- 1Department of Neuroradiology, Klinikum rechts der Isar, School of Medicine, Technical University of Munich, Munich, Germany
- 2TUM-NIC, Technical University of Munich Neuroimaging Center, Munich, Germany
- 3Department of Psychology, University of Warwick, Coventry, United Kingdom
- 4Department of Psychology, Bielefeld University, Bielefeld, Germany
- 5Clinical Functional Imaging Group, Department of Diagnostic and Interventional Radiology, University Hospital Bonn, Bonn, Germany
- 6Department of Neonatology, University Hospital Bonn, Bonn, Germany
- 7Department of Neonatology, Klinikum rechts der Isar, School of Medicine, Technical University of Munich, Munich, Germany
- 8Department of Psychiatry and Psychotherapy, Klinikum rechts der Isar, School of Medicine, Technical University of Munich, Munich, Germany
- 9Neuropsychiatry, Charité - Universitätsmedizin Berlin and German Center for Neurodegenerative Diseases (DZNE), Berlin, Germany
- 10UK Dementia Research Institute, University of Edinburgh (UK DRI), Edinburgh, United Kingdom
- 11Warwick Medical School, University of Warwick, Coventry, United Kingdom
Introduction: Preterm birth is associated with an increased risk for impaired body weight gain. While it is known that in prematurity several somatic and environmental factors (e.g., endocrine factors, nutrition) modulate short- and long-term body weight gain, the contribution of potentially impaired body weight control in the brain remains elusive. We hypothesized that the structure of hypothalamic nuclei involved in body weight control is altered after preterm birth, with these alterations being associated with aberrant body weight development into adulthood.
Materials and methods: We assessed 101 very preterm (i.e., <32 weeks of gestational age) and/or very low birth weight (i.e., <1500g; VP/VLBW) and 110 full-term born (FT) adults of the population-based Bavarian Longitudinal Study with T1-weighted MRI, deep learning-based hypothalamus subunit segmentation, and multiple body weight assessments from birth into adulthood.
Results: Volumes of the whole hypothalamus and hypothalamus subunits relevant for body weight control were reduced in VP/VLBW adults and associated with birth variables (i.e., gestational age and intensity of neonatal treatment), body weight (i.e., weight at birth and adulthood), and body weight trajectories (i.e., trajectory slopes and cluster/types such as long-term catch-up growth). Particularly, VP/VLBW subgroups, whose individuals showed catch-up growth and/or were small for gestational age, were mostly associated with volumes of distinct hypothalamus subunits such as lateral or infundibular/ventromedial hypothalamus.
Conclusion: Results demonstrate lower volumes of body weight control-related hypothalamus subunits after preterm birth that link with long-term body weight gain. Data suggest postnatal development of body weight -related hypothalamic nuclei in VP/VLBW individuals that corresponds with distinct body weight trajectories into adulthood.
1 Introduction
Preterm birth is defined as birth before 37 weeks of gestational age (GA) and is frequent with a worldwide prevalence of about 11% (1). Preterm birth is associated with increased risks for somatic, behavioral, and neuro-cognitive impairments, such as metabolic or cardio-vascular disorders (2, 3) or lower IQ (4, 5). Concerning metabolic impairments, preterm birth elevates the risk for impaired body weight gain, with increasing risks for those born very preterm (i.e., <32 GA) and/or with very low birth weight (i.e., <1500g; VP/VLBW); impaired postnatal to long-term body weight gain is broadly defined as the failure to achieve the body weight gain-potential expected for an individual at a certain age (6–8). While recent studies suggest that progress in modern neonatal management contributes to overcome postnatal differences in body height of VP/VLBW compared to full-term (FT; i.e., ≥37 GA and ≥1500g) born infants until childhood, body weight differences are not yet compensated (9). Particularly, VP/VLBW infants born below the 10th percentile of weight for their gestational age (i.e., born small for gestational age, SGA) have larger hazards for impaired postnatal body weight gain and/or impaired long-term catch-up growth, which, in turn, is associated with increased risks for long-term morbidity (8, 10, 11). Indeed, impaired body weight gain of, for example, preterm infants, links with increased risks for adverse outcomes - from neurodevelopmental aberrations to aberrant glucose tolerance and diabetes mellitus-type-II (12–14). Moreover, impaired body weight gain represents a complex and multifactorial condition modified by maternal, genetic, fetal, environmental, nutritional, stress-related, and endocrine factors (10, 11, 15). At the same time, also rapid gain of body weight during infancy (early catch-up growth), especially for those being SGA, is seen as an independent risk factor for e.g., obesity or hypertension later in life (11, 16–18). While our knowledge about environmental to somatic factors is remarkable, we still do not know, however, whether altered brain mechanisms of body weight control are also associated with aberrant body weight development after preterm birth.
The hypothalamus is critically involved in growth control, including control of body weight (19–21). It is a highly conserved brain structure across vertebrates, surrounding the infundibular recess of the forebrain’s third ventricle and consisting of at least 13 interconnected nuclei (22). Although neurogenesis of the hypothalamus starts already during gestational week 9 in humans (23), its development, ranging from neuronal migration, axon extension, dendritic arborization and synaptogenesis to myelination and epigenetic modifications, is not terminated prenatally, but includes subsequent postnatal development (24). It is known that the hypothalamus controls a variety of basic physiological-behavioral processes, from circadian rhythms to drinking, feeding, sexual, and threat behavior (21). In particular, several hypothalamic nuclei, including the paraventricular (PVN), infundibular (INF), dorso-/ventromedial nucleus (DM/VM), and the lateral hypothalamus (LH), are specifically involved in the control of appetite, food intake, and growth, including body weight (20, 25, 26). While most evidence about hypothalamic functions stems from animal studies (e.g., (27)), neuroimaging studies in humans have linked altered hypothalamic structure with impaired body weight control e.g., in obesity and anorexia nervosa (28, 29).
In-vivo imaging of the hypothalamus at a nuclear level in humans is challenging due to hypothalamus small size of about 1cm³ and surrounding gray matter (30, 31). It therefore requires high spatial resolution imaging and, particularly, optimal delineation methodology. While previous approaches on delineation relied on manual, semi-automated or automated Bayesian or multi-atlas segmentation techniques (see overview in (32)), recent improvements in deep learning-based segmentation (i.e., automated deep convolutional neural networks) (33) enable highly reliable hypothalamus delineation, sensitive to inter-individual differences and, most importantly, sensitive to the identification of hypothalamus subsegments that can be mapped on nuclei that are relevant for body weight control (Figure 1).
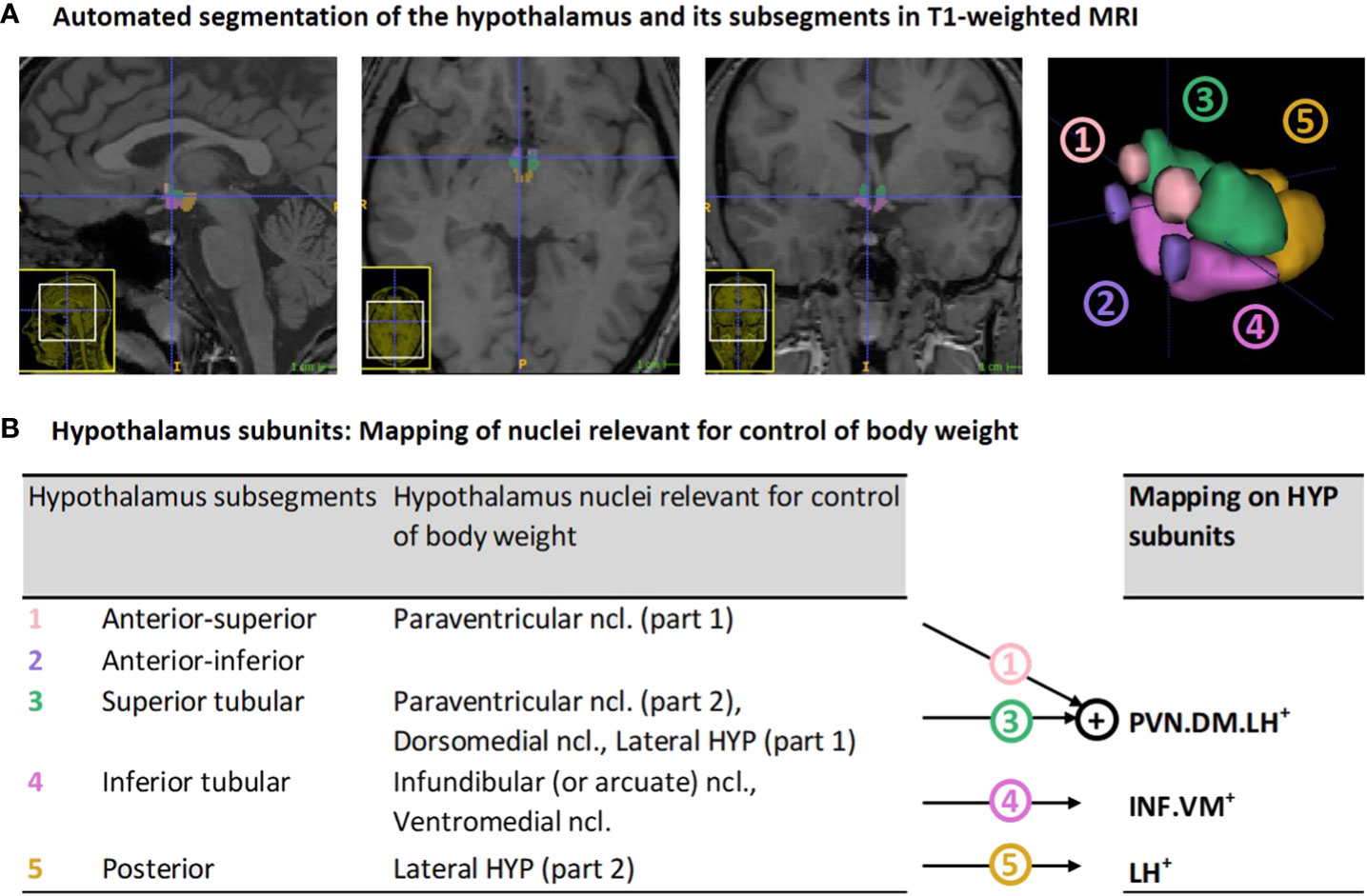
Figure 1 Hypothalamus segmentation and mapping of nuclei relevant for control of body weight on segmentation-based subunits: (A) Representative segmentation of the hypothalamus of a VP/VLBW adult via deep convolutional neural network algorithm in T1-weighted MRI. From left to right: sagittal, axial, coronal view, and 3D rendering of the whole hypothalamus and its subsegments. (B) Mapping of segmentation-based hypothalamic subsegments that contain nuclei relevant for control of body weight (segmentation algorithm based on Billot et al. (33)) onto three newly defined subunits of body weight control, namely PVN.DM.LH+, INF.VM+ and LH+. HYP, hypothalamus; MRI, magnetic resonance imaging; VP/VLBW, very preterm and/or very low birth weight.
We assessed the role of the hypothalamus in body weight control in VP/VLBW individuals by addressing the following hypotheses: After preterm birth, (i) the structure of whole hypothalamus and hypothalamic nuclei involved in body weight control is altered, and (ii) these alterations are associated (a) with aberrant body weight development into adulthood, particularly in individuals (b) with long-term catch-up growth and (c) born SGA. To test these hypotheses, we assessed 101 VP/VLBW and 110 FT adults with T1-weighted MRI, deep learning-based hypothalamus segmentation, hypothalamic volume as proxy for hypothalamic structure, and multiple body weight assessments, including both slope and cluster/type analysis of long-term body weight trajectories, from birth to adulthood.
2 Materials and methods
2.1 Participants
Data for this study were derived from the Bavarian Longitudinal Study, BLS, a prospectively geographically defined whole population study of neonatal at-risk VP/VLBW and FT individuals, described in detail in (34–36) and in the Supplement. Briefly, neonatal at-risk children and healthy controls from southern Bavaria were included between January 1985 and March 1986. 682 infants born VP/VLBW and 350 of 916 randomly selected FT controls from the same obstetric hospitals were enrolled and matched for sex and socioeconomic status. Of the initial 682 VP/VLBW infants, 411 were eligible for the 26-year follow-up assessment, and 260 (63.3%) participated in psychological assessments (37). Of the initial 350 FT born infants, 308 were eligible for the 26-year follow-up assessment, and 229 (74.4%) participated in psychological assessments. Of the sample assessed in adulthood, 101 VP/VLBW and 111 FT individuals underwent MRI at 26 years of age. MR-related exclusion criteria included (self-reported) claustrophobia, inability to lie still for > 30min, unstable medical conditions (e.g., severe asthma), epilepsy, tinnitus, pregnancy, non-removable MRI-incompatible metal implants, and a history of severe central nervous system (CNS) trauma or disease that would impair further analysis of the data. The most frequent reason not to perform the MRI exam, however, was lack of motivation. Supplement Figure S1 shows participant flowchart. For comparability of MRI-assessed VP/VLBW and FT groups with respect to brain abnormalities such as ventricle enlargement or neonatal intraventricular hemorrhage, see Supplement Methods and Table S1. MRI took place at two sites, namely Department of Neuroradiology, Klinikum rechts der Isar, Technical University of Munich (n=146), and Department of Radiology, University Hospital of Bonn (n=66).
2.2 Birth-related variables, neurocognitive assessment, and body weight development
We performed canonical measurements of birth-related variables (gestational age (GA), medical treatment at birth, family socioeconomic status (SES)), full-scale intelligence quotient (FS-IQ; for neurocognitive assessment), and body weight development, described in detail previously (36) and in the Supplement. Briefly, GA was estimated from maternal reports on the first day of the last menstrual period and from serial ultrasounds during pregnancy. To estimate medical impairments at birth, Intensity of Neonatal Treatment Index (INTI) was calculated via daily assessments of care level, respiratory support, feeding dependency, and neurological status (including mobility, muscle tone, and neurological excitability; Supplementary Table S2 for variable description). Family socioeconomic status (SES) at birth was collected through structured parental interviews within 10 days of childbirth. It was computed as a weighted composite score based on the profession of the self-identified head of each family together with the highest educational qualification held by either parent (38). Participants’ neurocognitive functioning at 26 years of age was assessed using the short version of the German Wechsler Adults Intelligence Scale, Third edition (WAIS-III) (39). The assessment took place prior to and independent of the MRI scan and was carried out by trained psychologists who were blinded to group membership. Consecutively, full-scale intelligence quotient (FS-IQ) performance was computed.
Longitudinal body weight measurements were undertaken at birth, at five and 20 months corrected for prematurity, at 56 months, 6, 8 and 26 years of chronological age, using predefined protocols (40). For body weight development analysis, we transformed body weight measurements from [g]/[kg] into z-scores relative to the exact age of each participant, typically used for the description of longitudinal changes of growth. Applied references for calculation of z-scores are described in the Supplement methods. “Small for gestational age” (SGA) versus “appropriate/large for gestational age” (AGA/LGA) was determined by birth weight (BW) z-scores being below (equivalent to z-scores <-1.282) versus above the 10th percentile, respectively (41), with a relevant number of SGA-born individuals only in the VP/VLBW (n=30) but not in the FT group (n=8). Within the SGA cohort, successful/failed endpoint catch-up growth was defined as an adult body weight z-score at age 26 above/below the 10th percentile i.e., we focused on long-term body weight gain (6). For body weight trajectory analysis, we performed both trajectory slope and trajectory type analysis using Python version 3.7.10 and especially the “scikit-learn” package, a machine learning focused Python library. For trajectory slope analysis, linear regression of longitudinal body weight z-scores (non-interpolated) was performed to receive regressed body weight trajectories; change of body weight z-scores from birth until adulthood of regressed body weight trajectories defined long-term delta slopes. For trajectory type analysis, we clustered VP/VLBW body weight trajectories via k-means algorithm in Python (sklearn.cluster.KMeans). The number of times the k-means algorithm was run with different centroid seeds as default initialization was n=100.
We additionally undertook an exploratory analysis to identify the relationship between adult hypothalamic volumes and early catch-up (or catch-down) growth in body weight. Following the literature, early catch-up growth was defined as an increase of z-score of at least 0.67 during the first two years of life, with early catch-down representing a decrease of 0.67, respectively (16, 42). We used the first three measurements of body weight z-scores within our dataset (birth, five and 20 months) to perform linear regression equivalent to the long-term slope analysis; change of body weight z-scores from birth until the age of 2 years of those regressed body weight trajectories defined “short-term delta slope” (defining: “early catch-up” if “short-term delta slope” > 0.67; “early catch-down” if “short-term delta slope” < -0.67).
2.3 MRI data acquisition and hypothalamus segmentation
MRI data acquisition (for details see (36)) was performed on Philips Achieva 3T systems or Philips Ingenia 3T systems using an 8-channel SENSE head coil. Subject distribution among scanners was: Bonn Achieva 3T: 5 VP/VLBW, 11 FT; Bonn Ingenia 3T: 33 VP/VLBW, 17 FT; Munich Achieva 3T: 60 VP/VLBW, 65 FT; Munich Ingenia 3T: 3 VP/VLBW, 17 FT. Across all scanners sequence parameters were kept identical, namely high-resolution T1-weighted 3D magnetization prepared rapid acquisition gradient echo sequence with TI=1.3ms, TR=7.7ms, TE=3.9ms, flip angle=15°, 180 sagittal slices, FOV=256×256×180mm, reconstruction matrix=256×256, and reconstructed isotropic voxel size=1mm³. To account for possible confounds by scanner differences, MRI data analyses included scanner dummy variables as covariates of no interest.
T1-weighted MRI scans in Nifti-format were processed by using FreeSurfer version 7.2 (http://surfer.nmr.mgh.harvard.edu/), which includes a deep convolutional neural network tool of Billot et al. (33) that enables for automated segmentation of the hypothalamus, including subsegment parcellation (Figure 1A). To ensure the reliability of the applied hypothalamic segmentation, we compared measured hypothalamic volumes with those of previous hypothalamus parcellation studies (see Supplementary Figure S2 and Table S3). We found similar hypothalamic volumes for our FT cohort, supporting the reliability of the hypothalamic segmentation.
Estimation of total intracranial volume (TIV; sum of segmented gray and white matter brain volumes and cerebrospinal fluid partitions) was performed with the CAT12 toolbox, version r1364 (http://www.neuro.uni-jena.de/cat/) (43) within SPM12 (https://www.fil.ion.ucl.ac.uk/spm/software/spm12/).
To focus on hypothalamic nuclei involved in body weight control, we mapped subsegments from the segmentation algorithm that contained nuclei relevant for body weight control onto three so-called subunits of body weight control, namely PVN.DM.LH+, INF.VM+ and LH+ (see Figure 1B). The PVN.DM.LH+ subunit is the aggregate of two subsegments of the segmentation of Billot et al. (33), namely the anterior-superior and superior tubular subsegment, and these two subsegments cover the preoptic area, the paraventricular nucleus (PVN), the dorsomedial nucleus (DM), and parts of the lateral hypothalamus (LH); the latter three nuclei are involved in body weight control, therefore defining the name of the subunit. INF.VM+ is identical to the inferior tubular subsegment, which comprises - amongst other nuclei - the body weight control-related infundibular (INF) and ventromedial (VM) nuclei. LH+ matches the posterior subsegment in Billot et al. (33), including mammillary bodies, and parts of the tuberomammillary nucleus, and, critically, of the body weight control-related lateral hypothalamus (LH). The final anterior-inferior subsegment (suprachiasmatic nucleus and parts of the supraoptic nucleus) was excluded from further analysis because it does not contain any body weight control-related nuclei. All subunits stated in the analysis already consider volumes of bilateral hypothalamus.
2.4 Statistical analysis
Statistical analyses were performed using SPSS version 27 (IBM SPSS Statistics). Regarding demographical characteristics, group differences between VP/VLBW and FT cohorts were assessed using chi-square tests (sex, SES) and two-sample t-tests (age, GA, FS-IQ). To test whether both hypothalamic volumes and body weights are altered in prematurity, general linear models were used (dependent variable: hypothalamic volumes or body weights; fixed factor: status of prematurity; covariates: sex and additionally only for hypothalamic volume changes: scanner, TIV), thus controlling for differences in head size, gender and subject-to-scanner distribution. We did, however, not account explicitly for additional perinatal and gestational factors also affecting outcomes of preterm birth, including those on the brain (comp. chapter “Strengths and limitations”), because we were primarily interested in the general relationship between preterm birth, body weight development, and hypothalamus. Partial correlation analysis, restricted to the VP/VLBW group and corrected for sex, scanner and TIV, was used to investigate the associations between hypothalamic volumes and variables of preterm birth or body weight trajectories, respectively. To assess potential mediation effects of hypothalamic volumes regarding the association of variables of prematurity (i.e., INTI and GA, respectively) with adult body weight, a mediation analysis restricted to the VP/VLBW cohort was performed using the PROCESS toolbox (version 3.5) of SPSS.
As adult body weight at age 26 was missing for four individuals of the VP/VLBW cohort, they were excluded from all analyses related to body weight. For trajectory type analysis (clustering of body weight trajectories) missing longitudinal body weight data points were approximated via linear interpolation (compare Supplement methods for more detailed information).
Statistical significance was set at p <0.05; all tests were two-sided. Tests were corrected for multiple comparisons for false discovery rate (FDR) according to the Benjamini-Hochberg procedure (44).
3 Results
3.1 Sample characteristics
Table 1 and Figure 2A show group demographical-clinical, neurocognitive, and body weight-related variables, including longitudinal body weight measurements and their z-scores leading to body weight trajectories of VP/VLBW individuals. 26 individuals of the VP/VLBW cohort fulfilled only the VP criterion (GA< 32 weeks), 21 only the VLBW criterion (BW<1,500g; 32 ≤ GA ≤ 36, GA mean: 33.7 thus still being preterm) and 54 fulfilled both criteria. There were no significant differences between the VP/VLBW and FT group regarding age at scanning (p=0.165), sex (p=0.806), and SES at birth (p=0.770). By design, VP/VLBW subjects had significantly lower GA (p<0.001) and lower BW (p<0.001). VP/VLBW subjects had significantly lower FS-IQ scores in adulthood (p<0.001). Notably, all longitudinal body weight measurements, including adult body weight, were significantly lower in VP/VLBW individuals compared to FT controls.
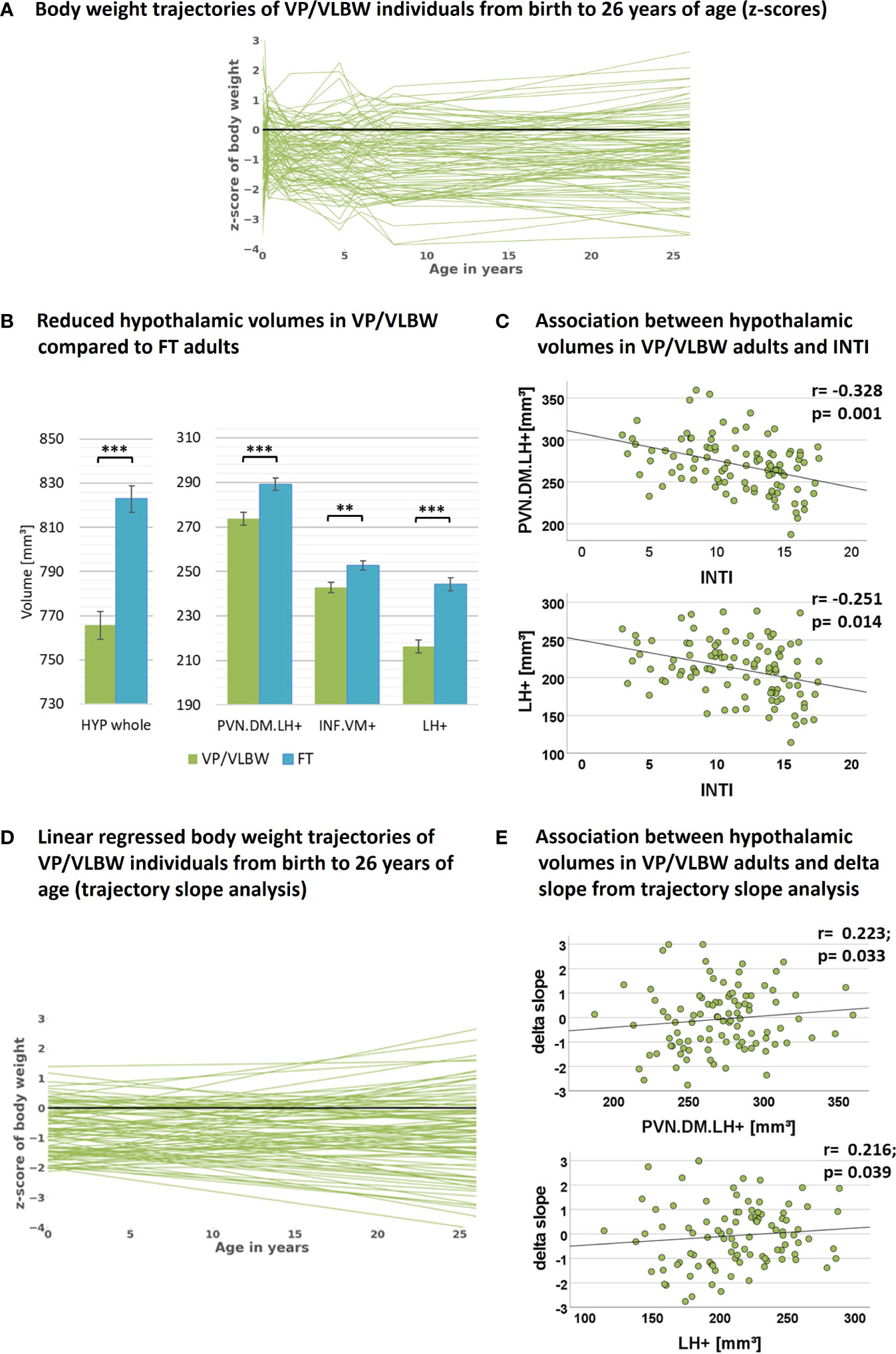
Figure 2 Hypothalamic volumes, prematurity, and body weight trajectories: (A) Individual body weight trajectories in the VP/VLBW cohort representing z-scores of body weight at birth and age 5, 20, 56 months, 6, 8 and 26 years. (B) Reduced whole hypothalamus and subunit volumes in the VP/VLBW compared to the FT cohort. Marginal means of whole hypothalamus and its subunits are given in mm³ and are shown as bar plots; error bars indicate SE. Group differences were assessed using a general linear model (fixed factor: prematurity at birth, covariates of no interest: sex, scanner, TIV). Group difference significance is marked by asterisks (**: p-FDR < 0.01; *** p-FDR < 0.001). (C) Associations between hypothalamic volumes and variables of preterm birth in the VP/VLBW cohort verified by partial correlation analysis. Scatterplots show exemplary relationships between hypothalamus subunit volumes and INTI (for further results see Supplementary Table S7). (D) Linear regression model of individual body weight trajectories in the VP/VLBW cohort representing body weight development slopes via linear regression of individual z-scores of body weight at birth, age 5, 20, 56 months and 6, 8 and 26 years (trajectory long-term slope analysis). (E) Association between hypothalamic volumes and long-term delta slope in the VP/VLBW sample. Scatterplots show relationships of hypothalamus subunits with long-term delta slope representing change of body weight z-score from birth until adulthood of linear regressed body weight trajectories. Linear regression lines and regression coefficients of partial regression analysis are added. Abbreviations: FDR, false discovery rate correction for multiple comparisons using the Benjamini–Hochberg method; FT, full-term; INTI, intensity of neonatal treatment; SE, standard error; VP/VLBW, very preterm and/or very low birth weight.
3.2 Lower hypothalamic volumes in VP/VLBW adults linking with body weight trajectories
To investigate whether hypothalamic volumes, including subunits involved in body weight control, are altered after preterm birth, we applied a general linear model approach controlling for sex, scanner, and TIV. We found significantly lower volumes in whole hypothalamus, PVN.DM.LH+, INF.VM+ and LH+ (all p-values <0.002) in VP/VLBW versus FT adults (Figure 2B; Table 2A).
As VP/VLBW subjects had enlarged lateral ventricles (ca. 25% of VP/VLBW, for detailed analysis see (45)), TIV as control variable might even underestimate the group differences in hypothalamus tissue volumes between the two groups. Therefore, we also adjusted for total gray matter volume instead of TIV, which resulted in p-values <0.001 for hypothalamus volume differences between VP/VLBW and FT adults (see Supplementary Table S4), suggesting that lower hypothalamus volumes were not influenced by ventricle volume changes.
Additionally, we performed exploratory analyses of sexual dimorphism and lateralization of hypothalamic volumes for the VP/VLBW and FT cohort. We used general linear models with fixed factor sex or sidedness of hypothalamic hemisphere, respectively, and covariates scanner and TIV (and sex for test of lateralization). No significant sex differences could be detected, although whole hypothalamic volumes of full-term born adults had a trend to be larger in male individuals (comp. Supplementary Table S5). In both cohorts, whole hypothalamus volume and all considered subunits (except for the LH+ subunit) showed a significant lateralization of hypothalamic volumes to the left (Supplement Table S6).
To test whether hypothalamic volume reductions in VP/VLBW adults are indeed related to preterm birth, namely preterm birth-related variables GA and INTI, we performed partial correlation analyses corrected for sex, scanner and TIV. We found significant positive associations between GA and volumes of whole hypothalamus (r=0.265, p=0.009), INF.VM+ (r=0.247, p=0.016), and LH+ (r=0.236, p=0.021) as well as significant negative associations between INTI and volumes of whole hypothalamus (r=-0.296, p=0.004), PVN.DM.LH+ (r=-0.328, p=0.001), and LH+ (r=-0.251, p=0.014) (Figure 2C for INTI only, Supplementary Table S7), suggesting that lower volumes are indeed related with preterm birth.
To test whether hypothalamic volume reductions were related with adult body weight, we applied with respect to adult body weight both partial correlation (corrected for sex, scanner and TIV) and mediation analyses. Concerning correlation analysis, we found a positive correlation between PVN.DM.LH+ volume and adult body weight (r=0.214, p=0.041; Supplementary Table S8). For mediation analysis (Supplementary Figure S3) the three body weight control-related hypothalamus subunits, PVN.DM.LH+, INF.VM+ and LH+, were taken as parallel potential mediators of the relation between INTI or GA, respectively, and adult body weight. We found that the PVN.DM.LH+ subunit served as a selective mediator of the association between INTI and adult body weight for VP/VLBW adults. These results indicate the relevance of the PVN.DM.LH+ subunit for adult body weight after preterm birth.
To test whether VP/VLBW body weight trajectories were linked with adult hypothalamic volumes, we performed a trajectory slope analysis in the VP/VLBW group. We identified body weight development slopes of individuals by adapting a linear regression model to the measured longitudinal body weight z-scores (Figure 2D). Subsequently, change of body weight z-scores from birth to adulthood of regressed body weight trajectories (long-term delta slope) was used for partial correlation analysis with hypothalamic volumes (Figure 2E; Supplementary Table S9). Whole hypothalamus volume (r=0.238, p=0.022), PVN.DM.LH+ (r=0.223, p=0.033), and LH+ (r=0.216, p=0.039) were positively correlated with body weight development trajectory long-term slopes, suggesting that body weight trajectories link with adult hypothalamic volumes in prematurity.
3.3 Subgroup analysis: adults with long-term catch-up growth
Next, to study further special subgroups for associations between hypothalamic volumes and body weight trajectories, we focused now on all VP/VLBW individuals who showed long-term catch-up growth (Figure 3). To identify these individuals, we performed a body weight trajectory cluster analysis using a k-means algorithm approach. Based on optimal cluster size of n=4, which was determined as a trade-off between maximizing explained variance score via Python (compare Supplementary Figure S4) and generating functionally explainable body weight trajectory cluster, we found four distinct types (i.e., cluster) of body weight development (Figure 3A) which we called “low” (i.e., individuals with stable relative low body weight; n=32 individuals), “increasing” (i.e., individuals with increasing - from relative low to almost normal - body weight; n=18), “decreasing” (i.e., individuals with decreasing - from almost normal to relative low - body weight; n=21) and “average” (i.e., individuals with stable normal body weight; n=26) weight trajectory. Particularly, the “increasing” trajectory group (red color) represented individuals who started with lowest birth weight z-scores (-2.026) in comparison to all other VP/VLBW clustered groups, but increased their body weight z-scores during development into adulthood to almost normal; we used this group as a proxy for the long-term catch-up growth group. Using a general linear model with these four clustered groups as fixed factor, we found that the “increasing” group had relatively largest hypothalamic volumes within the VP/VLBW cohort (see Figure 3B), with a volumetric difference most distinct for the LH+ subunit (p=0.024), when compared with the “decreasing” group (yellow color). By adding FT cohort as fixed factor into a further general linear model, we found that the “increasing” group’s hypothalamic volumes even were not different to those of the FT cohort, particularly not for whole hypothalamus, PVN.DM.LH+ and LH+ subunits, while for the other groups (i.e., low, decreasing, and average group) whole hypothalamus and LH+ volumes were smaller compared to FT (see Supplementary Figure S5).
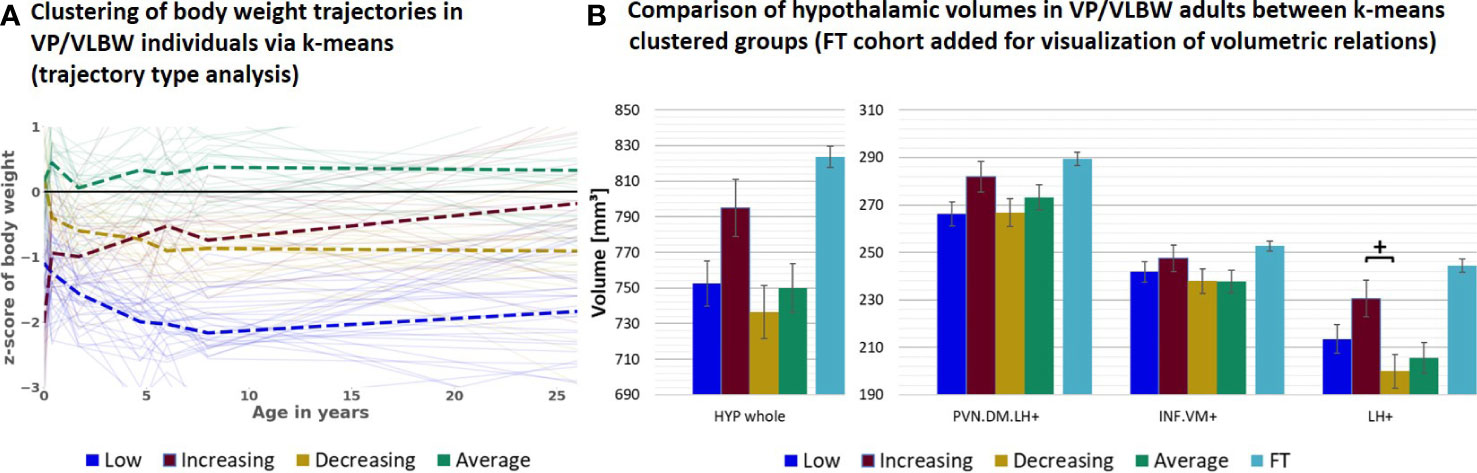
Figure 3 Hypothalamic volumes and body weight trajectories into adulthood – long-term catch-up growth subgroup: (A) Clustering of body weight trajectories in the VP/VLBW cohort via k-means algorithm (four cluster predefined); k-means grouping: “low” (dark blue), “increasing” (red), “decreasing” (yellow) and “average” (green) (trajectory type analysis). (B) Relatively largest HYP volumes of “increasing” group within VP/VLBW cohort. Marginal means of HYP volumes are given in mm³ for k-means clustered groups of VP/VLBW cohort and are shown as bar plots; subunit volumes of FT group (light blue) are added for evaluating volume changes in subgroups relative to FT subjects; error bars indicate SE. Group differences were assessed using a general linear model (fixed factor: four-part variable considering k-means clustered VP/VLBW group differentiation, covariates of no interest: sex, scanner, TIV). Group difference significance is marked by asterisks (+: p < 0.05). FDR, false discovery rate correction for multiple comparisons using the Benjamini–Hochberg method; FT, full-term; SE, standard error; VP/VLBW, very preterm and/or very low birth weight.
We additionally performed an exploratory analysis on the effect of early body weight development. Therefore, we focused on potential volumetric differences of adult hypothalamic volumes comparing VP/VLBW individuals with early catch-up or early catch-down growth in body weight within the first two years of life or stable conditions. No significant differences in whole hypothalamus and respective subunits were detected among these groups, although volumes had a trend to be larger particularly for the group of “early catch-up” in comparison to the group of “early catch-down” (compare Supplement Table S10). Partial correlation analysis, restricted to the VP/VLBW group and corrected for sex, scanner and TIV, was used to investigate the associations between adult hypothalamic volumes and “short-term delta slope”. In line with results on volumetric level this correlation analysis provided significant positive correlation factors for whole hypothalamus and the respective subunits (see Supplement Table S11). Additionally, there was a significant correlation between “short-term delta slope” and birth weight z-scores (r=-0.726; p<0.001) and between “short-term delta slope” and “long-term delta slope” (r=306; p=0.002). 10 out of the 19 VP/VLBW individuals showing early catch-up were also part of the 18 individuals with “increasing” long-term catch-up growth, 24 out of the 33 VP/VLBW individuals showing early catch-down were also part of the 53 individuals with “decreasing” or “low” long-term body weight development.
3.4 Subgroup analysis: adults born with very low birth weight
As a next subgroup, we focused on VP/VLBW individuals of lower birth weight, particularly individuals born SGA. To start with, we tested whether adult hypothalamic volumes were related to birth weight in the VP/VLBW group using a partial correlation approach corrected for sex, scanner, and TIV. We found, unexpectedly, significant negative correlations, namely for the whole hypothalamus (r=-0.262, p=0.012), INF.VM+ (r=-0.231, p=0.027), and LH+ (r=-0.273; p=0.009) (Supplementary Table S12). Correspondingly, when comparing VP/VLBW individuals born SGA (n=30) and those born AGA/LGA (n=67), we found larger volumes for the SGA group, significant for whole hypothalamus, LH+ and INF.VM+ (Table 2B). To test whether SGA hypothalamic volumes were comparable with those of FT controls, we added FT controls into the general linear model (Figure 4A; Supplementary Table S13) and found indeed that INF.VM+ volumes of the SGA group did not differ significantly from those of the FT group.
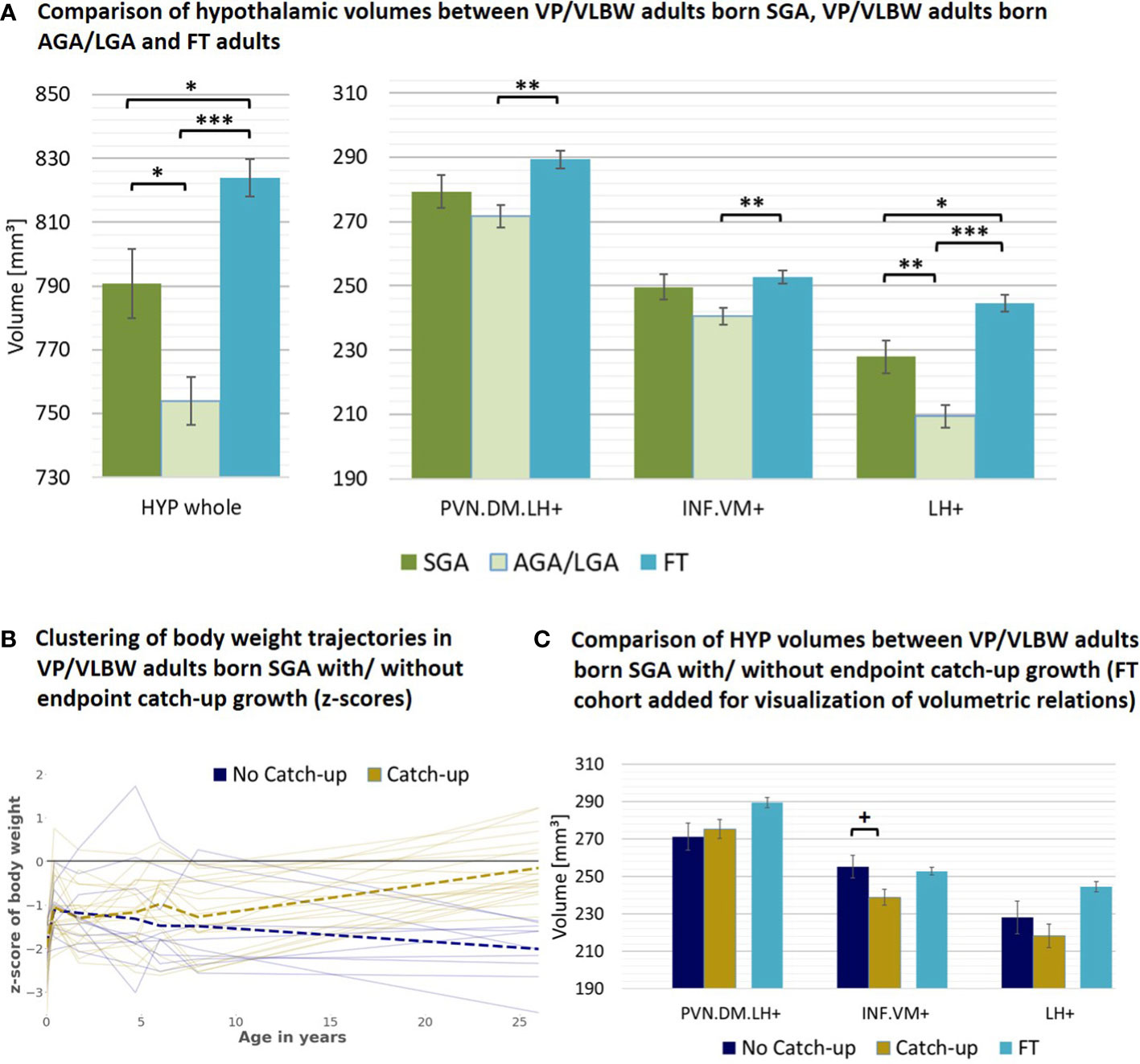
Figure 4 Hypothalamic volumes and birth weight – small for gestational age subgroup: (A) Relatively increased HYP volumes of VP/VLBW adults born SGA compared to those VP/VLBW adults born AGA/LGA. Marginal means of HYP volumes are given in mm³ and are shown as bar plots; error bars indicate SE. Group differences were assessed using a general linear model (fixed factor: SGA, AGA/LGA and FT group differentiation status, covariates of no interest: sex, scanner, TIV). Group difference significance is marked by asterisks (+: p < 0.05; *: p-FDR < 0.05; **: p-FDR < 0.01; *** p-FDR < 0.001). (B) Individual body weight trajectories in the VP/VLBW SGA cohort. Endpoint catch-up growth was assessed successful if SGA individuals exceeded 10th percentile of adult body weight z-score (yellow trajectories) and failed if not (blue trajectories). Dashed bold lines represent mean body weights of both cohorts at each point of time. (C) Hypothalamic volumes in endpoint catch-up and no endpoint catch-up SGA group. Marginal means of HYP subunits are given in mm³ for the SGA cohort comparing endpoint catch-up and no endpoint catch-up growth and are shown as bar plots; subunit volumes of FT group are added for evaluating volume differences in subgroups relative to FT subjects; error bars indicate SE. Group differences were assessed using a general linear model (fixed factor: endpoint catch-up growth status, covariates of no interest: sex, scanner, TIV). AGA/LGA, appropriate for gestational age/large for gestational age; FDR, false discovery rate correction for multiple comparisons using the Benjamini–Hochberg method; FT, full-term; SE, standard error; SGA, small for gestational age; VP/VLBW, very preterm and/or very low birth weight.
Then, we studied body weight trajectories of VP/VLBW adults born SGA. We asked whether - within this SGA subgroup - hypothalamic volumes differed with respect to individuals’ body weight trajectory after birth, namely for the subgroups of SGA with ‘successful’ (n=20) or ‘failed’ (n=10) endpoint catch-up growth, defined as adult body weight z-score being above or below the 10th percentile. Remarkably, the SGA group of failed endpoint catch-up growth had relatively larger volumes than their successful catch-up counterpart for INF.VM+ (p<0.05), with volumes in the range of that in the FT group (Figures 4B, C; Table 2C).
4 Discussion
Using T1-weighted MRI, deep-learning-based hypothalamus subunit segmentation, and longitudinal body weight assessment into adulthood, we observed reduced volumes of whole hypothalamus and hypothalamus subunits relevant for body weight control in VP/VLBW adults, with volume reductions being distinctively associated with long-term body weight trajectories after preterm birth.
4.1 Volumes of body weight control-related hypothalamus subunits are reduced in VP/VLBW adults and link with body weight trajectories into adulthood
Volumes of whole hypothalamus and those of body weight control-related subunits PVN.DM.LH+, INF.VM+ and LH+ were reduced in VP/VLBW adults compared to FT controls (Figure 2B). This result was not confounded by sex, scanner, and differences in TIV, as we controlled for these factors. Control of TIV suggests relatively stronger hypothalamic, as compared to general brain volume reduction after preterm birth. Volume reductions of VP/VLBW adults were related to GA and INTI, suggesting that hypothalamic volume reductions were indeed linked with preterm birth (Figure 2C). Hypothalamic volume reductions are in line with long-term volume reductions in other subcortical gray matter structures after preterm birth, such as cholinergic basal forebrain, thalamus or basal ganglia nuclei (46–49). Furthermore, hypothalamic volume reductions were associated with both altered adult body weight (Supplementary Figure S3, Table S8) and body weight trajectories in general, namely trajectory long-term slopes (Figure 2E, Supplementary Table S9), indicating that hypothalamic volume reductions are relevant for impaired long-term body weight gain of prematurity.
Regarding potential microscopic causes of hypothalamic volume reductions, we speculate that canonical hypoxic-ischemic events-induced pathways of both primary impairment of transient cells, namely pre-oligodendrocytes (Pre-OLs) and subplate neurons, and secondary activation of reactive neuroinflammatory cells, such as microglia or astrocytes, might be relevant (50–53). In particular, pre-OLs are responsible for myelination and axonal maturation of connections in general (51), suggesting that pre-OL development impairments are relevant for alterations of hypothalamic nuclei, which are characterized by a high level of long-range connections. We also cannot exclude direct hypoxic-ischemic damage on hypothalamic cells. Beyond perinatal brain injuries, it has been further suggested that additional factors, such as malnutrition and maternal, fetal or postnatal stress, do not only alter feeding behavior and body weight gain (54), but also hypothalamic development of preterm-born infants (24, 51, 55–57).
Based on our exploratory analyses, we could not detect significant sex differences in hypothalamic volumes in both the VP/VLBW and FT cohorts, although according to animal studies several hypothalamic nuclei are known to be sexually dimorphic with regards to regulatory functions and morphometry (21, 58–60). Remarkably, we found a trend to significance of larger whole hypothalamus volumes in full-term born males, being in line with a recent study detecting indeed larger volumes of the whole hypothalamus in adult males (28). We suggest that our MRI-based approach on hypothalamus structure might not be sensitive (enough) to sex differences.
Additionally, we could confirm human imaging studies (28, 61) detecting a larger left than right whole hypothalamus volume. In our case this link was also valid for the PVN.DM.LH+ and the INF.VM+, but not the LH+ subunit, namely for the FT as well as for the VP/VLBW cohort. For literature describing the asymmetrically representation of certain hypothalamic functions and transmitters compare (e.g., 21, 62, 63). In total, the last results further confirm the representative character of our sample with respect to hypothalamic volumes.
4.2 VP/VLBW adults with long-term body weight catch-up, relatively larger hypothalamus nuclei, and the lateral hypothalamus
By the use of k-means clustering of body weight trajectories (trajectory type analysis), we identified a special group of VP/VLBW individuals with long-term catch-up growth (“increasing” group; compare Figure 3). Remarkably, hypothalamic nuclei volumes of this VP/VLBW long-term catch-up group were not different to those of the FT group, while the other three VP/VLBW groups had lower hypothalamic volumes for whole hypothalamus and LH+ (Supplementary Figure S5); additionally, LH+ volumes of the long-term catch-up group were relatively larger than those of the other VP/VLBW trajectory groups (Figure 3B). Therefore, relatively larger hypothalamic volumes, particularly for subunits including the lateral hypothalamus, seem to be associated with more favorable long-term body weight trajectories in VP/VLBW. This observation is in line with findings that volumetric increases of hypothalamus gray matter and hence its nuclei are linked with altered hypothalamic regulation of body weight (64). Furthermore, it matches results in humans that revealed a positive association of general brain growth with optimized early nutrition (65) and body growth (66).
Based on our exploratory analysis on early catch-up, we could show that VP/VLBW individuals with relatively low birth weight and an early catch-up in the first two years of life are linked to the respective positive long-term trend of body weight development until adulthood and are more probable in expressing relatively larger adult hypothalamic volumes than those of e.g. early catch-down growth. Taken together, those relations match our observations on hypothalamic volumes for the long-term “increasing” catch-up group. Unfortunately, due to missing MRI data at birth, we cannot decide whether relatively larger hypothalamus volumes, especially of the lateral hypothalamus, are a result of a relatively increasing hypothalamus during development (i.e., volumetric hypothalamus catch-up) or of already at birth relatively larger hypothalamus volumes. Future studies of the infant hypothalamus are necessary to test this suggestions, with implications for both optimized nutrition (67) and neuroprotective/neurorestorative interventions (51) to prevent potential aberrant hypothalamic development.
4.3 VP/VLBW adults born SGA have larger hypothalamic volumes; SGA without endpoint catch-up growth and infundibular-ventromedial nuclei
We unexpectedly found negative correlations between adult hypothalamic volumes and birth weight in VP/VLBW adults (Supplementary Table S12). Correspondingly, VP/VLBW adults born SGA have relatively larger adult hypothalamic volumes than their AGA/LGA counterparts (Figure 4A).
In preterm-born babies being SGA, similar results have been reported for the pituitary structure, with its height being increased (68, 69). Contrary to that, several studies have linked preterm-born infants being SGA or those with intrauterine growth restriction (IUGR) with reduced cortical (70, 71) and subcortical gray matter volumes such as hippocampus, basal ganglia or thalamus nuclei (72, 73).
Therefore, to explain relatively increased hypothalamic volumes in VP/VLBW adults born SGA, two non-exclusive scenarios are conceivable: First, hypothalamic volume increases might already be present in infancy or even at birth (as in the case of the pituitary gland) and/or second, they might develop postnatally over time. The first hypothesis is supported by observed hyperactivity of the human hypothalamic-pituitary axis in preterm-born SGA neonates due to hormonal changes (e.g., growth-hormone (GH), IGF-1) (11, 68, 74), suggesting compensatory enlargement of the hypothalamus. It is also supported by animal studies showing higher cell counts or relative volumes of certain body weight control-related nuclei in a (non-prematurity) SGA model, potentially due to intrauterine neuroendocrine changes and epigenetic modifications (75, 76).
The second hypothesis is supported by studies that demonstrated higher vulnerability of gray matter for adverse effects of prematurity on SGA or IUGR infants in comparison to AGA (51, 77), suggesting a certain degree of perinatal hypothalamic volume reduction. In this case, the later increased hypothalamic volumes of VP/VLBW born SGA in adulthood could be explained by an altered volumetric hypothalamus development from birth into adulthood (24). Notably, long-term hypothalamic development can be affected by prolonged neurogenesis e.g., based on special neural stem cells called tanycytes, which are also supposed to regulate food intake and energy expenditure (78–80). These cells are located both along the lateral walls near to body weight control-related nuclei and at the floor of the third ventricle, namely nearby the INF.VM+ subunit. Their neurogenesis is controlled e.g., by neurotrophic factors like brain-derived neurotrophic factor (BDNF) (81), or nutrition and the endocrine system, including metabolic hormones such as leptin, insulin, ghrelin, and IGF-1 (82, 83). Concentrations of these hormones and molecules are altered in humans after preterm birth (15, 74, 84), specifically for SGA-born individuals (85–87). Besides prematurity and weight status at birth, body weight changes during development until adulthood are linked with altered ghrelin and leptin levels in humans (88, 89). Both hormones affect infundibular and ventromedial nuclei (as parts of INF.VM+), main sites of tanycyte neurogenesis (79). This might contribute to our findings of VP/VLBW adults born SGA without endpoint catch-up growth having relatively larger hypothalamic volumes, particularly for the INF.VM+ subunit, than those with endpoint catch-up growth (Figures 4B, C). This result suggests that for the SGA subgroup different hypothalamic nuclei might develop distinctively with respect to body weight development.
5 Strengths and limitations
Strengths of our study are the large sample size, enhancing power and generalizability of our findings. Homogenous mean across VP/VLBW and FT groups excludes confounding age effects. Notably, our results should be viewed as conservative estimates of the true group differences including hypothalamic volumes, as our sample is biased towards VP/VLBW adults with less severe neonatal complications and thus adult impairments. VP/VLBW adults with more neonatal complications or functional impairments had an increased probability not to participate in the study due to MRI exclusion criteria or to reject MRI screening. One should note that – beyond exclusion criteria – lack of motivation was the main reason for rejecting MRI and this reason was equally distributed across groups. One should note further that the sample was still representative of the full Bavarian Longitudinal Study cohort in terms of GA and BW distribution (36).
For calculation of body weight z-scores at distinct timepoints along the observed time frame, three different reference populations have been used. Though all three databases are large and represent data from the same country, z-score values may be biased due to relative differences in the reference populations’ body weight means and standard deviations at a particular timepoint.
For trajectory slope analysis (Figure 2D) - on first approximation - a linear body weight development model was preferred over a nonlinear one, despite the existence of nonlinearities in the body weight trajectories (Figure 2A). Although our sample size is large, future studies based on even larger sample sizes and more frequent body weight mapping along the age trajectory are necessary for nonlinear body weight development modelling
MRI brain scans were only available at age 26, whereas a longitudinal measurement of hypothalamic volumes during development would be required to address several relevant issues, such as separating cause from consequence regarding altered hypothalamic structure or revealing hypothalamic structure interdependency with long-term body weight gain.
This long-term body weight gain and hence its trajectories are – as mentioned – additionally influenced by many factors such as of maternal, genetic, fetal, environmental, nutritional, stress- or diseases related, endocrine, socioeconomic, and neurodevelopmental origin. Future studies are needed to include these factors, as within this study we were primarily interested in the general relationship between preterm birth, hypothalamus, and body weight gain.
A final limitation of our study is the subunit-focused parcellation methodology of the hypothalamus segmentation algorithm by Billot et al. (33). As their subunit definition is bound to anatomical landmarks, therefore aggregating several hypothalamic nuclei per subunit, it is challenging to allocate differences in subunit volumes to distinct body weight control-related hypothalamic nucleus volumes and hence their functional implications.
6 Conclusion and outlook
Our results demonstrate an association of preterm birth with body weight control-related hypothalamus subunits that link, in turn, with long-term body weight gain after preterm birth.
The presented approach suggests a potential of hypothalamus imaging for both weight development prognosis and potential treatment monitoring. It might also be translated to other disorders of impaired body weight development, from obesity to anorexia nervosa and treatment-induced body weight changes.
Data availability statement
The datasets presented in this study can be found in online repositories. The names of the repository/repositories and accession number(s) can be found below: Hypothalamus volumes and metadata of segmented individuals was uploaded via Dryad (https://datadryad.org/stash/share/qUpAwamRz0qThUhbBdqmtr5nTQqAYSdqR4uSDTfOxKs; doi:10.5061/dryad.vdncjsxwp). More data that support the findings of this study are available for interested researchers from the corresponding author upon reasonable request. The code used to generate and analyze the data was published by FreeSurfer (http://surfer.nmr.mgh.harvard.edu/) and can be found via https://surfer.nmr.mgh.harvard.edu/fswiki/HypothalamicSubunits.
Ethics statement
The studies involving human participants were reviewed and approved by Ethics committee of the Klinikum rechts der Isar and the University Hospital Bonn. Written informed consent to participate in this study was provided by the participants’ legal guardian/next of kin.
Author contributions
TR: Data curation; Software; Formal analysis; Validation; Investigation; Visualization; Methodology; Writing - original draft; Writing – review and editing. BS-K: Writing - review and editing. AM: Writing - review and editing. RE: Resources; Writing – review and editing. MD: Writing - review and editing. HB: Writing – review and editing. ER-F: Writing - review and editing. JP: Writing - review and editing. CZ: Writing - review and editing. PB: Funding acquisition; Writing - review and editing. DW: Resources; Funding acquisition; Writing - review and editing. CS: Conceptualization; Resources; Supervision; Funding acquisition; Methodology; Writing - original draft; Project administration; Writing - review and editing. DH: Conceptualization; Resources; Supervision; Funding acquisition; Methodology; Writing - original draft; Project administration; Writing - review and editing. All authors contributed to the article and approved the submitted version.
Funding
This study was supported by the Deutsche Forschungsgemeinschaft (BA 6370/2-1 to C.S.), the German Federal Ministry of Education and Science (BMBF 01ER0801 to PB and DW, BMBF 01ER0803 to CS) and the Kommission für Klinische Forschung, Technische Universität München (KKF 8700000474 to DH).
Acknowledgments
We thank all current and former members of the Bavarian Longitudinal Study Group who contributed to general study organization, recruitment, data collection and management as well as subsequent analyses, including (in alphabetical order): Barbara Busch, Stephan Czeschka, Claudia Grünzinger, Christian Koch, Diana Kurze, Sonja Perk, Andrea Schreier, Antje Strasser, Julia Trummer, and Eva van Rossum. We are grateful to the staff of the Department of Neuroradiology in Munich and the Department of Radiology in Bonn for their help in data collection. Most importantly, we thank all our study participants and their families for their efforts to take part in this study.
Conflict of interest
The authors declare that the research was conducted in the absence of any commercial or financial relationships that could be construed as a potential conflict of interest.
Publisher’s note
All claims expressed in this article are solely those of the authors and do not necessarily represent those of their affiliated organizations, or those of the publisher, the editors and the reviewers. Any product that may be evaluated in this article, or claim that may be made by its manufacturer, is not guaranteed or endorsed by the publisher.
Supplementary material
The Supplementary Material for this article can be found online at: https://www.frontiersin.org/articles/10.3389/fendo.2022.1057566/full#supplementary-material
Abbreviations
AGA/LGA, appropriate/large for gestational age; BW, birth weight; FT, full-term; GA, gestational age; HYP, hypothalamus; INTI, intensity of neonatal treatment index; SGA, small for gestational age; TIV, total intracranial volume; VP/VLBW, very preterm/very low birth weight.
References
1. Chawanpaiboon S, Vogel JP, Moller A-B, Lumbiganon P, Petzold M, Hogan D, et al. Global, regional, and national estimates of levels of preterm birth in 2014: a systematic review and modelling analysis. Lancet Glob Health (2019) 7(1):e37–46. doi: 10.1016/S2214-109X(18)30451-0
2. Markopoulou P, Papanikolaou E, Analytis A, Zoumakis E, Siahanidou T. Preterm birth as a risk factor for metabolic syndrome and cardiovascular disease in adult life: A systematic review and meta-analysis. J Pediatr (2019) 210:69–80.e5. doi: 10.1016/j.jpeds.2019.02.041
3. Abitbol CL, Rodriguez MM. The long-term renal and cardiovascular consequences of prematurity. Nat Rev Nephrol (2012) 8(5):265–74. doi: 10.1038/nrneph.2012.38
4. Wolke D, Johnson S, Mendonça M. The life course consequences of very preterm birth. Annu Rev Dev Psychol (2019) 1(1):69–92. doi: 10.1146/annurev-devpsych-121318-084804
5. Eves R, Mendonça M, Baumann N, Ni Y, Darlow BA, Horwood J, et al. Association of very preterm birth or very low birth weight with intelligence in adulthood: An individual participant data meta-analysis. JAMA Pediatr (2021) 175(8):e211058. doi: 10.1001/jamapediatrics.2021.1058
6. Euser AM, de Wit CC, Finken MJJ, Rijken M, Wit J-M. Growth of preterm born children. Horm Res (2008) 70(6):319–28. doi: 10.1159/000161862
7. Ford GW, Doyle LW, Davis NM, Callanan C. Very low birth weight and growth into adolescence. Arch Pediatr Adolesc Med (2000) 154(8):778–84. doi: 10.1001/archpedi.154.8.778
8. Lee SM, Kim N, Namgung R, Park M, Park K, Jeon J. Prediction of postnatal growth failure among very low birth weight infants. Sci Rep (2018) 8(1):3729. doi: 10.1038/s41598-018-21647-9
9. Geisler I, Rausch TK, Göpel W, Spiegler J. Extremely and very preterm-born children <1500 g show different weight development in childhood compared to their peers. Acta Paediatr (2021) 110(7):2093–9. doi: 10.1111/apa.15785
10. Boguszewski MCS, Cardoso-Demartini A. Management of endocrine disease: Growth and growth hormone therapy in short children born preterm. Eur J Endocrinol (2017) 176(3):R111–22. doi: 10.1530/EJE-16-0482
11. Saenger P, Czernichow P, Hughes I, Reiter EO. Small for gestational age: short stature and beyond. Endocr Rev (2007) 28(2):219–51. doi: 10.1210/er.2006-0039
12. Lapillonne A, Griffin IJ. Feeding preterm infants today for later metabolic and cardiovascular outcomes. J Pediatr (2013) 162(3 Suppl):S7–16. doi: 10.1016/j.jpeds.2012.11.048
13. Ordóñez-Díaz MD, Pérez-Navero JL, Flores-Rojas K, Olza-Meneses J, Muñoz-Villanueva MC, Aguilera-García CM, et al. Prematurity with extrauterine growth restriction increases the risk of higher levels of glucose, low-grade of inflammation and hypertension in prepubertal children. Front Pediatr (2020) 8:180. doi: 10.3389/fped.2020.00180
14. Ruys CA, Hollanders JJ, Bröring T, van Schie PEM, van der Pal SM, van de Lagemaat M, et al. Early-life growth of preterm infants and its impact on neurodevelopment. Pediatr Res (2019) 85(3):283–92. doi: 10.1038/s41390-018-0139-0
15. Möllers LS, Yousuf EI, Hamatschek C, Morrison KM, Hermanussen M, Fusch C, et al. Metabolic-endocrine disruption due to preterm birth impacts growth, body composition, and neonatal outcome. Pediatr Res (2021) 91(6):1350–60. doi: 10.1038/s41390-021-01566-8
16. Ong KK, Ahmed ML, Emmett PM, Preece MA, Dunger DB. Association between postnatal catch-up growth and obesity in childhood: prospective cohort study. BMJ (2000) 320(7240):967–71. doi: 10.1136/bmj.320.7240.967
17. Kerkhof GF, Willemsen RH, Leunissen RWJ, Breukhoven PE, Hokken-Koelega ACS. Health profile of young adults born preterm: negative effects of rapid weight gain in early life. J Clin Endocrinol Metab (2012) 97(12):4498–506. doi: 10.1210/jc.2012-1716
18. Monteiro POA, Victora CG. Rapid growth in infancy and childhood and obesity in later life–a systematic review. Obes Rev (2005) 6(2):143–54. doi: 10.1111/j.1467-789X.2005.00183.x
19. Bluet-Pajot MT, Epelbaum J, Gourdji D, Hammond C, Kordon C. Hypothalamic and hypophyseal regulation of growth hormone secretion. Cell Mol Neurobiol (1998) 18(1):101–23. doi: 10.1023/A:1022579327647
20. Rui L. Brain regulation of energy balance and body weight. Rev Endocr Metab Disord (2013) 14(4):387–407. doi: 10.1007/s11154-013-9261-9
21. Swaab DF. The human hypothalamus: basic and clinical aspects, part I: Nuclei of the human hypothalamus. In: Handbook of clinical neurology, 1st ed, vol. 79. . Amsterdam: Elsevier (2003-2004). Available at: http://www.sciencedirect.com/science/book/9780444513571.
22. Makris N, Swaab DF, van der Kouwe A, Abbs B, Boriel D, Handa RJ, et al. Volumetric parcellation methodology of the human hypothalamus in neuroimaging: normative data and sex differences. Neuroimage (2013) 69:1–10. doi: 10.1016/j.neuroimage.2012.12.008
23. Koutcherov Y, Mai JK, Ashwell KWS, Paxinos G. Organization of human hypothalamus in fetal development. J Comp Neurol (2002) 446(4):301–24. doi: 10.1002/cne.10175
24. Sominsky L, Jasoni CL, Twigg HR, Spencer SJ. Hormonal and nutritional regulation of postnatal hypothalamic development. J Endocrinol (2018) 237(2):R47–64. doi: 10.1530/JOE-17-0722
25. Yeo GSH, Heisler LK. Unraveling the brain regulation of appetite: lessons from genetics. Nat Neurosci (2012) 15(10):1343–9. doi: 10.1038/nn.3211
26. Morton GJ, Meek TH, Schwartz MW. Neurobiology of food intake in health and disease. Nat Rev Neurosci (2014) 15(6):367–78. doi: 10.1038/nrn3745
27. Andermann ML, Lowell BB. Toward a wiring diagram understanding of appetite control. Neuron (2017) 95(4):757–78. doi: 10.1016/j.neuron.2017.06.014
28. Thomas K, Beyer F, Lewe G, Zhang R, Schindler S, Schönknecht P, et al. Higher body mass index is linked to altered hypothalamic microstructure. Sci Rep (2019) 9(1):17373. doi: 10.1038/s41598-019-53578-4
29. Florent V, Baroncini M, Jissendi-Tchofo P, Lopes R, Vanhoutte M, Rasika S, et al. Hypothalamic structural and functional imbalances in anorexia nervosa. Neuroendocrinology (2020) 110(6):552–62. doi: 10.1159/000503147
30. Saper CB. 15 - hypothalamus. In: Paxinos G, editor. The human nervous system. San Diego: Academic Press (San Diego (1990). p. 389–413. Available at: https://www.sciencedirect.com/science/article/pii/B9780125476256500209. ISBN: 978-0-12-374245-2.
31. Neudorfer C, Germann J, Elias GJB, Gramer R, Boutet A, Lozano AM. A high-resolution in vivo magnetic resonance imaging atlas of the human hypothalamic region. Sci Data (2020) 7(1):305. doi: 10.1038/s41597-020-00644-6
32. Spindler M, Özyurt J, Thiel CM. Automated diffusion-based parcellation of the hypothalamus reveals subunit-specific associations with obesity. Sci Rep (2020) 10(1):22238. doi: 10.1038/s41598-020-79289-9
33. Billot B, Bocchetta M, Todd E, Dalca AV, Rohrer JD, Iglesias JE. Automated segmentation of the hypothalamus and associated subunits in brain MRI. Neuroimage (2020) 223:117287. doi: 10.1016/j.neuroimage.2020.117287
34. Wolke D, Ratschinski G, Ohrt B, Riegel K. The cognitive outcome of very preterm infants may be poorer than often reported: an empirical investigation of how methodological issues make a big difference. Eur J Pediatr (1994) 153(12):906–15. doi: 10.1007/BF01954744
35. Wolke D, Meyer R. Cognitive status, language attainment, and prereading skills of 6-year-old very preterm children and their peers: the Bavarian longitudinal study. Dev Med Child Neurol (1999) 41(2):94–109. doi: 10.1017/S0012162299000201
36. Schmitz-Koep B, Zimmermann J, Menegaux A, Nuttall R, Bäuml JG, Schneider SC, et al. Decreased amygdala volume in adults after premature birth. Sci Rep (2021) 11(1):5403. doi: 10.1038/s41598-021-84906-2
37. Breeman LD, Jaekel J, Baumann N, Bartmann P, Wolke D. Preterm cognitive function into adulthood. Pediatrics (2015) 136(3):415–23. doi: 10.1542/peds.2015-0608
38. Bauer A. Ein verfahren zur messung des für das bildungsverhalten relevanten sozial status (BRSS)–überarbeitete fassung. Frankfurt: Deutsches Institut fuer Internationale Paedagogische Forschung (1988).
39. Aster M, Neubauer A, Horn R. Wechsler intelligenztest für erwachsene (WIE) [Wechsler adult intelligence scale (WAIS III)] - deutschsprachige bearbeitung und adaptation des WAIS-III von David wechsler. 3rd ed. Frankfurt, Germany: Pearson (2006).
40. Gutbrod T, Wolke D, Soehne B, Ohrt B, Riegel K. Effects of gestation and birth weight on the growth and development of very low birthweight small for gestational age infants: a matched group comparison. Arch Dis Child Fetal Neonatal Ed (2000) 82(3):F208–14. doi: 10.1136/fn.82.3.F208
41. WHO. Physical status: the use and interpretation of anthropometry. report of a WHO expert committee. World Health Organ Tech Rep Ser (1995) 854:1–452.
42. Olbertz DM, Mumm R, Wittwer-Backofen U, Fricke-Otto S, Pyper A, Otte J, et al. Identification of growth patterns of preterm and small-for-gestational age children from birth to 4 years - do they catch up? J Perinat Med (2019) 47(4):448–54. doi: 10.1515/jpm-2018-0239
43. Gaser C, Dahnke R, Thompson PM, Kurth F, Luders E. CAT – a computational anatomy toolbox for the analysis of structural MRI data. bioRxiv (2022) 2022:06.11.495736. doi: 10.1101/2022.06.11.495736
44. Benjamini Y, Hochberg Y. Controlling the false discovery rate: A practical and powerful approach to multiple testing. J R Stat Society Ser B (Methodological) (1995) 57(1):289–300. doi: 10.1111/j.2517-6161.1995.tb02031.x
45. Hedderich DM, Boeckh-Behrens T, Bäuml JG, Menegaux A, Daamen M, Zimmer C, et al. Sequelae of premature birth in young adults : Incidental findings on routine brain MRI. Clin Neuroradiol (2021) 31(2):325–33. doi: 10.1007/s00062-020-00901-6
46. Ball G, Boardman JP, Rueckert D, Aljabar P, Arichi T, Merchant N, et al. The effect of preterm birth on thalamic and cortical development. Cereb Cortex (2012) 22(5):1016–24. doi: 10.1093/cercor/bhr176
47. Nosarti C, Nam KW, Walshe M, Murray RM, Cuddy M, Rifkin L, et al. Preterm birth and structural brain alterations in early adulthood. NeuroImage Clin (2014) 6:180–91. doi: 10.1016/j.nicl.2014.08.005
48. Loh WY, Anderson PJ, Cheong JLY, Spittle AJ, Chen J, Lee KJ, et al. Longitudinal growth of the basal ganglia and thalamus in very preterm children. Brain Imaging Behav (2020) 14(4):998–1011. doi: 10.1007/s11682-019-00057-z
49. Grothe MJ, Scheef L, Bäuml JG, Meng C, Daamen M, Baumann N, et al. Reduced cholinergic basal forebrain integrity links neonatal complications and adult cognitive deficits after premature birth. Biol Psychiatry (2017) 82(2):119–26. doi: 10.1016/j.biopsych.2016.12.008
50. Salmaso N, Jablonska B, Scafidi J, Vaccarino FM, Gallo V. Neurobiology of premature brain injury. Nat Neurosci (2014) 17(3):341–6. doi: 10.1038/nn.3604
51. Volpe JJ. Dysmaturation of premature brain: Importance, cellular mechanisms, and potential interventions. Pediatr Neurol (2019) 95:42–66. doi: 10.1016/j.pediatrneurol.2019.02.016
52. Reemst K, Noctor SC, Lucassen PJ, Hol EM. The indispensable roles of microglia and astrocytes during brain development. Front Hum Neurosci (2016) 10:566. doi: 10.3389/fnhum.2016.00566
53. Hoerder-Suabedissen A, Molnár Z. Development, evolution and pathology of neocortical subplate neurons. Nat Rev Neurosci (2015) 16(3):133–46. doi: 10.1038/nrn3915
54. Maniam J, Morris MJ. The link between stress and feeding behaviour. Neuropharmacology (2012) 63(1):97–110. doi: 10.1016/j.neuropharm.2012.04.017
55. Lammertink F, Vinkers CH, Tataranno ML, Benders MJNL. Premature birth and developmental programming: Mechanisms of resilience and vulnerability. Front Psychiatry (2020) 11:531571. doi: 10.3389/fpsyt.2020.531571
56. Howland MA, Sandman CA, Glynn LM. Developmental origins of the human hypothalamic-pituitary-adrenal axis. Expert Rev Endocrinol Metab (2017) 12(5):321–39. doi: 10.1080/17446651.2017.1356222
57. Finken MJJ, van der Voorn B, Hollanders JJ, Ruys CA, de WM, van Goudoever JB, et al. Programming of the hypothalamus-Pituitary-Adrenal axis by very preterm birth. Ann Nutr Metab (2017) 70(3):170–4. doi: 10.1159/000456040
58. Gorski RA, Gordon JH, Shryne JE, Southam AM. Evidence for a morphological sex difference within the medial preoptic area of the rat brain. Brain Res (1978) 148(2):333–46. doi: 10.1016/0006-8993(78)90723-0
59. Matsumoto A, Arai Y. Sex difference in volume of the ventromedial nucleus of the hypothalamus in the rat. Endocrinol Jpn (1983) 30(3):277–80. doi: 10.1507/endocrj1954.30.277
60. Hofman MA, Zhou JN, Swaab DF. Suprachiasmatic nucleus of the human brain: an immunocytochemical and morphometric analysis. Anat Rec (1996) 244(4):552–62. doi: 10.1002/(SICI)1097-0185(199604)244:4<552::AID-AR13>3.0.CO;2-O
61. Wolff J, Schindler S, Lucas C, Binninger A-S, Weinrich L, Schreiber J, et al. A semi-automated algorithm for hypothalamus volumetry in 3 Tesla magnetic resonance images. Psychiatry Res Neuroimaging (2018) 277:45–51. doi: 10.1016/j.pscychresns.2018.04.007
62. Ishunina TA, Swaab DF. Vasopressin and oxytocin neurons of the human supraoptic and paraventricular nucleus: size changes in relation to age and sex. J Clin Endocrinol Metab (1999) 84(12):4637–44. doi: 10.1210/jcem.84.12.6187
63. Kiss DS, Toth I, Jocsak G, Barany Z, Bartha T, Frenyo LV, et al. Functional aspects of hypothalamic asymmetry. Brain Sci (2020) 10(6):389. doi: 10.3390/brainsci10060389
64. Le TM, Liao D-L, Ide J, Zhang S, Zhornitsky S, Wang W, et al. The interrelationship of body mass index with gray matter volume and resting-state functional connectivity of the hypothalamus. Int J Obes (Lond) (2020) 44(5):1097–107. doi: 10.1038/s41366-019-0496-8
65. Schneider J, Fischer Fumeaux CJ, Duerden EG, Guo T, Foong J, Graz MB, et al. Nutrient intake in the first two weeks of life and brain growth in preterm neonates. Pediatrics (2018) 141(3). doi: 10.1542/peds.2017-2169
66. Coviello C, Keunen K, Kersbergen KJ, Groenendaal F, Leemans A, Peels B, et al. Effects of early nutrition and growth on brain volumes, white matter microstructure, and neurodevelopmental outcome in preterm newborns. Pediatr Res (2018) 83(1-1):102–10. doi: 10.1038/pr.2017.227
67. Ottolini KM, Andescavage N, Keller S, Limperopoulos C. Nutrition and the developing brain: the road to optimizing early neurodevelopment: a systematic review. Pediatr Res (2020) 87(2):194–201. doi: 10.1038/s41390-019-0508-3
68. Kiortsis D, Xydis V, Drougia AG, Argyropoulou PI, Andronikou S, Efremidis SC, et al. The height of the pituitary in preterm infants during the first 2 years of life: an MRI study. Neuroradiology (2004) 46(3):224–6. doi: 10.1007/s00234-003-1126-6
69. Xydis V, Drougia A, Giapros V, Argyropoulou M, Andronikou S. Brain growth in preterm infants is affected by the degree of growth restriction at birth. J Matern Fetal Neonatal Med (2013) 26(7):673–9. doi: 10.3109/14767058.2012.746300
70. Tolsa CB, Zimine S, Warfield SK, Freschi M, Sancho Rossignol A, Lazeyras F, et al. Early alteration of structural and functional brain development in premature infants born with intrauterine growth restriction. Pediatr Res (2004) 56(1):132–8. doi: 10.1203/01.PDR.0000128983.54614.7E
71. Miller SL, Huppi PS, Mallard C. The consequences of fetal growth restriction on brain structure and neurodevelopmental outcome. J Physiol (2016) 594(4):807–23. doi: 10.1113/JP271402
72. Lodygensky GA, Seghier ML, Warfield SK, Tolsa CB, Sizonenko S, Lazeyras F, et al. Intrauterine growth restriction affects the preterm infant's hippocampus. Pediatr Res (2008) 63(4):438–43. doi: 10.1203/PDR.0b013e318165c005
73. Padilla N, Junqué C, Figueras F, Sanz-Cortes M, Bargalló N, Arranz A, et al. Differential vulnerability of gray matter and white matter to intrauterine growth restriction in preterm infants at 12 months corrected age. Brain Res (2014) 1545:1–11. doi: 10.1016/j.brainres.2013.12.007
74. Kajantie E. Insulin-like growth factor (IGF)-I, IGF binding protein (IGFBP)-3, phosphoisoforms of IGFBP-1 and postnatal growth in very-low-birth-weight infants. Horm Res (2003) 60 Suppl 3:124–30. doi: 10.1159/000074513
75. Plagemann A, Harder T, Rake A, Melchior K, Rohde W, Dörner G. Hypothalamic nuclei are malformed in weanling offspring of low protein malnourished rat dams. J Nutr (2000) 130(10):2582–9. doi: 10.1093/jn/130.10.2582
76. Grissom NM, Reyes TM. Gestational overgrowth and undergrowth affect neurodevelopment: similarities and differences from behavior to epigenetics. Int J Dev Neurosci (2013) 31(6):406–14. doi: 10.1016/j.ijdevneu.2012.11.006
77. Vinall J, Grunau RE, Brant R, Chau V, Poskitt KJ, Synnes AR, et al. Slower postnatal growth is associated with delayed cerebral cortical maturation in preterm newborns. Sci Transl Med (2013) 5(168):168ra8. doi: 10.1126/scitranslmed.3004666
78. Pellegrino G, Trubert C, Terrien J, Pifferi F, Leroy D, Loyens A, et al. A comparative study of the neural stem cell niche in the adult hypothalamus of human, mouse, rat and gray mouse lemur (Microcebus murinus). J Comp Neurol (2018) 526(9):1419–43. doi: 10.1002/cne.24376
79. Bolborea M, Dale N. Hypothalamic tanycytes: potential roles in the control of feeding and energy balance. Trends Neurosci (2013) 36(2):91–100. doi: 10.1016/j.tins.2012.12.008
80. Bolborea M, Langlet F. What is the physiological role of hypothalamic tanycytes in metabolism? Am J Physiol Regul Integr Comp Physiol (2021) 320(6):R994–R1003. doi: 10.1152/ajpregu.00296.2020
81. Pencea V, Bingaman KD, Wiegand SJ, Luskin MB. Infusion of brain-derived neurotrophic factor into the lateral ventricle of the adult rat leads to new neurons in the parenchyma of the striatum, septum, thalamus, and hypothalamus. J Neurosci (2001) 21(17):6706–17. doi: 10.1523/JNEUROSCI.21-17-06706.2001
82. Chaker Z, George C, Petrovska M, Caron J-B, Lacube P, Caillé I, et al. Hypothalamic neurogenesis persists in the aging brain and is controlled by energy-sensing IGF-I pathway. Neurobiol Aging (2016) 41:64–72. doi: 10.1016/j.neurobiolaging.2016.02.008
83. Desai M, Li T, Ross MG. Fetal hypothalamic neuroprogenitor cell culture: preferential differentiation paths induced by leptin and insulin. Endocrinology (2011) 152(8):3192–201. doi: 10.1210/en.2010-1217
84. Krey FC, Stocchero BA, Creutzberg KC, Heberle BA, Tractenberg SG, Xiang L, et al. Neurotrophic factor levels in preterm infants: A systematic review and meta-analysis. Front Neurol (2021) 12:643576. doi: 10.3389/fneur.2021.643576
85. Martos-Moreno GA, Barrios V, Sáenz de Pipaón M, Pozo J, Dorronsoro I, Martínez-Biarge M, et al. Influence of prematurity and growth restriction on the adipokine profile, IGF1, and ghrelin levels in cord blood: relationship with glucose metabolism. Eur J Endocrinol (2009) 161(3):381–9. doi: 10.1530/EJE-09-0193
86. Jaquet D, Leger J, Levy-Marchal C, Oury JF, Czernichow P. Ontogeny of leptin in human fetuses and newborns: effect of intrauterine growth retardation on serum leptin concentrations. J Clin Endocrinol Metab (1998) 83(4):1243–6. doi: 10.1210/jcem.83.4.4731
87. Finken MJJ, van der Steen M, Smeets CCJ, Walenkamp MJE, de Bruin C, Hokken-Koelega ACS, et al. Children born small for gestational age: Differential diagnosis, molecular genetic evaluation, and implications. Endocr Rev (2018) 39(6):851–94. doi: 10.1210/er.2018-00083
88. Klok MD, Jakobsdottir S, Drent ML. The role of leptin and ghrelin in the regulation of food intake and body weight in humans: a review. Obes Rev (2007) 8(1):21–34. doi: 10.1111/j.1467-789X.2006.00270.x
Keywords: body weight gain, hypothalamus subunits, preterm birth, small for gestational age, catch-up growth, magnetic resonance imaging
Citation: Ruzok T, Schmitz-Koep B, Menegaux A, Eves R, Daamen M, Boecker H, Rieger-Fackeldey E, Priller J, Zimmer C, Bartmann P, Wolke D, Sorg C and Hedderich DM (2022) Lower hypothalamus subunit volumes link with impaired long-term body weight gain after preterm birth. Front. Endocrinol. 13:1057566. doi: 10.3389/fendo.2022.1057566
Received: 29 September 2022; Accepted: 30 November 2022;
Published: 15 December 2022.
Edited by:
Ben Nephew, Worcester Polytechnic Institute, United StatesReviewed by:
Laura Dearden, University of Cambridge, United KingdomValeria Carlini, National University of Cordoba, Argentina
Copyright © 2022 Ruzok, Schmitz-Koep, Menegaux, Eves, Daamen, Boecker, Rieger-Fackeldey, Priller, Zimmer, Bartmann, Wolke, Sorg and Hedderich. This is an open-access article distributed under the terms of the Creative Commons Attribution License (CC BY). The use, distribution or reproduction in other forums is permitted, provided the original author(s) and the copyright owner(s) are credited and that the original publication in this journal is cited, in accordance with accepted academic practice. No use, distribution or reproduction is permitted which does not comply with these terms.
*Correspondence: Tobias Ruzok, dG9iaWFzLnJ1em9rQHR1bS5kZQ==
†These authors have contributed equally to this work and share last authorship