- 1Department of Ultrasound, Guangdong Provincial People’s Hospital, Guangdong Academy of Medical Sciences, Guangzhou, China
- 2The Second School of Clinical Medicine, Southern Medical University, Guangzhou, China
- 3Department of Ultrasonic Imaging, Xiangya Hospital, Central South University, Changsha, China
Background: Many clinicians are facing the dilemma about whether they should apply the active surveillance (AS) strategy for managing Clinically Node-negative (cN0) PTMC patients in daily clinical practice. This research plans to construct a dynamic nomogram based on network, connected with ultrasound characteristics and clinical data, to predict the risk of central lymph node metastasis (CLNM) in cN0 PTMC patients before surgery.
Methods: A retrospective analysis of 659 patients with cN0 PTMC who had underwent thyroid surgery and central compartment neck dissection. Patients were randomly (2:1) divided into the development cohort (439 patients) and validation cohort (220 patients). The group least absolute shrinkage and selection operator (Group Lasso) regression method was used to select the ultrasonic features for CLNM prediction in the development cohort. These features and clinical data were screened by the multivariable regression analysis, and the CLNM prediction model and web-based calculator were established. Receiver operating characteristic, calibration curve, Clinical impact curve and decision curve analysis (DCA) were used to weigh the performance of the prediction model in the validation set.
Results: Multivariable regression analysis showed that age, tumor size, multifocality, the number of contact surface, and real-time elastography were risk factors that could predict CLNM. The area under the curve of the prediction model in the development and validation sets were 0.78 and 0.77, respectively, with good discrimination and calibration. A web-based dynamic calculator was built. DCA proved that the prediction model had excellent net benefits and clinical practicability.
Conclusions: The web-based dynamic nomogram incorporating US and clinical features was able to forecast the risk of preoperative CLNM in cN0 PTMC patients, and has good predictive performance. As a new observational indicator, NCS can provide additional predictive information.
Introduction
The increase in the occurrence of thyroid cancer is mainly due to the increase in the incidence of papillary thyroid microcarcinoma (PTMC). Hence, PTMC dominates in the diagnosis and treatment of thyroid cancer. Since most tumors are not clinically obvious or palpable, most PTMCs are diagnosed by high-resolution ultrasound (US) or US-guided fine-needle aspiration. With these techniques becoming more common, an increasing number of cases were diagnosed as PTMC (1), leading to over-diagnosis and over-treatment.
In the recent years, the active surveillance (AS) strategy for low-risk PTMC has been gradually adopted worldwide to avoid over-treatment. However, AS is not suitable for all PTMC cases. Among them, central lymph node metastasis (CLNM) is the predominant influencing component. CLNM is more general in PTMC patients and the occurrence can be as high as 64.1% (2). PTMC patients with CLNM usually require surgery. For Clinically Node-negative (cN0) PTMC, a growing number of physicians tend to apply AS. However, many clinicians are facing the dilemma about whether they should apply this management strategy in daily clinical practice (3). Despite the low probability of CLNM in cN0 PTMC, if metastasis occurs during AS, patients will have to undergo a wider range of treatments that may lead to more complications (4). Additionally, it is important to consider patient-specific psychological factors. Studies show that only 25% of patients choose AS when the cytological diagnosis is PTC (< 15 mm), while more patients choose surgery (5). Therefore, examination of cervical lymph nodes, particularly the central compartment lymph nodes, is essential for determining the surgical scope of PTMC thyroidectomy, for screening candidates suitable for AS, and for following disease progression during AS.
US is the primary method to evaluate TNM staging of PTMC before surgery and AS, which is helpful for diagnosis and evaluation of PTMC that may relapse (6). The occurrence of lateral lymph node metastasis (LLNM) was lower in PTMC than in CLNM, and US showed higher diagnostic accuracy for LLNM (7–9). Due to obesity (10), the interference from the thyroid, trachea, and other adjoining organs, the sensitivity of US in recognizing CLNM is unsatisfactory (11). Hence, to forecast CLNM in cN0 PTMC patients is extremely challenging, but also of high significance. More accurate prediction of the probability to develop CLNM will increase the willingness of clinicians and patients to opt for AS, when appropriate. Even if patients choose surgery out of fear or anxiety, it can also be used as a reference for the decision of performing central compartment neck dissection (CCND).
In the studies involving cN0 PTMC patients, the independent predictors of CLNM were not consistent (12–16). In addition, prediction of CLNM in cN0 PTMC patients is mostly centered on the postoperative period. Prediction is achieved by incorporating clinical data and pathological results (12, 13), or by combining ultrasonic features and clinical-pathological risk factors (14–16). Studies also proved that the ultrasonic predictors of cN0 PTMC can predict CLNM (14–16). However, preoperative forecast of CLNM US-based nomogram in cN0 PTMC patients and genuine visualization has not been investigated.
The purpose of this study was to advance and validate a US-based dynamic nomogram to foresee the hazard of CLNM in cN0 PTMC patients.
Materials and Methods
Patients
This research employed a single-center retrospective design, and all participated patients were anonymized. Hence, this research was permitted by the research ethics committee and the informed consent requirement was waved. Medical records from January 2018 to February 2020 were retrieved from our institution database. This research listed 659 patients totally with cN0 PTMC who met the demands. Figure 1 shows the process of patient enrollment. According to the random allocation (2:1), the study cohort was divided into the development cohort consisting of 439 patients (100 men and 339 women, average age: 42.31 ± 9.06 years) and the validation cohort consisting of 220 patients (52 men, 168 women, average age: 44.00 ± 8.90 years).
Inclusion criteria: (1) patients who underwent thyroidectomy and CCND for the first time and were diagnosed with PTMC with histopathology; (2) status of CLNM were confirmed by pathology; (3) preoperative imaging diagnosis was negative for CLNM; (4) patients who had undergone US examination in our department one month before surgery; (5) the tumors underwent real-time elastography (RTE); (6) there were complete medical data.
Exclusion criteria: (1) patients who had thyroid carcinoma surgery or treatment before; (2) patients with other thyroid malignant tumors; (3) patients with distant metastasis or malignant tumors in other organs; (4) patients with few tumor foci or lymph node (LN) metastases, and pathological diagnosis of PTMC or CLNM metastasis could not be confirmed; (5) thyroid ablation was performed before surgery; (6) incomplete US image information, or poor image quality.
US and RTE Images Analysis
During the study, all ultrasound examinations were performed by color Doppler diagnostic tools (Hitachi, Ltd., Tokyo, Japan), with high frequency of 6-13 MHz linear array probe. All patients with thyroid nodules experienced preoperative US examination, and data were stored for follow-up analysis.
Two independent radiologists with 11 and 16 years of thyroid imaging experience retrospectively analyzed these ultrasound pictures. In cases that the radiologists had different opinions, the third experts who have over 20 years of experience was involved in the discussion and a final decision was made. These three radiologists were not aware of patient’s medical data, and surgical and pathological results.
The sonographic features of the tumor under ultrasound were observed and recorded, including tumor size, tumor position, multifocality, bilateral tumor (0 represents unilateral multifocality, while 1 suggests bilateral multifocality), echogenicity, calcification patterns (Mode 0 represents no calcification, mode 1 represents microcalcification, and mode 2 represents coarse calcification. When coarse calcification and micro calcification coexisted in the lesion, it was classified as mode 1), number of contact surface (NCS, including anterior capsule, posterior capsule, upper pole, lower pole, medial and lateral, was evaluated based on US images. Capsule contact or involvement was defined as more than 25% of the tumor surface in contact or adjacent to the thyroid capsule on US images, or when the continuity of thyroid capsule echo around the tumor was interrupted. 0 represents that the tumor was not in contact with the capsule. 1 suggests that the tumor was in contact with one side of the capsule, while 2 represents that the tumor contacted ≥ two sides of the capsule), composition, shape, margin, taller-than-wide, chronic lymphocytic thyroiditis (CLT), internal color Doppler vascularity (The evaluation criteria were based on Adler grade classification (17), which represents semi quantitative classification), and cervical lymph node status (Suspicious US Features included calcification, cystic degeneration, increased cortical echo and/or rich or irregular blood flow in lymph nodes). When more than one suspicious thyroid malignancies were found, the relevant data of the largest suspicious tumor would be analyzed and recorded.
Routine US examination was followed by RTE, performed by the same radiologist. The same US machine and probe were used for elastography of thyroid nodules detected by conventional US. The elasticity score (ES) for each nodule would be assigned different points (0–4-point scale) according to Asteria et al. (18) standard. The values of RTE were: 0 for tumor ES 0-2; 1 for ES 3; 2 for ES 4.
Statistical Analysis
All tests are two-sided tests, P <0.05, the difference is statistically significant. Continuous variables used Wilcoxon-Mann-Whitney U or test t-test. Pearson χ2 test or Fisher’s exact test was employed for categorical variables. Categorical variables used Fisher’s exact test or Pearson χ2 test. As there were many multi-categorical variables, the group least absolute shrinkage and selection operator (Group lasso) method using 10-fold cross-validation was applied to choose the most predictive US features in the development dataset. Multivariable logistic regression analysis was made on the above US risk factors and clinical data, and the risk predictors and regression coefficients were obtained, and the nomogram was generated. In the development and verification data set, the receiver operating characteristic (ROC) curve and calibration curve are derived to assess the performance of the web-based nomogram. In the validation set, decision curve analysis (DCA) and clinical impact curve were applied to assess the feasibility and application value of the optimization model in clinical practice (19). Using the “Dynnom” and “shiny” software packages of the R language, a web-based probability calculator is constructed.
SPSS 26.0 software (IBM Corp., Armonk, NY, USA) and R software (Rstudio version 1.3.1073, Rstudio Inc., Boston, MA, USA) were used for statistical analysis.
Results
US and Clinical Features
Table 1 concludes the US features and clinical characteristics of the patients in the development and validation set. The positive rates of CLNM in the development and validation sets were 36.4% and 35% individually. Hence, the prevalence of CLNM in the two datasets had no significant difference (P =0.715). In addition, there were no significant differences in sex and age between the CLNM positive and the negative group, indicating the applicability of the development and validation data sets.
Construction of the Predictive Model and Nomogram
The Group Lasso was used to select the non-zero coefficient features of the prediction model in the development dataset (Figure 2). The 14 US features were reduced to four potential risk prediction factors: tumor size, multifocality, NCS, and RTE. Multivariable regression analysis presented that age was also a risk predictor in the prediction model (Table 2). The above five risk predictors were used to build a predictive model and plot a nomogram (Figure 3).
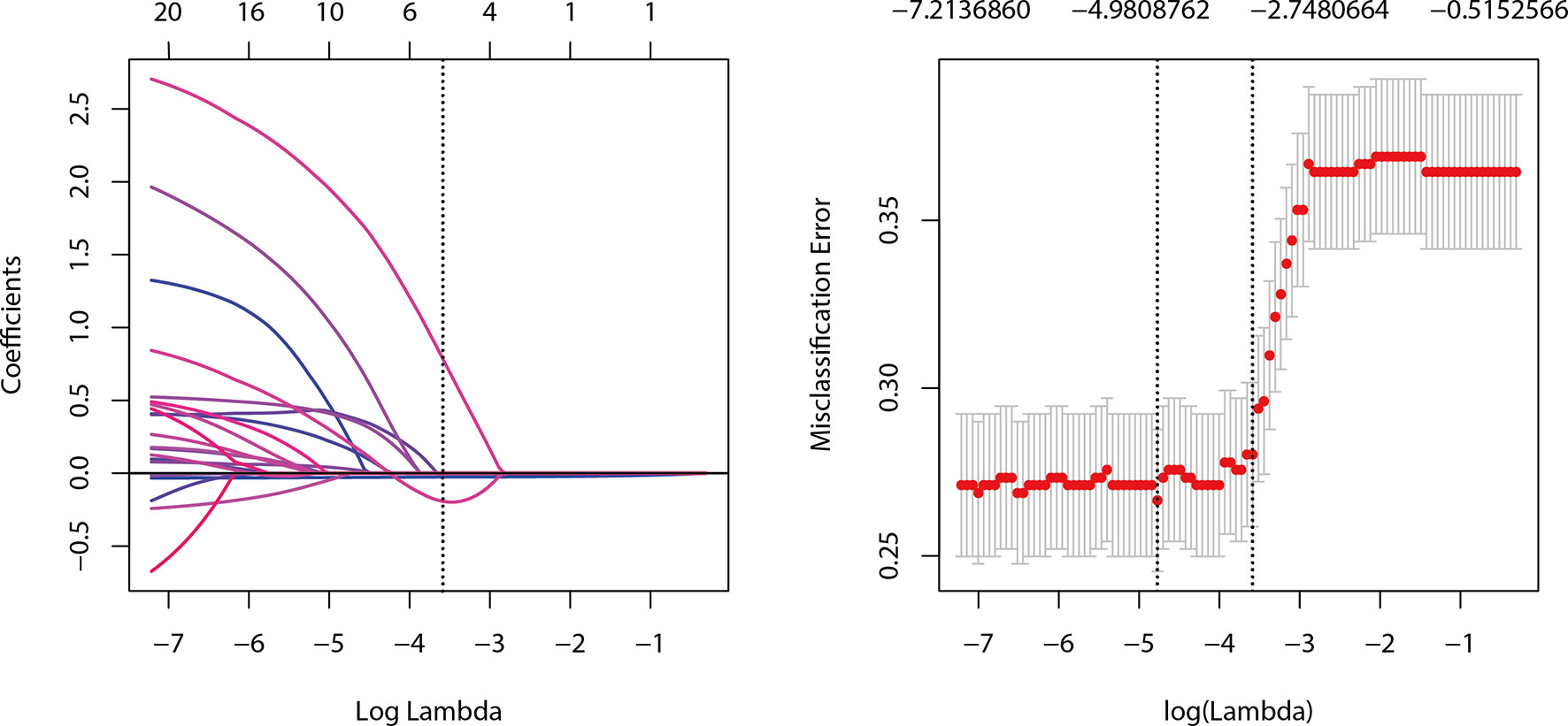
Figure 2 Selection of ultrasound features using the Group LASSO regression in the development dataset.
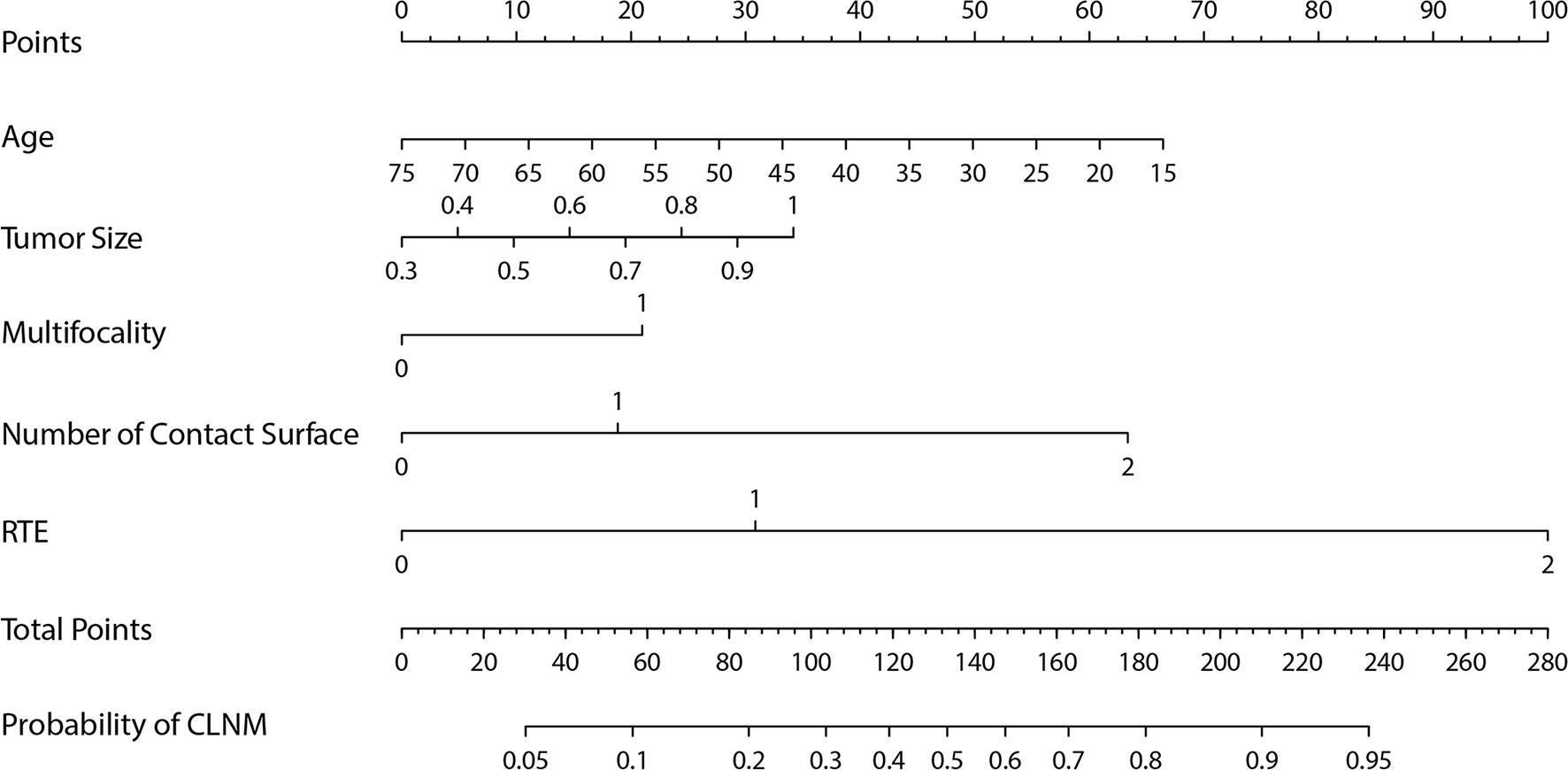
Figure 3 The nomogram for predicting the central lymph node metastasis probability in cN0 papillary thyroid microcarcinoma patients.
Performance of US-Based Nomogram
The AUC of the prediction model in the development set was 0.78 (95% CI: 0.735-0.825), and in the validation set was 0.77 (95% CI: 0.703-0.837) (Figure 4). Figure 5 showed the calibration curve of CLNM predicted by the prediction model. The Hosmer–Lemeshow test of the predictive model in the development set showed no significant difference (P = 0.956), demonstrating that the model fitted well. The calibration curve shows that the predicted value is in good agreement with the actual value, and there is no deviation from the ideal model. The Hosmer-Lemeshow test of the predictive model in the validation set also did not show a significant difference (P=0.343). According to the calibration curve, when the prediction probability was between 22% and 50%, the development model slightly overestimated the prevalence (Figure 5).
Decision Curve Analysis and Clinical Impact Curve
Figure 6 shows the DCA results of the prediction model. The abscissa represents the threshold probability, and the value on the ordinate represents the net benefits. The prediction model is represented with a red line. The gray line assumes that all patients have CLNM, while the black line indicates the hypothesis that all patients didn’t have CLNM. According to DCA, when the threshold probability was between 25-95%, the US prediction model can achieve better net benefits. Additionally, when the threshold probability was less than 25%, AS would be better.
Figure 7 demonstrates the clinical impact curve of the predictive model. The X-axis represents the threshold probability, and the Y-axis is the number of high-risk cases (based on the prediction of 1,000 cases). The red curve (number high risk) indicates the number of cases predicted as positive (high risk) by the US predicting model at each threshold probability; the blue curve (number high risk with event) refers to the number of true positive cases under each threshold probability. The curve shows that the number of positive cases predicted by the model was close to the actual number of positive cases. As the risk threshold became higher, the number of cases predicted by model was closer to the actual number of cases.
Web-Based Dynamic Calculator
Based on the results of DCA, a web-based dynamic calculator (https://predictclnminptc.shinyapps.io/DynNomapp3/) was generated to forecast the likelihood of CLNM in patients with cN0 PTMC. It is very convenient to input the risk prediction factors, such as patient’s age and US image features on the web page for real-time personalized prediction of patient’s CLNM probability. For example, the black line represents the probability (81.2%) and 95% CI (0.598-0.927) of CLNM in patients who are 30 years old, tumor diameter of 0.7 cm, multifocality, NCS ≥ 2, and ES of 3 (Figure 8).
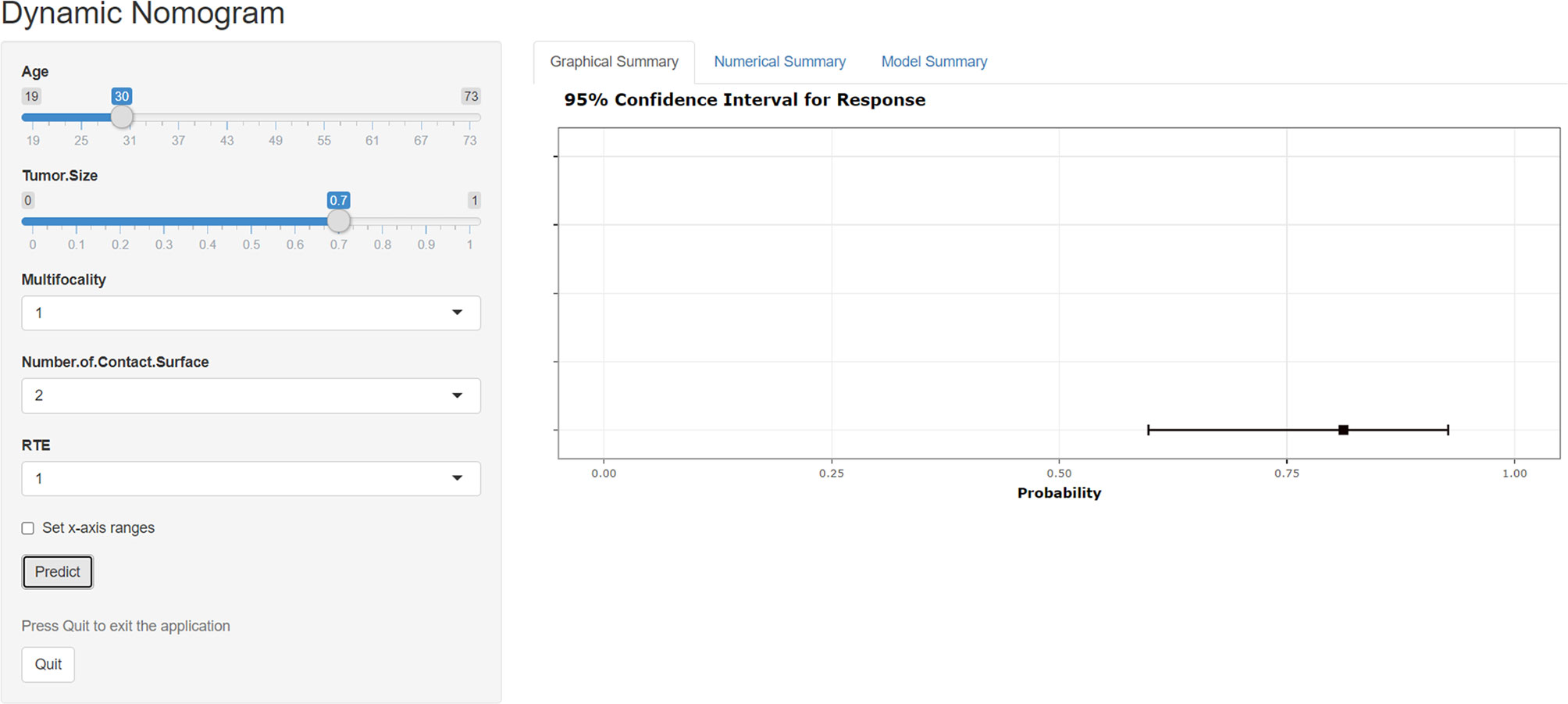
Figure 8 Screen shot of the dynamic web-based nomogram used to predict central lymph node metastasis in cN0 papillary thyroid microcarcinoma (https://predictclnminptc.shinyapps.io/DynNomapp3/).
Discussion
The study showed the occurrence of CLNM in patients who had cN0 PTMC was 36% (237/659), while other studies showed that the occurrence of CLNM in patients who had cN0 PTMC confirmed by histopathology was 31-60.9% (2, 20). Most cN0-PTMCs are inert and have a good prognosis. Conservative treatment including AS may be safe (21). However, many healthcare providers still express various concerns regarding AS provision in real life, because several studies have found that patients with PTMC and CLNM were more prone to relapse and poor prognosis (22, 23). When clinical CLNM occurs during AS, patients will receive a wider range of treatment despite the low incidence, causing more complications (4). Additionally, the psychological factors of patients are also a key factor that influences the choice of clinicians. Therefore, it is essential to determine the risk probability of CLNM, which could help patients who have low risk of cN0 PTMC to choose AS with more confidence. Even if patients choose to undergo surgery, clinicians can decide whether to perform CCND according to risk probability. As far as we know, this study has established a dynamic nomogram based on the network for the first time, combining clinical and US risk predictors to forecast the probability of CLNM in cN0 PTMC patients. This nomogram could achieve a real visualization with better operability and practicality.
According to the multivariable regression analysis, our study found that young patients, maximum tumor diameter, multifocality, NCS, and RTE were independent risk factors that may predict CLNM. These predictors can be easily obtained before surgery. The model built in this study shows a high predictive value in the development and validation cohorts, and good consistency and recognition ability. Patients with more NCS and higher RTE scores may be, relatively, at higher risk of CLNM. Moreover, young patients with multifocality and larger tumor size should not be ignored, they are more likely to develop CLNM.
The threshold for tumor size and age reported in the literature vary considerably (1, 12–14, 23, 24). For example, the threshold for age was 40 (25), 43 (26), 45 (14), and 55 years (24). The threshold for tumor size was 0.5 mm (27), 0.6 mm (13), 0.7 mm (23, 26), and 0.75 cm (12). Considering these differences and the convenience of clinical practice, we selected tumor size and age as continuous variables to analyze and build a prediction model. In this study, CLNM risk increased with decrease of age, in agreement with other studies (14, 24–26). These studies have shown that younger age suggests worse prognosis; therefore, Zhang et al. (28) proposed that dynamic observation of the central lymph nodes may be an option for patients over 60 years of age. Controversial findings were also reported with respect to sex. Some studies (16, 29) showed, through multivariable regression analysis, that sex was significantly correlated with CLNM in PTMC patients, while others showed the opposite effect (14, 30). Our study got a higher CLNM rate significantly in males (71/152, 46.7%) than in females (166/507, 32.7%), P < 0.001. However, the multivariable analysis did not demonstrate a significant relationship between sex of cN0 PTMC patients and CLNM. The conclusion was consistent with the previous study (14).
As part of the characteristics of thyroid nodules, US elastography, especially the use of semi-quantitative method, has been of primary importance in the preoperative quantitative evaluation of thyroid malignancy (31). Eventhough there are some limitations in the diagnostic value of ultrasound elastography for large nodules and coarse calcified nodules. However, PTMC usually comprises small and solid nodules. Therefore, diagnosis of PTMC with elastography is not characterized by such limitations. A meta-analysis (32) reported that the pooled sensitivity and specificity of RTE in the diagnosis of thyroid malignant nodules were higher than that of shear-wave elastography (SWE) recently. The AUC of SWE and RTE were 0.842 and 0.885 individually. This meta-analysis uncovered that RTE had greater diagnostic value than SWE in distinguishing malignant from benign nodules (32). Furthermore, the ES of RTE was intensely associated with the malignant risk stratification of TI-RADS (33). Some researches applied SWE to forecast CLNM in PTC patients, the results demonstrated that SWE can improve the performance for preoperative lymph node staging (34, 35). Nevertheless, no literature has been reported so far regarding RTE predicting CLNM in patients who have cN0 PTMC. We found that there is a significant correlation between ES and CLNM. The probability of CLNM in cN0 PTMC patients growths with increased ES, and ES 4 showed the strongest predictive performance. The reason can be that extracellular matrix cross-linking takes a vital action in the biological aggressiveness of cancer cells and associates with tissue stiffening in tissue fibrosis (36, 37). Tumor progression is accompanied by cell proliferation and fibrosis, which may influence the hardness and invasiveness of the tumor, including the evolution of lymph node metastasis (38).
A recent study using univariate analysis showed that the US feature in recognizing capsule status was related to CLNM, and the sensitivity and specificity of US assessing capsule were 71.05% and 89.27%, respectively (39). A retrospective study revealed that negative predictive value for the extrathyroidal expansion (ETE) based on detailed US appraisal was 100%, which could reliably exclude microscopic ETE and reduce the proportion of avoidable total thyroidectomy from 57% to 31% (40). Therefore, preoperative evaluation of the capsule is valuable for the upcoming clinical strategy. This study analyzed the performance of US in evaluating NCS and predicting the risk probability of CLNM. As far as we know, no previous literature has studied US-based NCS of PTMC. US-based NCS incorporated the US evaluation of capsule infiltration to a large extent, and many studies have investigated the US evaluation of capsule infiltration. Hence, this study chose NCS as the observation index. Our research revealed that US-based NCS was an independent predictor of CLNM in cN0 PTMC patients. The possibility of CLNM increased with an increase of NCS.
One research suggested that multifocality and CLNM do not have any significant correlation (16). However, this study and other researches (9, 24, 26, 41) proved that multifocality was one of the high-risk factor for CLNM. Furthermore, other study has shown that pathological CLNM in PTMC patients was significantly correlated with US-reported CLNM, multifocality, and bilateral tumors (6). We found that multifocality and bilateral tumors were both significantly correlated with CLNM through univariate analysis. Moreover, the multivariable logistic regression analysis revealed that bilateral tumors were excluded from the prediction model, which may be due to multifocality incorporated bilateral tumors and US is more accurate in diagnosis of multifocal PTMC.
As a very practical, intuitive and innovative tool, the nomogram has been widely recognized (42–44). Although some previous studies have developed nomograms to forecast the likelihood of CLNM in PTMC patients, including clinical data and postoperative pathological features, most of these predictors were obtained after surgery, and did not include preoperative US features (24). Furthermore, some researchers have studied the association between the US findings of PTMC patients and the cervical lymph nodes, but no nomogram was established or validated (45, 46). To fill this gap, this study built a personalized and quantitative nomogram to forecast the CLNM risk probability of cN0 PTMC patients based on five preoperative independent predictors. The results fully prove that the nomogram in this study shows a favorable predictive ability for CLNM in cN0 PTMC patients. In addition, a web-based dynamic nomogram was constructed, which assisted in clinical management, and can provide a personalized and visual prediction for clinicians and patients at any time and place in the network.
Some limitations exist in our study. First of all, the nomogram was formed in a single-center study, which may be biased by the institutional diagnostic model. Therefore, it is necessary to conduct further prospective, multi-center, and larger sample size studies. Second, US is limited to small (less than 2 mm) lesions; thus, it is often unable to find all lesions, which may underestimate the role of multifocality and bilateral tumors in predicting CLNM (34). Finally, evaluation of some US features is subjective, and inter-observer variability may occur. Therefore, a third radiologist participated in the final decision to reduce inter-observer disagreement. Our retrospective study preliminarily explored the possibility of US features to predict CLNM in cN0 PTMC patients, and it is necessary to conduct prospective, multi-center, and larger sample size studies.
In conclusion, the web-based dynamic nomogram incorporating US and clinical features was able to forecast the risk of preoperative CLNM in cN0 PTMC patients, and has good predictive performance. As a new observational indicator, NCS can provide additional predictive information. The possibility of CLNM increased with an increase of NCS and ES.
Data Availability Statement
The raw data supporting the conclusions of this article will be made available by the authors, without undue reservation.
Ethics Statement
The studies involving human participants were reviewed and approved by The Research Ethics Committee of Guangdong Provincial People’s Hospital, Guangdong Academy of Medical Sciences. Written informed consent from the participants’ legal guardian/next of kin was not required to participate in this study in accordance with the national legislation and the institutional requirements.
Author Contributions
CH, SC, and BZ designed this study. SS, MW, HZ, SW, and XA collected the data. CH, BZ, SS, and ZL analyzed the data. All authors contributed to the article and approved the submitted version.
Funding
This work was supported by the Guangzhou Municipal Science and Technology Planning Project (CN) (202002030235, 202102020004), Medical Scientific Research Foundation of Guangdong Province (A2019080).
Conflict of Interest
The authors declare that the research was conducted in the absence of any commercial or financial relationships that could be construed as a potential conflict of interest.
Publisher’s Note
All claims expressed in this article are solely those of the authors and do not necessarily represent those of their affiliated organizations, or those of the publisher, the editors and the reviewers. Any product that may be evaluated in this article, or claim that may be made by its manufacturer, is not guaranteed or endorsed by the publisher.
References
1. Zheng X, Wei S, Han Y, Li Y, Yu Y, Yun X, et al. Papillary Microcarcinoma of the Thyroid: Clinical Characteristics and BRAF(V600E) Mutational Status of 977 Cases. Ann Surg Oncol (2013) 20(7):2266–73. doi: 10.1245/s10434-012-2851-z
2. Wada N, Duh QY, Sugino K, Iwasaki H, Kameyama K, Mimura T, et al. Lymph Node Metastasis From 259 Papillary Thyroid Microcarcinomas: Frequency, Pattern of Occurrence and Recurrence, and Optimal Strategy for Neck Dissection. Ann Surg (2003) 237(3):399–407. doi: 10.1097/01.SLA.0000055273.58908.19
3. Sugitani I, Ito Y, Takeuchi D, Nakayama H, Masaki C, Shindo H, et al. Indications and Strategy for Active Surveillance of Adult Low-Risk Papillary Thyroid Microcarcinoma: Consensus Statements From the Japan Association of Endocrine Surgery Task Force on Management for Papillary Thyroid Microcarcinoma. Thyroid (2021) 31(2):183–92. doi: 10.1089/thy.2020.0330
4. Youngwirth LM, Adam MA, Scheri RP, Roman SA, Sosa JA. Patients Treated at Low-Volume Centers Have Higher Rates of Incomplete Resection and Compromised Outcomes: Analysis of 31,129 Patients With Papillary Thyroid Cancer. Ann Surg Oncol (2016) 23(2):403–9. doi: 10.1245/s10434-015-4867-7
5. Smulever A, Pitoia F. Active Surveillance in Papillary Thyroid Carcinoma: Not Easily Accepted But Possible in Latin America. Arch Endocrinol Metab (2019) 63(5):462–9. doi: 10.20945/2359-3997000000168
6. Jin ZQ, Lin MY, Hu WH, Li WY, Bai SJ. Gray-Scale Ultrasonography Combined With Elastography Imaging for the Evaluation of Papillary Thyroid Microcarcinoma: As a Prognostic Clinicopathology Factor. Ultrasound Med Biol (2014) 40(8):1769–77. doi: 10.1016/j.ultrasmedbio.2014.02.015
7. Cho SY, Lee TH, Ku YH, Kim HI, Lee GH, Kim MJ. Central Lymph Node Metastasis in Papillary Thyroid Microcarcinoma can be Stratified According to the Number, the Size of Metastatic Foci, and the Presence of Desmoplasia. Surgery (2015) 157(1):111–8. doi: 10.1016/j.surg.2014.05.023
8. Zeng RC, Li Q, Lin KL, Zhang W, Gao EL, Huang GL, et al. Predicting the Factors of Lateral Lymph Node Metastasis in Papillary Microcarcinoma of the Thyroid in Eastern China. Clin Transl Oncol (2012) 14(11):842–7. doi: 10.1007/s12094-012-0875-2
9. Zhang L, Wei W-J, Ji Q-H, Zhu Y-X, Wang Z-Y, Wang Y, et al. Risk Factors for Neck Nodal Metastasis in Papillary Thyroid Microcarcinoma: A Study of 1066 Patients. J Clin Endocrinol Metab (2012) 97(4):1250–7. doi: 10.1210/jc.2011-1546
10. Choi JS, Lee HS, Kim E-K, Moon HJ, Kwak JY. The Influence of Body Mass Index on the Diagnostic Performance of Pre-Operative Staging Ultrasound in Papillary Thyroid Carcinoma. Clin Endocrinol (Oxf) (2015) 83(4):550–5. doi: 10.1111/cen.12638
11. Ito Y, Tomoda C, Uruno T, Takamura Y, Miya A, Kobayashi K, et al. Clinical Significance of Metastasis to the Central Compartment From Papillary Microcarcinoma of the Thyroid. World J Surg (2006) 30(1):91–9. doi: 10.1007/s00268-005-0113-y
12. Feng JW, Ye J, Wu WX, Qu Z, Qin AC, Jiang Y. Management of Cn0 Papillary Thyroid Microcarcinoma Patients According to Risk-Scoring Model for Central Lymph Node Metastasis and Predictors of Recurrence. J Endocrinol Invest (2020) 43(12):1807–17. doi: 10.1007/s40618-020-01326-1
13. Li M, Zhu XY, Lv J, Lu K, Shen MP, Xu ZL, et al. Risk Factors for Predicting Central Lymph Node Metastasis in Papillary Thyroid Microcarcinoma (CN0): A Study of 273 Resections. Eur Rev Med Pharmacol Sci (2017) 21(17):3801–7.
14. Zhang C, Li BJ, Liu Z, Wang LL, Cheng W. Predicting the Factors Associated With Central Lymph Node Metastasis in Clinical Node-Negative (Cn0) Papillary Thyroid Microcarcinoma. Eur Arch Otorhinolaryngol (2020) 277(4):1191–8. doi: 10.1007/s00405-020-05787-1
15. Chen BD, Zhang Z, Wang KK, Shang MY, Zhao SS, Ding WB, et al. A Multivariable Model of BRAF(V600E) and Ultrasonographic Features for Predicting the Risk of Central Lymph Node Metastasis in Cn0 Papillary Thyroid Microcarcinoma. Cancer Manag Res (2019) 11:7211–7. doi: 10.2147/cmar.S199921
16. Yang Y, Chen C, Chen Z, Jiang J, Chen Y, Jin L, et al. Prediction of Central Compartment Lymph Node Metastasis in Papillary Thyroid Microcarcinoma. Clin Endocrinol (Oxf) (2014) 81(2):282–8. doi: 10.1111/cen.12417
17. Adler DD, Carson PL, Rubin JM, Quinn-Reid D. Doppler Ultrasound Color Flow Imaging in the Study of Breast Cancer: Preliminary Findings. Ultrasound Med Biol (1990) 16(6):553–9. doi: 10.1016/0301-5629(90)90020-d
18. Asteria C, Giovanardi A, Pizzocaro A, Cozzaglio L, Morabito A, Somalvico F, et al. US-Elastography in the Differential Diagnosis of Benign and Malignant Thyroid Nodules. Thyroid: Off J Am Thyroid Assoc (2008) 18(5):523–31. doi: 10.1089/thy.2007.0323
19. Vickers AJ, Cronin AM, Elkin EB, Gonen M. Extensions to Decision Curve Analysis, A Novel Method for Evaluating Diagnostic Tests, Prediction Models and Molecular Markers. BMC Med Inform Decis Mak (2008) 8:53. doi: 10.1186/1472-6947-8-53
20. Lim YC, Choi EC, Yoon YH, Kim EH, Koo BS. Central Lymph Node Metastases in Unilateral Papillary Thyroid Microcarcinoma. Br J Surg (2009) 96(3):253–7. doi: 10.1002/bjs.6484
21. Jeon MJ, Kim WG, Chung KW, Baek JH, Kim WB, Shong YK. Active Surveillance of Papillary Thyroid Microcarcinoma: Where Do We Stand? Eur Thyroid J (2019) 8(6):298–306. doi: 10.1159/000503064
22. Lee J, Song Y, Soh EY. Central Lymph Node Metastasis Is an Important Prognostic Factor in Patients With Papillary Thyroid Microcarcinoma. J Korean Med Sci (2014) 29(1):48–52. doi: 10.3346/jkms.2014.29.1.48
23. Lee KJ, Cho YJ, Kim SJ, Lee SC, Kim JG, Ahn CJ, et al. Analysis of the Clinicopathologic Features of Papillary Thyroid Microcarcinoma Based on 7-Mm Tumor Size. World J Surg (2011) 35(2):318–23. doi: 10.1007/s00268-010-0886-5
24. Wang Y, Guan Q, Xiang J. Nomogram for Predicting Central Lymph Node Metastasis in Papillary Thyroid Microcarcinoma: A Retrospective Cohort Study of 8668 Patients. Int J Surg (2018) 55:98–102. doi: 10.1016/j.ijsu.2018.05.023
25. Oh HS, Park S, Kim M, Kwon H, Song E, Sung TY, et al. Young Age and Male Sex Are Predictors of Large-Volume Central Neck Lymph Node Metastasis in Clinical N0 Papillary Thyroid Microcarcinomas. Thyroid (2017) 27(10):1285–90. doi: 10.1089/thy.2017.0250
26. Wang WH, Xu SY, Zhan WW. Clinicopathologic Factors and Thyroid Nodule Sonographic Features for Predicting Central Lymph Node Metastasis in Papillary Thyroid Microcarcinoma: A Retrospective Study of 1204 Patients. J Ultrasound Med (2016) 35(11):2475–81. doi: 10.7863/ultra.15.10012
27. Agcaoglu O, Sengun B, Ozoran E, Bilgic C, Karabay O, Taskin OC, et al. Should We Perform Routine Prophylactic Central Neck Dissection in Patients With Thyroid Papillary Microcarcinoma? Ann Ital Chir (2018) 89:485–8.
28. Zhang L, Yang J, Sun Q, Liu Y, Liang F, Liu Z, et al. Risk Factors for Lymph Node Metastasis in Papillary Thyroid Microcarcinoma: Older Patients With Fewer Lymph Node Metastases. Eur J Surg Oncol (2016) 42(10):1478–82. doi: 10.1016/j.ejso.2016.07.002
29. Zhu M, Zheng W, Xiang Y, Gu J, Wang K, Shang J. The Relationship Between Central Lymph Node Metastasis and the Distance From Tumor to Thyroid Capsule in Papillary Thyroid Microcarcinoma Without Capsule Invasion. Gland Surg (2020) 9(3):727–36. doi: 10.21037/gs-20-478
30. Siddiqui S, White MG, Antic T, Grogan RH, Angelos P, Kaplan EL, et al. Clinical and Pathologic Predictors of Lymph Node Metastasis and Recurrence in Papillary Thyroid Microcarcinoma. Thyroid: Off J Am Thyroid Assoc (2016) 26(6):807–15. doi: 10.1089/thy.2015.0429
31. Săftoiu A, Gilja OH, Sidhu PS, Dietrich CF, Cantisani V, Amy D, et al. The EFSUMB Guidelines and Recommendations for the Clinical Practice of Elastography in Non-Hepatic Applications: Update 2018. Ultraschall Med (2019) 40(4):425–53. doi: 10.1055/a-0838-9937
32. Tian W, Hao S, Gao B, Jiang Y, Zhang S, Guo L, et al. Comparison of Diagnostic Accuracy of Real-Time Elastography and Shear Wave Elastography in Differentiation Malignant From Benign Thyroid Nodules. Med (Baltimore) (2015) 94(52):e2312. doi: 10.1097/MD.0000000000002312
33. Pei S, Zhang B, Cong S, Liu J, Wu S, Dong Y, et al. Ultrasound Real-Time Tissue Elastography Improves the Diagnostic Performance of the ACR Thyroid Imaging Reporting and Data System in Differentiating Malignant From Benign Thyroid Nodules: A Summary of 1525 Thyroid Nodules. Int J Endocrinol (2020) 2020:1749351. doi: 10.1155/2020/1749351
34. Guo JN, Song LH, Yu PY, Yu SY, Deng SH, Mao XH, et al. Ultrasound Elastic Parameters Predict Central Lymph Node Metastasis of Papillary Thyroid Carcinoma. J Surg Res (2020) 253:69–78. doi: 10.1016/j.jss.2020.03.042
35. Jiang M, Li C, Tang S, Lv W, Yi A, Wang B, et al. Nomogram Based on Shear-Wave Elastography Radiomics Can Improve Preoperative Cervical Lymph Node Staging for Papillary Thyroid Carcinoma. Thyroid: Off J Am Thyroid Assoc (2020) 30(6):885–97. doi: 10.1089/thy.2019.0780
36. Levental KR, Yu H, Kass L, Lakins JN, Egeblad M, Erler JT, et al. Matrix Crosslinking Forces Tumor Progression by Enhancing Integrin Signaling. Cell (2009) 139(5):891–906. doi: 10.1016/j.cell.2009.10.027
37. Lu P, Weaver VM, Werb Z. The Extracellular Matrix: A Dynamic Niche in Cancer Progression. J Cell Biol (2012) 196(4):395–406. doi: 10.1083/jcb.201102147
38. Park AY, Kim J-A, Son EJ, Youk JH. Shear-Wave Elastography for Papillary Thyroid Carcinoma Can Improve Prediction of Cervical Lymph Node Metastasis. Ann Surg Oncol (2016) 23(Suppl 5):722–9. doi: 10.1245/s10434-016-5572-x
39. Luo X, Wang J, Xu M, Zou X, Lin Q, Zheng W, et al. Risk Model and Risk Stratification to Preoperatively Predict Central Lymph Node Metastasis in Papillary Thyroid Carcinoma. Gland Surg (2020) 9(2):300–10. doi: 10.21037/gs.2020.03.02
40. Kuo EJ, Thi WJ, Zheng F, Zanocco KA, Livhits MJ, Yeh MW. Individualizing Surgery in Papillary Thyroid Carcinoma Based on a Detailed Sonographic Assessment of Extrathyroidal Extension. Thyroid: Off J Am Thyroid Assoc (2017) 27(12):1544–9. doi: 10.1089/thy.2017.0457
41. Wu X, Li B, Zheng C, He X. Risk Factord for Central Lymph Node Metastases in Patients With Papillary Thyroid Microcarcinoma. Endocr Pract (2018) 24(12):1057–62. doi: 10.4158/EP-2018-0305
42. Balachandran VP, Gonen M, Smith JJ, DeMatteo RP. Nomograms in Oncology: More Than Meets the Eye. Lancet Oncol (2015) 16(4):e173–80. doi: 10.1016/s1470-2045(14)71116-7
43. Huang YQ, Liang CH, He L, Tian J, Liang CS, Chen X, et al. Development and Validation of a Radiomics Nomogram for Preoperative Prediction of Lymph Node Metastasis in Colorectal Cancer. J Clin Oncol (2016) 34(18):2157–64. doi: 10.1200/jco.2015.65.9128
44. Kim SK, Chai YJ, Park I, Woo JW, Lee JH, Lee KE, et al. Nomogram for Predicting Central Node Metastasis in Papillary Thyroid Carcinoma. J Surg Oncol (2017) 115(3):266–72. doi: 10.1002/jso.24512
45. Wei X, Wang M, Wang X, Zheng X, Li Y, Pan Y, et al. Prediction of Cervical Lymph Node Metastases in Papillary Thyroid Microcarcinoma by Sonographic Features of the Primary Site. Cancer Biol Med (2019) 16(3):587–94. doi: 10.20892/j.issn.2095-3941.2018.0310
46. Jin WX, Ye DR, Sun YH, Zhou XF, Wang OC, Zhang XH, et al. Prediction of Central Lymph Node Metastasis in Papillary Thyroid Microcarcinoma According to Clinicopathologic Factors and Thyroid Nodule Sonographic Features: A Case-Control Study. Cancer Manag Res (2018) 10:3237–43. doi: 10.2147/CMAR.S169741
Keywords: ultrasound, papillary thyroid microcarcinoma, clinically node-negative, central lymph node metastasis, nomogram, web-based
Citation: Huang C, Cong S, Shang S, Wang M, Zheng H, Wu S, An X, Liang Z and Zhang B (2021) Web-Based Ultrasonic Nomogram Predicts Preoperative Central Lymph Node Metastasis of cN0 Papillary Thyroid Microcarcinoma. Front. Endocrinol. 12:734900. doi: 10.3389/fendo.2021.734900
Received: 01 July 2021; Accepted: 18 August 2021;
Published: 07 September 2021.
Edited by:
Paolo Miccoli, University of Pisa, ItalyReviewed by:
Celestino Pio Lombardi, Catholic University of the Sacred Heart, ItalyStefano Spiezia, Local Health Authority Naples 1 Center, Italy
Copyright © 2021 Huang, Cong, Shang, Wang, Zheng, Wu, An, Liang and Zhang. This is an open-access article distributed under the terms of the Creative Commons Attribution License (CC BY). The use, distribution or reproduction in other forums is permitted, provided the original author(s) and the copyright owner(s) are credited and that the original publication in this journal is cited, in accordance with accepted academic practice. No use, distribution or reproduction is permitted which does not comply with these terms.
*Correspondence: Bo Zhang, zhangbo8095@csu.edu.cn
†These authors have contributed equally to this work and share first authorship