- 1Guangxi Key Laboratory of Environmental Exposomics and Entire Lifecycle Heath, Guangxi Health Commission Key Laboratory of Entire Lifecycle Health and Care, School of Public Health, Guilin Medical University, Guilin, China
- 2Scientific Experiment Center, Guilin Medical University, Guilin, China
- 3Laboratory of Gynecologic Oncology, Department of Gynecology, Fujian Maternity and Child Health Hospital, College of Clinical Medicine for Obstetrics and Gynecology and Pediatrics, Fujian Medical University, Fuzhou, China
Objective: To explore the relationship between angiotensin-converting enzyme 2 (ACE2) genetic variants and gestational diabetes mellitus (GDM) in a southern Chinese population.
Methods: Potential functional variants (rs2106809, rs6632677, and rs2074192) of ACE2 were selected and genotyped in 566 GDM patients and 710 normal pregnaõncies in Guilin, China. The odds ratio (OR) and its corresponding 95% confidence interval (CI) were used to evaluate the association between genetic variant and GDM risk, and then the false positive report probability, multifactor dimensional reduction (MDR), and bioinformatics tools were used to confirm the significant association in the study.
Results: After adjusting for age and prepregnancy body mass index, logistic regression analysis showed that ACE2 rs6632677 was significantly associated with a decreased risk of GDM (CC vs. GG: adjusted OR = 0.09, 95% CI: 0.01 – 0.71, P = .023; GC/CC vs. GG: adjusted OR = 0.68, 95% CI = 0.46 – 0.99, P = .048; and CC vs. GG/GC: adjusted OR = 0.09, 95% CI = 0.01 – 0.72, P = .024), whereas rs2074192 was associated with increased GDM risk (TT vs. CC/CT: adjusted OR = 1.38, 95% CI = 1.08 – 1.75, P = .009). Furthermore, we found that rs6632677 interacted with SBP (Pinteraction = .043) and FPG (Pinteraction = .021) and rs2074192 interacted with HDL-c (Pinteraction = .029) and LDL-c (Pinteraction = .035) to influence the GDM risk of the individual. In the MDR analysis, the rs6632677 was the best one-locus model, and the three-loci model was the best interaction model to predict GDM risk. In addition, functional analysis confirmed that rs2074192 may regulate the splicing process of ACE2 gene.
Conclusion: ACE2 gene variants are significantly associated with the risk of GDM via gene–gene and gene–environment combinations. The rs2074192 C > T affects the splicing of the ACE2 gene, which may be a potential mechanism leading to the changed susceptibility of an individual female during pregnancy to GDM.
Introduction
Gestational diabetes mellitus (GDM) is a complex disease caused by environmental and genetic factors, which are characterized by different degrees of impaired glucose tolerance during pregnancy. It is reported that the global prevalence rate of GDM is approximately 1%–14% (1). In China, the incidence rate of GDM is as high as 14.8% and is on the rise (2, 3). It is known that GDM can lead to serious maternal and infant complications, such as polyhydramnios, gestational hypertension, spontaneous abortion, pre-eclampsia (PE), preterm birth, respiratory distress syndrome, small for gestational age (SGA), large for gestational age (LGA), fetal macrosomia, shoulder dystocia, hypoglycemia, and even stillbirth (4, 5). In addition, GDM has a long-term impact on patients themselves and their offspring. For example, the risk of type 2 diabetes (T2DM) after childbirth is more than seven times higher than that of pregnant women with normal blood glucose, and the risk of metabolic diseases, such as obesity and T2DM, in their offspring is also greatly increased in the future (6).
It is generally believed that GDM has similar pathophysiological mechanisms as T2DM, such as insulin resistance and β-cell dysfunction. In addition, advanced gestational age, obesity, family history of T2DM, previous history of GDM, and previous poor obstetric history, etc., are also considered as risk factors for GDM (7–9). However, the specific etiological mechanism of GDM has not been fully revealed so far. Epidemiological evidence shows that GDM presents familial genetic characteristics. A family history of diabetes is an independent risk factor for GDM, and the closer the family relationship between diabetes patients and pregnant women, the greater the risk of GDM in pregnant women (6). Meanwhile, the incidence rate of GDM in Asian women during pregnancy is about three to seven times higher than that in Caucasians (10).
Single-nucleotide polymorphisms (SNPs) are DNA sequence variations caused by the conversion or transversion of a single nucleotide. Such genetic variants have the core information that determines the genetic susceptibility of human diseases and play important roles in the genetic anatomy of complex traits (11, 12). SNPs may act as quantitative trait loci (QTLs) and be associated with complex disease phenotypes by affecting the mRNA/protein levels, methylation rate, and physiological and biochemical indicators, etc. (13–15). For instance, studies show that the genetic variant rs10830963 of the MTNR1B gene is associated with the pathogenesis of T2DM by affecting the expression of MTNR1B (16), and the rs10830963G allele could significantly increase the odds of antenatal insulin therapy of GDM in pregnancy (17). We also found that angiotensin-converting enzyme 2 (ACE2) rs2106809 may be involved in the pathogenesis of PE as an expression quantitative trait locus (eQTL) that regulates the transcription of functional genes. These findings reveal that inherited genetic factors play a key role in the genesis and development of GDM.
ACE2, a key component of the renin angiotensin system (RAS), transforms angiotensin (ANG) II to Ang-(1–7) and protects against ANG II–induced oxidative stress and inflammation (18). It is reported that the ACE2 gene and its variants are closely related to T2DM risk or pregnancy complications such as fetal growth restriction (FGR), PE with placental insufficiency, SGA, oxidative stress (OS), and inflammation (19–22). Evidence suggests an association between ACE2 and glucose regulation. Ace2-knockout mice were more susceptible than wild-type mice to pancreatic β-cell dysfunction (23). ACE2 can inhibit the expression of insulin resistance (IR)–related cytokines and, thus, reverse IR (24). It is now known that placental ACE2 is significantly decreased from the first to the third trimester of pregnancy (25). Thus, we speculate that the decrease of ACE2 level during pregnancy may participate in the pathological development of GDM.
ACE2 gene variants were significantly associated with multiple pregnancy complications and T2DM and its complications (19, 26–28). We propose the hypothesis that the functional genetic variations may be involved in the transcription or posttranscriptional regulation of ACE2 and change the risk of individual GDM. Therefore, this study intends to clarify the contribution of genetic variants of the ACE2 gene to GDM risk in a case-control study with 566 GDM patients and 710 normal pregnancies.
Methods and materials
Study population
All 1276 subjects (566 GDM cases with a mean age of 31.52 ± 4.72 years and 710 normal pregnancies ages 28.93 ± 4.23 years) were recruited from the Affiliated Hospital of Guilin Medical University between September 2014 and April 2016. Participants who met the diagnostic criteria for GDM recommended by the International Association of Diabetes and Pregnancy Study Group (IADPSG) in 2010 can be diagnosed as GDM if their 75 g oral glucose tolerance test (OGTT) values reach or exceed any of the following thresholds: fasting blood glucose (5.1 mmol/L), 1h blood glucose (10.0 mmol/L), and 2h blood glucose (8.5 mmol/L). The subjects need to have lived in Guilin for more than 2 years, have no kinship with each other, and have singleton pregnancies this time. If pregnancies had been previously diagnosed with endocrine diseases, serious systemic diseases, history of prepregnancy type 1 or type 2 diabetes, long-term use of glucose metabolism–affecting drugs, and other pregnancy complications, they were excluded. Each subject signed the informed consent, and the Ethics Committee of Guilin Medical University approved this research scheme. The screening flow of samples in this study is shown in Figure 1.
Clinical and biochemical data
Clinical and biological characteristics were obtained from a unified questionnaire and patient medical records, including age, systolic blood pressure (SBP), diastolic blood pressure (DBP), fasting plasma glucose (FPG), triglyceride (TG), total cholesterol (TC), 75g OGTT 1h and 2h blood glucose, hemoglobin A1c (HbA1c), low-density lipoprotein (LDL), and high-density lipoprotein (HDL), etc. Meanwhile, the prepregnancy weight (Kg) and height (m) of the subjects were measured to calculate prepregnancy body mass index (pre-BMI) as weight (kg)/height (m)2.
Genomic DNA extraction
Genomic DNA was extracted from EDTA-treated whole blood using a DNA extraction kit (Aidlab Biotechnologies Co.,Ltd, China) and stored at -80°C prior to PCR.
Candidate variants selection
The potential functional variants in the ACE2 gene region were screened by using the NCBI dbSNP database (http://www.ncbi.nlm.nih.gov/projects/SNP) and SNPinfo Web Server (http://snpinfo.niehs.nih.gov/). At first, 169 functional variants of the ACE2 gene region were identified by SNPinfo’s SNP function prediction tool with gene name as the keyword. Then, because the minimum allele frequency (MAF) of candidate variants is less than 0.05 in the Chinese Han Beijing population (CHB), 166 loci were excluded. Finally, three functional variants (rs6632677 G > C, rs2074192 C > T, and rs2106809 A > G) with linkage unbalance coefficient less than 0.8 between candidate variants were selected according to LD TAG SNP Selection of SNPinfo.
Candidate variants genotyping by KASP method
Candidate ACE2 gene variants were genotyped by the competitive allele–specific PCR (KASP) method, and the corresponding specific PCR primers were designed and synthesized by Sangon Biotech (Shanghai) Co., Ltd. A volume of 10 µl reaction system was deposited in a 96-well plate, including 5 µl of FLu-Arms 2x PCR mix, 0.5 µl of 10 μM three specific primers (F1: 0.1µl, F2: 0.1µl, and R: 0.3 µl), 0.5 µl (25 – 150 ng) of DNA, and 4 µl of ddH2O. Two allele-specific forward primers were labeled with the fluorochrome FAM and HEX, respectively. Reactions were performed according to the following standard KASP-PCR program: predenaturation at 95°C for 3 min, then 10 touchdown cycles of 95°C for 15 s (denaturing), 61°C–55°C for 60 s (annealing and elongation), and followed by 30 cycles of 95°C for 15 s and 55°C for 60 s and plate read 30°C for 30 s. See Figure 2 for the genotyping scatterplot of candidate loci.
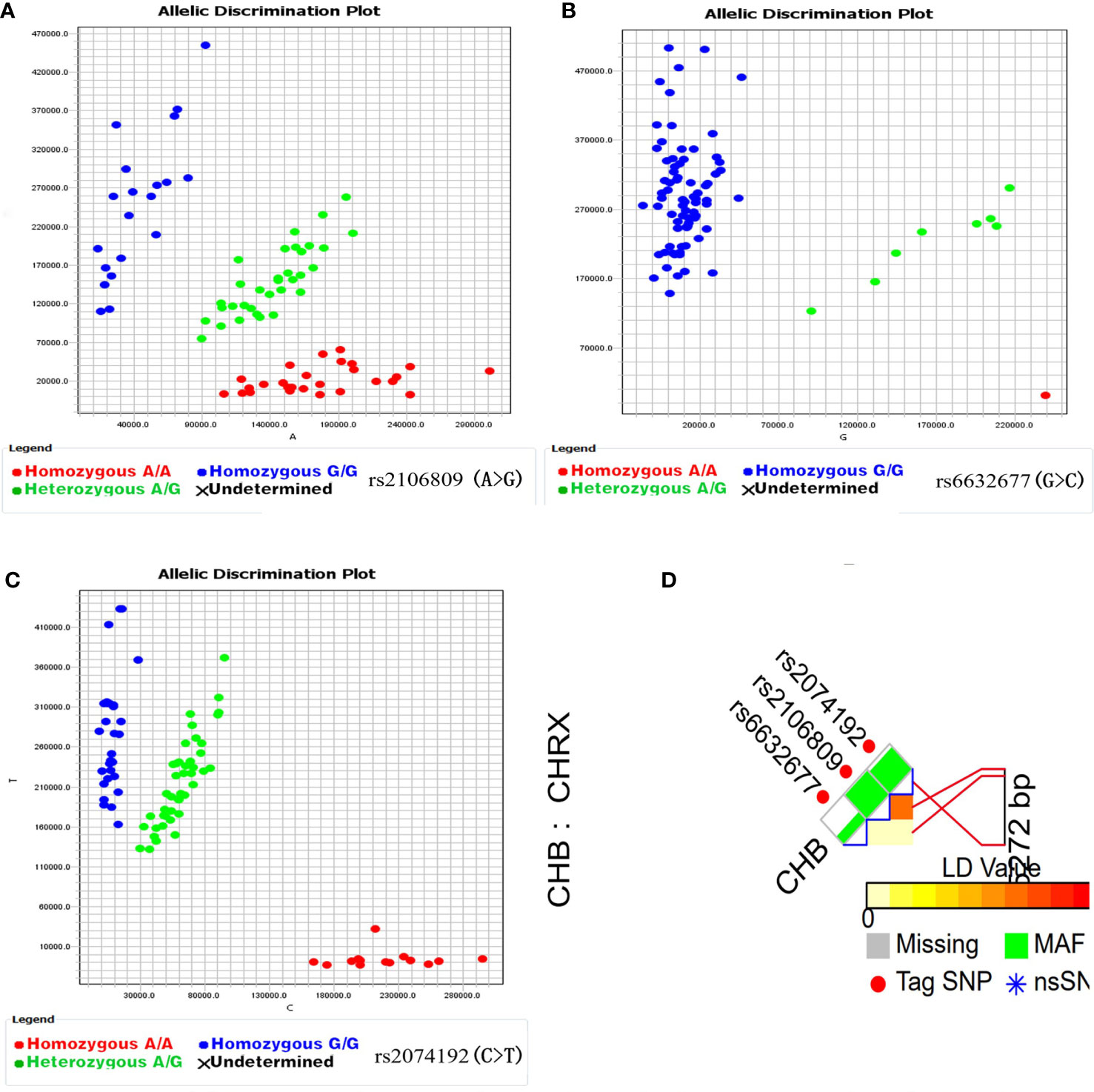
Figure 2 ACE2 gene candidate genetic variations selection and genotyping (A–C are genotyping scatter plots of rs6632677 G>C, rs2074192 C>T, rs2106809 A>G respectively; (D) is SNP selection by SNPinfo Web Server online tool, LD: r2<0.8).
Statistical analysis
Statistical analysis was performed with IBM SPSS Statistics 28 for Windows (IBM Corp., Armonk, NY, USA). Clinical and biochemical variables are shown as mean ± SD ( ± sd). The chi-square (χ2) test was adopted to detect the differences in selected demographic data. A χ2 goodness-of-fit test was performed to determine whether the distribution of genotypes of SNPs in the control group conformed to Hardy–Weinberg equilibrium (HWE). Binary logistic regression analyses were used to evaluate the associations between the genotypes of variants and the risk of GDM by calculating the odds ratios (ORs) and their 95% confidence intervals (CIs). In this study, the two-sided test was used, and P <.05 was considered statistically significant.
The false-positive reporting probability (FPRP) test described by Wacholder S et al. (29) was used to assess the robustness of the statistically significant associations detected in this study. During FPRP-value calculation, we first preset the prior probability of 0.1 and a relatively more stringent cutoff value of 0.2. Then, three values of odds ratios (OR = 1.2, 1.5, 2.0) that are most likely were set, assuming that there is a nonnull association. Finally, the OR estimate, 95% CI, and P-value associated with GDM risk of the genotype of the studied variant was entered to obtain the FPRP-value.
Multifactor dimension reduction (MDR) software (version 3.0.2) was applied to investigate the interaction effects between variants. Hundredfold cross-validation and 1000-fold permutation testing were adopted under the null hypothesis of no association, and the best multifactorial model was recognized with values of cross-validation consistency (CVC) and testing balanced accuracy (TBA) variables (30). In view of the fact that the research variation is located in the gene intron and may regulate gene transcription, the Alternative Splice Site Predictor (ASSP) tool was used to analyze their posttranscriptional splicing regulation (31). In the ASSP analysis, codon usage and stop codons for all three possible reading frames (F1 − frame 1, F2 − frame 2, F3 − frame 3) and scores of the preprocessing models reflecting splice site strength are calculated. We further applied GWAS4D online analysis (http://www.mulinlab.org/gwas4d/) (32) based on the GTEx database to analyze eQTL between studied variants and gene transcription levels.
Results
Characteristics of study subjects
The main characteristics of 566 GDM pregnancies and 710 healthy controls are shown in Table 1. Comparison of general data characteristics indicate that the age, BMI, SBP, DBP, TG, and HbA1c in GDM cases were significantly higher than those in the controls (P <.001). Also, the markers of glucose homeostasis, including FBG, 75g OGTT 1h blood glucose, and OGTT 2h blood glucose were also relatively higher (P <.001).
Association between studied variants and the risk of GDM
ACE2 rs6632677 G>C and GDM risk
Logistic regression analysis after adjustment of age and pre-BMI shows that ACE2 rs6632677 was significantly associated with the risk of GDM. Compared with the GG genotype, the CC genotype can significantly reduce the GDM risk of individuals by 91% (CC vs. GG, adjusted OR = 0.09, 95% CI = 0.01 − 0.71, P = .023). Moreover, under the dominant model (GC/CC vs. GG) compared with the GG genotype, GC/CC genotype carriers have lower GDM risk (adjusted OR = 0.68, 95% CI = 0.46 − 0.99, P = .048). Under the recessive model (CC vs. GG/GC), the CC genotype carriers could significantly reduce GDM risk compared with GG/GC genotypes (adjusted OR = 0.09, 95% CI = 0.01 − 0.72, P = .024) as shown in Table 2.
Then, we utilized stratified analysis to evaluate the risk of rs6632677 C > G with GDM under a dominant genetic model. After adjusting for age and pre-BMI, compared with the GG genotype, GC/CC genotype carriers have lower GDM risk in the SBP (> 110.2 mmHg) subgroup (adjusted OR=0.45, 95% CI= 0.26-0.79, P = .005), in the FPG (≤ 4.77 mmol/L) subgroup (adjusted OR=0.46, 95% CI= 0.26-0.84, P = .021), and in the Hb (≤ 5.15 mg/dl) subgroup (adjusted OR=0.50, 95% CI = 0.25-0.99, P = .049). Interestingly, significant interaction effects between rs6632677 and SBP (Pinteraction = .043) and FPG (Pinteraction = .021) were detected, influencing individual’s susceptibility to GDM (Table 3).
ACE2 rs2074192 C>T and GDM risk
It is shown that rs2074192 can significantly reduce the risk of individuals suffering from GDM; compared with the CC genotype, the CT genotype can significantly reduce the GDM risk by 28% (adjusted OR = 0.72, 95% CI = 0.52 − 0.99, P = .043). In addition, under the recessive model (TT vs. CC/CT), a significantly increased GDM risk of the TT genotype is detected compared with CC/CT genotypes (adjusted OR = 1.38, 95% CI = 1.08 − 1.75, P = .009) as shown in Table 2.
Stratified analysis reveals the significant associations between rs2074192 and age ≤ 30.04 years (adjusted OR = 1.41, 95% CI = 1.02 – 1.95, P = .036), the pre-BMI ≤ 22.3 kg/m2 group (adjusted OR = 1.40, 95% CI = 1.01 – 1.95, P = .042), the SBP ≤ 110.2 mmHg group (adjusted OR = 1.44, 95% CI = 1.03 – 2.01, P = .034), the DBP > 69.2 mmHg subgroup (adjusted OR = 1.43, 95% CI = 1.01 – 2.03, P = .044), TG > 2.55 mmol/L subjects (adjusted OR = 1.73, 95% CI = 1.19 − 2.54, P = .005), the TC > 5.30 mmol/L subgroup (adjusted OR = 1.71, 95% CI = 1.21 − 2.41, P = .003), HDL-c ≤ 1.66 mmol/L subjects (adjusted OR = 1.81, 95% CI = 1.29 − 2.54, P = .001), and LDL-c > 3.41 mmol/L subjects (adjusted OR = 1.82, 95% CI = 1.28 − 2.59, P = .001), respectively, under the recessive genetic model. Meanwhile, significant interaction effects between rs2074192 and HDL (Pinteraction = .029) and LDL (Pinteraction = .035) were detected. See Table 4.
However, we failed to find significant associations between rs2106809 A>G and the risk of GDM in the present study (P >.05).
False-positive reporting probability analysis
The FPRP test was adopted to assess the noteworthiness of the observed significant associations between the studied rs6632677 and rs2074192 and GDM risk. The prior probability of 0.1 and a relatively stringent FPRP cutoff value of 0.2 were set. The FPRP value was 0.155 for the association between rs2074192 and the risk of GDM under the recessive model (TT vs. CC/CT) and suggests that the significant correlation found above may be authentic. However, other significant associations found in the study may not be true, and the conclusion should be recognized with caution as shown in Tables 5 and 6.
High-order interaction with GDM risk by MDR analysis
MDR analysis indicates that rs6632677 made the best one-locus model for GDM with a maximum CVC of 100/100, a testing balanced accuracy of 0.5456, and a P-value of statistical test of.0009. Meanwhile, the three-loci model was the best interaction model to predict GDM risk with a maximum CVC of 100/100, a testing balanced accuracy of 0.5710, and a P-value <.0001 as shown in Table 7.
Potential regulatory function analysis
Alternative Splice Site Predictor (ASSP) tool analysis suggests that ACE2 rs2074192 C>T may lead to changes in the activity of putative splice sites, which are characterized by score activation, intron GC activation, alt. isoform/cryptic activation, constitutive activation, and confidence activation near the polymorphism. For instance, from the analysis results, we noticed that the activity of the putative splice site in the 75 bp position of the examined DNA sequence had a score activation of 5.938, intron GC activation of 0.357, alt. isoform/cryptic activation of 0.942, constitutive activation of 0.038, and confidence activation of 0.959 with the C allele of rs2074192, whereas the rs2074192 T allele had a score activation of 7.476, intron GC activation of 0.343, alt. isoform/cryptic activation of 0.938, constitutive activation of 0.041, and confidence activation of 0.956. In addition, it seems that this mutation created a new splicing site at the 79 bp position of the examined DNA sequence only with the rs2074192 T allele but not the C allele as shown in Figure 3.
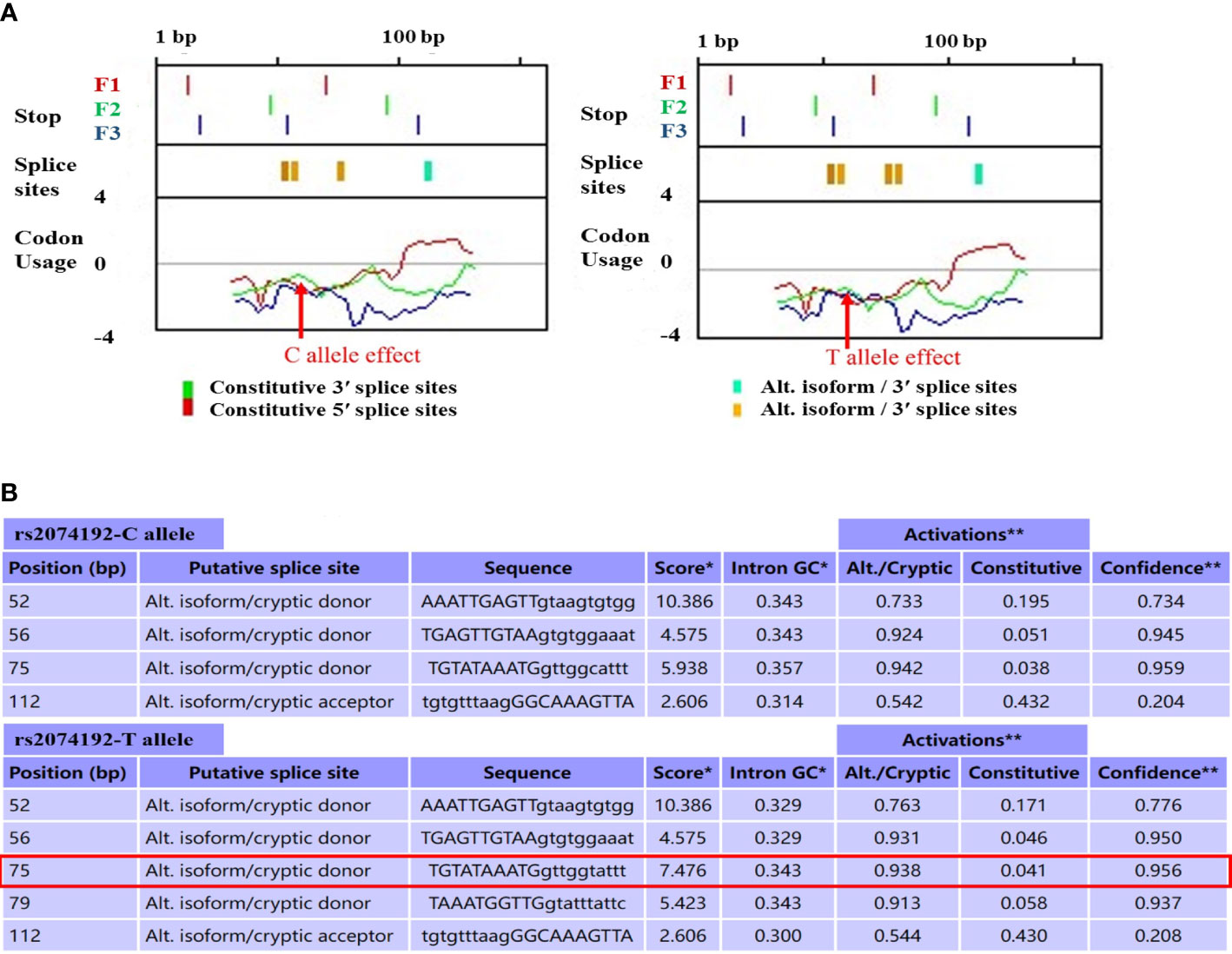
Figure 3 ACE2 rs2074192 (C > T) potential regulatory function analysis by Alternative Splice Site Predictor (ASSP) (A) Schematic representation of splicing positions reflecting the ACE2 rs2074192; (B) Score plot for preprocessing model reflecting sequences of putative splice sites; strenght: 161bp; Acceptor site cutoff: 2.2; Donor site cutoff: 4.5).
Furthermore, using the GTEx database, which incorporates 127 tissue/cell type–specific epigenome data sets, the GWAS4D online tool was used to analyze eQTL between rs2074192 and the regulation of gene transcription. This analysis indicated that rs2074192 could potentially regulate the expression of functional genes associated with GDM risk in a variety of specific tissues and cells, including PIR (total of 48 studies involved), and VEGFD (47 studies involved), which were identified as eQTL as shown in Table 8.
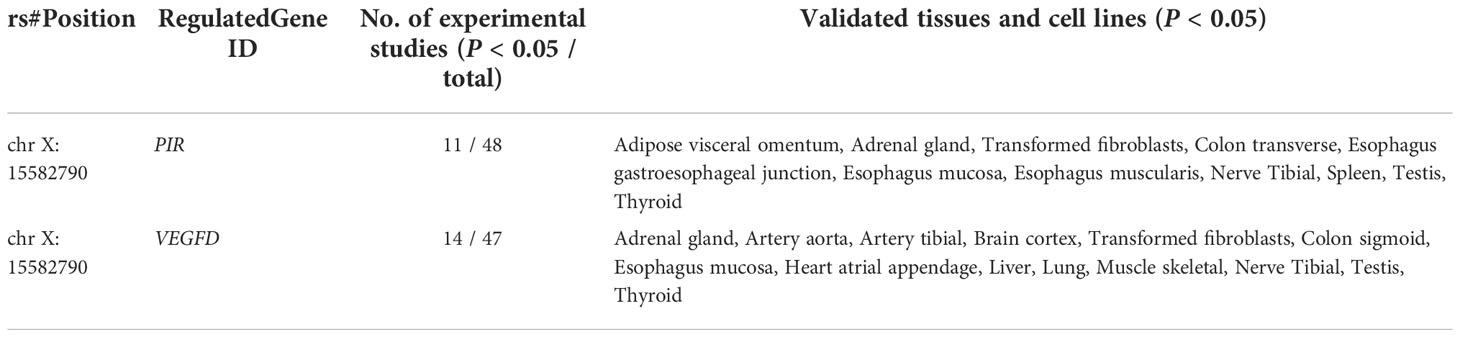
Table 8 The eQTL analysis on rs2074192 C > T and functional gene transcription levels by GWAS4D online tool.
Discussion
In this study, we propose the hypothesis that ACE2 and its variants may participate in the pathological mechanism of GDM. Thereupon, a case control study involving 566 GDM patients and 710 normal pregnancies at the same time was conducted to investigate the relationship between ACE2 functional variants and GDM. We found that rs6632677 G > C and rs2074192 C > T were significantly related to the incidence of GDM in Guilin women, and the mutant rs6632677 C allele and rs2074192 T allele showed an effect of reducing and increasing the risk of GDM, respectively. ACE2 variants are likely to modify the genetic background and affect the risk of individuals suffering from GDM under the same environmental risk exposure. The findings of this study provide some genetic clues for the susceptibility of the Guilin population to GDM, and the specific mechanisms need to be further analyzed. Studies indicate that gene variants may be associated with disease susceptibility by gene–gene interaction or affecting complex traits, such as mRNA/protein/methylation levels, biochemical indicators, etc. (14, 15). Liu C et al. found that ACE2 variants can significantly affect blood pressure (SBP or DBP) and blood lipid (TG, TC, HDL-C, or LDL-C) and regulate the onset of T2DM and its complications (33). This may be one of the mechanisms by which functional variants lead to individual susceptibility to disease. Therefore, we also carried out an association analysis between the studied variants and clinical traits of subjects.
Similar to this, we found that rs6632677 particularly affected the risk of GDM in SBP > 110.2 mmHg, FPG ≤ 4.77 mmol/L, and Hb ≤ 5.15 mg/dl subjects, and significant interaction effects between rs6632677 and SBP and FPG were detected. The associations between rs2074192 and GDM risk was even more pronounced in subjects of age ≤ 30.04 years, pre-BMI ≤ 22.3 kg/m2, SBP ≤ 110.2 mmHg, Hb ≤ 5.15mg/dl, TG > 2.55 mmol/L, HDL-c ≤ 1.66 mmol/L, and LDL-c > 3.41 mmol/L subgroups, and significant interaction effects were detected between rs2074192 and HDL-c and LDL-c. ACE2 variants might alter an individual’s GDM risk by regulating the key physiological and biochemical variables of the organism. Meanwhile, although no significant association between rs2106809 and the risk of GDM was detected in the single variant analysis, a complex gene–gene combination was detected in the MDR analysis. That is, the three-loci model was the best interaction model to predict the risk of GDM. These findings indicate that ACE2 gene variants show differences in effect among people with specific characteristics, and the combined effects or interactions between genetic and environmental factors (blood pressure, blood glucose, or blood lipid) may be one of the potential pathological mechanisms of GDM.
FPRP analysis is an effective method for determining the biological importance of associations (34). In this study, a strict FPRP threshold of 0.2 was set. In the recessive model, a significant correlation detected between rs2074192 and the risk of GDM risk was considered probably true and reliable. However, the FPRP values obtained in some comparisons that were much greater than 0.2 suggest that these associations may have been observed by chance. Therefore, the conclusions drawn here are preliminary and should be recognized with caution.
According to the research, genetic variants in the gene intron region can regulate mRNA transcription levels, thereby ultimately affecting an individual’s GDM risk (35, 36). In view of this, we explored the potential biological function of ACE2-positive associated variants using bioinformatics tools and found that rs2074192 is likely to regulate the posttranscriptional splicing process of ACE2 by modifying the activity of splice sites with different alleles. The change of the rs2074192 allele from C to T can affect the efficacy of potential splicing sites and even directly erase an existing or create a new splice site. Furthermore, eQTL analyses using the GTEx database, which incorporates 127 tissue/cell type–specific epigenome data sets, suggest that rs2074192 could regulate the expression of various functional genes. This evidence may provide new research perspectives and clues for revealing the genetic mechanism of GDM susceptibility. However, it is still essential to explore the biological function of rs2106809 in future studies to verify its role in the pathogenesis of GDM.
This study explores the relationship between ACE2 variants and GDM risk of Guilin, China, and finally, some etiological clues have been yielded from the perspective of genetics. However, this study still has some shortcomings. First, this was a hospital-based study and, therefore, might have selection bias. Second, potential influencing factors of GDM, such as smoking status, poor obstetrics, malnutrition, dangerous society, etc., were not measured, and these are likely to affect the final association effects between the studied ACE2 functional variants and GDM risk. Third, although a large sample study design was adopted in this study, the very low frequency genotypes tested in studied variants may still limit the efficiency, especially in subgroup analysis. Fourth, this study did not experimentally explore the biological function of the significant association locus.
Conclusion
ACE2 genes rs6632677 and rs2074192 are significantly associated with the risk of GDM. The underlying mechanism may be that the single locus effects and/or complex gene–gene and gene–environment interactions regulate the transcription of ACE2 gene and, thus, change the susceptibility of Guilin women to GDM during pregnancy.
Data availability statement
The original contributions presented in the study are publicly available. This data can be found here: Dryad, Dataset, https://doi.org/10.5061/dryad.34tmpg4pb.
Ethics statement
The studies involving human participants were reviewed and approved by Guilin Medical University and the Affiliated Hospital of Guilin Medical University. The patients/participants provided their written informed consent to participate in this study. Written informed consent was obtained from the individual(s) for the publication of any potentially identifiable images or data included in this article.
Author contributions
XY and LL: protocol/project development and manuscript editing. GH, QL and YW: data collection and analysis, and manuscript writing. QL and LQ: data analysis and manuscript writing. HY: data collection and management. All authors contributed to the article and approved the submitted version.
Funding
This study was supported by the Guangxi Natural Science Foundation of China (2020GXNSFAA23 8025); Guangxi Young and middle-aged teachers’ basic ability improvement project (2020KY12028); Maternal and Child Health Research Project of Guangxi Bagui Scholars (Zhang Jun); College Students’ Innovation Project (201810601031) of Guangxi, China; Fujian Provincial Health Technology Project (2020CXA017) and Fujian Provincial Maternity and Child Health Hospital Foundation (YCXM 20-16).
Conflict of interest
The authors declare that the research was conducted in the absence of any commercial or financial relationships that could be construed as a potential conflict of interest.
Publisher’s note
All claims expressed in this article are solely those of the authors and do not necessarily represent those of their affiliated organizations, or those of the publisher, the editors and the reviewers. Any product that may be evaluated in this article, or claim that may be made by its manufacturer, is not guaranteed or endorsed by the publisher.
References
1. Goedegebure EAR, Koning SH, Hoogenberg K, Korteweg FJ, Lutgers HL, Diekman MJM, et al. Pregnancy outcomes in women with gestational diabetes mellitus diagnosed according to the WHO-2013 and WHO-1999 diagnostic criteria: A multicentre retrospective cohort study. BMC pregnancy childbirth (2018) 18(1):152. doi: 10.1186/s12884-018-1810-5
2. Gao C, Sun X, Lu L, Liu F, Yuan J. Prevalence of gestational diabetes mellitus in mainland China: A systematic review and meta-analysis. J Diabetes Investig (2019) 10(1):154–62. doi: 10.1111/jdi.12854
3. Duman NB. Frequency of gestational diabetes mellitus and the associated risk factors. Pak J Med Sci (2015) 31(1):194–7. doi: 10.12669/pjms.311.5617
4. Poomalar GK. Changing trends in management of gestational diabetes mellitus. World J Diabetes (2015) 6(2):284–95. doi: 10.4239/wjd.v6.i2.284
5. Bodmer-Roy S, Morin L, Cousineau J, Rey E. Pregnancy outcomes in women with and without gestational diabetes mellitus according to the international association of the diabetes and pregnancy study groups criteria. Obstet Gynecol (2012) 120(4):746–52. doi: 10.1097/AOG.0b013e31826994ec
6. Wei W, He Y, Wang X, Tan G, Zhou F, Zheng G, et al. Gestational diabetes mellitus: The genetic susceptibility behind the disease. Horm Metab Res (2021) 53(8):489–98. doi: 10.1055/a-1546-1652
7. Zhang C, Bao W, Rong Y, Yang H, Bowers K, Yeung E, et al. Genetic variants and the risk of gestational diabetes mellitus: A systematic review. Hum Reprod Update (2013) 19(4):376–90. doi: 10.1093/humupd/dmt013
8. Chu SY, Callaghan WM, Kim SY, Schmid CH, Lau J, England LJ, et al. Maternal obesity and risk of gestational diabetes mellitus. Diabetes Care (2007) 30(8):2070–6. doi: 10.2337/dc06-2559a
9. Rhee SY, Kim JY, Woo JT, Kim YS, Kim SH. Familial clustering of type 2 diabetes in Korean women with gestational diabetes mellitus. Korean J Intern Med (2010) 25(3):269–72. doi: 10.3904/kjim.2010.25.3.269
10. Benny P, Ahn HJ, Burlingame J, Lee MJ, Miller C, Chen J, et al. Genetic risk factors associated with gestational diabetes in a multi-ethnic population. PloS One (2021) 16(12):e0261137. doi: 10.1371/journal.pone
11. Wang X, Tomso DJ, Liu X, Bell DA. Single nucleotide polymorphism in transcriptional regulatory regions and expression of environmentally responsive genes. Toxicol Appl Pharmacol (2005) 207(2 Suppl):84–90. doi: 10.1016/j.taap.2004.09.024
12. Kim S, Misra A. SNP genotyping: Technologies and biomedical applications. Annu Rev BioMed Eng (2007) 9:289–320. doi: 10.1146/annurev.bioeng.9.060906.152037
13. Li G. Expression quantitative trait loci analysis in multiple tissues. Methods Mol Biol (2020) 2082:231–7. doi: 10.1007/978-1-0716-0026-9_16
14. Prasad RB, Kristensen K, Katsarou A, Shaat N. Association of single nucleotide polymorphisms with insulin secretion, insulin sensitivity, and diabetes in women with a history of gestational diabetes mellitus. BMC Med Genomics (2021) 14(1):274. doi: 10.1186/s12920-021-01123-6
15. McRae AF, Marioni RE, Shah S, Yang J, Powell JE, Harris SE, et al. Identification of 55,000 replicated DNA methylation QTL. Sci Rep (2018) 8(1):17605. doi: 10.1038/s41598-018-35871-w
16. Gaulton KJ, Ferreira T, Lee Y, Raimondo A, Magi R, Reschen ME, et al. Genetic fine mapping and genomic annotation defines causal mechanisms at type 2 diabetes susceptibility loci. Nat Genet (2015) 47(12):1415–25. doi: 10.1038/ng.3437
17. Firneisz G, Rosta K, Al-Aissa Z, Hadarits O, Harreiter J, Nadasdi A, et al. The MTNR1B rs10830963 variant in interaction with pre-pregnancy BMI is a pharmacogenetic marker for the initiation of antenatal insulin therapy in gestational diabetes mellitus. Int J Mol Sci (2018) 19(12):3734. doi: 10.3390/ijms19123734
18. Tamanna S, Lumbers ER, Morosin SK, Delforce SJ, Pringle KG. ACE2: a key modulator of the renin-angiotensin system and pregnancy. Am J Physiol Regul Integr Comp Physiol (2021) 321(6):R833–R43. doi: 10.1152/ajpregu.00211.2021
19. Younas H, Ijaz T, Choudhry N. Investigation of angiotensin-1 converting enzyme 2 gene (G8790A) polymorphism in patients of type 2 diabetes mellitus with diabetic nephropathy in Pakistani population. PloS One (2022) 17(2):e0264038. doi: 10.1371/journal.pone.0264038
20. Irani RA, Xia Y. The functional role of the renin-angiotensin system in pregnancy and preeclampsia. Placenta (2008) 29(9):763–71. doi: 10.1016/j.placenta.2008.06.011
21. Song W, Wang H, Ma L, Chen Y. Associations between the TNMD rs4828038 and ACE2 rs879922 polymorphisms and preeclampsia susceptibility: a case-control study. J Obstet Gynaecol (2022) 42(5):1132–6. doi: 10.1080/01443615.2021.2012438
22. He J, Lu YP, Li J, Li TY, Chen X, Liang XJ, et al. Fetal but not maternal angiotensin converting enzyme (ACE)-2 gene Rs2074192 polymorphism is associated with increased risk of being a small for gestational age (SGA) newborn. Kidney Blood Press Res (2018) 43(5):1596–606. doi: 10.1159/000494449
23. Lu CL, Wang Y, Yuan L, Li Y, Li XY. The angiotensin-converting enzyme 2/angiotensin (1-7)/Mas axis protects the function of pancreatic beta cells by improving the function of islet microvascular endothelial cells. Int J Mol Med (2014) 34(5):1293–300. doi: 10.3892/ijmm.2014.1917
24. Zhong JC, Yu XY, Lin QX, Li XH, Huang XZ, Xiao DZ, et al. Enhanced angiotensin converting enzyme 2 regulates the insulin/Akt signalling pathway by blockade of macrophage migration inhibitory factor expression. Br J Pharmacol (2008) 153(1):66–74. doi: 10.1038/sj.bjp.0707482
25. Pringle KG, Tadros MA, Callister RJ, Lumbers ER. The expression and localization of the human placental prorenin/renin-angiotensin system throughout pregnancy: roles in trophoblast invasion and angiogenesis? Placenta (2011) 32(12):956–62. doi: 10.1016/j.placenta.2011.09.020
26. Patel SK, Wai B, Ord M, MacIsaac RJ, Grant S, Velkoska E, et al. Association of ACE2 genetic variants with blood pressure, left ventricular mass, and cardiac function in caucasians with type 2 diabetes. Am J Hypertens (2012) 25(2):216–22. doi: 10.1038/ajh.2011.188
27. Chaoxin J, Daili S, Yanxin H, Ruwei G, Chenlong W, Yaobin T. The influence of angiotensin-converting enzyme 2 gene polymorphisms on type 2 diabetes mellitus and coronary heart disease. Eur Rev Med Pharmacol Sci (2013) 17(19):2654–9.
28. Schmidt CB, Voorhorst I, van de Gaar VHW, Keukens A, Potter van Loon BJ, Snoek FJ, et al. Diabetes distress is associated with adverse pregnancy outcomes in women with gestational diabetes: A prospective cohort study. BMC Pregnancy Childbirth (2019) 19(1):223. doi: 10.1186/s12884-019-2376-6
29. Wacholder S, Chanock S, Garcia-Closas M, El Ghormli L, Rothman N. Assessing the probability that a positive report is false: an approach for molecular epidemiology studies. J Natl Cancer Inst (2004) 96(6):434–42. doi: 10.1093/jnci/djh075
30. Hahn LW, Ritchie MD, Moore JH. Multifactor dimensionality reduction software for detecting gene-gene and gene-environment interactions. Bioinformatics (2003) 19(3):376–82. doi: 10.1093/bioinformatics/btf869
31. Wang M, Marin A. Characterization and prediction of alternative splice sites. Gene (2006) 366(2):219–27. doi: 10.1016/j.gene.2005.07.015
32. Huang D, Yi X, Zhang S, Zheng Z, Wang P, Xuan C, et al. GWAS4D: multidimensional analysis of context-specific regulatory variant for human complex diseases and traits. Nucleic Acids Res (2018) 46(W1):W114–W20. doi: 10.1093/nar/gky407
33. Liu C, Li Y, Guan T, Lai Y, Shen Y, Zeyaweiding A, et al. ACE2 polymorphisms associated with cardiovascular risk in uygurs with type 2 diabetes mellitus. Cardiovasc Diabetology (2018) 17(1):127. doi: 10.1186/s12933-018-0771-3
34. Tong X, Ma Y, Deng H, Wang X, Liu S, Yan Z, et al. The SDF-1 rs1801157 polymorphism is associated with cancer risk: An update pooled analysis and FPRP test of 17,876 participants. Sci Rep (2016) 6:27466. doi: 10.1038/srep27466
35. Jacob AG, Smith CWJ. Intron retention as a component of regulated gene expression programs. Hum Genet (2017) 136(9):1043–57. doi: 10.1007/s00439-017-1791-x
Keywords: angiotensin-converting enzyme 2, gestational diabetes mellitus, risk, variant, association, functional
Citation: Huang G, Liang Q, Wang Y, Qin L, Yang H, Lin L and Yu X (2022) Association of ACE2 gene functional variants with gestational diabetes mellitus risk in a southern Chinese population. Front. Endocrinol. 13:1052906. doi: 10.3389/fendo.2022.1052906
Received: 24 September 2022; Accepted: 31 October 2022;
Published: 01 December 2022.
Edited by:
Marcia Hiriart, Universidad Nacional Autonoma de Mexico, MexicoReviewed by:
Felipe Vadillo-Ortega, Universidad Nacional Autónoma de México, MexicoGuoli Zhou, Michigan State University, United States
Copyright © 2022 Huang, Liang, Wang, Qin, Yang, Lin and Yu. This is an open-access article distributed under the terms of the Creative Commons Attribution License (CC BY). The use, distribution or reproduction in other forums is permitted, provided the original author(s) and the copyright owner(s) are credited and that the original publication in this journal is cited, in accordance with accepted academic practice. No use, distribution or reproduction is permitted which does not comply with these terms.
*Correspondence: Lin Lin, 13489997701@139.com; Xiangyuan Yu, Guilinxiangyuan123@163.com
†These authors have contributed equally to this work and share first authorship