risk for cardiovascular disease in older women. Am J Physiol Endocrinol Metab (2002) 282(5):E1023–8. doi: 10.1152/ajpendo.00467.2001
40. Tankó LB, Bagger YZ, Alexandersen P, Larsen PJ, Christiansen C. Peripheral adiposity exhibits an independent dominant antiatherogenic effect in elderly women. Circulation (2003) 107(12):1626–31. doi: 10.1161/01.CIR.0000057974.74060.68
41. Ferreira I, Snijder MB, Twisk JW, van Mechelen W, Kemper HC, Seidell JC, et al. Central fat mass versus peripheral fat and lean mass: opposite (adverse versus favorable) associations with arterial stiffness? the Amsterdam growth and health longitudinal study. J Clin Endocrinol Metab (2004) 89(6):2632–9. doi: 10.1210/jc.2003-031619
Keywords: Mendelian randomization, diabetic retinopathy, unhealthy lifestyle factors, obesity, smoking, alcohol intake
Citation: Su Z, Wu Z, Liang X, Xie M, Xie J, Li H, Wang X and Jiang F (2023) Diabetic retinopathy risk in patients with unhealthy lifestyle: A Mendelian randomization study. Front. Endocrinol. 13:1087965. doi: 10.3389/fendo.2022.1087965
Received: 02 November 2022; Accepted: 28 December 2022;
Published: 17 January 2023.
Edited by:
Mohd Imtiaz Nawaz, Department of Ophthalmology, College of Medicine, King Saud University, Saudi ArabiaReviewed by:
Jeena Gupta, Lovely Professional University, IndiaKei Hang Katie Chan, City University of Hong Kong, Hong Kong SAR, China
Copyright © 2023 Su, Wu, Liang, Xie, Xie, Li, Wang and Jiang. This is an open-access article distributed under the terms of the Creative Commons Attribution License (CC BY). The use, distribution or reproduction in other forums is permitted, provided the original author(s) and the copyright owner(s) are credited and that the original publication in this journal is cited, in accordance with accepted academic practice. No use, distribution or reproduction is permitted which does not comply with these terms.
*Correspondence: Fagang Jiang, fgjiang@hotmail.com; Xinghua Wang, xinghua_wang@hust.edu.cn
†These authors have contributed equally to this work and share first authorship