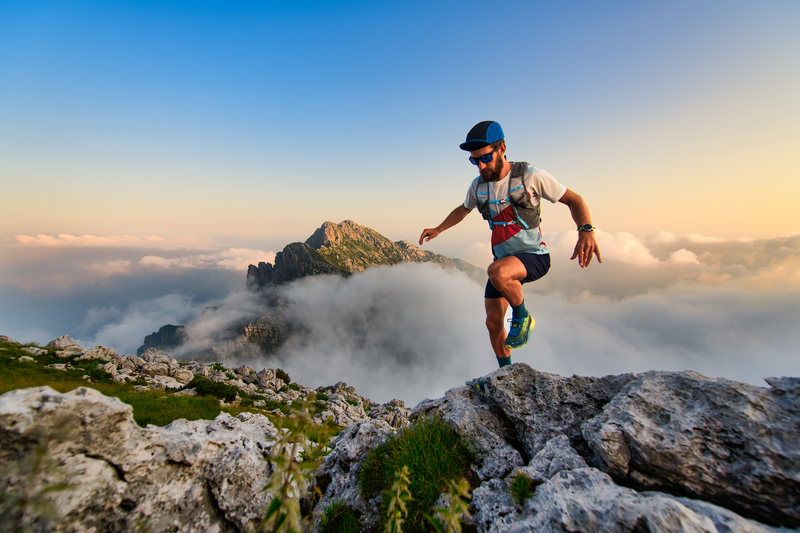
95% of researchers rate our articles as excellent or good
Learn more about the work of our research integrity team to safeguard the quality of each article we publish.
Find out more
ORIGINAL RESEARCH article
Front. Endocrinol. , 22 December 2021
Sec. Renal Endocrinology
Volume 12 - 2021 | https://doi.org/10.3389/fendo.2021.737996
This article is part of the Research Topic Diagnosis, Prevention and Treatment in Diabetic Nephropathy View all 16 articles
Objective: We aimed to analyze the risk factors affecting all-cause mortality in diabetic patients with acute kidney injury (AKI) and to develop and validate a nomogram for predicting the 90-day survival rate of patients.
Methods: Clinical data of diabetic patients with AKI who were diagnosed at The First Affiliated Hospital of Guangxi Medical University from April 30, 2011, to April 30, 2021, were collected. A total of 1,042 patients were randomly divided into a development cohort and a validation cohort at a ratio of 7:3. The primary study endpoint was all-cause death within 90 days of AKI diagnosis. Clinical parameters and demographic characteristics were analyzed using Cox regression to develop a prediction model for survival in diabetic patients with AKI, and a nomogram was then constructed. The concordance index (C-index), receiver operating characteristic curve, and calibration plot were used to evaluate the prediction model.
Results: The development cohort enrolled 730 patients with a median follow-up time of 87 (40–98) days, and 86 patients (11.8%) died during follow-up. The 90-day survival rate was 88.2% (644/730), and the recovery rate for renal function in survivors was 32.9% (212/644). Multivariate analysis showed that advanced age (HR = 1.064, 95% CI = 1.043–1.085), lower pulse pressure (HR = 0.964, 95% CI = 0.951–0.977), stage 3 AKI (HR = 4.803, 95% CI = 1.678–13.750), lower 25-hydroxyvitamin D3 (HR = 0.944, 95% CI = 0.930–0.960), and multiple organ dysfunction syndrome (HR = 2.056, 95% CI = 1.287–3.286) were independent risk factors affecting the all-cause death of diabetic patients with AKI (all p < 0.01). The C-indices of the prediction cohort and the validation cohort were 0.880 (95% CI = 0.839–0.921) and 0.798 (95% CI = 0.720–0.876), respectively. The calibration plot of the model showed excellent consistency between the prediction probability and the actual probability.
Conclusion: We developed a new prediction model that has been internally verified to have good discrimination, calibration, and clinical value for predicting the 90-day survival rate of diabetic patients with AKI.
In recent years, the incidence of diabetes has increased globally. According to the International Diabetes Federation Atlas, 9th edition, 463 million adults worldwide live with diabetes as of 2019, with a prevalence rate of approximately 9.3% and an average annual growth rate of 51% (1). Diabetes easily leads to several complications that affect the prognosis of patients with diabetes (2, 3). Approximately 4.2 million people worldwide died from diabetes or its complications in 2019, accounting for approximately 11.3% of all-cause deaths worldwide (1).
Patients with diabetes often develop acute kidney injury (AKI) due to poor blood glucose control, infection, organ failure, contrast agents, and reduced resistance (4, 5). A large retrospective cohort study has shown that the incidence of AKI is 48.6% in diabetic patients, which is significantly higher than that in non-diabetic patients (17.2%) (6). Diabetes can increase the incidence of AKI and the risk of poor renal outcomes (7). Diabetic patients with AKI without timely treatment will progress to chronic renal failure (CRF) and even end-stage renal disease (ESRD), which should be treated by renal replacement therapy (RRT). One study has shown that the RRT rate of AKI in diabetic patients is approximately 5-fold higher than that in non-diabetic patients (8). AKI is not only a common complication of diabetes but also an independent risk factor associated with the survival rate and CRF of diabetic patients (9–11). Diabetic patients with AKI have poorer clinical outcomes (12). Therefore, early identification and intervention of risk factors affecting clinical outcomes can help to delay the progression and improve the survival rate of diabetic patients with AKI.
However, there have been few studies on the factors affecting the prognosis of diabetic patients with AKI. Due to the high prevalence and poor prognosis of AKI in diabetic patients, it is necessary to develop a prognostic model for diabetic patients with AKI. A nomogram is considered a reliable tool that can be used to create a simple intuitive predictive model that quantifies the risk of a clinical event (13, 14). In the present study, an accurate and beneficial prediction model based on a nomogram for predicting the 90-day survival rate of diabetic patients with AKI was developed, aiming to explore the risk factors for poor short-term prognosis and to provide a reference for the prevention and treatment of diabetic patients with AKI.
All subjects were patients treated at The First Affiliated Hospital of Guangxi Medical University from April 30, 2011 to April 30, 2021, who were diagnosed with diabetes and AKI. The inclusion criteria were as follows: 1) a clear diagnosis of diabetes before AKI and 2) changes in serum creatinine (Scr) consistent with the diagnostic criteria for AKI. The exclusion criteria were as follows: 1) age <18 years; 2) patients diagnosed with stage 5 chronic kidney disease (CKD) or who received regular RRT; 3) incomplete baseline data; and 4) patients lost to follow-up within 90 days of AKI diagnosis. The present study was approved by the Ethics Committee of The First Affiliated Hospital of Guangxi Medical University [approval no. 2019(KY-E-028)]. As this was a retrospective analysis of anonymized clinically obtained data and all patient identifiers were removed, there was no need for patients to sign an informed consent form. The present study was conducted in accordance with the tenets of the Declaration of Helsinki (15).
We followed the Transparent Reporting of a Multivariable Prediction Model for Individual Prognosis or Diagnosis (TRIPOD) statement for reporting multivariable prediction model development and validation (16, 17).The TRIPOD checklist of the present study is found in the Supplementary Material. A retrospective cohort study was performed, and diabetic patients with AKI were followed up for 90 days or death (death within 90 days). The primary study endpoint was all-cause death within 90 days of AKI diagnosis. Patients were randomly divided into a development cohort and a validation cohort at a ratio of 7:3. The development cohort was used to construct the prediction model, and the validation cohort was used to verify the prediction accuracy of the model.
Clinical parameters and demographic data were collected, including age, sex, diabetes duration, complications, smoking, drinking, body mass index (BMI), blood pressure, baseline levels of routine blood tests, liver function, renal function, electrolytes, myocardial enzymes, N-terminal prohormone of brain natriuretic peptide (NT-proBNP), 25-hydroxyvitamin D3 [25(OH)D3], AKI stage, infection, heart failure, cerebrocardiovascular diseases, and multiple organ dysfunction syndrome (MODS). The baseline Scr was defined as a stable Scr within the last 3 months or longer if none was available within 3 months (18). ΔScr was calculated as the Scr difference at the end of follow-up and baseline.
The diagnosis of diabetes in our institution complies with the World Health Organization criteria as follows: diabetic symptoms and 1) random blood glucose ≥11.1 mmol/L, 2) fasting blood glucose (FBG) ≥7.0 mmol/L, or 3) postprandial blood glucose (PBG) ≥11.1 mmol/L (19). AKI was diagnosed in accordance with the diagnostic criteria in the guidelines of KDIGO as follows: increase in Scr ≥26.5 μmol/L within 48 h or an increase from the baseline value by ≥50% within 7 days (20). The criteria of AKI stages were as follows: stage 1, AKI was defined by the AKI Network as at least a ≥50% rise or a ≥0.3 mg/dl rise from baseline Scr; stage 2, AKI was defined as a doubling in Scr from baseline; and stage 3, AKI was defined as a tripling in Scr from baseline or receiving acute dialysis during the hospital stay (21). Heart failure complied with the European Society of Cardiology guidelines as follows: the symptoms and/or signs of heart failure with left ventricular ejection fraction less than 40% (22). MODS was defined as acute and potentially reversible dysfunction of two or more organ systems (23).
Statistical analyses were performed and graphics were produced with SPSS version 22.0 (IBM Corp., Armonk, NY, USA) and R software version 4.0.3 (https://www.r-project.org/). For continuous variables, data are presented as the mean ± standard deviation (normal distribution) or median and interquartile range (abnormal distribution). For dichotomous variables, data are presented as whole numbers and proportions [n(%)]. T-tests, chi-square tests, or Wilcoxon rank-sum tests were used to compare differences in the clinical data between the development cohort and the validation cohort by SPSS. Univariate Cox regression was used to screen the risk factors affecting the prognosis, and the “forward LR” method was then used to screen the variables of p < 0.05 that were included in the multivariate Cox proportional hazards regression model. Based on the results of multivariate Cox analysis, the “rms” package in R statistical software was used to construct the nomogram according to the hazard ratio (HR) and 95% confidence interval (95% CI) of the risk factors. Bootstrapping resampling techniques with 1,000 replications were used to perform internal validation. The concordance index (C-index) and receiver operating characteristic (ROC) curve were used to evaluate the differentiation of the prediction model in the development and validation cohorts. A C-index or area under the ROC curve (AUROC) >0.70 indicated that the prediction effect of the model was good. Calibration plots were drawn to evaluate the accuracy of the prediction model in the development and validation cohorts. The “rms” package was used to draw the calibration plots. Regarding the model, calibration lines closer to the standard line indicate better calibration degree of the model. A p < 0.05 was considered statistically significant in all analyses.
There were 1,254 patients diagnosed with diabetic AKI, of whom 52 were younger than 18 years, 98 received regular RRT, 36 had incomplete baseline data, and 26 were lost to follow-up. Finally, a total of 1,042 patients were enrolled in our study, with 730 and 312 patients assigned to the development and validation cohorts, respectively. In the development cohort, 21.2% (n = 155) had stage 1 AKI, 24.4% (n = 178) had stage 2 AKI, and 54.4% (n = 397) had stage 3 AKI; the median follow-up time was 87 (40–98) days. By the end of follow-up, 86 patients had died (11.8%) within 90 days of AKI diagnosis. The 90-day cumulative survival rate was 88.2% (644/730), and the recovery rate for renal function in survivors was 32.9% (212/644). The main causes of death were cerebrocardiovascular diseases in 37 cases (43.0%), bacterial infection in 28 cases (32.6%), and other or unknown causes in 21 cases (24.4%). In the validation cohort, the median follow-up time was 86.5 (36–99) days. Forty patients died (12.8%) within 90 days of AKI diagnosis. The 90-day cumulative survival rate was 87.2% (272/312), and the recovery rate for renal function in survivors was 33.8% (92/272). The main causes of death were cerebrocardiovascular diseases in 17 cases (42.5%), bacterial infection in 14 cases (35.0%), and other or unknown causes in 9 cases (22.5%).
Table 1 shows the patient characteristics by cohort. Compared to the validation cohort, patients in the development cohort had higher creatine kinase and lower endogenous creatinine clearance (p < 0.05). There was no significant difference in sex, age, diabetes duration, BMI, blood pressure, white blood cell count (WBC), platelets, hemoglobin, 25(OH)D3, creatine kinase-MB (CK-MB), lactate dehydrogenase (LDH), NT-proBNP, FBG, PBG, glycosylated hemoglobin A1c (HbA1c), blood urea nitrogen (BUN), baseline Scr, uric acid (UA), serum kalium levels, incidence of RRT, heart failure, CKD, bacterial infections, or MODS (p > 0.05). Kaplan–Meier survival analysis showed that there was no significant difference in the 90-day survival rates between the development and validation cohorts (log rank χ2 = 0.208, p = 0.648).
Table 1 Differences in the development cohort and the validation cohort in terms of demographic characteristics and laboratory values.
Cox regression analysis was used to construct the prediction model because the Cox proportional hazards assumption was met. As shown in Table 2, univariate Cox analysis of the development cohort revealed that advanced age, pulse pressure, WBC, NT-proBNP, ΔScr, AKI stage, serum kalium levels, 25(OH)D3, heart failure, and MODS were related factors for all-cause death of diabetic patients with AKI (p < 0.05). Sex, diabetes duration, length of hospital stay, smoking, drinking, BMI, blood pressure, platelets, hemoglobin, CK-MB, LDH, FBG, PBG, HbA1c, BUN, baseline Scr, UA, incidence of proteinuria, RRT, bacterial infection, CKD, coronary heart disease, and cerebrovascular disease were not correlated with death (p > 0.05). Hence, these significant indicators [advanced age, pulse pressure, WBC, NT-proBNP, ΔScr, AKI stage, serum kalium levels, 25(OH)D3, heart failure, and MODS] with statistical significance from the univariate analysis were included in the multivariate Cox regression analysis. The results showed that advanced age (every 1 year increase: HR = 1.064, 95% CI = 1.043–1.085, p < 0.001), stage 3 AKI (HR = 4.803, 95% CI = 1.678–13.750, p = 0.003), and MODS (HR = 2.056, 95% CI = 1.287–3.286, p = 0.003) were independent risk factors affecting the all-cause death of diabetic patients with AKI, while higher pulse pressure (every 1 mmHg increase: HR = 0.964, 95% CI = 0.951–0.977, p < 0.001) and higher 25(OH)D3 (every 1 nmol/L increase: HR = 0.944, 95% CI = 0.930–0.960, p < 0.001) were independent protective factors affecting the all-cause death of diabetic patients with AKI.
Table 2 Analysis of risk factors for prognosis in the development cohort (univariate and multivariate Cox regression).
A prediction model that incorporated the above independent predictors was developed as the nomogram (Figure 1). The 90-day survival rate after the diagnosis of AKI was estimated by calculating risk factor scores. Taking a 65-year-old patient with MODS as an example, the scores of each influencing factor were calculated as follows: 57.5 points for a 65-year-old, 37.5 points for a pulse pressure of 85 mmHg, 26.25 points for stage 3 AKI, 65 points for 25(OH)D3 of 55 nmol/L, and 13.75 points for MODS. The total score of this patient was 200 points (57.5 + 37.5 + 26.25 + 65 + 13.75), and the predicted 90-day survival rate was approximately 42%.
Figure 1 Nomogram predicting the 90-day survival rate in diabetic patients with acute kidney injury (AKI).
The C-index of the prediction model in the development cohort was 0.880 (95% CI = 0.839–0.921). As shown in Figure 2A, the AUROC of the prediction model for the 90-day survival rate was 0.860, and the sensitivity and specificity were 0.766 and 0.937, respectively. The C-index of the prediction model in the validation cohort was 0.798 (95% CI = 0.720–0.876) according to the internal verification by Bootstrap. As shown in Figure 2B, the AUROC of the prediction model for the 90-day survival rate was 0.774, and the sensitivity and specificity were 0.705 and 0.821, respectively. The risks of death in the decile groups were calibrated using a smoothing function, with the X-axis as the predicted probabilities and the Y-axis as the actual probabilities. In both the development and validation groups, the calibration plots of the prediction model were close to a straight line with a slope of 1 (Figures 3A, B).
Figure 2 (A) Receiver operating characteristic (ROC) curve of the prediction model in the development cohort. (B) ROC curve of the prediction model in the validation cohort.
Figure 3 (A) Calibration curve of the nomogram in the development cohort. (B) Calibration curve of the nomogram in the validation cohort.
The prognosis of diabetic patients with AKI is worse than that of non-diabetic or non-AKI patients (12, 24, 25). Therefore, it is particularly important to explore the risk factors affecting the clinical outcomes and to construct a prognostic model for diabetic patients with AKI. In the present study, the risk factors for short-term prognosis were evaluated using Cox regression analysis, and a prediction model of prognostic risk was constructed based on the clinical parameters and demographic characteristics of diabetic patients with AKI. The results of our study showed that the 90-day survival rate was 88.2%, and advanced age, lower pulse pressure, stage 3 AKI, lower 25(OH)D3, and MODS were independent risk factors affecting the all-cause death of diabetic patients with AKI. Based on these risk factors, a model was established to predict the short-term survival of diabetic patients with AKI. In addition, calibration plots, the C-index of the validation cohort, the AUROC of the validation cohort, and bootstrapping resampling techniques were used for the internal validation of the predictive model. The accuracy verification showed that the model had a certain predictive ability.
Several studies have shown that ketoacidosis, hyperosmolar and hyperglycemic coma, rhabdomyolysis, contrast agents, sepsis, and heart failure are risk factors for the development of AKI in diabetes mellitus (26, 27). If AKI is not corrected in time, the degree of kidney injury might be aggravated. AKI is associated with poor prognosis in patients, including the occurrence of CKD, progression of CKD, prolonged hospital stays, increased adverse cardiovascular events, and mortality (28–31). Previous studies on predictive models of AKI have mainly focused on specific populations of patients with AKI after cardiac surgery (32, 33), AKI after non-cardiac surgery (34, 35), septic AKI (36, 37), tumor-related AKI (38), and critical AKI (39). James et al. (40) constructed a predictive model of progression to advanced CKD after discharge in patients with AKI, and the results showed that older age, female sex, higher baseline Scr, higher baseline proteinuria, more severe AKI, and higher Scr at discharge were associated with a higher risk of progression to advanced CKD. However, few studies have developed or validated prediction models for all-cause mortality in diabetic patients with AKI. To our knowledge, this is the first study to develop a prognostic model for the 90-day survival rate in diabetes with AKI, which can help identify risk factors for poor prognosis in diabetes with AKI at an early stage and improve the short-term prognosis through timely interventions.
The results of our study showed that 54.4% of patients had stage 3 AKI, indicating that this prediction model might be more suitable for predicting the prognosis of diabetic patients with AKI in more severe AKI stages. For patients with stage 1 or 2 AKI, its predictive effect still needs to be further explored. In addition, our study showed that more severe AKI was an important risk factor for increased all-cause mortality in diabetic AKI patients (HR = 4.803). Previous studies have shown that the overall in-hospital mortality rates are 0.6% in no AKI, 5.3% in stage 1 AKI, 13.4% in stage 2 AKI, and 35.4% in stage 3 AKI (41, 42), which also supported our study. Therefore, the short-term prognosis of diabetes with AKI can be preliminarily evaluated and predicted according to the stage of AKI in clinical practice.
Previous studies have shown that advanced age is an important risk factor for the occurrence and development of patients with diabetes mellitus and AKI (43, 44). Our study also showed that advanced age was an independent risk factor for all-cause mortality in diabetic patients with AKI. A previous animal study has shown that elderly type 2 diabetes mellitus (T2DM) rats have a greater decrease in medullary blood flow and glomerular filtration rate after renal ischemia reperfusion than middle-aged T2DM rats. The expression of renal adhesion molecules and the number of infiltrating immune cells in elderly T2DM rats are higher than those in young or middle-aged rats (45). A large multicenter cohort study of 72,310 elderly patients with T2DM has shown that congestive heart failure, cerebrovascular diseases, and mortality significantly increase with increasing age (46). With age, the physiological function, self-regulation, and reserve ability of the human body decrease. In addition, the decrease in arterial wall elasticity and compliance may also aggravate vascular damage, other complications, and the incidence of clinical events in advanced-age diabetic patients with AKI.
Our study showed that a lower 25(OH)D3 was a risk factor for all-cause mortality in diabetic patients with AKI. Fernandez-Juarez et al. (47) followed up 133 patients with T2DM with proteinuria, and they reported that a low 25(OH)D3 is associated with poor prognosis (Scr increase >50%, ESRD, and mortality). A previous study has also shown a strong association between vitamin D deficiency and the increased risk of heart failure in older patients (OR = 12.19), which was similar to the result of our study (48). An animal study has shown that activation of vitamin D receptors might alleviate cisplatin-induced AKI by inhibiting iron death (49). Vitamin D3 supplementation ameliorates kidney injury induced by hyperglycemia in diabetic mice by regulating lipid metabolism, oxidative stress, apoptosis, and autophagy (50). Therefore, a lower 25(OH)D3 might contribute to all-cause mortality in diabetic patients with AKI by increasing the risk of renal damage and cardiovascular events. In addition, our study also showed that MODS was another independent risk factor for all-cause mortality in diabetic patients with AKI. A retrospective study has shown that MODS is an independent risk factor for poor prognosis in hospital-acquired AKI (OR = 3.538), which was consistent with our study (51). Hemodynamic instability and volume overload are common in patients with MODS, and the mortality of MODS is approximately 40%, which increases with the number of failing organs (52). Thus, MODS might increase the risk of all-cause mortality in diabetic patients with AKI.
The present study had several limitations. Firstly, the sample size was small and from only one medical center. We only verified the model internally in the same center, and the conclusions should be further confirmed by external validation at other centers. Secondly, the diagnosis of AKI in this study was only based on the criterion of Scr, which did not include the diagnostic criterion of urine volume. Thus, some patients may have been missed. Thirdly, the present study may have missed a few potential risk factors, such as microalbuminuria, albumin-to-creatinine ratio, drugs, and other therapeutic measures. Therefore, this prediction model still requires collecting more clinical data and conducting external validation in other centers to further determine its accuracy and applicability.
We developed a newly generated prognostic model that has predictive value for the prognosis of diabetic patients with AKI, which has been internally verified to have good discrimination, calibration, and clinical benefit for predicting the 90-day survival rate of patients. Future studies and external validation should validate this model in different cohorts.
The raw data supporting the conclusions of this article will be made available by the authors, without undue reservation.
MM designed the study, analyzed data, and wrote the manuscript. LP designed the study, collected and analyzed data, and wrote the manuscript. ZH designed the study and revised the manuscript. YuL collected and analyzed data. YunL and NX collected data and revised the manuscript. All authors read and approved the final manuscript.
This work was supported by the National Natural Science Foundation of China (no. 8176030057), the Guangxi Natural Science Foundation (no. 2018GXNSFBA050040), and the Scientific Research and Technological Development Program of Guangxi (no. GuiKeGong 1598011-6).
The authors declare that the research was conducted in the absence of any commercial or financial relationships that could be construed as a potential conflict of interest.
All claims expressed in this article are solely those of the authors and do not necessarily represent those of their affiliated organizations, or those of the publisher, the editors and the reviewers. Any product that may be evaluated in this article, or claim that may be made by its manufacturer, is not guaranteed or endorsed by the publisher.
The Supplementary Material for this article can be found online at: https://www.frontiersin.org/articles/10.3389/fendo.2021.737996/full#supplementary-material
1. IDF. Diabetes Atlas (2019). Available at: https://www.diabetesatlas.org/en/.
2. Kotwas A, Karakiewicz B, Zabielska P, Wieder-Huszla S, Jurczak A. Epidemiological Factors for Type 2 Diabetes Mellitus: Evidence From the Global Burden of Disease. Arch Public Health (2021) 79(1):110. doi: 10.1186/s13690-021-00632-1
3. Wang L, Li X, Wang Z, Bancks MP, Carnethon MR, Greenland P, et al. Trends in Prevalence of Diabetes and Control of Risk Factors in Diabetes Among US Adults, 1999-2018. JAMA Internal Med (2021) 6(1):1–13. doi: 10.1001/jama.2021.9883
4. Advani A. Acute Kidney Injury: A Bona Fide Complication of Diabetes. Diabetes (2020) 69(11):2229–37. doi: 10.2337/db20-0604
5. Yu SM, Bonventre JV. Acute Kidney Injury and Progression of Diabetic Kidney Disease. Adv chronic Kidney Dis (2018) 25(2):166–80. doi: 10.1053/j.ackd.2017.12.005
6. Hapca S, Siddiqui MK, Kwan RSY, Lim M, Matthew S, Doney ASF, et al. The Relationship Between AKI and CKD in Patients With Type 2 Diabetes: An Observational Cohort Study. J Am Soc Nephrol JASN (2021) 32(1):138–50. doi: 10.1681/ASN.2020030323
7. Patschan D, Muller GA. Acute Kidney Injury in Diabetes Mellitus. Int J Nephrol (2016) 2016:6232909. doi: 10.1155/2016/6232909
8. Harding JL, Li Y, Burrows NR, Bullard KM, Pavkov ME. US Trends in Hospitalizations for Dialysis-Requiring Acute Kidney Injury in People With Versus Without Diabetes. Am J Kidney Dis Off J Natl Kidney Found (2020) 75(6):897–907. doi: 10.1053/j.ajkd.2019.09.012
9. Wehbe E, Duncan AE, Dar G, Budev M, Stephany B. Recovery From AKI and Short- and Long-Term Outcomes After Lung Transplantation. Clin J Am Soc Nephrol CJASN (2013) 8(1):19–25. doi: 10.2215/CJN.04800512
10. Kellum JA, Sileanu FE, Murugan R, Lucko N, Shaw AD, Clermont G. Classifying AKI by Urine Output Versus Serum Creatinine Level. J Am Soc Nephrol JASN (2015) 26(9):2231–8. doi: 10.1681/ASN.2014070724
11. Thakar CV, Christianson A, Himmelfarb J, Leonard AC. Acute Kidney Injury Episodes and Chronic Kidney Disease Risk in Diabetes Mellitus. Clin J Am Soc Nephrol CJASN (2011) 6(11):2567–72. doi: 10.2215/CJN.01120211
12. See EJ, Jayasinghe K, Glassford N, Bailey M, Johnson DW, Polkinghorne KR, et al. Long-Term Risk of Adverse Outcomes After Acute Kidney Injury: A Systematic Review and Meta-Analysis of Cohort Studies Using Consensus Definitions of Exposure. Kidney Int (2019) 95(1):160–72. doi: 10.1016/j.kint.2018.08.036
13. Jiang S, Fang J, Yu T, Liu L, Zou G, Gao H, et al. Novel Model Predicts Diabetic Nephropathy in Type 2 Diabetes. Am J Nephrol (2020) 51(2):130–8. doi: 10.1159/000505145
14. Guan C, Li C, Xu L, Zhen L, Zhang Y, Zhao L, et al. Risk Factors of Cardiac Surgery-Associated Acute Kidney Injury: Development and Validation of a Perioperative Predictive Nomogram. J Nephrol (2019) 32(6):937–45. doi: 10.1007/s40620-019-00624-z
15. World Medical Association declaration of Helsinki. Recommendations Guiding Physicians in Biomedical Research Involving Human Subjects. Jama (1997) 277(11):925–6. doi: 10.1001/jama.277.11.925
16. Collins GS, Reitsma JB, Altman DG, Moons KG. Transparent Reporting of a Multivariable Prediction Model for Individual Prognosis or Diagnosis (TRIPOD): The TRIPOD Statement. Ann Intern Med (2015) 162(1):55–63. doi: 10.7326/m14-0697
17. Collins GS, Reitsma JB, Altman DG, Moons KG. Transparent Reporting of a Multivariable Prediction Model for Individual Prognosis or Diagnosis (TRIPOD): The TRIPOD Statement. Bmj (2015) 350:g7594. doi: 10.1136/bmj.g7594
18. Chao CT, Tsai HB, Wu CY, Lin YF, Hsu NC, Chen JS, et al. The Severity of Initial Acute Kidney Injury at Admission of Geriatric Patients Significantly Correlates With Subsequent in-Hospital Complications. Sci Rep (2015) 5:13925. doi: 10.1038/srep13925
19. Alberti KG, Zimmet PZ. Definition, Diagnosis and Classification of Diabetes Mellitus and its Complications. Part 1: Diagnosis and Classification of Diabetes Mellitus Provisional Report of a WHO Consultation. Diabetic Med J Br Diabetic Assoc (1998) 15(7):539–53. doi: 10.1002/(SICI)1096-9136(199807)15:7<539::AID-DIA668>3.0.CO;2-S
20. Palevsky PM, Liu KD, Brophy PD, Chawla LS, Parikh CR, Thakar CV, et al. KDOQI US Commentary on the 2012 KDIGO Clinical Practice Guideline for Acute Kidney Injury. Am J Kidney Dis Off J Natl Kidney Found (2013) 61(5):649–72. doi: 10.1053/j.ajkd.2013.02.349
21. Mehta RL, Kellum JA, Shah SV, Molitoris BA, Ronco C, Warnock DG, et al. Acute Kidney Injury Network: Report of an Initiative to Improve Outcomes in Acute Kidney Injury. Crit Care (2007) 11(2):R31. doi: 10.1186/cc5713
22. Seferovic PM, Ponikowski P, Anker SD, Bauersachs J, Chioncel O, Cleland JGF, et al. Clinical Practice Update on Heart Failure 2019: Pharmacotherapy, Procedures, Devices and Patient Management. An Expert Consensus Meeting Report of the Heart Failure Association of the European Society of Cardiology. Eur J Heart fail (2019) 21(10):1169–86. doi: 10.1002/ejhf.1531
23. Gourd NM, Nikitas N. Multiple Organ Dysfunction Syndrome. J Intensive Care Med (2020) 35: (12):1564–75. doi: 10.1177/0885066619871452
24. Heung M, Steffick DE, Zivin K, Gillespie BW, Banerjee T, Hsu CY, et al. Acute Kidney Injury Recovery Pattern and Subsequent Risk of CKD: An Analysis of Veterans Health Administration Data. Am J Kidney Dis Off J Natl Kidney Found (2016) 67(5):742–52. doi: 10.1053/j.ajkd.2015.10.019
25. Monseu M, Gand E, Saulnier PJ, Ragot S, Piguel X, Zaoui P, et al. Acute Kidney Injury Predicts Major Adverse Outcomes in Diabetes: Synergic Impact With Low Glomerular Filtration Rate and Albuminuria. Diabetes Care (2015) 38(12):2333–40. doi: 10.2337/dc15-1222
26. Skinner DL, Hardcastle TC, Rodseth RN, Muckart DJ. The Incidence and Outcomes of Acute Kidney Injury Amongst Patients Admitted to a Level I Trauma Unit. Injury (2014) 45(1):259–64. doi: 10.1016/j.injury.2013.07.013
27. Fahling M, Seeliger E, Patzak A, Persson PB. Understanding and Preventing Contrast-Induced Acute Kidney Injury. Nat Rev Nephrol (2017) 13(3):169–80. doi: 10.1038/nrneph.2016.196
28. Bell S, Dekker FW, Vadiveloo T, Marwick C, Deshmukh H, Donnan PT, et al. Risk of Postoperative Acute Kidney Injury in Patients Undergoing Orthopaedic Surgery–Development and Validation of a Risk Score and Effect of Acute Kidney Injury on Survival: Observational Cohort Study. Bmj (2015) 351:h5639. doi: 10.1136/bmj.h5639
29. Go AS, Hsu CY, Yang J, Tan TC, Zheng S, Ordonez JD, et al. Acute Kidney Injury and Risk of Heart Failure and Atherosclerotic Events. Clin J Am Soc Nephrol CJASN (2018) 13(6):833–41. doi: 10.2215/CJN.12591117
30. Villeneuve PM, Clark EG, Sikora L, Sood MM, Bagshaw SM. Health-Related Quality-of-Life Among Survivors of Acute Kidney Injury in the Intensive Care Unit: A Systematic Review. Intensive Care Med (2016) 42(2):137–46. doi: 10.1007/s00134-015-4151-0
31. Chawla LS, Amdur RL, Shaw AD, Faselis C, Palant CE, Kimmel PL. Association Between AKI and Long-Term Renal and Cardiovascular Outcomes in United States Veterans. Clin J Am Soc Nephrol CJASN (2014) 9(3):448–56. doi: 10.2215/CJN.02440213
32. Chen Z, Chen L, Yao G, Yang W, Yang K, Xiong C. Novel Blood Cytokine-Based Model for Predicting Severe Acute Kidney Injury and Poor Outcomes After Cardiac Surgery. J Am Heart Assoc (2020) 9(22):e018004. doi: 10.1161/JAHA.120.018004
33. Hu P, Chen Y, Wu Y, Song L, Zhang L, Li Z, et al. Development and Validation of a Model for Predicting Acute Kidney Injury After Cardiac Surgery in Patients of Advanced Age. J cardiac Surg (2021) 36(3):806–14. doi: 10.1111/jocs.15249
34. Park S, Cho H, Park S, Lee S, Kim K, Yoon HJ, et al. Simple Postoperative AKI Risk (SPARK) Classification Before Noncardiac Surgery: A Prediction Index Development Study With External Validation. J Am Soc Nephrol JASN (2019) 30(1):170–81. doi: 10.1681/ASN.2018070757
35. Zhao BC, Zhuang PP, Lei SH, Qiu SD, Yang X, Li C, et al. Pre-Operative N-Terminal Pro-B-Type Natriuretic Peptide for Prediction of Acute Kidney Injury After Noncardiac Surgery: A Retrospective Cohort Study. Eur J Anaesthesiol (2021) 38(6):591–9. doi: 10.1097/EJA.0000000000001495
36. Zhou J, Bai Y, Wang X, Yang J, Fu P, Cai D, et al. A Simple Risk Score for Prediction of Sepsis Associated-Acute Kidney Injury in Critically Ill Patients. J Nephrol (2019) 32(6):947–56. doi: 10.1007/s40620-019-00625-y
37. Fan C, Ding X, Song Y. A New Prediction Model for Acute Kidney Injury in Patients With Sepsis. Ann Palliative Med (2021) 10(2):1772–8. doi: 10.21037/apm-20-1117
38. Li Y, Chen X, Shen Z, Wang Y, Hu J, Zhang Y, et al. Prediction Models for Acute Kidney Injury in Patients With Gastrointestinal Cancers: A Real-World Study Based on Bayesian Networks. Renal fail (2020) 42(1):869–76. doi: 10.1080/0886022X.2020.1810068
39. Huang H, Liu Y, Wu M, Gao Y, Yu X. Development and Validation of a Risk Stratification Model for Predicting the Mortality of Acute Kidney Injury in Critical Care Patients. Ann Trans Med (2021) 9(4):323. doi: 10.21037/atm-20-5723
40. James MT, Pannu N, Hemmelgarn BR, Austin PC, Tan Z, McArthur E, et al. Derivation and External Validation of Prediction Models for Advanced Chronic Kidney Disease Following Acute Kidney Injury. Jama (2017) 318(18):1787–97. doi: 10.1001/jama.2017.16326
41. Zeng X, McMahon GM, Brunelli SM, Bates DW, Waikar SS. Incidence, Outcomes, and Comparisons Across Definitions of AKI in Hospitalized Individuals. Clin J Am Soc Nephrol CJASN (2014) 9(1):12–20. doi: 10.2215/CJN.02730313
42. Duan S, Liu Q, Pan P, Xu J, Liu N, Li Y, et al. RIFLE and AKIN Criteria for Mortality and Risk Factors of Acute Kidney Injury in Hospitalized Patients. Zhong nan da xue xue bao Yi xue ban (2013) 38(12):1243–52. doi: 10.3969/j.issn.1672-7347.2013.12.007
43. Hoste EA, Bagshaw SM, Bellomo R, Cely CM, Colman R, Cruz DN, et al. Epidemiology of Acute Kidney Injury in Critically Ill Patients: The Multinational AKI-EPI Study. Intensive Care Med (2015) 41(8):1411–23. doi: 10.1007/s00134-015-3934-7
44. Xia M, Liu K, Feng J, Zheng Z, Xie X. Prevalence and Risk Factors of Type 2 Diabetes and Prediabetes Among 53,288 Middle-Aged and Elderly Adults in China: A Cross-Sectional Study. Diabetes Metab syndrome Obes Targets Ther (2021) 14:1975–85. doi: 10.2147/DMSO.S305919
45. Muroya Y, He X, Fan L, Wang S, Xu R, Fan F, et al. Enhanced Renal Ischemia-Reperfusion Injury in Aging and Diabetes. Am J Physiol Renal Physiol (2018) 315(6):F1843–F54. doi: 10.1152/ajprenal.00184.2018
46. Huang ES, Laiteerapong N, Liu JY, John PM, Moffet HH, Karter AJ. Rates of Complications and Mortality in Older Patients With Diabetes Mellitus: The Diabetes and Aging Study. JAMA Internal Med (2014) 174(2):251–8. doi: 10.1001/jamainternmed.2013.12956
47. Fernandez-Juarez G, Luno J, Barrio V, de Vinuesa SG, Praga M, Goicoechea M, et al. 25 (OH) Vitamin D Levels and Renal Disease Progression in Patients With Type 2 Diabetic Nephropathy and Blockade of the Renin-Angiotensin System. Clin J Am Soc Nephrol CJASN (2013) 8(11):1870–6. doi: 10.2215/CJN.00910113
48. Porto CM, Silva VL, da Luz JSB, Filho BM, da Silveira VM. Association Between Vitamin D Deficiency and Heart Failure Risk in the Elderly. ESC Heart fail (2018) 5(1):63–74. doi: 10.1002/ehf2.12198
49. Hu Z, Zhang H, Yi B, Yang S, Liu J, Hu J, et al. VDR Activation Attenuate Cisplatin Induced AKI by Inhibiting Ferroptosis. Cell Death Dis (2020) 11(1):73. doi: 10.1038/s41419-020-2256-z
50. Lee H, Lee H, Lim Y. Vitamin D3 Improves Lipophagy-Associated Renal Lipid Metabolism and Tissue Damage in Diabetic Mice. Nutr Res (2020) 80:55–65. doi: 10.1016/j.nutres.2020.06.007
51. Gao J, Chen M, Wang X, Wang H, Zhuo L. Risk Factors and Prognosis of Acute Kidney Injury in Adult Hospitalized Patients: A Two-Year Outcome. Minerva Urol Nefrol (2015) 67(3):179–85.
Keywords: diabetes, acute kidney injury, prognosis, nomogram, prediction model
Citation: Mo M, Pan L, Huang Z, Liang Y, Liao Y and Xia N (2021) Development and Validation of a Prediction Model for Survival in Diabetic Patients With Acute Kidney Injury. Front. Endocrinol. 12:737996. doi: 10.3389/fendo.2021.737996
Received: 16 August 2021; Accepted: 01 December 2021;
Published: 22 December 2021.
Edited by:
Nehal Mohsen Elsherbiny, Mansoura University, EgyptReviewed by:
Ibrahim Ali, The University of Manchester, United KingdomCopyright © 2021 Mo, Pan, Huang, Liang, Liao and Xia. This is an open-access article distributed under the terms of the Creative Commons Attribution License (CC BY). The use, distribution or reproduction in other forums is permitted, provided the original author(s) and the copyright owner(s) are credited and that the original publication in this journal is cited, in accordance with accepted academic practice. No use, distribution or reproduction is permitted which does not comply with these terms.
*Correspondence: Ning Xia, bmluZ3hpYWd4bXVAMTYzLmNvbQ==
Disclaimer: All claims expressed in this article are solely those of the authors and do not necessarily represent those of their affiliated organizations, or those of the publisher, the editors and the reviewers. Any product that may be evaluated in this article or claim that may be made by its manufacturer is not guaranteed or endorsed by the publisher.
Research integrity at Frontiers
Learn more about the work of our research integrity team to safeguard the quality of each article we publish.