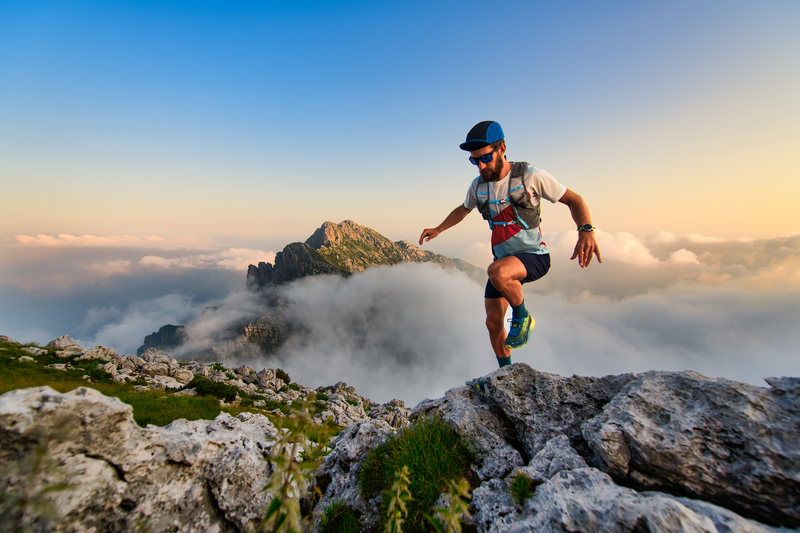
94% of researchers rate our articles as excellent or good
Learn more about the work of our research integrity team to safeguard the quality of each article we publish.
Find out more
ORIGINAL RESEARCH article
Front. Endocrinol. , 13 October 2022
Sec. Pituitary Endocrinology
Volume 13 - 2022 | https://doi.org/10.3389/fendo.2022.1011492
This article is part of the Research Topic Metabolic Effects of Prolactin View all 5 articles
Background and aim: Available evidence suggests elevated serum prolactin (PRL) levels in olanzapine (OLZ)-treated patients with schizophrenia. However, machine learning (ML)-based comprehensive evaluations of the influence of pathophysiological and pharmacological factors on PRL levels in OLZ-treated patients are rare. We aimed to forecast the PRL level in OLZ-treated patients and mine pharmacovigilance information on PRL-related adverse events by integrating ML and electronic health record (EHR) data.
Methods: Data were extracted from an EHR system to construct an ML dataset in 672×384 matrix format after preprocessing, which was subsequently randomly divided into a derivation cohort for model development and a validation cohort for model validation (8:2). The eXtreme gradient boosting (XGBoost) algorithm was used to build the ML models, the importance of the features and predictive behaviors of which were illustrated by SHapley Additive exPlanations (SHAP)-based analyses. The sequential forward feature selection approach was used to generate the optimal feature subset. The co-administered drugs that might have influenced PRL levels during OLZ treatment as identified by SHAP analyses were then compared with evidence from disproportionality analyses by using OpenVigil FDA.
Results: The 15 features that made the greatest contributions, as ranked by the mean (|SHAP value|), were identified as the optimal feature subset. The features were gender_male, co-administration of risperidone, age, co-administration of aripiprazole, concentration of aripiprazole, concentration of OLZ, progesterone, co-administration of sulpiride, creatine kinase, serum sodium, serum phosphorus, testosterone, platelet distribution width, α-L-fucosidase, and lipoprotein (a). The XGBoost model after feature selection delivered good performance on the validation cohort with a mean absolute error of 0.046, mean squared error of 0.0036, root-mean-squared error of 0.060, and mean relative error of 11%. Risperidone and aripiprazole exhibited the strongest associations with hyperprolactinemia and decreased blood PRL according to the disproportionality analyses, and both were identified as co-administered drugs that influenced PRL levels during OLZ treatment by SHAP analyses.
Conclusions: Multiple pathophysiological and pharmacological confounders influence PRL levels associated with effective treatment and PRL-related side-effects in OLZ-treated patients. Our study highlights the feasibility of integration of ML and EHR data to facilitate the detection of PRL levels and pharmacovigilance signals in OLZ-treated patients.
Prolactin (PRL), a polypeptide hormone, is primarily synthesized in and secreted from the anterior pituitary gland, and plays multiple roles in lactation, reproduction, and organ homeostasis (1). PRL secretion is regulated by stimulatory factors like the thyrotropin-releasing hormone (TRH) and inhibitory factors like dopamine (DA) in the hypothalamus, and is influenced by alterations in both physiological (e.g., pregnancy, stress, and sleep states) and pathological conditions (e.g., pituitary disorders, central nervous system disorders, and systemic diseases) (2, 3). Hyperprolactinemia is commonly defined as the condition of a sustained increase in PRL up to that of a fasting level (at least 2 h after waking) of above 20 ng/mL (~424 mIU/L) in men, and above 25 ng/mL (~530 mIU/L) in women (4). It has been found to be associated with an increased risk of many diseases, such as cardiovascular mortality in males (5), cancer (6), bone loss, and fractures (7). In particular, psychiatric patients with hyperprolactinemia usually exhibit short-term sexual dysfunction, amenorrhoea or galactorrhoea, and long-term sequelae such as osteoporosis (8). Recently, a nationwide study in Finland demonstrated that the long-term use of PRL-increasing antipsychotics is significantly associated with the increased risk of breast cancer in females with schizophrenia (9). Studies in vivo and in vitro have revealed that hyperprolactinemia-inducing antipsychotics can prompt precancerous lesions to progress to cancer via activating JAK-STAT5 (10).
A large group of drugs, including psychotropic drugs like antipsychotics, have the potential to cause the hypersecretion of PRL, which is the most common pharmacological cause of hyperprolactinemia (3, 11). In both short- and long-term toxicological studies with rodents, the drug-induced mechanisms of hypo- and hyperprolactinemia commonly involve the dopaminergic system (12). For example, Kunimatsu et al. (13) demonstrated that chronic hyperprolactinemia and maintained corpora lutea causing the decrease of bone density are commonly inducible in female rats undergoing long-term treatment with antipsychotics haloperidol and chlorpromazine (i.e., the DA D2 receptor antagonists). The affinity for DA D2 receptors, the penetration of the blood–brain barrier (BBB), and the dose required to adequately occupy cerebral D2 receptors play major roles in the hyperprolactinemic effects of antipsychotics and other xenobiotics (14). Serotonin (5-HT), which serves as an indirect modulator, also has a stimulatory role in PRL secretion in both the hypothalamus and the pituitary, probably mediated via the stimulation of PRL-releasing factors (15).
Olanzapine (OLZ), an atypical antipsychotic drug, has an intermediary binding affinity for DA D2 receptors, thereby inducing a moderate and dose-dependent elevation of PRL levels (15, 16). It also exerts antipsychotic effects and induces weight gain by blocking the 5-HT2A and 5-HT2c receptors, respectively (16, 17). Thus, the antagonism of OLZ toward the 5-HT2 receptor might partly explain its moderate PRL-elevating tendency (18, 19). In this regard, some gene polymorphisms in DA D2 and 5-HT2A receptors (e.g., DRD2 and 5-HTR2A) have been found to affect PRL levels after OLZ administration (20). A logistic regression analysis of only 10 variables revealed that other risk factors, such as gender, dose, and fasting glucose levels, are also significantly correlated with elevated PRL levels in patients taking OLZ (21). Nevertheless, few studies have investigated the factors influencing PRL levels in OLZ-treated patients in light of multi-dimensional electronic health record (EHR) data. In addition, Wu et al. (19) reported that elevated PRL levels were significantly associated with sexual dysfunction in patients with schizophrenia who had received OLZ treatment. A previous study revealed the alterations in mitochondria of the rat spermatozoa after experimental hyperprolactinemia (22). Recently, an in vivo animal study by Khalaf et al. (23) demonstrated the role of ovarian mitochondrial dysfunction and oxidative stress in ovarian toxicity induced by antipsychotics. On the other hand, the findings by Chen et al. (24) revealed that changes in PRL levels in the course of OLZ treatment are closely correlated with improvement in positive symptoms of schizophrenia, indicating that the PRL level of serum is a useful biological marker for predicting the effectiveness of antipsychotics (25, 26). Hence, monitoring PRL levels during OLZ treatment is vital to minimize the risk of PRL-related adverse events and maximize the response to treatment by antipsychotics.
Interest in Artificial Intelligence (AI)-assisted pharmacovigilance has grown in recent years (27). Within the field of AI, machine learning (ML) is a data-driven computational methodology increasingly applied for predictions of the post-marketing side-effects of drugs (28). The EHR is a source of data for detecting such adverse drug reactions (ADRs) due to its advantages of housing a collection of accurate, detailed, and abundant information on patients (28, 29). For example, On et al. (30) developed ML models for eight types of chemotherapy-induced ADRs (e.g., the nausea–vomiting prediction model) by using EHR data. In addition, it has been demonstrated that ML algorithms allow for the prediction of responses to drug treatment (e.g., antidepressants and anti-cancer drugs) (31, 32) and disease outcomes (e.g., stroke) (33).
In this study, we use the eXtreme gradient boosting (XGBoost) algorithm, a well-known supervised ML algorithm widely used in medicine (34), to construct a model of PRL prediction associated with the side-effects and clinical effectiveness of OLZ by using EHR data. The objectives of this study are to i) develop an XGBoost model for the detection of PRL levels in OLZ-treated patients, and ii) identify multiple factors, particularly co-administered drugs, that may cause hypo- or hyperprolactinemia during OLZ treatment by using an interpretable ML method–the SHapley Additive exPlanations (SHAP) analysis (35). The results are then compared with evidence from real-world disproportionality analyses by using the pharmacovigilance analysis tool OpenVigil FDA (http://openvigil.pharmacology.uni-kiel.de/openvigilfda.php). This online tool uses the “openFDA” API of the US Food and Drug Administration (FDA) to access pharmacovigilance data from the FDA Adverse Event Reporting System (FAERS) (36). The flowchart of this work is shown in Figure 1.
Clinical data on inpatients during OLZ treatment in the latter half of 2018 were mined from the EHR system of the Affiliated Brain Hospital of Guangzhou Medical University in China. The independent ethics committee of the hospital approved the data collection and waived the requirement of informed consent owing to the retrospective nature of our analyses ([2021] No. 027). We obtained 672 PRL measurements of 393 inpatients who had received OLZ treatment, along with information on the patients’ demographic characteristics, diagnoses, history of disorders, and combined medications and biochemical analyses that were determined at the same time points as their PRL levels. Finally, 473 features were identified that, along with the label–PRL, formed the dataset for the ML tasks. A summary of these features is provided in Table 1.
Table 1 A summary of the features of patients taking olanzapine (OLZ) in our original data derived from the EHR system.
Data preprocessing is vital for acquiring high-quality data for modeling. We first omitted the features that had more than 50% missing values and then imputed those with fewer than 50% missing values by using the k-nearest neighbor method (37). Subsequently, min–max normalization and one-hot encoding were applied to the continuous variables and the categorical variables, respectively. Finally, the labels were transformed into the logarithmic scale.
The final dataset in 672×384 matrix format was generated after data preprocessing, and was subsequently randomly divided into a derivation cohort (80%) for model development and a validation cohort (20%) for model validation. The XGBoost algorithm with the default hyperparameter settings was chosen for the regression prediction task. The metrics used for model evaluation were the mean absolute error (MAE), mean squared error (MSE), root-mean-squared error (RMSE), and mean relative error (MRE) (%). They are defined as follows:
Where yi and are the predicted and the actual values, respectively.
Redundant and irrelevant features can increase the computation time, and negatively impact and reduce the learning accuracy of the models. This problem can be solved by feature selection (38). We used the mean absolute SHAP values (|SHAP value|) to illustrate the global importance of features (39). The sequential forward feature selection approach was then employed to generate the optimal feature subset (40). The general practices in this bottom-to-top search method involved starting with an empty feature subset, adding one feature out of the remaining features in each iteration (the order of addition depended on the feature importance calculated by the SHAP values: the more important the feature was, the greater was the precedence it had), and then evaluating the pros and cons of the generated feature subset by using 10-fold cross-validation on the derivation cohort. The optimal feature combination was obtained when “no considerable alteration” of the MAE values was observed in the test sets. Subsequently, SHAP plots were drawn to interpret the contributions of these features to the outputs of the model. Finally, the overall performance of models before and after feature selection was compared on the validation cohort by using the abovementioned metrics.
We focused on co-administered medications that may have an impact on the PRL levels during OLZ treatment. To this end, the results of our SHAP analyses were compared with real-world evidence from disproportionality analyses based on the relative reporting ratio (RRR), a frequentist method, offered by OpenVigil FDA. The RRR was calculated as follows (36):
where N denotes the total number of reports, DE, E, and D denote the numbers of reports when both the drug was used and the event occurred, the drug was used, and the event occurred, respectively.
We used the RRR to compare the strength of associations among a given list of antipsychotics (including OLZ, risperidone, sulpiride, amisulpride, aripiprazole, clozapine, quetiapine, ziprasidone, paliperidone, and perphenazine) with the adverse events “blood prolactin increased,” “hyperprolactinemia,” and “blood prolactin decreased.” The drug with the largest RRR value indicated the most proportional reporting of the reaction for it. Stopping the administration of this drug was thus considered first.
Data processing and modeling were conducted by using the libraries pandas, numpy, scipy, matplotlib, seaborn, missingno, sklearn, XGBoost, shap and palettable. All the ML tasks were implemented in Python by using the Jupyter notebook.
The final dataset consisted of 672 log-transformed PRL label values and 383 features (115 continuous features and 268 categorical features). Figure 2A shows the 110 continuous features with less than 50% missing values, represented by the white lines in each column. Figures 2B, C show the frequency histograms and quantile–quantile (Q–Q) plots of the labels before and after log-transformation, respectively. They indicated that the distribution of the log-transformed PRL ranging from 0.44 to 1 was the most symmetric and normal. Table 2 shows the descriptions of the labels and partial features in our original data, without data preprocessing, derived from the EHR system.
Figure 2 (A) Visualizing the missing data for features with fewer than 50% missing values by using the missingno library. Frequency histograms and quantile–quantile (Q–Q) plots of (B) PRL and (C) log-transformed PRL [calculated by log10(PRL)/log10(PRLmax)].
Figure 3A presents the trend of evolution of the decline in the MAE in the training and test sets of the derivation cohort by using the forward feature selection strategy based on feature importance computed by using SHAP values. The top 15 features were identified as the optimal feature subset because the MAE declined imperceptibly with the addition of subsequent features. They were ranked according to the mean (|SHAP value|) as follows (Figure 3B): gender_male, co-administration of risperidone (Risperidone), age, co-administration of aripiprazole (Aripiprazole), concentration of aripiprazole (C_Aripiprazole), concentration of OLZ (C_OLZ), progesterone, co-administration of sulpiride (Sulpiride), creatine kinase (CK), serum sodium (Na), serum phosphorus (P), testosterone, platelet distribution width (PDW), α-L-fucosidase (AFU), and lipoprotein (a) [Lp(a)]. Figure 3C shows the Pearson’s correlations between the log-transformed PRL and these features, and indicates no prominent multi-collinear relationships among the features. Figure 3D presents the direction of effects of a variable on the output of the model. The SHAP dependence plots of these features show how they affected the outputs of our model (Figure 4). They indicate that higher PRL levels were related to females as well as the concomitant use of risperidone and sulpiride, and co-administered aripiprazole might have caused lower PRL levels. The comparisons of the influences of these three co-administered antipsychotics on PRL levels in terms of the gender of patients taking OLZ, based on the original data derived from the EHR system, are presented in Figure 5.
Figure 3 (A) The evolution of the performance of XGBoost models on the derivation cohort based on different compositions of the feature set. (B) The ranking by importance of the top 15 features according to the mean (|SHAP value|). (C) Heat map of the correlations between the log-transformed PRL and the selected features as analyzed by Pearson’s correlation coefficient. (D) The SHAP summary plot of the top 15 features. The red (blue) dots denote the high (low) values of the features. The high (low) SHAP values of the features denote their high (low) log-transformed PRL values.
Figure 4 The SHAP dependence plots of the top 15 features. The SHAP values that exceeded zero represent high log-transformed PRL values, and vice versa.
Figure 5 Comparisons of the influence of the co-administered risperidone, aripiprazole, and sulpiride on the PRL levels in terms of the gender of patients taking olanzapine (OLZ) according to the original data. The green multiplication sign indicates the mean PRL values.
An overall comparison of the performance of models before and after feature selection is listed in Table 3. Among them, the XGBoost model after feature selection had better predictive performance, with lower values of the MAE, MSE, RMSE, and MRE. The lack of clear patterns and the symmetrical distribution of the residuals indicated that our proposed XGBoost model after feature selection was suitable for fitting the data in the validation cohort (Figures 6A, B). Figure 6C shows that 47.41% and 68.89% of the predicted values were within ranges of ±30% and ±50% of the actual values, respectively.
Table 3 Comparison of performance of XGBoost models on the validation cohort (N = 135) before and after feature selection.
Figure 6 (A) Plot of residuals vs. the predicted log-transformed PRL values. (B) The normal plot of the residuals. (C) Scatterplot of the predicted PRL values vs. actual PRL values.
Table 4 shows comparisons of the disproportionality measures for the 10 antipsychotics and PRL-related adverse events. Risperidone, paliperidone, and amisulpride were the top three antipsychotics associated with the risk of increased PRL concentration in the blood and hyperprolactinemia according to their RRR values. Similarly, aripiprazole exhibited the strongest association with decreased PRL content in blood, indicating that its use may protect patients taking OLZ from hyperprolactinemia.
Table 4 Relative reporting ratios (RRR) of prolactin (PRL)-related adverse events for a given list of antipsychotics.
ML techniques have been successfully and widely applied to multiple fields in medicine, such as cancer diagnostics (41), treatment outcome predictions in patients with first-episode psychosis (42), and exploration of risk factors for the use of direct coercive measures in offender patients with schizophrenia spectrum disorders (43). AI-assisted pharmacovigilance has made large advancements but is still in its infancy. Chandak et al. (44) used ML to identify adverse drug effects posing increased risk to women based on public databases. We proposed a novel strategy that integrated ML with real-world clinical data of representative Chinese patient populations to advance AI-assisted pharmacovigilance studies. To the best of the authors’ knowledge, this is the first study to forecast the PRL level in OLZ-treated patients and mine pharmacovigilance information on PRL-related adverse events by integrating ML and EHR data. We used the XGBoost algorithm to construct an accurate model of PRL prediction that uses only two demographic characteristic predictors (i.e., gender_male and age), five predictors of drug information (i.e., risperidone, aripiprazole, C_Aripiprazole, C_OLZ, and sulpiride), and eight predictors of biochemical metrics [i.e., progesterone, CK, Na, P, testosterone, PDW, AFU, and Lp(a)]. This helps better understand these confounders that influence the PRL levels in OLZ-treated patients. They were found to be associated with the effectiveness of treatment and PRL-related side-effects.
The XGBoost algorithm, an ensemble learning method under the gradient boosting framework, is a scalable and distributed gradient-boosted decision tree ML library that allows for parallel tree boosting in both the classification and the regression tasks. It thus provides fast and accurate solutions for many problems in data science (45). It is generally considered to be a “black-box” model that loses the interpretability of the relationships between the inputs and the outputs of the models (46). In this study, we computed feature importance of the black-box XGBoost model by using the SHAP library, which used the SHAP values from game theory to estimate the contribution of each feature to the prediction in a model-agnostic manner (47). Furthermore, we drew more plots of interpretation, such as the SHAP summary plot and SHAP dependence plots, to show the general direction of influence and distributions of the SHAP outputs of each feature in the XGBoost model (48). In particular, our SHAP dependence plots demonstrated a non-linear relationship between C_OLZ and PRL, namely, a prominent trend of increase in the log-transformed PRL was observed as the normalized C_OLZ ranged from zero to approximately 0.4, and the trend of subsequent increase was not apparent. Moreover, we found that the log-transformed PRL was positively correlated with the range of the normalized C_OLZ from approximately 0.2 to 0.8 (corresponding to the C_OLZ ranging from approximately 25.03 ng/mL to 100.14 ng/mL). This range is close to the recommended therapeutic reference range (i.e., 20–80 ng/mL) and the laboratory alert level (i.e., 100 ng/mL) of OLZ according to the latest consensus-based guidelines of the Arbeitsgemeinschaft für Neuropsychopharmakologie und Pharmakopsychiatrie (AGNP)-Therapeutic Drug Monitoring (TDM) expert group (49). On the other hand, typical PRL levels with regard to the etiology of drugs are 25–100 ng/mL (approximately 530–2120 mIU/L) (50), which is broadly in line with our PRL measurements of OLZ-treated patients (see Figure 2B). Severe drug-related hyperprolactinemia (commonly defined as PRL values above 100 ng/mL), in the context of 100–250 ng/mL (approximately 2120–5300 mIU/L) and > 250 ng/mL (> ~5300 mIU/L), were found to occur in ~30% and ~5% of the cases (particularly with antipsychotics), respectively (50, 51). The aforementioned findings show that PRL may be a potential biological correlate for predicting the therapeutic effectiveness of OLZ and its PRL-related side-effects as well as their severity.
We also identified multiple confounders that might have influenced the PRL levels. They involved pathophysiological and pharmacological factors. The most important one was gender, i.e., females had more elevated RPL levels than males. A possible explanation for this is that females were more affected by antipsychotic-induced hyperprolactinemia than males (52). Likewise, our study revealed age-related changes in PRL levels, indicating that age is important because concomitant drug use and illnesses such as hypothyroidism and the degeneration of the ovarian secretion function, may be common in elderly populations (53, 54). Other hormones, including progesterone and testosterone, were also demonstrated to have effects on the PRL level. PRL commonly works antagonistically with estrogen and testosterone. It can inhibit the secretion of gonadotropin-releasing hormone by modulating the dopaminergic pathway, and thus may reduce testosterone levels associated with hypogonadism (55). It also acts directly on the granulosa cells of Graafian follicles to stimulate the release of progesterone and suppress estradiol production (56). The results indicated that hormone replacement (estrogen or testosterone) therapy may be an alternative pharmacological treatment strategy for hyperprolactinemia (57, 58). However, this strategy for antipsychotic-induced hyperprolactinemia is not recommended based on the latest evidence from network meta-analyses (59). PDW directly reflects the variability in platelet size, is considered to be an indicator of platelet activation and function, and is thus related to the extent of coronary artery disease (60). There are discrepancies in findings among some studies regarding the effects of PRL on platelet activation. Previous studies have demonstrated that hyperprolactinemia can cause adenosine diphosphate (ADP)-stimulated platelet activation, particularly in patients treated with antipsychotics. This might explain the increased risk for venous thromboembolism among them (61, 62). By contrast, Reuwer et al. (63) have suggested that PRL does not affect platelet aggregation or secretion in humans. Wahlberg et al. (64) found that PRL affected platelets in hyperprolactinemic patients in an indirect inhibitory way, indicating that it might have a protective role in thromboembolic disease. The positive correlation between PDW and the log-transformed PRL in our study suggests that hyperprolactinemia in OLZ-treated patients might be associated with the increased risk of thromboembolic events because PDW increases during platelet activation (65). Moreover, PRL may have direct effects on the metabolism of lipids—for example, reducing lipoprotein lipase activity in the human adipose tissue (66). Nevertheless, the positive correlation between the log-transformed PRL and Lp(a) in OLZ-treated patients, which has not been reported in any previous study to the best of our knowledge, indicates that increased cardiovascular risk in OLZ-treated patients with hyperprolactinemia should be the focus of research in this context, especially in males (5), as Lp(a) is an independent marker of cardiovascular risk (67). Our study also revealed the negative correlation between the log-transformed PRL and Na. This result is in line with that of a previous study suggesting that serum PRL may participate in sodium retention (68). CK, P, and AFU also played contributory roles in the log-transformed PRL levels, although few past studies have revealed the detailed mechanisms underlying their relationships. They possibly involve other physiological processes, such as bone mineral liberation and glycoprotein metabolism (69, 70).
In addition to pathophysiological factors, the concomitant use of risperidone, aripiprazole, and sulpiride were identified as the top three pharmacological contributors to the effects of PRL levels in OLZ-treated patients. These findings are largely consistent with our disproportionality analyses of PRL-related adverse events with antipsychotics, which suggest that risperidone should be discontinued first in OLZ-treated patients with hyperprolactinemia, and that the use of aripiprazole may protect them from this adverse event. Therefore, our study has highlighted the feasibility of AI-based pharmacovigilance detection in resource-limited settings by extracting various sources of data recorded in the EHR (71). Compared with OLZ and other atypical antipsychotics, risperidone is more likely to cause hyperprolactinemia owing to its incomplete crossing of the BBB, where this results in higher occupancy by DA D2 receptors in the pituitary gland than in the striatum (72). Compared to OLZ, risperidone demonstrated a more robust and persistent increase in PRL over a 24-h period in rats following acute and chronic administration (73). Aripiprazole is a partial agonist to the dopaminergic receptor D2, and has been endorsed by some guidelines for the treatment of antipsychotic-induced hyperprolactinemia (74, 75). Adjunctive aripiprazole or switching to aripiprazole in titration has been proved to be good PRL decrease effects (more than 50 ng/mL) for antipsychotic-induced hyperprolactinemia (59). Our work revealed gender-specific differences in these decreased effects (see Figure 5). Moreover, as a suitable alternative for OLZ-induced hyperprolactinemia, aripiprazole may not only diminish PRL levels but may also clear PRL-related symptoms that may occur with borderline or normal standardized PRL values while maintaining clinical stabilization (76). Notably, an abnormally low PRL level after switching to aripiprazole might occur, and this is a potential warning sign of a psychotic rebound. Routinely monitoring PRL levels may help avoid such a rebound (77). Eftekhari et al. (78) reported that oxidative stress and mitochondrial dysfunction played key roles in liver injury caused by OLZ, indicating that antioxidants, particular the nanoantioxidants involving increased bioavailability, stability, and target specificity (79, 80), were able to prevent OLZ-induced toxicity related to hyperprolactinemia (e.g., sexual dysfunction) (22, 23). Components of chamomile, a fascinating, well-known, and widely used medicinal plant, have effects on osteoporosis prevention, as well as the potent antioxidant, anti-inflammatory, and anti-cancer activities, which seem to be effective in the treatment of idiopathic hyperprolactinemia (75, 81). Therefore, antioxidants and herbal medications could be management options in OLZ-induced hyperprolactinemia when switching the antipsychotic is not an option.
Several limitations of this study should be noted. First, the small sample size might have affected the power of ML because the smaller the dataset is, the less powerful and less accurate are the results of ML algorithms (82). Thus, a larger number of samples is needed to improve the model generalization capability of the model. Second, although our findings were in accordance with previous reports demonstrating no influence of CYP2D6 variation on PRL levels in antipsychotic-induced hyperprolactinemia (83), some candidate genes associated with changes in PRL were not included in our study. For example, DRD2 may influence the susceptibility to hyperprolactinemia associated with OLZ treatment (84). Genetic associations of alterations in PRL in OLZ-treated patients may warrant further exploration. The confounding factors influencing the sex differences of pharmacovigilance signals on PRL-related adverse events and the pharmacogenetic mechanisms to explain these sex risks may be our future works.
In this study, we constructed an ML-based model of PRL prediction in OLZ-treated patients by using the XGBoost algorithm and EHR data. Based on SHAP analyses, we also identified multiple pathophysiological and pharmacological confounders that influence PRL levels as tightly related to the effectiveness of treatment and PRL-related side-effects in OLZ-treated patients. Furthermore, our work suggests the feasibility of AI-based pharmacovigilance detection by using EHR as a source of data. In short, ML and EHR data can partner to facilitate the detection of PRL levels and pharmacovigilance signals in OLZ-treated patients.
The original contributions presented in the study are included in the article/Supplementary Material. Further inquiries can be directed to the corresponding authors.
The studies involving human participants were reviewed and approved by the independent ethics committee of the Affiliated Brain Hospital of Guangzhou Medical University. Written informed consent from the participants’ legal guardian/next of kin was not required to participate in this study in accordance with the national legislation and the institutional requirements.
DS and YW together conceived and designed the study. XZ wrote the original draft preparation. TX and SH performed the data collection. JH conducted the data analyses. All authors contributed to the article and approved the submitted version.
This work was supported by the Guangzhou municipal key discipline in medicine (2021-2023), Guangzhou Municipal Science and Technology Project for Medicine and Healthcare (grant numbers 20201A011047 and 20202A011016), Natural Science Foundation of Guangdong Province (grant numbers 2018A0303130074 and 2021A1515011325), Guangdong Provincial Hospital Pharmaceutical Research Fund (grant number 2022A22) and Science and Technology Plan Project of Guangdong Province (grant number 2019B030316001).
We thank International Science Editing (http://www.internationalscienceediting.com) for editing this manuscript.
The authors declare that the research was conducted in the absence of any commercial or financial relationships that could be construed as a potential conflict of interest.
All claims expressed in this article are solely those of the authors and do not necessarily represent those of their affiliated organizations, or those of the publisher, the editors and the reviewers. Any product that may be evaluated in this article, or claim that may be made by its manufacturer, is not guaranteed or endorsed by the publisher.
1. Freeman ME, Kanyicska B, Lerant A, Nagy G. Prolactin: structure, function, and regulation of secretion. Physiol Rev (2000) 80(4):1523–631. doi: 10.1152/physrev.2000.80.4.1523
2. Egli M, Leeners B, Kruger TH. Prolactin secretion patterns: basic mechanisms and clinical implications for reproduction. Reproduction (2010) 140(5):643–54. doi: 10.1530/REP-10-0033
3. Torre DL, Falorni A. Pharmacological causes of hyperprolactinemia. Ther Clin Risk Manage (2007) 3(5):929–51.
4. Halbreich U, Kinon BJ, Gilmore JA, Kahn LS. Elevated prolactin levels in patients with schizophrenia: mechanisms and related adverse effects. Psychoneuroendocrinology (2003) 28 Suppl 1:53–67. doi: 10.1016/s0306-4530(02)00112-9
5. Krogh J, Selmer C, Torp-Pedersen C, Gislason GH, Kistorp C. Hyperprolactinemia and the association with all-cause mortality and cardiovascular mortality. Horm Metab Res (2017) 49(6):411–7. doi: 10.1055/s-0043-107243
6. Berinder K, Akre O, Granath F, Hulting AL. Cancer risk in hyperprolactinemia patients: A population-based cohort study. Eur J Endocrinol (2011) 165(2):209–15. doi: 10.1530/EJE-11-0076
7. di Filippo L, Doga M, Resmini E, Giustina A. Hyperprolactinemia and bone. Pituitary (2020) 23(3):314–21. doi: 10.1007/s11102-020-01041-3
8. Holt RI. Medical causes and consequences of hyperprolactinaemia. A Context Psychiatrists J Psychopharmacol (2008) 22(2 Suppl):28–37. doi: 10.1177/0269881107087951
9. Taipale H, Solmi M, Lähteenvuo M, Tanskanen A, Correll CU, Tiihonen J. Antipsychotic use and risk of breast cancer in women with schizophrenia: A nationwide nested case-control study in Finland. Lancet Psychiatry (2021) 8(10):883–91. doi: 10.1016/S2215-0366(21)00241-8
10. Johnston AN, Bu W, Hein S, Garcia S, Camacho L, Xue L, et al. Hyperprolactinemia-inducing antipsychotics increase breast cancer risk by activating JAK-STAT5 in precancerous lesions. Breast Cancer Res (2018) 20(1):42. doi: 10.1186/s13058-018-0969-z
11. Milano W, Colletti C, Capasso A. Hyperprolactinemia induced by antipsychotics: from diagnosis to treatment approach. Endocr Metab Immune Disord Drug Targets (2017) 17(1):38–55. doi: 10.2174/1871530317666170424102332
12. Hargreaves A, Harleman J. Preclinical risk assessment of drug-induced hypo- and hyperprolactinemia. J Appl Toxicol (2011) 31(7):599–607. doi: 10.1002/jat.1723
13. Kunimatsu T, Kimura J, Funabashi H, Inoue T, Seki T. The antipsychotics haloperidol and chlorpromazine increase bone metabolism and induce osteopenia in female rats. Regul Toxicol Pharmacol (2010) 58(3):360–8. doi: 10.1016/j.yrtph.2010.08.001
14. Voicu V, Medvedovici A, Ranetti AE, Rădulescu FŞ. Drug-induced hypo- and hyperprolactinemia: mechanisms, clinical and therapeutic consequences. Expert Opin Drug Metab Toxicol (2013) 9(8):955–68. doi: 10.1517/17425255.2013.791283
15. Peuskens J, Pani L, Detraux J, De Hert M. The effects of novel and newly approved antipsychotics on serum prolactin levels: A comprehensive review. CNS Drugs (2014) 28(5):421–53. doi: 10.1007/s40263-014-0157-3
16. Bhana N, Foster RH, Olney R, Plosker GL. Olanzapine: an updated review of its use in the management of schizophrenia. Drugs (2001) 61(1):111–61. doi: 10.2165/00003495-200161010-00011
17. Lord CC, Wyler SC, Wan R, Castorena CM, Ahmed N, Mathew D, et al. The atypical antipsychotic olanzapine causes weight gain by targeting serotonin receptor 2C. J Clin Invest (2017) 127(9):3402–6. doi: 10.1172/JCI93362
18. Cowen PJ, Anderson IM, Gartside SE. Endocrinological responses to 5-HT. Ann N Y Acad Sci (1990) 600:250–257.discussion 257-259. doi: 10.1111/j.1749-6632.1990.tb16887.x
19. Wu TH, Lin CH, Goh KK, Chen CY, Chen CH, Lane HY, et al. The relationships between hyperprolactinemia, metabolic disturbance, and sexual dysfunction in patients with schizophrenia under olanzapine treatment. Front Pharmacol (2021) 12:718800. doi: 10.3389/fphar.2021.718800
20. Cabaleiro T, López-Rodríguez R, Ochoa D, Román M, Novalbos J, Abad-Santos F. Polymorphisms influencing olanzapine metabolism and adverse effects in healthy subjects. Hum Psychopharmacol (2013) 28(3):205–14. doi: 10.1002/hup.2308
21. He S, Yu WJ, Wang X, Zhang L, Zhao N, Li G, et al. Risk factors of hyperprolactinemia induced by risperidone and olanzapine and their correlations with plasma glucose and lipids. Gen Psychiatr (2020) 33(4):e100206. doi: 10.1136/gpsych-2020-100206
22. Laszczyńska M, Piasecka M, Kram A. Alterations in the mitochondria of rat spermatozoa after experimental hyperprolactinemia. Folia Histochem Cytobiol (1999) 37(2):87–8.
23. Khalaf HA, Elmorsy E, Mahmoud EM, Aggour AM, Amer SA. The role of oxidative stress in ovarian toxicity induced by haloperidol and clozapine-a histological and biochemical study in albino rats. Cell Tissue Res (2019) 378(2):371–83. doi: 10.1007/s00441-019-03067-x
24. Chen YL, Cheng TS, Lung FW. Prolactin levels in olanzapine treatment correlate with positive symptoms of schizophrenia: results from an open-label, flexible-dose study. Prim Care Companion J Clin Psychiatry (2009) 11(1):16–20. doi: 10.4088/pcc.08m00668
25. Gault JM, Nussbaum AM. Review of serum prolactin levels as an antipsychotic-response biomarker. Open Access J Transl Med Res (2018) 2(3):84–91. doi: 10.15406/oajtmr.2018.02.00043
26. Agarwal SM, Rao NP, Jhamnani K, Venkatasubramanian G, Behere RV, Varambally S, et al. Prolactin-a biomarker for antipsychotic treatment response? Prim Care Companion CNS Disord (2011) 13(4):PCC.11l01155. doi: 10.4088/PCC.11l01155
27. Hauben M, Hartford CG. Artificial intelligence in pharmacovigilance: scoping points to consider. Clin Ther (2021) 43(2):372–9. doi: 10.1016/j.clinthera.2020.12.014
28. Lee CY, Chen YP. Machine learning on adverse drug reactions for pharmacovigilance. Drug Discov Today (2019) 24(7):1332–43. doi: 10.1016/j.drudis.2019.03.003
29. Banda JM, Callahan A, Winnenburg R, Strasberg HR, Cami A, Reis BY, et al. Feasibility of prioritizing drug-drug-event associations found in electronic health records. Drug Saf (2016) 39(1):45–57. doi: 10.1007/s40264-015-0352-2
30. On J, Park HA, Yoo S. Development of a prediction models for chemotherapy-induced adverse drug reactions: A retrospective observational study using electronic health records. Eur J Oncol Nurs (2022) 56:102066. doi: 10.1016/j.ejon.2021.102066
31. Kautzky A, Möller HJ, Dold M, Bartova L, Seemüller F, Laux G, et al. Combining machine learning algorithms for prediction of antidepressant treatment response. Acta Psychiatr Scand (2021) 143(1):36–49. doi: 10.1111/acps.13250
32. Sammut SJ, Crispin-Ortuzar M, Chin SF, Provenzano E, Bardwell HA, Ma W, et al. Multi-omic machine learning predictor of breast cancer therapy response. Nature (2022) 601(7894):623–9. doi: 10.1038/s41586-021-04278-5
33. Heo J, Yoon JG, Park H, Kim YD, Nam HS, Heo JH. Machine learning-based model for prediction of outcomes in acute stroke. Stroke (2019) 50(5):1263–5. doi: 10.1161/STROKEAHA.118.024293
34. Khera R, Haimovich J, Hurley NC, McNamara R, Spertus JA, Desai N, et al. Use of machine learning models to predict death after acute myocardial infarction. JAMA Cardiol (2021) 6(6):633–41. doi: 10.1001/jamacardio.2021.0122
35. Lundberg SM, Lee SI. (2017). A unified approach to interpreting model predictions, in: Proceedings of the 31st international conference on neural information processing systems (NIPS’17), pp. 4768–77. Red Hook, NY, USA: Curran Associates Inc. Available at: https://dl.acm.org/doi/pdf/10.5555/3295222.3295230.
36. Böhm R, von Hehn L, Herdegen T, Klein HJ, Bruhn O, Petri H, et al. OpenVigil FDA - inspection of U.S. American adverse drug events pharmacovigilance data and novel clinical applications. PloS One (2016) 11(6):e0157753. doi: 10.1371/journal.pone.0157753
37. Beretta L, Santaniello A. Nearest neighbor imputation algorithms: A critical evaluation. BMC Med Inform Decis Mak (2016) 16 Suppl 3(Suppl 3):74. doi: 10.1186/s12911-016-0318-z
38. Cai J, Luo JW, Wang SL, Yang S. Feature selection in machine learning: A new perspective. Neurocomputing (2018) 300:70–9. doi: 10.1016/j.neucom.2017.11.077
39. Gong X, Zheng B, Xu G, Chen H, Chen C. Application of machine learning approaches to predict the 5-year survival status of patients with esophageal cancer. J Thorac Dis (2021) 13(11):6240–51. doi: 10.21037/jtd-21-1107
40. Aatila M, Lachgar M, Hamid H, Kartit A. Keratoconus severity classification using features selection and machine learning algorithms. Comput Math Methods Med (2021) 2021:9979560. doi: 10.1155/2021/9979560
41. Benning L, Peintner A, Peintner L. Advances in and the applicability of machine learning-based screening and early detection approaches for cancer: A primer. Cancers (Basel) (2022) 14(3):623. doi: 10.3390/cancers14030623
42. Koutsouleris N, Kahn RS, Chekroud AM, Leucht S, Falkai P, Wobrock T, et al. Multisite prediction of 4-week and 52-week treatment outcomes in patients with first-episode psychosis: A machine learning approach. Lancet Psychiatry (2016) 3(10):935–46. doi: 10.1016/S2215-0366(16)30171-7
43. Günther MP, Kirchebner J, Lau S. Identifying direct coercion in a high risk subgroup of offender patients with schizophrenia via machine learning algorithms. Front Psychiatry (2020) 11:415. doi: 10.3389/fpsyt.2020.00415
44. Chandak P, Tatonetti NP. Using machine learning to identify adverse drug effects posing increased risk to women. Patterns (N Y) (2020) 1(7):100108. doi: 10.1016/j.patter.2020.100108
45. Chen T, Guestrin C. (2016). XGBoost: A scalable tree boosting system, in: KDD ‘16: proceedings of the 22nd ACM SIGKDD international conference on knowledge discovery and data mining 2016,. pp. 785–94. (New York, NY, United States: Association for Computing Machinery). doi: 10.1145/2939672.2939785
46. Linardatos P, Papastefanopoulos V, Kotsiantis S. Explainable AI: A review of machine learning interpretability methods. Entropy (Basel) (2020) 23(1):18. doi: 10.3390/e23010018
47. Štrumbelj E, Kononenko I. Explaining prediction models and individual predictions with feature contributions. Knowl Inf Syst (2014) 41:647–65. doi: 10.1007/s10115-013-0679-x
48. Zhang Y, Yang D, Liu Z, Chen C, Ge M, Li X, et al. An explainable supervised machine learning predictor of acute kidney injury after adult deceased donor liver transplantation. J Transl Med (2021) 19(1):321. doi: 10.1186/s12967-021-02990-4
49. Hiemke C, Bergemann N, Clement HW, Conca A, Deckert J, Domschke K, et al. Consensus guidelines for therapeutic drug monitoring in neuropsychopharmacology: update 2017. Pharmacopsychiatry (2018) 51(1-02):9–62. doi: 10.1055/s-0043-116492
50. Rizzo LFL, Mana DL, Serra HA, Danilowicz K. Hyperprolactinemia associated with psychiatric disorders. Med (B Aires) (2020) 80(6):670–80.
51. Montejo ÁL, Arango C, Bernardo M, Carrasco JL, Crespo-Facorro B, Cruz JJ, et al. Spanish Consensus on the risks and detection of antipsychotic drug-related hyperprolactinaemia. Rev Psiquiatr Salud Ment (2016) 9(3):158–73. doi: 10.1016/j.rpsm.2015.11.003
52. Smith S, Wheeler MJ, Murray R, O’Keane V. The effects of antipsychotic-induced hyperprolactinaemia on the hypothalamic-pituitary-gonadal axis. J Clin Psychopharmacol (2002) 22(2):109–14. doi: 10.1097/00004714-200204000-00002
53. Sawin CT, Carlson HE, Geller A, Castelli WP, Bacharach P. Serum prolactin and aging: basal values and changes with estrogen use and hypothyroidism. J Gerontol (1989) 44(4):M131–5. doi: 10.1093/geronj/44.4.m131
54. González-Rodríguez A, Labad J, Seeman MV. Antipsychotic-induced hyperprolactinemia in aging populations: prevalence, implications, prevention and management. Prog Neuropsychopharmacol Biol Psychiatry (2020) 101:109941. doi: 10.1016/j.pnpbp.2020.109941
55. Darbandi M, Darbandi S, Agarwal A, Sengupta P, Durairajanayagam D, Henkel R, et al. Reactive oxygen species and male reproductive hormones. Reprod Biol Endocrinol (2018) 16(1):87. doi: 10.1186/s12958-018-0406-2
56. Wang C, Chan V. Divergent effects of prolactin on estrogen and progesterone production by granulosa cells of rat graafian follicles. Endocrinology (1982) 110(4):1085–93. doi: 10.1210/endo-110-4-1085
57. Molitch ME. Medication-induced hyperprolactinemia. Mayo Clin Proc (2005) 80(8):1050–7. doi: 10.4065/80.8.1050
58. Miller KK. Management of hyperprolactinemia in patients receiving antipsychotics. CNS Spectr (2004) 9(8 Suppl 7):28–32. doi: 10.1017/s1092852900002340
59. Lu Z, Sun Y, Zhang Y, Chen Y, Guo L, Liao Y, et al. Pharmacological treatment strategies for antipsychotic-induced hyperprolactinemia: A systematic review and network meta-analysis. Transl Psychiatry (2022) 12(1):267. doi: 10.1038/s41398-022-02027-4
60. De Luca G, Venegoni L, Iorio S, Secco GG, Cassetti E, Verdoia M, et al. Platelet distribution width and the extent of coronary artery disease: results from a large prospective study. Platelets (2010) 21(7):508–14. doi: 10.3109/09537104.2010.494743
61. Anaforoglu I, Ertorer ME, Kozanoglu I, Unal B, Haydardedeoglu FE, Bakiner O, et al. Macroprolactinemia, like hyperprolactinemia, may promote platelet activation. Endocrine (2010) 37(2):294–300. doi: 10.1007/s12020-009-9304-x
62. Wallaschofski H, Eigenthaler M, Kiefer M, Donné M, Hentschel B, Gertz HJ, et al. Hyperprolactinemia in patients on antipsychotic drugs causes ADP-stimulated platelet activation that might explain the increased risk for venous thromboembolism: pilot study. J Clin Psychopharmacol (2003) 23(5):479–83. doi: 10.1097/01.jcp.0000088914.24613.51
63. Reuwer AQ, Nieuwland R, Fernandez I, Goffin V, van Tiel CM, Schaap MC, et al. Prolactin does not affect human platelet aggregation or secretion. Thromb Haemost (2009) 101(6):1119–27. doi: 10.1016/S1567-5688(09)70307-1
64. Wahlberg J, Tillmar L, Ekman B, Lindahl TL, Landberg E. Effects of prolactin on platelet activation and blood clotting. Scand J Clin Lab Invest (2013) 73(3):221–8. doi: 10.3109/00365513.2013.765963
65. Vagdatli E, Gounari E, Lazaridou E, Katsibourlia E, Tsikopoulou F, Labrianou I. Platelet distribution width: A simple, practical and specific marker of activation of coagulation. Hippokratia (2010) 14(1):28–32.
66. Ling C, Svensson L, Odén B, Weijdegård B, Edén B, Edén S, et al. Identification of functional prolactin (PRL) receptor gene expression: PRL inhibits lipoprotein lipase activity in human white adipose tissue. J Clin Endocrinol Metab (2003) 88(4):1804–8. doi: 10.1210/jc.2002-021137
67. Saeedi R, Frohlich J. Lipoprotein (a), an independent cardiovascular risk marker. Clin Diabetes Endocrinol (2016) 2:7. doi: 10.1186/s40842-016-0024-x
68. Hanssen KF, Torjesen PA. Increased serum prolactin in diabetic ketoacidosis; correlation between serum sodium and serum prolacting concentration. Acta Endocrinol (Copenh) (1977) 85(2):372–8. doi: 10.1530/acta.0.0850372
69. Pahuja DN, DeLuca HF. Stimulation of intestinal calcium transport and bone calcium mobilization by prolactin in vitamin d-deficient rats. Science (1981) 214(4524):1038–9. doi: 10.1126/science.7302575
70. Jayakumar PG, Arunakaran J, Aruldhas MM, Govindarajulu P. Role of prolactin on epididymal glycoprotein metabolism in matured monkeys, macaca radiata: specific activities of glycosyltransferases and glycosidases. Indian J Exp Biol (1992) 30(11):1075–8.
71. Liang L, Hu J, Sun G, Hong N, Wu G, He Y, et al. Artificial intelligence-based pharmacovigilance in the setting of limited resources. Drug Saf (2022) 45(5):511–9. doi: 10.1007/s40264-022-01170-7
72. Stojkovic M, Radmanovic B, Jovanovic M, Janjic V, Muric N, Ristic DI. Risperidone induced hyperprolactinemia: From basic to clinical studies. Front Psychiatry (2022) 13:874705. doi: 10.3389/fpsyt.2022.874705
73. Rourke C, Starr KR, Reavill C, Fenwick S, Deadman K, Jones DN. Effects of the atypical antipsychotics olanzapine and risperidone on plasma prolactin levels in male rats: A comparison with clinical data. Psychopharmacol (Berl) (2006) 184(1):107–14. doi: 10.1007/s00213-005-0230-1
74. Grigg J, Worsley R, Thew C, Gurvich C, Thomas N, Kulkarni J. Antipsychotic-induced hyperprolactinemia: synthesis of world-wide guidelines and integrated recommendations for assessment, management and future research. Psychopharmacol (Berl) (2017) 234(22):3279–97. doi: 10.1007/s00213-017-4730-6
75. Rusgis MM, Alabbasi AY, Nelson LA. Guidance on the treatment of antipsychotic-induced hyperprolactinemia when switching the antipsychotic is not an option. Am J Health Syst Pharm (2021) 78(10):862–71. doi: 10.1093/ajhp/zxab065
76. Barata PC, Santos MJ, Melo JC, Maia T. Olanzapine-induced hyperprolactinemia: two case reports. Front Pharmacol (2019) 10:846. doi: 10.3389/fphar.2019.00846
77. Jen YW, Hwang TJ, Chan HY, Hsieh MH, Liu CC, Liu CM, et al. Abnormally low prolactin levels in schizophrenia patients after switching to aripiprazole in a randomized trial: A biomarker for rebound in psychotic symptoms? BMC Psychiatry (2020) 20(1):552. doi: 10.1186/s12888-020-02957-7
78. Eftekhari A, Azarmi Y, Parvizpur A, Eghbal MA. Involvement of oxidative stress and mitochondrial/lysosomal cross-talk in olanzapine cytotoxicity in freshly isolated rat hepatocytes. Xenobiotica (2016) 46(4):369–78. doi: 10.3109/00498254.2015.1078522
79. Omran B, Baek KH. Nanoantioxidants: pioneer types, advantages, limitations, and future insights. Molecules (2021) 26(22):7031. doi: 10.3390/molecules26227031
80. Khalil I, Yehye WA, Etxeberria AE, Alhadi AA, Dezfooli SM, Julkapli NBM, et al. Nanoantioxidants: recent trends in antioxidant delivery applications. Antioxid (Basel) (2019) 9(1):24. doi: 10.3390/antiox9010024
81. Kabiri M, Kamalinejad M, Bioos S, Shariat M, Sohrabvand F. Comparative study of the effects of chamomile (matricaria chamomilla l.) and cabergoline on idiopathic hyperprolactinemia: A pilot randomized controlled trial. Iran J Pharm Res (2019) 18(3):1612–21. doi: 10.22037/ijpr.2019.1100758
82. Kokol P, Kokol M, Zagoranski S. Machine learning on small size samples: a synthetic knowledge synthesis. Sci Prog (2022) 105(1):368504211029777. doi: 10.1177/00368504211029777
83. Calafato MS, Austin-Zimmerman I, Thygesen JH, Sairam M, Metastasio A, Marston L, et al. The effect of CYP2D6 variation on antipsychotic-induced hyperprolactinaemia: A systematic review and meta-analysis. Pharmacogenom J (2020) 20(5):629–37. doi: 10.1038/s41397-019-0142-9
Keywords: machine learning, prolactin, olanzapine, electronic health record, hyperprolactinemia, pharmacovigilance, XGBoost, SHAP
Citation: Zhu X, Hu J, Xiao T, Huang S, Shang D and Wen Y (2022) Integrating machine learning with electronic health record data to facilitate detection of prolactin level and pharmacovigilance signals in olanzapine-treated patients. Front. Endocrinol. 13:1011492. doi: 10.3389/fendo.2022.1011492
Received: 04 August 2022; Accepted: 27 September 2022;
Published: 13 October 2022.
Edited by:
Jesper Krogh, Rigshospitalet, DenmarkReviewed by:
Aziz Eftekhari, Joint Ukraine-Azerbaijan Research Center of Nanobiotechnology, AzerbaijanCopyright © 2022 Zhu, Hu, Xiao, Huang, Shang and Wen. This is an open-access article distributed under the terms of the Creative Commons Attribution License (CC BY). The use, distribution or reproduction in other forums is permitted, provided the original author(s) and the copyright owner(s) are credited and that the original publication in this journal is cited, in accordance with accepted academic practice. No use, distribution or reproduction is permitted which does not comply with these terms.
*Correspondence: Dewei Shang, c2hhbmdfZGV3ZWlAMTYzLmNvbQ==; Yuguan Wen, d2VueXVndWFuZGVkZUAxNjMuY29t
†These authors have contributed equally to this work
Disclaimer: All claims expressed in this article are solely those of the authors and do not necessarily represent those of their affiliated organizations, or those of the publisher, the editors and the reviewers. Any product that may be evaluated in this article or claim that may be made by its manufacturer is not guaranteed or endorsed by the publisher.
Research integrity at Frontiers
Learn more about the work of our research integrity team to safeguard the quality of each article we publish.