- 1Department of Orthopaedics, Peking University Third Hospital, Beijing, China
- 2Peking University Third Hospital, Beijing Key Laboratory of Spinal Disease Research, Beijing, China
- 3Engineering Research Center of Bone and Joint Precision Medicine, Ministry of Education, Beijing, China
Background: This study aimed to explore the association between serum amino acids (AAs) levels and bone mineral density (BMD).
Methods: We performed a two-sample Mendelian randomization (MR) analysis to analyze the associations between the levels of eight AAs and BMD values by using summary-level genome-wide association study (GWAS) data. We applied the MR Steiger filtering method and MR Pleiotropy RESidual Sum and Outlier (MR-PRESSO) global test to check for and remove single nucleotide polymorphisms (SNPs) that were horizontally pleiotropic. The associations were estimated with the inverse variance weighted (IVW), MR-Egger, weighted median and MR Robust Adjusted Profile Score (MR.RAPS) methods.
Results: Our study found that genetically increased isoleucine (Ile) [IVW: effect = 0.1601, 95% confidence interval (CI) = 0.0604 ~ 0.2597, p = 0.0016] and valine (Val) levels (IVW: effect = 0.0953, 95% CI = 0.0251 ~ 0.1655, p = 0.0078) were positively associated with total body BMD (TB-BMD). The results also revealed that genetically increased tyrosine (Tyr) levels were negatively associated with TB-BMD (IVW: effect = -0.1091, 95% CI = -0.1863 ~ -0.0320, p = 0.0055).
Conclusions: In this study, associations between serum AA levels and BMD were established. These findings underscore the important role that serum AAs play in the development of osteoporosis and provide evidence that osteoporosis can be prevented and treated by the intake of certain AAs.
Introduction
Osteoporosis is the most common bone disease, and it is characterized by low bone mass, bone tissue deterioration and bone structure disruption (1). Osteoporosis is also the reason for fragility fractures, and the most common fracture sites are the spine, hip and distal forearm. The one-year estimated mortality of hip fractures in mainland China is 13.96% (2). Therefore, osteoporosis is a major threat to an enormous number of people and exacts a terrible toll on elderly adults, who constitute a rapidly growing population in the world. The measurement of bone mineral density (BMD) has been proven to be an effective method for diagnosing osteoporosis and assessing the risk of fragility fracture (3). Although osteoporosis is an important and common public health problem, the mechanisms and risk factors underlying osteoporosis and BMD are still poorly understood.
Optimal intake of certain nutrients, such as calcium and selenium, has a substantial impact on BMD and is positively correlated with BMD (4). Dietary proteins are important nutrients for maintaining musculoskeletal health. Both bone and muscle are lost with age, with up to 1% lost per year after age 50, and increased dietary protein intake with age is recommended to ameliorate this loss (5). A systematic review and meta-analysis performed in 2009 of published papers from January 1966 to July 2008 showed that protein supplementation had a significant positive influence on lumbar spine BMD in human adults; moreover, nearly all published cross-sectional studies demonstrated a positive association between dietary protein intake and bone health (6). As the main components of proteins, amino acids (AAs) also play an important role in regulating bone metabolism. However, a clear consensus has not been reached on the role of AAs in bone health because AAs may have competing effects on bone. A recent study in monozygotic twins demonstrated the genetically independent benefit of several specific AAs for bone health (7). One large-scale cohort study by Su et al. (8) suggested that a specific AA profile is correlated with greater BMD and lower subsequent fracture risk, independent of diet and lifestyle factors. Male patients with idiopathic osteoporosis also presented changes in free AA profiles, which indicated the role of AA utilization in osteoporosis (9). However, observational studies that estimated causal inference have numerous inherent limitations, such as reverse causality and confounding effects, thus making the interpretation of these associations difficult and their meaning uncertain (10).
Mendelian randomization (MR) analyses can overcome the limitations of conventional studies by using single nucleotide polymorphisms (SNPs) as instrumental variables (IVs) for assessing the causal effect of a risk factor (exposure) on an outcome (11). MR relies on three assumptions: (a) the genetic variant is associated with the exposure; (b) the genetic variant is not associated with confounders; and (c) the genetic variant influences the outcome only through the exposure. A two-sample MR obtains IV-exposure and IV-outcome associations from two different sets of participants. The IVs used in MR are derived from genome-wide association studies (GWAS) and available due to the development of high-throughput genomic technologies. Therefore, in this study, we used the MR approach to explore the causal effect of circulating AA levels on total body and site-specific BMD. This approach can provide estimates of the effects of traits while reducing bias due to confounding and reverse causation. The design strategy for the two-sample MR in our study is shown in Figure S1 in the Supplementary Material.
Methods
We performed a two-sample MR analyses to study the effect of AA levels on BMD values. Our approach relied upon summary-level GWAS data to obtain MR estimates (12, 13). We selected SNPs with a genome-wide association (p<5E-08), with independent inheritance (R2<0.001), and without linkage disequilibrium (LD) with each AA as IVs. Proxy SNPs (R2>0.9) from LDlink (https://ldlink.nci.nih.gov/) were used when the SNPs were not available for the outcome (14). To estimate the LD level, we selected the reference sample formed by European ancestral individuals from the 1000 Genomes Project (15). Palindromic SNPs with intermediate allele frequencies (palindromic SNPs refer to SNPs with the A/T or G/C alleles and “intermediate allele frequencies” refer to 0.01<allele frequency<0.30) were excluded from the selected instrument SNPs. We also calculated the F statistics for the SNPs to measure the strength of the instruments. IVs with an F statistic less than 10 were excluded and frequently labeled “weak instruments” (16). SNPs with a minor allele frequency (MAF) of < 0.01 were also excluded to avoid potential the statistical bias from the original GWAS since they usually carry with low confidence. Moreover, we used the PhenoScanner tool (17, 18) to check whether any of the selected SNPs were associated with potential confounders at risk of affecting BMD. We set the threshold at genome-wide significance (p<5E-08) when using the PhenoScanner tool.
The summary data for the associations between SNPs and AAs were retrieved from the Nightingale Health UK Biobank Initiative. The Finnish innovator of an internationally recognized blood biomarker technology for studying chronic diseases will analyze the biomarker profiles of 500,000 blood samples from the UK Biobank. Nightingale’s biomarker profiling technology will be used to analyze the UK Biobank blood samples by measuring metabolic biomarkers found by recent studies. The UK Biobank recruited 502,639 European participants aged 37~70 years in 22 assessment centers across the UK. All study participants reached the assessment centers by their own means, and enrollment was not performed at nursing homes. All participants provided written informed consent, and ethical approval was obtained from the North West Multicenter Research Ethics Committee. Blood samples were drawn at baseline between 2007 and 2010 (19). A random subset of nonfasting baseline plasma samples from 118,466 individuals and 1,298 replication samples were measured using high-throughput nuclear magnetic resonance spectroscopy (Nightingale Health Plc; biomarker quantification version 2020), which provided simultaneous quantification of 249 metabolic biomarkers, including AAs, routine lipids, lipoprotein subclass, fatty acid composition, and other low-molecular weight metabolites, such as ketone bodies and glycolysis metabolites quantified in molar concentration units, in a single assay (20). The metabolic biomarker dataset from the Nightingale Health UK Biobank Initiative was made available for the research community through the IEU GWAS database, which is a database of genetic associations in the GWAS summary datasets (https://gwas.mrcieu.ac.uk/) (13). We only focused on the particular set of AAs and extracted summary statistics about eight single AAs, namely, alanine (Ala), glutamine (Gln), histidine (His), isoleucine (Ile), leucine (Leu), phenylalanine (Phe), tyrosine (Tyr) and valine (Val), because we wanted to investigate whether AA metabolism could be associated with BMD values.
We extracted summary statistics on femoral neck (FN), lumbar spine (LS) and forearm (FA) BMD (g/cm^2) from the GEnetic Factors for OSteoporosis Consortium website (21). Genetic variants with large effects on BMD were identified in 53,236 individuals of European ancestry. Genetic variants with a minor allele frequency (MAF)>0.5% were tested for their effects on femoral neck, lumbar spine (L1-4), and forearm BMD, and the values were adjusted for sex, age, age2, weight and standardized to have a mean of zero and a standard deviation of one because different dual-energy X-ray absorptiometry (DXA) machines have known systematic differences in BMD measurements. The summary-level data for total body BMD (TB-BMD) (g/cm^2) were extracted from one GWAS meta-analyses including 30 epidemiological studies comprising individuals from populations across America, Europe, and Australia, with a variety of designs and participant characteristics (22). Most participants in the study were from population-based cohorts of European ancestry, two cohorts comprised African American individuals, and four cohorts included individuals with a mixed background. TB-BMD was also measured by DXA following standard manufacturer protocols. TB-BMD values were corrected for age, weight, height, and genomic principal components. The detailed characteristics of the GWAS associated with exposures and outcomes are shown in Table S1 in the Supplementary Material.
We applied the MR Pleiotropy RESidual Sum and Outlier (MR-PRESSO) global test (23) to remove SNPs that were horizontal pleiotropic outliers to reduce heterogeneity in the estimate of the causal effect. We conducted this analysis by using the MR-PRESSO R package (https://github.com/rondolab/MR-PRESSO). The number of distributions was set to 1,000 and the threshold was set to 0.05. Moreover, we applied MR Steiger filtering (24) as implemented in the TwoSampleMR R package to test the causal direction of each of the extracted SNPs on the exposures and outcomes. This approach calculated the variance explained in the exposure and the outcome by the instrument SNPs and tests whether the variance in the outcome is less than the exposure. A “TRUE” MR Steiger result suggested causality in the expected direction, while a “FALSE” result suggested causality in the reverse direction. We excluded SNPs with “FALSE” results, which meant that it showed evidence of primarily affecting outcomes rather than exposures.
We conducted the MR with inverse variance weighted (IVW) (13, 25), MR-Egger (26, 27) and Weighted median estimate methods (28). The IVW method uses a meta-analysis approach to combine the Wald ratios of the causal effects of each SNP and can provide the most precise estimates (13, 25). The Weighted median estimate provides a reliable effect estimate of the causal effect when at least 50% of the weight in the analysis comes from effective IVs (28). MR-Egger regression is used to create a weighted linear regression of the outcome coefficients with the exposure coefficients. The slope of the weighted regression line provides an asymptotically unbiased causal estimate of the exposure on the outcome if the INSIDE (instrument strength is independent of direct effect) assumption is met. In addition, the intercept of the MR-Egger regression line was used to quantify the amount of horizontal pleiotropy present in the data averaged across the genetic instruments (26, 27). Under the INSIDE assumption, the MR-Egger intercept test identifies horizontal pleiotropy if the intercept from the MR-Egger analysis is not equal to zero (27). We also calculated the MR Robust Adjusted Profile Score (MR.RAPS) to estimate the causal effects because it can lead to considerably higher statistical power than conventional MR analysis (29). MR.RAPS considers the measurement error in SNP-exposure effects, and it is unbiased when weak instruments are used and robust to systematic and idiosyncratic pleiotropy (29). The MR.RAPS method can also alleviate but cannot solve the problem of horizontal pleiotropy (29). We used the IVW (30) method to detect heterogeneity, which was quantified by the Cochran Q statistic. Moreover, we also performed multivariable MR (MVMR) analysis to control for genetic associations of AAs with some BMD potential risk factors, such as alcohol consumption, BMI and education attainment to adjust for the effect of confounders. The summary-level data of alcohol consumption were extracted from the GWAS study in the UK Biobank (UKB) sample of white British individuals (31), BMI were extracted from the meta-analysis of GWAS in European adults (32) and education attainment were extracted from the GWAS conducted in a discovery sample of 101,069 individuals and a replication sample of 25,490 (33). We estimated the power of our study according to a method suggested by Brion et al. (34). This method uses a noncentrality parameter to calculate the statistical power of the continuous outcome and an approximate linear model on the observed binary scale adapted for binary outcome. The method required several parameters to estimate the power. For the continuous outcomes, the first parameter was the proportion of phenotypic variation (r2) explained by IV SNPs, which was estimated on the original GWAS. The second was the effect size of the exposure to the outcome at the epidemiological level, which was estimated from another independent observational cohort (8). Addition parameters included the sample size and standard deviation (SD) of exposure and outcome. The summary-level MR analysis was performed by the TwoSampleMR package (version 0.5.0) in R (version 3.6.1, the R foundation). The statistical tests of the MR analysis were two-sided, and the results of the MR analyses regarding the causal effects of AAs on BMD were considered statistically significant at a Bonferroni-corrected p<0.0125 (e.g., 0.050/4 outcomes). Relationships for which the p value was below 0.05 but above 0.0125 were considered nominally significant.
Results
According to the SNP selection criteria, we first extracted 36, 46, 17, 10, 17, 9, 38 and 22 significant genome-wide and independently inherited SNPs associated with eight AAs. When extracting the corresponding SNPs for outcomes, we had to exclude some SNPs that were absent and no proxy SNPs in high LD (R2>0.9) found from LDlink in the summary statistics of outcomes. We also removed the palindromic SNPs when harmonizing the effect of IVs and excluded the SNPs with false causal direction identified by the MR Steiger filtering. Moreover, when using the PhenoScanner tool, we excluded some SNPs that were associated with confounders, which were proved to be causally associated with BMD such as body mass index (BMI), weight, calcium and low density lipoprotein (LDL) (4, 35), which might violate the second assumption of MR. We also excluded the horizontal pleiotropic outliers through the MR-PRESSO global test. The selection process and the reasons for selecting the SNPs are described in detail in Figures S2–9. The final numbers of SNPs included in the MR are presented in Tables 1–4. For all the included IVs, the F statistics were above 10 (e.g., Ala: ranging from 30.0542 to 249.5155 for FN-BMD; from 30.0542 to 249.5155 for LS-BMD; from 30.8591 to 249.5155 for FA-BMD and from 30.0542 to 249.5155 for TB-BMD), which indicated that they satisfy the strong relevance assumption of MR and that weak instrument bias would not substantially influence the estimations of causal effects. We also confirmed the true causal direction for the included SNPs with the MR Steiger method. The proportion of phenotypic variation explained by each genetic variant was also calculated. The detailed characteristics of the included SNPs are shown in Tables S2–9.
Figure 1 and Tables 1–4 display the causal effects of AAs on BMD based on IVW, MR-Egger, Weighted median, MR.RAPS and MR-PRESSO methods. At the Bonferroni-corrected p threshold of 0.0125, the results provided evidence that genetically increased Ile (e.g., IVW: effect = 0.1601, 95% confidence interval [CI] = 0.0604 ~ 0.2597, p = 0.0016) and Val levels (e.g., IVW: effect = 0.0953, 95% CI = 0.0251 ~ 0.1655, p = 0.0078) were positively associated with TB-BMD (Table 4). The results revealed that genetically increased Tyr levels were negatively associated with TB-BMD (e.g., IVW: effect = -0.1091, 95% CI = -0.1863 ~ -0.0320, p = 0.0055) (Table 4). We did not observe the statistically significant associations between AAs and site-specific BMD (FN, LS and FA-BMD) (Tables 1–3), although Phe levels were negatively associated with FA-BMD at a nominal threshold (p<0.050) (e.g., IVW: effect = -0.2717, 95% CI=-0.4816 ~ -0.0619, p = 0.0111) (Table 3). For the other AAs, such as Ala and His, significant causal effects were not observed on FN, LS, FA and TB-BMD based on IVW, MR-Egger, Weighted median, MR.RAPS and MR-PRESSO methods (Tables 1–4).
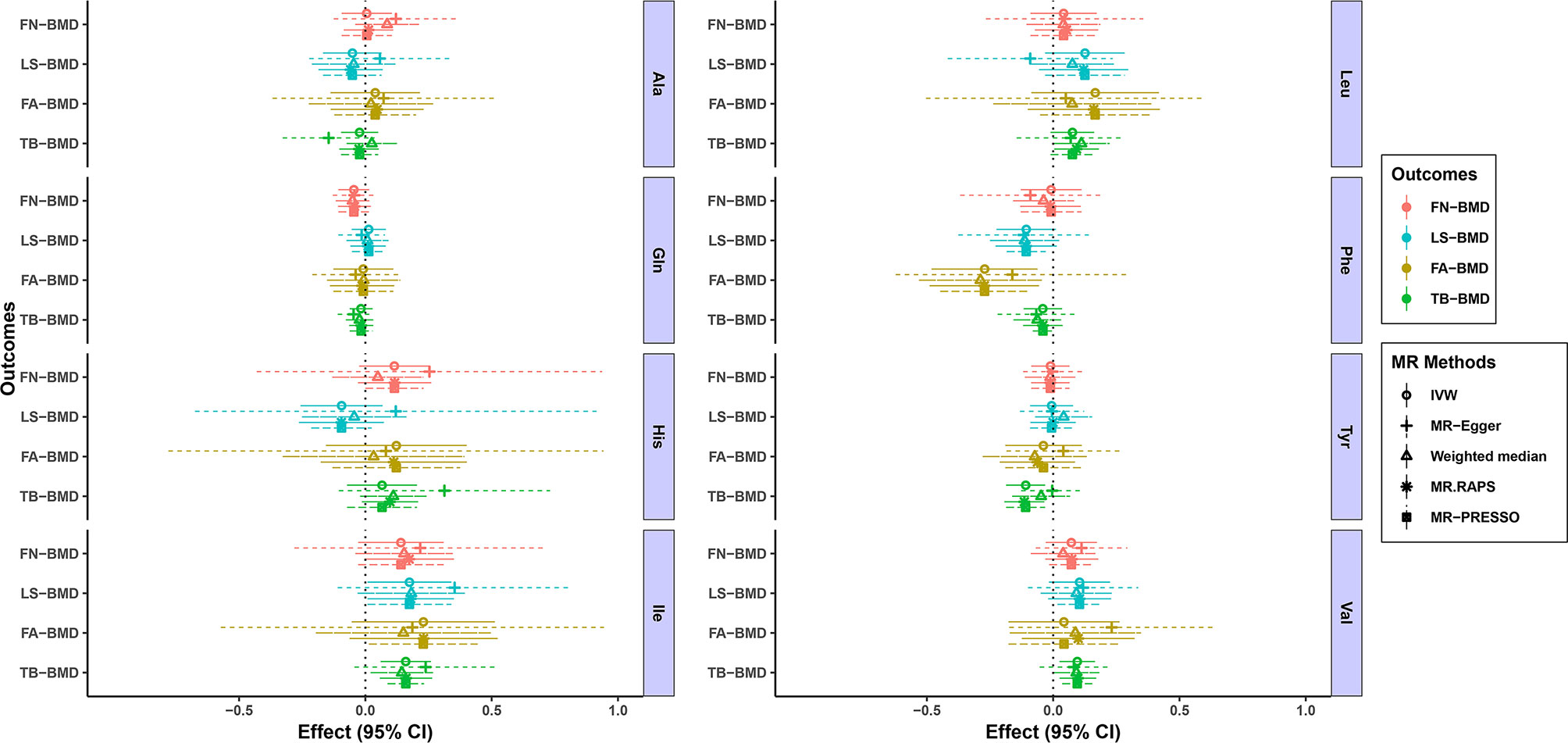
Figure 1 MR estimates of the associations between eight AA levels and BMD. The x-axis is the effects of AAs on BMD values. The vertical dashed line is the reference at effect = 0. The y-axis presents different BMD types, which are highlighted in different colors. Different MR methods are displayed with different line types. AAs, amino acids; BMD, bone mineral density; CI, confidence interval; IVW, inverse variance weighted; MR.RAPS, MR Robust Adjusted Profile Score; MR-PRESSO, MR Pleiotropy RESidual Sum and Outlier; Ala, alanine; Gln, glutamine; His, histidine; Ile, isoleucine; Leu, leucine; Phe, phenylalanine; Tyr, tyrosine; Val, valine; FN-BMD, femoral neck BMD; LS-BMD, lumbar spine BMD; FA-BMD, forearm BMD; TB-BMD, total body BMD.
We conducted heterogeneity analyses using the IVW method and conducted the pleiotropy analyses using the MR-Egger intercept test (Tables 1–4). Heterogeneity was not observed in the MR analyses for the causal associations of AAs on BMD changes (e.g, for the causal association between Ile and the TB-BMD, Q = 3.6813, p = 0.8157) (Table 4). Based on the MR-Egger intercept test, we did not find evidence of directional pleiotropy between the AA levels and BMD (e.g., Ile: intercept = -0.0041, p = 0.7601 for FN-BMD; intercept = -0.0095, p = 0.4520 for LS-BMD; intercept = 0.0023, p = 0.9089 for FA-BMD; and intercept = -0.0041, p = 0.5808 for TB-BMD) (Tables 1–4). The MR-PRESSO global test also revealed that no horizontal pleiotropic outliers were identified in the MR analyses (e.g., Ile: p = 0.1655 for FN-BMD; p = 0.3640 for LS-BMD; p = 0.7540 for FA-BMD; and p = 0.8277 for TB-BMD) (Tables 1–4). The results of MVMR adjusted for alcohol consumption, BMI and educational attainment were similar to the univariable MR results, with significant direct associations identified between Ile, Tyr, Val and TB-BMD (e.g., Ile: p = 0.0001 adjusted for alcohol consumption; p = 0.0097 adjusted for BMI; and p = 0.0058 adjusted for educational attainment) (Tables S10–12). The sample sizes of BMD traits in the current analysis are presented in Table S13. We calculated the proportions of AA variation explained by IVs ranging from 0.0056 to 0.0394. Under the current sample size and exposure variations, we provided the minimum and maximum detectable causal effects required to achieve 80% statistical power for the MR analysis, and they were located in the CI of our results. Therefore, our study had 80% power to detect a causal effect of 0.1535 g/cm^2 increase in TB-BMD per 1-SD increase of Ile levels, 0.0967 g/cm^2 increase in TB-BMD per 1-SD increase of Val levels and 0.1003 g/cm^2 decrease in TB-BMD per 1-SD increase of Tyr levels.
Discussion
Molecular mechanism analyses have suggested that a number of AAs may be associated with BMD. Bone marrow stromal cells were demonstrated to express both intracellular and extracellular nutrient-sensing pathways for AAs, and certain AAs were described as potent stimulators of an increase in intracellular calcium, suggesting that AAs were important signaling molecules for normal cell function (36). Osteoblasts can express specialized AA receptors and transporters that enable the adjustment of cellular bioenergetics according to fluctuations in AA availability (37). Some AA was a potent stimulus of growth hormone secretion, which in turn results in an increase in circulating levels of insulin-like growth factor-1 (IGF-1), a known anabolic stimulus for osteoblasts. Bone mass can be elevated by AA-induced increases in calcium absorption efficiency, osteoblast proliferation and bone mineralization, synthesis of type I collagen, circulating levels of IGF-1, reduced bone resorption, osteoclast attachment and suppressed osteoclast differentiation (38). AAs can also enhance intestinal calcium absorption in vivo, increase the secretion of Alk Phos and decrease the production of interleukin-6 from osteoblasts in vitro. Increases in Alk Phos and decreases in interleukin-6 levels may result in increases in bone collagen synthesis and bone formation and reduced bone resorption (38).
In the present study, we reported for the first time the causal associations between AAs and BMD through a MR analysis. We provided evidence to support the causal effects of Ile,Val and Tyr on TB-BMD. In the MR study, we used strong IVs from the summary statistics of the largest GWAS conducted for AAs and BMD. We employed a range of methods known to control for pleiotropy and checked the heterogeneity, and we obtained highly consistent results. Pleiotropic effects were detected by using the MR-Egger intercept and MR-PRESSO method. Using the MR design, we could mitigate the confounding factors due to the application of Mendel’s second law of the random assortment of alleles. Reverse causality was also prevented because genetic variants were fixed at conception and could not be affected by disease processes. The results above showed that the presence of pleiotropic SNPs was minimal. Besides the univariable MR analysis, we also conducted the MVMR analysis taking into account the effect of some potential risk factors of BMD. We found the results were stable after adjusting for these risk factors. In addition, we also calculated the power of MR. Taken together, our MR results have high precision and stability to support the evidence.
Val, Leu, and Ile are branched-chain AAs (BCAAs) that are critical for the maintenance of bone strength and density and associated with greater muscle and fat mass (39). BCAAs have a direct effect on the initiation of mRNA translation and are the most potent stimulator of muscle protein synthesis, which is critical for the maintenance of adequate bone strength and density (40). In our study, we found that Ile and Val were positively associated with TB-BMD. Tyr, Phe and His are aromatic amino acids (AAAs) involved in protein synthesis. AAAs and their metabolites are involved in the synthesis of various secondary metabolites, including pigment compounds, plant hormones and biological polymers (41). The molecular mechanisms underlying the associations between AAAs and bone metabolism have been partially revealed. AAAs reduced the expression of the calcitonin receptor, carbonic anhydrase II and cathepsin K in osteoclasts in vitro, which may suppress osteoclast differentiation (42). Increasing the intake of AAAs might stimulate an increase in the circulating levels of IGF-1 and influence calcium homeostasis, which is involved in the stimulation of mature osteoblasts and regulates skeletal growth (43, 44). However, Le et al. (45) suggested that dietary AAA intake was not significantly associated with hip fractures, hip BMD, or any measurements of body composition. Our study support the negative causal effects of Tyr and Phe on BMD, although the findings indicated that Phe was negatively associated with FA-BMD at a nominal threshold. The negative associations between AAs and BMD were surprising, although some AAs were reported to cause bone loss and increase the risk of fracture. Higher homocysteine (Hcy) was associated with significant BMD decline and independently associated with a higher risk of fracture (8). The MR results from Wang et al. (46) also revealed a negative association between Hcy and BMD. However, they also indicated that decreased plasma Hcy was not associated with FN-BMD, LS-BMD and the risk for bone fracture. In vitro studies have revealed that Hcy might also promote collagen accumulation in bone, contribute to decreased bone strength and reduce bone blood flow (47), thus suggesting a pathogenic role of Hcy in bone health. A cross-sectional study involving a total of 773 Taiwanese women revealed that elevated Gln was significantly associated with low BMD (48). Gln might convert to glutamate, which would lead to bone resorption through the expression of glutamate receptors on bone cells, especially osteoclasts. This finding explained the association between elevated Gln and low BMD (48). However, we did not identify causal associations between Gln and BMD. Currently, only one clinical study has been published about the association between Ala and BMD. A cross-sectional study (49) of 103 patients with spinal cord injury found that higher alanine levels were not related to BMD after controlling for confounders, including demographic and injury-related characteristics and calcium intake. Our results did not support the causal associations between Ala and BMD. Although the causal associations were found between AAs and TB-BMD, we still did not support the causal associations between AAs and site-specific BMD (FN, LS and FA-BMD). This result also suggested that the effects of circulating AAs on bone metabolism might be systemic rather than local. The molecular mechanism of function of AAs on the bone metabolism also supported the hypothesis (36–38).
Although the design of MR analyses makes this method less susceptible to confounders than other observational studies, limitations still exist. First, we only evaluated the association between a single AA and BMD and did not consider the interactions between the AAs and the interactions with other nutritional factors, such as calcium, which might lead to potential pleiotropy. This limitation might cause the inconclusive causal associations between serum AA levels and BMD. However, we assessed potential pleiotropy using the MR-Egger method and MR-PRESSO method. We also used the PhenoScanner tool to exclude the SNPs associated with confounders. Hence, although the risk of a residual horizontal pleiotropic effect cannot be ruled out, it likely did not change the conclusions of this study in a clinically meaningful way. Second, we did not perform age and gender stratification for the population, which are two essential factors that can affect BMD (1). However, excluding these processes likely did not have a large effect on our analyses because of the large sample size included for AAs and BMD, which might have reduced the bias. Second, most of the population in the original GWAS were from European ancestry, but the participants in the TB-BMD GWAS were of mixed ancestry. The population stratification may not have been completely ruled out and may have influenced the causal estimates, although most participants were from population-based cohorts of European ancestry in the TB-BMD GWAS (22). Last, we did not thoroughly explore the mechanism underlying the causality between AAs and BMD. Therefore, mechanistic research should focus on specific AAs at cellular and individual levels in the future.
Conclusion
In summary, we provided precise evidence that the levels of certain AAs in the serum, namely, Ile and Val, were positively associated with TB-BMD; and Tyr was negatively associated with TB-BMD. We did not observe the statistically significant associations between AAs and site-specific BMD (FN, LS and FA-BMD). These findings underscore the important role that serum AAs play in the development of osteoporosis and provide evidence that osteoporosis can be treated and prevented by supplementing certain AAs. Future studies are needed to investigate the potential mechanisms by which AAs influence bone metabolism and to examine the potential role of these mechanisms in the treatment of osteoporosis.
Data Availability Statement
The original contributions presented in the study are included in the article/Supplementary Material. Further inquiries can be directed to the corresponding author.
Author Contributions
YT, JH, and ZC conceptualized and designed the study. ZC and BH provided the package codes in R language and analyzed the data in the study. ZC drafted the manuscript. HF gave constructive suggestions when writing the manuscript. All authors contributed to the article and approved the submitted version.
Funding
This work was supported by grants from National Natural Science Foundation of China (Grant No.51873214).
Conflict of Interest
The authors declare that the research was conducted in the absence of any commercial or financial relationships that could be construed as a potential conflict of interest.
Publisher’s Note
All claims expressed in this article are solely those of the authors and do not necessarily represent those of their affiliated organizations, or those of the publisher, the editors and the reviewers. Any product that may be evaluated in this article, or claim that may be made by its manufacturer, is not guaranteed or endorsed by the publisher.
Acknowledgments
We are grateful to the Nightingale Health UK Biobank Initiative for releasing the metabolites GWAS summary statistics (19), GEFOS consortium for releasing the FN, LS, and FA-BMD GWAS summary statistics (21), and Medina-Gomez and colleagues for releasing the TB-BMD GWAS summary statistics (22). The authors also wish to thank Dr Siying Zhuang for the support in the preparation of this manuscript.
Supplementary Material
The Supplementary Material for this article can be found online at: https://www.frontiersin.org/articles/10.3389/fendo.2021.763538/full#supplementary-material
References
1. Lorentzon M, Cummings SR. Osteoporosis: The Evolution of a Diagnosis. J Intern Med (2015) 277(6):650–61. doi: 10.1111/joim.12369
2. Cui Z, Feng H, Meng X, Zhuang S, Liu Z, Ye K, et al. Age-Specific 1-Year Mortality Rates After Hip Fracture Based on the Populations in Mainland China Between the Years 2000 and 2018: A Systematic Analysis. Arch Osteoporos (2019) 14(1):55. doi: 10.1007/s11657-019-0604-3
3. Kanis J, Melton LJR, Christiansen C, Johnston C, Khaltaev N. The Diagnosis of Osteoporosis. J Bone Miner Res (1994) 9(8):1137–41. doi: 10.1002/jbmr.5650090802
4. Qu Z, Yang F, Yan Y, Hong J, Wang W, Li S, et al. Relationship Between Serum Nutritional Factors and Bone Mineral Density: A Mendelian Randomization Study. J Clin Endocrinol Metab (2021) 106(6):e2434–43. doi: 10.1210/clinem/dgab085
5. Gaffney-Stomberg E, Insogna KL, Rodriguez NR, Kerstetter JE. Increasing Dietary Protein Requirements in Elderly People for Optimal Muscle and Bone Health. J Am Geriatr Soc (2009) 57(6):1073–9. doi: 10.1111/j.1532-5415.2009.02285.x
6. Darling AL, Millward DJ, Torgerson DJ, Hewitt CE, Lanham-New SA. Dietary Protein and Bone Health: A Systematic Review and Meta-Analysis. Am J Clin Nutr (2009) 90(6):1674–92. doi: 10.1007/s00198-019-04933-8
7. Jennings A, MacGregor A, Spector T, Cassidy A. Amino Acid Intakes Are Associated With Bone Mineral Density and Prevalence of Low Bone Mass in Women: Evidence From Discordant Monozygotic Twins. J Bone Miner Res (2016) 31(2):326–35. doi: 10.1002/jbmr.2703
8. Su Y, Elshorbagy A, Turner C, Refsum H, Chan R, Kwok T. Circulating Amino Acids Are Associated With Bone Mineral Density Decline and Ten-Year Major Osteoporotic Fracture Risk in Older Community-Dwelling Adults. Bone (2019) 129:115082. doi: 10.1016/j.bone.2019.115082
9. Pernow Y, Thorén M, Sääf M, Fernholm R, Anderstam B, Hauge EM, et al. Associations Between Amino Acids and Bone Mineral Density in Men With Idiopathic Osteoporosis. Bone (2010) 47(5):959–65. doi: 10.1016/j.bone.2010.08.017
10. Greenland S, Morgenstern H. Confounding in Health Research. Annu Rev Public Health (2001) 22:189–212. doi: 10.1146/annurev.publhealth.22.1.189
11. Emdin C, Khera A, Kathiresan S. Mendelian Randomization. JAMA (2017) 318(19):1925–6. doi: 10.1001/jama.2017.17219
12. Lawlor D, Harbord R, Sterne J, Timpson N, Davey Smith G. Mendelian Randomization: Using Genes as Instruments for Making Causal Inferences in Epidemiology. Stat Med (2008) 27(8):1133–63. doi: 10.1002/sim.3034
13. Hemani G, Zheng J, Elsworth B, Wade K, Haberland V, Baird D, et al. The MR-Base Platform Supports Systematic Causal Inference Across the Human Phenome. ELife (2018) 7:e34408. doi: 10.7554/eLife.34408
14. Machiela M, Chanock S. Ldlink: A Web-Based Application for Exploring Population-Specific Haplotype Structure and Linking Correlated Alleles of Possible Functional Variants. Bioinformatics (2015) 31(21):3555–7. doi: 10.1093/bioinformatics/btv402
15. 1000 Genomes Project Consortium, Abecasis G, Altshuler D, Auton A, Brooks L, Durbin R, et al. A Map of Human Genome Variation From Population-Scale Sequencing. Nature (2010) 467(7319):1061–73. doi: 10.1038/nature09534
16. Burgess S, Small D, Thompson S. A Review of Instrumental Variable Estimators for Mendelian Randomization. Stat Methods Med Res (2017) 26(5):2333–55. doi: 10.1177/0962280215597579
17. Kamat M, Blackshaw J, Young R, Surendran P, Burgess S, Danesh J, et al. Phenoscanner V2: An Expanded Tool for Searching Human Genotype-Phenotype Associations. Bioinformatics (2019) 35(22):4851–3. doi: 10.1093/bioinformatics/btz469
18. Staley J, Blackshaw J, Kamat M, Ellis S, Surendran P, Sun B, et al. Phenoscanner: A Database of Human Genotype-Phenotype Associations. Bioinformatics (2016) 32(20):3207–9. doi: 10.1093/bioinformatics/btw373
19. Sudlow C, Gallacher J, Allen N, Beral V, Burton P, Danesh J. UK Biobank: An Open Access Resource for Identifying the Causes of a Wide Range of Complex Diseases of Middle and Old Age. PloS Med (2015) 12(3):e1001779. doi: 10.1371/journal.pmed.1001779
20. Julkunen H, Cichońska A, Slagboom P, Würtz P, Nightingale Health UK Biobank Initiative. Metabolic Biomarker Profiling for Identification of Susceptibility to Severe Pneumonia and COVID-19 in the General Population. Elife (2021) 10:e63033. doi: 10.7554/eLife.63033
21. Zheng H, Forgetta V, Hsu Y, Estrada K, Rosello-Diez A, Leo P. Whole-Genome Sequencing Identifies EN1 as a Determinant of Bone Density and Fracture. Nature (2015) 526(7571):112–7. doi: 10.1038/nature14878
22. Medina-Gomez C, Kemp JP, Trajanoska K, Luan J, Chesi A, Ahluwalia TS, et al. Life-Course Genome-Wide Association Study Meta-Analysis of Total Body BMD and Assessment of Age-Specific Effects. Am J Hum Genet (2018) 102(1):88–102. doi: 10.1016/j.ajhg.2017.12.005
23. Verbanck M, Chen C, Neale B, Do R. Detection of Widespread Horizontal Pleiotropy in Causal Relationships Inferred From Mendelian Randomization Between Complex Traits and Diseases. Nat Genet (2018) 50(5):693–8. doi: 10.1038/s41588-018-0099-7
24. Hemani G, Tilling K, Davey Smith G. Orienting the Causal Relationship Between Imprecisely Measured Traits Using GWAS Summary Data. PloS Genet (2017) 13(11):e1007081. doi: 10.1371/journal.pgen.1007081
25. Burgess S, Butterworth A, Thompson S. Mendelian Randomization Analysis With Multiple Genetic Variants Using Summarized Data. Genet Epidemiol (2013) 37(7):658–65. doi: 10.1002/gepi.21758
26. Bowden J, Del Greco M, Minelli C, Davey Smith G, Sheehan N, Thompson J. Assessing the Suitability of Summary Data for Two-Sample Mendelian Randomization Analyses Using MR-Egger Regression: The Role of the I2 Statistic. Int J Epidemiol (2016) 45(6):1961–74. doi: 10.1093/ije/dyw220
27. Burgess S, Thompson S. Interpreting Findings From Mendelian Randomization Using the MR-Egger Method. Eur J Epidemiol (2017) 32(5):377–89. doi: 10.1007/s10654-017-0255-x
28. Bowden J, Davey Smith G, Haycock P, Burgess S. Consistent Estimation in Mendelian Randomization With Some Invalid Instruments Using a Weighted Median Estimator. Genet Epidemiol (2016) 40(4):304–14. doi: 10.1002/gepi.21965
29. Zhao Q, Chen Y, Wang J, Small D. Powerful Three-Sample Genome-Wide Design and Robust Statistical Inference in Summary-Data Mendelian Randomization. Int J Epidemiol (2019) 48(5):1478–92. doi: 10.1093/ije/dyz142
30. Pierce B, Burgess S. Efficient Design for Mendelian Randomization Studies: Subsample and 2-Sample Instrumental Variable Estimators. Am J Epidemiol (2013) 178(7):1177–84. doi: 10.1093/aje/kwt084
31. Clarke TK, Adams MJ, Davies G, Howard DM, Hall LS, Padmanabhan S, et al. Genome-Wide Association Study of Alcohol Consumption and Genetic Overlap With Other Health-Related Traits in UK Biobank (N=112 117). Mol Psychiatry (2017) 22(10):1376–84. doi: 10.1038/mp.2017.153
32. Locke AE, Kahali B, Berndt SI, Justice AE, Pers TH, Day FR, et al. Genetic Studies of Body Mass Index Yield New Insights for Obesity Biology. Nature (2015) 518(7538):197–206. doi: 10.1038/nature14177
33. Okbay A, Beauchamp JP, Fontana MA, Lee JJ, Pers TH, Rietveld CA, et al. Genome-Wide Association Study Identifies 74 Loci Associated With Educational Attainment. Nature (2016) 533(7604):539–42. doi: 10.1038/nature17671
34. Brion M, Shakhbazov K, Visscher P. Calculating Statistical Power in Mendelian Randomization Studies. Int J Epidemiol (2013) 42(5):1497–501. doi: 10.1093/ije/dyt179
35. Yang X, Cui Z, Zhang H, Wei X, Feng G, Liu L, et al. Causal Link Between Lipid Profile and Bone Mineral Density: A Mendelian Randomization Study. Bone (2019) 127:37–43. doi: 10.1016/j.bone.2019.05.037
36. Ding K, Cain M, Davis M, Bergson C, McGee-Lawrence M, Perkins C, et al. Amino Acids as Signaling Molecules Modulating Bone Turnover. Bone (2018) 115:15–24. doi: 10.1016/j.bone.2018.02.028
37. Dirckx N, Moorer MC, Clemens TL, Riddle RC. The Role of Osteoblasts in Energy Homeostasis. Nat Rev Endocrinol (2019) 15(11):651–65. doi: 10.1038/s41574-019-0246-y
38. Bihuniak JD, Insogna KL. The Effects of Dietary Protein and Amino Acids on Skeletal Metabolism. Mol Cell Endocrinol (2015) 410:78–86. doi: 10.1016/j.mce.2015.03.024
39. Ho-Pham LT, Nguyen UDT, Nguyen TV. Association Between Lean Mass, Fat Mass, and Bone Mineral Density: A Meta-Analysis. J Clin Endocrinol Metab (2014) 99(1):30–8. doi: 10.1210/jc.2014-v99i12-30A
40. Fujita S, Volpi E. Amino Acids and Muscle Loss With Aging. J Nutr (2006) 136(1 Suppl):277S–80S. doi: 10.1093/jn/136.1.277S
41. Parthasarathy A, Cross PJ, Dobson RCJ, Adams LE, Savka MA, Hudson AO. A Three-Ring Circus: Metabolism of the Three Proteogenic Aromatic Amino Acids and Their Role in the Health of Plants and Animals. Front Mol Biosci (2018) 5:29. doi: 10.3389/fmolb.2018.00029
42. Refaey ME, Zhong Q, Ding K, Shi X, Xu J, Bollag WB, et al. Impact of Dietary Aromatic Amino Acids on Osteoclastic Activity. Calcif Tissue Int (2014) 95(2):174–82. doi: 10.1007/s00223-014-9878-z
43. Giustina A, Mazziotti G, Canalis E. Growth Hormone, Insulin-Like Growth Factors, and the Skeleton. Endocr Rev (2008) 29(5):535–59. doi: 10.1210/er.2007-0036
44. Dawson-Hughes B, Harris SS, Rasmussen HM, Dallal GE. Comparative Effects of Oral Aromatic and Branched-Chain Amino Acids on Urine Calcium Excretion in Humans. Osteoporos Int (2007) 18(7):955–61. doi: 10.1007/s00198-006-0320-x
45. Le B, Bůžková P, Robbins JA, Fink HA, Raiford M, Isales CM, et al. The Association of Aromatic Amino Acids With Incident Hip Fracture, Abmd, and Body Composition From the Cardiovascular Health Study. Calcif Tissue Int (2019) 105(2):161–72. doi: 10.1007/s00223-019-00562-9
46. Wang P, Liu L, Lei S. Causal Effects of Homocysteine Levels on the Changes of Bone Mineral Density and Risk for Bone Fracture: A Two-Sample Mendelian Randomization Study. Clin Nutr (2021) 40(4):1588–95. doi: 10.1016/j.clnu.2021.02.045
47. Tyagi N, Kandel M, Munjal C, Qipshidze N, Vacek JC, Pushpakumar SB, et al. Homocysteine Mediated Decrease in Bone Blood Flow and Remodeling: Role of Folic Acid. J Orthop Res (2011) 29(10):1511–6. doi: 10.1002/jor.21415
48. You Y, Lin C, Liang H, Lee S, Tsai K, Chiou J, et al. Association Between the Metabolome and Low Bone Mineral Density in Taiwanese Women Determined by (1)H NMR Spectroscopy. J Bone Miner Res (2014) 29(1):212–22. doi: 10.1002/jbmr.2018
Keywords: amino acid, bone mineral density – BMD, Mendelian randomization, valine, tyrosine, isoleucine
Citation: Cui Z, Feng H, He B, He J and Tian Y (2021) Relationship Between Serum Amino Acid Levels and Bone Mineral Density: A Mendelian Randomization Study. Front. Endocrinol. 12:763538. doi: 10.3389/fendo.2021.763538
Received: 24 August 2021; Accepted: 07 October 2021;
Published: 09 November 2021.
Edited by:
Zhi-Feng Sheng, Central South University, ChinaReviewed by:
Peng-Fei Shan, Zhejiang University, ChinaHou-Feng Zheng, Westlake Institute for Advanced Study (WIAS), China
Yanping Du, Fudan University, China
Copyright © 2021 Cui, Feng, He, He and Tian. This is an open-access article distributed under the terms of the Creative Commons Attribution License (CC BY). The use, distribution or reproduction in other forums is permitted, provided the original author(s) and the copyright owner(s) are credited and that the original publication in this journal is cited, in accordance with accepted academic practice. No use, distribution or reproduction is permitted which does not comply with these terms.
*Correspondence: Yun Tian, tiany@bjmu.edu.cn