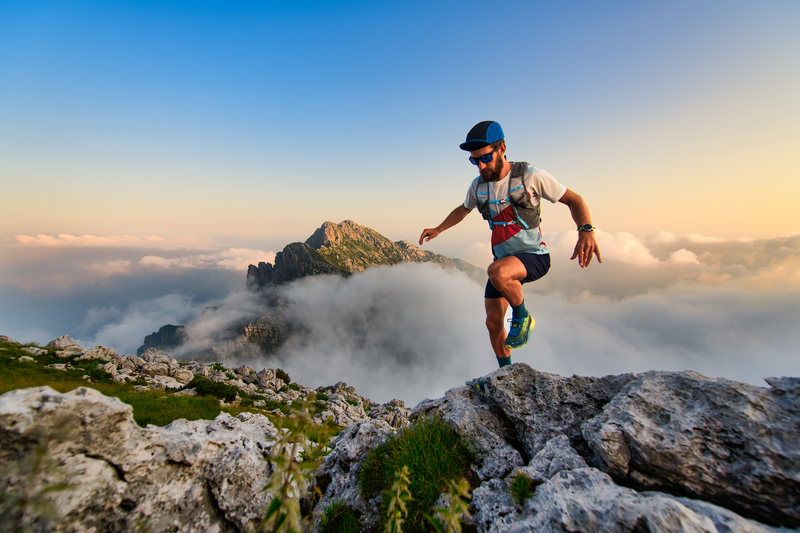
94% of researchers rate our articles as excellent or good
Learn more about the work of our research integrity team to safeguard the quality of each article we publish.
Find out more
REVIEW article
Front. Endocrinol. , 13 August 2021
Sec. Bone Research
Volume 12 - 2021 | https://doi.org/10.3389/fendo.2021.709711
This article is part of the Research Topic The “GEnomics of MusculoSkeletal traits TranslatiOnal NEtwork” (GEMSTONE) View all 6 articles
Genetic disorders of the skeleton encompass a diverse group of bone diseases differing in clinical characteristics, severity, incidence and molecular etiology. Of particular interest are the monogenic rare bone mass disorders, with the underlying genetic defect contributing to either low or high bone mass phenotype. Extensive, deep phenotyping coupled with high-throughput, cost-effective genotyping is crucial in the characterization and diagnosis of affected individuals. Massive parallel sequencing efforts have been instrumental in the discovery of novel causal genes that merit functional validation using in vitro and ex vivo cell-based techniques, and in vivo models, mainly mice and zebrafish. These translational models also serve as an excellent platform for therapeutic discovery, bridging the gap between basic science research and the clinic. Altogether, genetic studies of monogenic rare bone mass disorders have broadened our knowledge on molecular signaling pathways coordinating bone development and metabolism, disease inheritance patterns, development of new and improved bone biomarkers, and identification of novel drug targets. In this comprehensive review we describe approaches to further enhance the innovative processes taking discoveries from clinic to bench, and then back to clinic in rare bone mass disorders. We highlight the importance of cross laboratory collaboration to perform functional validation in multiple model systems after identification of a novel disease gene. We describe the monogenic forms of rare low and high rare bone mass disorders known to date, provide a roadmap to unravel the genetic determinants of monogenic rare bone mass disorders using proper phenotyping and genotyping methods, and describe different genetic validation approaches paving the way for future treatments.
Skeletal development is regulated by numerous genetic factors that guide the growth, modeling and remodeling of skeletal structures starting in early fetal development and continuing throughout life. These processes are crucial for attainment of normal height, skeletal patterning, bone shape, and mobility, but also for maintenance of normal bone mass and fracture resistance. Defects in the involved genes result in a large and heterogeneous group of disorders, collectively called skeletal dysplasias, in which the primary features are confined to the skeleton. More than 460 different forms of skeletal dysplasia, most of them monogenic, have been recognized (1). They are estimated to affect approximately 1/5,000 children (2, 3), and can have distinct clinical manifestations and course. Clinical outcomes range in severity from neonatal lethality to only mild growth retardation, deformity or fracture risk. Diagnosis is based on growth pattern and other clinical characteristics, skeletal imaging, bone density testing, biochemical diagnostics, and genetic tests. Although the genetic basis has been described and mutations in the responsible genes identified in a significant proportion of these conditions, for several distinct skeletal dysplasia phenotypes the genetic cause is still not known (1).
Within this large group of genetic skeletal disorders, monogenic disorders affecting bone mass comprise an expanding subgroup (1, 4). This includes disorders with low bone mass and skeletal fragility, and disorders leading to increased bone mass, both commonly associated with extra-skeletal complications (5, 6). Due to significant variability in severity, diagnosis can be challenging. Importantly, the underlying molecular genetic mechanisms for these disorders remain inadequately explored and, in several entities, the causative genetic defect, and underlying cellular and molecular pathophysiology are still uncharacterized.
The various skeletal dysplasia delineated to date have provided important information about the molecular pathways governing skeletal health both in these conditions and in the general population, underscoring the significance of new gene discoveries not only for the individuals affected by the monogenic rare bone mass disorder, but also more widely to the musculoskeletal research field (7). Indeed, the large wealth of data generated from monogenic and polygenic bone mass disorders, frailty and other musculoskeletal traits, have led to establishment of GEMSTONE, a COST action (CA18139; https://cost-gemstone.eu) set up to bring together multidisciplinary researchers actively involved in the musculoskeletal field. The aim of GEMSTONE is to translate the genetic discoveries into meaningful clinical applications and personalized medicine, discussed in more detail in Koromani et al., “GEnomics of MusculoSkeletal traits TranslatiOnal NEtwork (GEMSTONE): origins, rationale, organization and prospects” (8).
This paper aims to provide an overview of the presently known monogenic disorders of low and high bone mass, and to provide a roadmap to move forward in identifying and characterizing novel genetic forms, with the aim of utilizing these discoveries to develop novel therapies. We have elucidated the various steps of this process: deep phenotyping of the patient with a potentially novel skeletal condition, genetic evaluation and discovery of the causative gene defect, functional in vitro and in vivo validation, and potential approaches for novel drug discoveries.
The composition, amount and microarchitecture of human bone tissue determines its fracture load and resistance to bending and torsional forces. Increased bone fragility with low bone mass is either acquired (most commonly secondary to medications e.g., corticosteroids, aging, immobility, low body weight, nutritional deficiencies, systemic disorders e.g., chronic inflammatory conditions, endocrine pathology), or genetic, or a multitude of both (7, 9). Relevant to GEMSTONE, more specifically Working Group 3 (Monogenic conditions – human knockout models), are the rare monogenetic forms of low and high bone mass, that result from mutations in genes regulating bone composition and function of essential proteins required for type I collagen assembly, osteoblastic bone formation, bone mineralization and osteoclastic bone resorption. The advancements in sequencing methods, especially massively parallel sequencing, have enabled identification of many new monogenic disorders caused by defects in genes that encode proteins in various metabolic pathways (10).
Osteogenesis imperfecta (OI) is, by far, the most common heritable cause of increased bone fragility. It is characterized by fractures, often at a young age, low bone mass, deformities and variable short stature, but also by a number of extra-skeletal features such as blue sclerae, dental problems, skin and joint laxity, and a hearing deficit (11). Currently, more than 20 distinct genetic entities have been grouped under this diagnosis (12). They have in common that they all, via different mechanisms, affect the quality or quantity of type I collagen, the main protein component of the skeleton. However, the spectrum of mechanisms ultimately leading to defective collagen assembly in the extracellular matrix has expanded considerably recently, necessitating several revisions in classifications (13, 14). In most cases, studies on disease mechanisms in vitro and in animal models have indicated defects in collagen secretion and structure, procollagen transport, folding, post-translational modification, processing and crosslinking. By contrast, some forms of OI are associated with impaired mineralization (IFITM5, SERPINF1), or defective osteoblast differentiation and function (SP7, WNT1), rather than a direct defect in type I collagen deposition (13, 15).
Clinically, the variability of presentation and genotypes has made it difficult to differentiate between OI and so-called primary osteoporosis, which is why we present a spectrum of these entities (Table 1). For example, non-collagen pathways associated with bone fragility include the osteoblastic WNT signaling pathway (e.g., WNT1, LRP5) which regulates bone formation and the OPG/RANKL system (e.g., TNFRSF11A) which regulates osteoclast activity. Various forms of OI and primary osteoporosis are still incompletely understood; the extremely rare conditions associated with bone fragility continue to hold secrets to our understanding of bone fragility which future research will need to explore (13, 15).
Table 1 Bone fragility conditions with low and high bone mass; the gene defects, function and clinical characteristics.
While not commonly included in bone fragility classifications, we have included pure demineralization disorders caused by a lack of bone mineral supply (genetic forms of rickets/osteomalacia) and osteopetrotic conditions that increase the density of bone mineral and fracture risk, discussed further below (Table 1).
Several rare genetic disorders with skeletal effects, collectively termed osteopetroses and sclerosing bone dysplasias, are associated with a generalized increase in BMD. These disorders can be divided into those in which bone resorption is suppressed, those in which bone formation is enhanced, and those with a disturbed balance between formation and resorption (Table 1). Of the currently described 462 genetic disorders of the skeleton, 45 are characterized by osteosclerosis or osteopetrosis, implicating 40 genes (1).
The osteopetroses are rare genetic conditions of reduced osteoclastic bone resorption. Defective bone remodeling induces skeletal sclerosis and abnormally dense, and therefore brittle bones. Osteopetrosis is classified by clinical severity (Table 1). Severe neonatal or infantile forms have the worst prognosis (16), while CLCN7 variants causing osteopetrosis late‐onset form type 2 (OPTA2) (formerly autosomal dominant type II osteopetrosis (ADOII) have a varied clinical phenotype, including asymptomatic forms (17). These mildest forms can be detected as an incidental radiographic finding (18). Pycnodysostosis is caused by defective enzymatic degradation of organic bone matrix, due to autosomal recessive mutations in the gene encoding cathepsin K. Secreted by osteoclasts, cathepsin K cleaves type I collagen (19). The characteristic bone dysplasia includes skull deformities with micrognathia, short stature, dental caries, and abnormally dense, brittle bones (20–22). Understanding of pycnodysostosis prompted development of a novel class of anti-resorptive therapy (e.g., odanacatib) (23).
By contrast, the sclerosing bone dysplasias are associated with increased bone strength and resistance to fracture due to increased bone formation (Table 1). Loss-of-function (LoF) SOST variants cause sclerosteosis, a rare condition of excessive bone overgrowth (24); a 52-kb deletion located approximately 35-kb downstream of SOST is thought responsible for the milder phenotype of van Buchem’s disease (25, 26). Sclerosteosis causes tall stature, mandible enlargement, torus palatinus and mandibularis which complicate tooth extractions (27, 28). Calvarial overgrowth can lead to compression of cranial nerves, particularly facial nerves, sometimes from infancy (27). The underlying SOST gene codes for Sclerostin, an inhibitor of WNT signaling that binds to LRP5/LRP6 co-receptors to decrease osteoblastic bone formation. LoF SOST mutations result in increased osteoblastic bone formation. Similarly, variants in LRP5 and LRP6 which prevent sclerostin binding can lead to HBM (29, 30). More recently a novel, likely LoF variant in the DNA-binding domain of SMAD9 was reported in three kindreds with HBM, displaying features of mandible enlargement, torus palatinus and torus mandibularis (31). Unusually, a feature which seems to be common to these sclerosing bone dysplasias is an inability to float!
It is evident that the spectrum of abnormal skeletal phenotypes is wide, highlighting the importance of a thorough phenotypic evaluation to determine disease characteristics and the extent of skeletal and extra-skeletal manifestations in patients with a suspected rare bone disease. A detailed patient history is essential and should provide information regarding growth and development, previous and concurrent illnesses including any medical treatment, dental health, skeletal and non-skeletal symptoms, physical activity and potential restricted mobility, and other lifestyle factors. Family history is important, both in adults and children. A fracture history should cover all fractures sustained from childhood to present age, and must be accompanied by age at fracture, fracture site, fracture mechanism (low, moderate or high-energy trauma), treatment, and fracture healing duration (1).
Careful phenotyping includes routine physical examination, with attention for the existence of extra skeletal signs of OI such as blue sclerae, dental abnormalities, hyperlaxity of skin and joints, and signs of secondary diseases like Cushing syndrome, thyroid disease or malnutrition. Laboratory investigations should include relevant parameters to determine calcium-phosphate homeostasis and to exclude secondary causes of osteoporosis. The extent of tests depends on the patient’s age and clinical manifestations, and can include e.g., serum calcium, phosphate, alkaline phosphatase, 25-hydroxyvitamin D, parathyroid hormone, thyroid function, gonadal hormones, prolactin, glucose, complete blood count, erythrocyte sedimentation rate, C-reactive protein, kidney and liver function, ferritin, celiac serology, urinary calcium excretion, and bone turnover markers. Further analyses should include appropriate radiological investigations (plain radiography, CT, MRI, radionuclide bone scintigraphy), and bone density assessment by DXA (Table 2). In selected cases, a transiliac bone biopsy may help in diagnosis but requires analysis by an experienced histopathologist with very specific bone expertise. Repeat measurements of BMD may indicate whether low bone mass is due to inadequate bone accrual or increased bone loss.
Table 2 Tools and relevant clinical outcomes to be considered for careful skeletal phenotyping in patients with a skeletal phenotype.
In a clinical setting, the means to obtain detailed information about the bone tissue characteristics are usually limited. However, if a novel skeletal phenotype with low or high bone mass is suspected, several research tools can be used to determine the bone characteristics in peripheral (pQCT, HRpQCT) or central (QCT) sites. Furthermore, a double-labelled, transiliac bone biopsy can be analysed using multiple techniques. The standard histological and histomorphometric evaluations can be complemented with back-scattering electron imaging, Raman microspectroscopy and even immunohistochemistry. In addition, a transiliac bone biopsy provides the opportunity to obtain a bone marrow sample for mesenchymal stem cell (MSC) and osteoblast cultures. In case of HBM disorder it can be hard to obtain a bone biopsy. Skin fibroblasts, retrieved from the affected individual either at the bone biopsy site or, more commonly, from the forearm skin, are also widely used in various functional studies.
Altogether, rigorous phenotyping remains an imperative step in disease discovery and interpretation of disease causation. To this effect, GEMSTONE has recognized the importance of careful phenotyping, setting up Working Group 2 (‘Phenotyping’) aimed at disentangling the complex heterogenous components of monogenic and polygenic bone disease into more well-defined processes. A detailed review of this is described elsewhere (32).
In individuals with a suspected monogenic disorder of increased or decreased bone mass, the approach presented in the flowchart could be used to identify the genetic cause (Figure 1).
Figure 1 Flowchart for identifying the underlying genetic cause in disorders with increased or decreased bone mass. Affected individuals, either as isolated cases or as part of a family study, are subjected to genetic testing in the form of targeted sequencing (using Sanger or high-throughput sequencing of candidate genes), or WES/WGS, with the latter possibly undergoing prior linkage analysis to define loci that are shared by affected relatives and thus likely to harbour the causal gene(s). Sequential filtering steps are applied to narrow down the extensive list of gene variants to a few variants that based on database and literature searches are most likely to explain the disease. Shortlisted gene variants are functionally validated using in vitro cell work or in vivo animal models.
If the clinical diagnosis is clear, the genetic analysis can be restricted to one gene or a limited number of genes. Depending on the size and the number of genes, Sanger sequencing may be appropriate. However, in most cases high-throughput sequencing using a gene panel will be more cost- and labor-efficient. For the analysis of genes involved in OI, several gene panels are commercially available. For cases with increased bone mass, availability is more limited but often the relevant genes are included in wider skeletal dysplasia gene panels. In addition, academic groups have developed gene panels for research purposes.
Since the number of gene panels is ever increasing and patients with less severe phenotypes are subjected to genetic investigations, clinicians often face difficulties interpreting genetic results. A general and reasonable recommendation is to follow the American College of Medical Genetics and Genomics (ACMG) guidelines (33) and only report those variants found to be either ‘likely pathogenic’ or ‘pathogenic’ (ACMG scores 4 and 5, respectively). For variants of unknown significance (ACMG = 3) the clinical significance remains uncertain and further work may be required to confirm or refute pathogenicity of the variant. Sometimes, analyses of family members can help to identify whether a variant segregates with the phenotype. In research settings, it would be optimal to set up a “rapid throughput screening pipeline” to help prioritize the most interesting potentially causal variants, which might be carried forward for functional analyses.
A negative genetic screening result might indicate the involvement of a currently unknown disease gene, as such genes are obviously not included in established gene panels. An exome-wide analysis starting from data generated by whole exome sequencing (WES) or whole genome sequencing (WGS), assessing all genes in the human genome, may offer an opportunity to identify these novel genes and/or gene variants. However, this approach, as well as the use of extended gene panels, will result in the identification of a large number of gene variants, necessitating variant filtering and variant prioritization in order to end up with a short list of putative causal variants.
A plethora of filtering steps can be used for WES and WGS data analyses. First degree relatives share 50% of their genome. Thus, inclusion of other affected and non-affected relatives from an extended family can help eliminate the shared benign genetic variation shifting the focus on causal gene variants. This is a pertinent step when dealing with the wealth of data generated from high-throughput sequencing. Contrary to the family-based approach, incorporation of unrelated patients sharing the phenotype should be done with caution. The genetic basis of phenotypically similar monogenic conditions can be heterogeneous, and assuming a shared disease-causing gene variant in all similarly affected individuals may well result in the exclusion of the true causative variant.
The presence of variants in adult population databases such as gnomAD (34) with a frequency above a selected threshold can serve as a criterion for exclusion of these variants. According to ACMG guidelines, an allele frequency above 5% can be used directly to dismiss a variant as disease-causing. Furthermore, as shown in Figure 2, reducing this threshold to 0.1% is acceptable, as it is unlikely to result in a high risk of missing the relevant variant, especially in autosomal dominant diseases. Short insertions or deletions (indels) need special attention, because although they are often predicted to cause LoF, the variant calling algorithms are not as reliable as for single nucleotide variants (SNVs) (36). For further selection, functional predictions for the variants and/or current knowledge regarding gene function must be taken into account. Many bioinformatics tools are available for pathogenicity prediction of variants, such as Sorting Intolerant From Tolerant, SIFT [(37) (https://sift.bii.a-star.edu.sg/), Polymorphism Phenotyping v2, PolyPhen-2 (38) (http://genetics.bwh.harvard.edu/pph2/], Combined Annotation Dependent Depletion, CADD (39) (https://cadd.gs.washington.edu/), and Variant Effect Predictor, VEP (35) (https://useast.ensembl.org/Homo_sapiens/Tools/VEP), and for linking the variants with disorders by matching the clinical phenotypes as defined by HPO (human phenotype ontology) terms (40). In order to check whether other variants in the same gene have been found somewhere in the scientific community, data can be uploaded to GeneMatcher (41) (https://genematcher.org). When assessing the relevance of genes in a candidate variant list, one can integrate information from several resources. Some examples are the Musculoskeletal Knowledge Portal (42) (http://mskkp.org/), which provides a search engine for the results of genome-wide association studies (GWAS) on bone phenotypes, and the International Mouse Phenotyping consortium, IMPC (43) (www.mousephenotype.org) to consult bone phenotypes of knock-out mice. As the number of new gene discoveries increases rapidly in low and high bone mass disorders, a thorough literature review should also be a part of the assessment process. Filtering of variants to only one or very few potentially disease-causing variants should be first validated by Sanger sequencing or other genotyping methods, followed by appropriate functional studies, in vitro or in vivo, to validate the disease causality of the selected variant.
Figure 2 Allele frequencies of LoF variants in the OI database. The figure displays all variants, and their allele frequencies, reported to the OI database which is contained within the Leiden Open Variation Database (LOVD). Because the LOVD also contains benign variants, we have chosen to use LoF variants as a proxy for all pathogenic variants. As is shown, pathogenic variants are not only very rare in dominant OI genes, but they are also very rarely found in recessive OI genes. *Number of unique LoF variants in OI genes reported in the LOVD. **Genes with reported LoF variants in LOVD. Note that not all known OI genes have LoF variants, as annotated by VEP (35), reported in LOVD.
Compared to the genome-wide approach, WES is currently a more money and time saving option in terms of data storage and data analysis. However, as the costs of DNA sequencing are rapidly decreasing, WGS is becoming increasingly affordable, even within a clinical setting. One can use the obtained data as a virtual WES analysis by only looking at the coding parts of the genome. A very important advantage of WGS is that all genes are in general completely covered with relatively even coverage, unlike WES which requires an additional step of DNA capture that results in incomplete and uneven coverage of some genes. Furthermore, WGS allows for structural variant analysis (44) and opens up possibilities to gain insights into the involvement of non-coding parts of the human genome.
As described, filtering of identified variants by WES is already a difficult process which becomes even more challenging when using WGS data. The number of variants increases exponentially and the development of tools for validation of variants in non-coding areas is currently one of the most challenging aspects of genetic research. Copy number variant (CNV) detection, although possible from WGS data, is limited with the current short-read technology. However, long-read massive sequencing such as that provided by PacBio or Oxford Nanopore, may become available for clinical applications in the near future. Germline variants in microRNAs and long non-coding RNAs should also be kept in mind as they have been reported as an underlying cause in a few skeletal disorders (45).
If no variant is identified by WES or WGS that can reasonably explain the patient’s high or low bone mass phenotype, it may be worth exploring patterns of gene expression using RNAseq approaches. In this way, splice variants far from the canonical splice-site can be detected, as well as monoallelic expression. Also, total absence of a given transcript or appearance of a new, potentially rare, alternative transcript unique to the patient’s RNA and absent in control samples, provides strong evidence for the involvement of the gene in disease pathogenesis. Ideally, one would choose a single-cell approach and access bone cells to obtain total RNA, which is understandably, not always feasible. Instead, proxy tissues must often be used, such as lymphocytes or skin fibroblasts. RNAseq is becoming quite popular as a second line tool for gene identification and some nice examples of new pseudo-exons due to deep intronic variants have been identified in this way (44). However, methods are relatively expensive, technically demanding and require specialized analysis.
Finally, a note about GWAS. It is important to understand that in general such studies do not serve as tools for gene discovery in monogenic diseases. GWAS performed at the population level using large cohorts, have been very powerful in defining the polygenic basis of BMD. They have been crucial in highlighting pathways and genes involved in the regulation of bone mass and fracture susceptibility. In many instances, the same gene, with rare penetrant variants causing a monogenic BMD phenotype, has been associated with common variants conferring susceptibility for the polygenic inheritance of BMD (7, 46). The paradigm of this is LRP5, a gene responsible for several monogenic conditions including HBM and osteoporosis-pseudoglioma syndrome, but also the locus of genome-wide-significant SNVs strongly associated with hip and spine BMD (47, 48). Nevertheless, this does not apply to all genes underlying monogenic skeletal dysplasias. GWAS results may soon lead to the development of a tool to calculate a polygenic risk score (PRS) for any given individual, allowing for prediction of the risk for abnormal bone mass and possibly fracture risk, based on an individual’s combination of susceptibility variants (49). While this is still not a clinically available application, there will probably be a time when we can combine PRS and rare variant scrutiny through WES or WGS to gain precision in genetic diagnosis of high or low bone mass phenotypes.
A fast and simple approach to functionally validate novel candidate SNVs is through cell-based in vitro/ex vivo studies. Experiments should be designed according to the type of identified gene variant, the disease inheritance model, gene expression levels and the associated protein function. Use of appropriate cell lines is fundamental in recapitulating the observed phenotype seen in affected individuals. Primary bone cells harbor numerous advantages since they provide insight into the function of previously unknown genes and can also be used to investigate the defective cells’ function in comparison to that exhibited by otherwise ‘healthy’ cells. For instance, primary osteoblast cultures retrieved from a type VI OI patient with a novel de novo dominant IFITM5 mutation, revealed that the defective IFITM5 resulted in a reduced SERPINF1 expression during cell differentiation, decreased type I collagen expression and secretion, and induced mineralization defects. Type VI OI is typically caused by recessive null mutations in SERPINF1. However, findings supported the relationship between the two genes and highlighted their role in different OI types and bone mineralization (50). Moreover, rescue experiments have proved to be an effective tool in recovering protein function, complementing the results obtained in gene manipulation studies (51).
Unfortunately, access to bone biopsies remains a challenge and further complicates the limited availability of abnormal tissue. To overcome this difficulty, induced pluripotent stem cells (iPSCs) derived from patient fibroblasts can be reprogrammed to differentiate into bone cells, reproducing the disease model of interest (52–54). Moreover, if patient samples are unavailable, cell lines modified by genetic engineering techniques (such as CRISPR/Cas9) can be applied for validation studies. Immortalized primary cells (from humans or mice) such as osteoblasts (bone-forming cells e.g., hFOB), mechanosensing osteocytes (e.g., ocy454, MLO-Y4), osteoclasts (bone-resorbing cells e.g., RAW264.7), bone marrow-derived MSCs (precursors of osteoblasts); and cancer-derived (i.e., osteosarcoma cells [e.g., SaOS2, MG-63], or osteolytic [e.g., PC-3]) cell lines or adipose tissue-derived stem cells can be utilized. Next, it is necessary to simulate the pathological phenotype by generating or introducing the genetic abnormality in the selected cells. The best way to achieve this is to disrupt gene function and analyze cell function. It is crucial to assess the steady state levels of the protein encoded by the mutated gene, as well as the effect of the variant on protein function. Aberrant protein function, possibly complete ablation, is expected for LoF and missense variants. Researchers can experimentally reduce gene expression at the translational level using RNAi (knockdown) (55) or interrogate gene function by completely and permanently silencing it through CRISPR/Cas9 genome editing (knockout) (56). Alternatively, identified gene variants (such as SNVs, and indels) can be introduced in plasmids expressing the gene of interest using site-directed mutagenesis (SDM) or CRISPR-directed gene editing, followed by transfection in specific cell types. Indeed, Collet et al. (2018) demonstrated the pathogenicity of several LRP5 missense variants following SDM that led to diminished luciferase activity due to reduced activation of canonical WNT signaling (57). Similarly, different PHEX variants identified in X-linked hypophosphatemic rickets, both splicing and de novo mutations, were introduced in minigene constructs by SDM and functionally confirmed by gene expression profiling (58) and evaluation of protein stability (59).
Finally, once cells are genetically modified, several tests may be applied according to the expected pathogenic phenotype in the evaluated cell type. For example, mineralization capacity may be evaluated in osteoblasts by means of Alizarin Red S staining of calcium precipitates. Alternatively, pit assays and tartrate-resistant acid phosphatase (TRAcP), together with actin ring staining can be used for exploring osteoclast differentiation and function. Bone cell-specific markers may also be measured at the messenger RNA level (using quantitative real-time PCR) or at protein level (by ELISA or Western blot) (60). As an illustration, bi-allelic variants in the tissue non-specific alkaline phosphatase (ALPL) gene, involved in the hypophosphatasia (HPP) phenotype, were expressed in human osteosarcoma (U2OS) cells and assessed by measuring ALP activity, as well as the mineral deposition using Alizarin Red S staining. Results demonstrated that the location of the variant in the ALP enzyme structure correlated with the severity of the phenotype (61). Another example is the comprehensive study of differentiated osteoclasts from Pls3 knockout and Pls3 overexpressing mouse models. PLS3 variants were found to influence bone resorption in patients with X-linked early onset low-turnover osteoporosis (62). Osteoclasts lacking or overexpressing PLS3 were evaluated using several techniques, including TRAcP staining and resorption assay; protein expression analysis by immunohistochemistry and western blotting; identification of PLS3-binding partners by mass spectrometry and co-immunoprecipitation; and gene expression profiling. Finally, authors concluded that unbalanced PLS3 levels affected osteoclast development and function by dysregulation of the NFκB pathway (62).
The current limitation of in vitro cell studies is the difficulty in predicting or interpreting the in vivo behavior since cells lack critical cell-tissue interactions, mechanical stimuli and paracrine factors that are pertinent in understanding the complexity of bone physiology. Hence, cell-based results should be interpreted with caution when extrapolated to bone as a whole organ.
One of the biggest challenges in the validation of a specific SNV as the cause of a monogenic disease is how to model the genetic condition. Although there are different strategies for validating SNVs, the use of a mouse model remains the current gold standard. Indeed, there are many key advantages and characteristics favoring this model for the investigation of heritable conditions, whether they are rare or common. These include the high degree of genetic and mechanistic conservation between mice and humans, the high fecundity, and the short life cycle and gestation period (63). Furthermore, mouse models allow the consequence of the genetic abnormality to be determined during intrauterine development, postnatal growth, adulthood and ageing and following appropriate provocation. Nevertheless, limitations concerning the use of mouse models with respect to humans exist. Indeed, some human diseases are not easily modelled in mice, most of the mouse models used in research are inbred and do not capture the genetic variation that exists in humans, and early mutant phenotypes are difficult to study. Moreover, other limitations of this animal model are represented by the differences between mouse and human skeletal structures and dynamics, lack of standardized protocols for analysis, difficulty of in vivo cell imaging, as well as statistical and ethical issues (64).
Over the years, genetic bone diseases have been studied by applying reverse and forward genetic approaches in the mouse model (65). An important example is represented by mouse models carrying variants in the Wnt1 (66) and Pls3 (62), recapitulating low bone mass syndromes according to the underlying genetic variant.
Before proceeding with the selection or generation of a mouse model, a primary consideration should be made regarding the nature of the SNV to be investigated. Firstly, cross-species gene conservation should be determined, along with the mode of inheritance of the disease (67). This represents a crucial step in establishing the design strategy or choice of animal model. Currently, different methods exist to generate a mouse model, including gene targeting embryonic stem (ES) cells, conditional mutagenesis, pronuclear injection-based transgenesis and CRISPR/Cas9-based genome editing (68). Whichever strategy is used, the model generated must have a measurable phenotype that recapitulates the human disease in terms of pathological characteristics and disease progression.
Once the mouse model has been generated and validated, the resulting bone phenotype must be assessed, possibly at different levels. One of the most commonly used methods to investigate the presence of bone alterations in small rodents is microcomputed tomography (µCT), which provides information on bone mass, density and 3D microarchitecture (69). To date, µCT allows both ex vivo and in vivo bone analysis. A quicker phenotyping approach is through classical 2D X-rays. Unlike µCT, X-ray analysis has low resolution and information is limited to the presence of gross skeletal abnormalities (69). Furthermore, ex vivo tissue retrieved from mice can be used to model bone fragility and bone quality (46) by indentation or three-point bend and compression testing.
Another essential tool for the investigation of the bone phenotype at the cellular level is histomorphometry (70). Serological analysis of several bone formation and resorption markers can also be performed, including N-Terminal Propeptide (PINP), bone ALP (bALP), TRAcP, N-terminal telopeptide (NTx), and C-terminal telopeptide (CTx) (71).
Finally, the mouse model may represent a source of primary bone cells. This aspect is very important especially if primary cells from patients are not available due to technical or ethical issues (72, 73). Overall, the mouse model remains an essential tool for modeling monogenic bone disorders. The techniques, benefits, limitations, costs and applications of different translational model systems are described in more detail in another review by GEMSTONE Working Group 4 entitled ‘Functional Investigations’ (74).
A major bottleneck in translating findings from clinics to lab benches is the need for a fast and affordable process to test gene function and drug effects. Zebrafish (Danio rerio) are a small tropical fish that are highly fecund, have a fully annotated genome (75) and are relatively cheap to maintain since they are kept in large shoals. As the vertebrate skeleton is largely conserved over evolution, zebrafish have osteoblasts, osteocytes, and osteoclasts (both mono and multi-nucleated) (76) that are tightly coupled with bone metabolism regulated by similar pathways (WNT, BMP, parathyroid hormone, etc.) as described in humans (77). Traditionally, zebrafish have been a popular model organism in developmental biology as the translucent embryos are laid extra-maternally making them very accessible for in vivo imaging experiments. More importantly, they are easy to manipulate genetically and have been used for forward genetic screening, gene knockdown (by micro-injecting antisense RNA morpholinos), or reverse genetic approaches using targeted endonucleases (gene editing) micro-injected into one-cell stage zygotes (78, 79). The embryos develop rapidly; the first cartilage structures appear at 2 days post fertilization (dpf) in the craniofacial area, whereas dermal bone elements form as early as 3 dpf. Endochondral ossification occurs as early as 5 dpf and all these processes can be imaged in vivo and tracked over time. The notochord initiates its mineralization process as early as 4 dpf and acts as a template to give rise to the spinal cord structures comprising of vertebrae and intervertebral discs (80).
With the recent advent of fast CRISPR/Cas9 protocols and in combination with the widely available transgenic reporter lines that drive fluorescent proteins under control of cell-type specific promoters (such as sp7(osx):GFP [osteoblasts] and col2a1a:mCherry [chondrocytes]), it is feasible to rapidly test gene function related to skeletal dysplasias or early mineralization defects in zebrafish larvae (77, 81, 82) (Figure 3). Furthermore, it is possible to ablate specific cell types, such as osteoblasts, chemically by fusing nitroreductase (83) with a fluorescent protein which is driven by a cell-type specific promoter (e.g. sp7(osx):mCherry-NTR). This allows temporal control of the ablation by simply adding NTR to its substrate Nifurpirinol (antibiotic) in water (84). These and other genetic tools are valuable to follow de novo osteoblastogenesis and bone formation in a mutant fish or in a pharmacological setting.
Figure 3 Cross laboratory collaboration to perform functional validation in multiple model systems following a novel gene and variant discovery. Schematic representation of proposed pipeline to functionally validate a newly identified damaging mutation in a gene. First, evolutionary conservation of the gene and ideally of the variant should be checked to determine downstream study strategies in the three main model systems relevant to bone: mouse (A), tissue culture cells (B), and zebrafish (C). These model systems are often utilized sequentially but their use in parallel is likely to enhance output. The main focus of primary functional studies are gene manipulation (knockdown or knockout) of the whole gene which generate read outs relevant to bone (i.e. CT or mineralization assays). If all three model systems provide compelling data, the pharmacogenetics phase could provide first data of potential ways to target the new protein by performing high performance computing in silico drug docking strategies and/or screen compound libraries in multi-well settings (mainly in vitro cultured cells and/or zebrafish ex vivo cultured scales). Newly identified ‘high confidence’ compounds could then be assessed in adult mice and/or zebrafish for further validation.
Only in the past few years have the adult skeletal ageing phenotypes been more thoroughly explored. Adult zebrafish have extensive endoskeletal (e.g., vertebrae, dermal skull elements, endochondral jaw elements) and exoskeletal (e.g., scales, fin rays, teeth of dermal origin) structures that can be assessed (80). As the zebrafish skeleton is fully mineralized by 3 months of age, the development of computed tomography (33) techniques for small samples (µCT) allow detailed quantification of shape, calcium content and bone volume of the adult zebrafish skeleton (82, 85). Just as in mammals, the zebrafish bone architecture responds to glucocorticoids, and loading related to physical exercise and diet (e.g., high fat, high glucose, ferric ammonium citrate and high calcitriol) (86–90). Moreover, the zebrafish exoskeleton (scales and fin rays harboring osteoblasts and osteoclasts) is easily accessible for experimental procedures and exhibit the same osteoanabolic gene expression profile (91), providing a source of bone remodeling tissue that can be used for bone injury assays (92, 93) and ex vivo multi-well culture experiments (94).
As demonstrated with the chihuahua (col1a1a) mutant, an OI model identified during a forward genetic screen, chihuahua mutant fish have increased mineralized matrix but have brittle bones that remarkably recapitulate the phenotype exhibited in affected humans (95). More detailed description of additional mutants (such as sp7, bmp1a, plod2, entpd5a, mmp9) as bone disease models have been discussed in recent reviews (77, 82, 85, 96). Since adult fish are kept in large shoals, it is possible to screen libraries of mutants for specific bone phenotypes using µCT and then correlate that to the genotype using high-throughput sequencing approaches (97). Reverse genetic protocols have improved with CRISPR/Cas9, as specific point mutations can be introduced in the zebrafish genome (98, 99). This allows the study of specific genes and gene variants implicated in HBM, LBM, and other skeletal dysplasia in zebrafish skeletal models. Hence, combining the power of genetics and the relative ease of pharmacological manipulation (in vivo and ex vivo), make zebrafish are an attractive model to study novel genetic factors discovered in humans.
Increasing knowledge of genetic factors involved in bone metabolism, particularly mechanisms controlling gene regulation, cell signaling and differentiation, has inspired the design of drugs to directly target the underlying defect by inhibiting osteoclastic bone resorption and/or promoting osteoblastic bone formation. The goal is to identify small molecular weight compounds that selectively activate or repress expression of key genes or target specific histone marks that subsequently alter the corresponding protein level and signaling pathway. This can be achieved using cells and animal models as discussed below. Alternatively, the availability of the ever-growing, highly dimensional omics data that is tissue- and species-specific has necessitated the need for computational methods using computer-aided drug design to analyze gene expression profiles for drug target predictions (100).
An initial mandatory step in drug identification and preclinical testing of a novel pharmacological compound involves the use of cells, ranging from in vitro cell cultures (primary cells, cell lines or iPCS), ex vivo bone explants, and the more recent 3D cell culture models, to determine the beneficial and toxic effects of candidate drugs. Additionally, banked stem cells with a wide variety of genetic backgrounds enable a more thorough interrogation of the therapeutic options increasing the possibility of identifying novel drug targets. Overall, the major challenge of an effective treatment is to rescue the “healthy” phenotype and, if possible, keep this improvement permanent.
Studies have also demonstrated the utility of cells in relation to drug dosing. Li et al. utilized induced primary osteoclasts to identify the minimal effective concentration exerted by zoledronic acid for osteoclast suppression with sufficient alteration in cell adhesion, migration and resorption (101). In a second example, low doses of odanacatib induced higher anti-resorptive activity in cultured osteoclasts compared to those treated with a 100-fold higher dose of the same drug. Exposure triggered the stimulation of other cysteine proteinases culminating in increased collagen degradation mimicking that observed in patients (102).
Despite their limited costs and quick turnaround time, in vitro cell experiments suffer from several pitfalls in that they cannot fully capture the complex drug effect and interactions manifested in vivo. Hence, results obtained in a culture plate should be interpreted with caution.
The use of mammalian models represents a “mandatory” step in pharmacological discovery, both prior to or following gene identification. Animal models present a more holistic and unbiased set-up for the investigation of known or novel bone bioactive drug compounds. In vivo compound testing across different model systems can be performed with varying degrees of success. Zebrafish are increasingly used for in vivo drug discovery, drug repurposing, and chemical risk assessment. They bridge the gap between in vitro cellular studies and in vivo mouse models, and in contrast to mouse models, can be scaled up for high-throughput drug screening. This is because zebrafish embryos/larvae (WT or genetically altered) can be arrayed in 96-well plates exposed to small molecules dissolved in their water and several replicates can be tested concurrently increasing statistical power. Additionally, assays are of shorter duration. Thanks to a rapidly developing skeletal system, transgenic lines (e.g., sp7(osx), oc2, runx2a, col2a1a) can be tested for specific skeletal elements and cells, circumventing the need for sacrificing. In addition, only small drug quantities are required unlike other mammalian dosing studies (103). A successful example of an osteogenic drug identified in a zebrafish chemical screen is Dorsomorphin, a SMAD-dependent BMP type-II receptor signaling inhibitor specifically named after the striking phenotypic dorsalization effect in zebrafish embryos (104), having therapeutic potential to treat fibrodysplasia ossificans progressive (FOP). Additionally, the larval superficially located operculum and adult zebrafish scales and caudal fin (before and after removal) can be used to study the drug’s effect on osteogenesis. Indeed, prednisolone-treated zebrafish scales (containing osteoblasts and osteoclasts in their native environment) reproduces the same glucocorticoid-driven osteoporotic phenotype as observed in humans (105); the phenotype is rescued with alendronate (106). Moreover, scales can be harvested from adult fish and then cultured ex vivo in a 96-well setting which allows testing of various compounds simultaneously without exposing the individual fish to potentially harmful pharmacological agents (77, 94).
Zebrafish mutants modelled on rare human bone disorders, such as OI, can also provide new avenues for drug discovery. An improvement in the degree of bone mineralization was observed in chihuahua mutant larvae treated with the chemical chaperones 4PBA and TUDCA (107), whereas the frilly fins mutant (defective bmp1a) responded to anti-resorptive therapy, similar to OI patients with BMP1 mutations (93, 108). In summary, zebrafish provide a versatile platform for drug discovery in bone disorders, with pharmacological effects seen in zebrafish and humans being highly conserved. Thus, any promising hits are more likely to succeed and can be taken to higher animal models prior to clinical trials (109).
Rodents, such as mice and rats, have been widely used for drug testing in common and rare bone diseases. A classic example is represented by ovariectomized mice or rats, which have been used to test anti-resorptive (e.g., bisphosphonates, odanacatib) and anabolic drugs (e.g., teriparatide, anti-sclerostin monoclonal antibody [Scl-Ab]) (110, 111). Moreover, the use of transgenic mouse models has also allowed drug discovery studies to be extended to rare bone diseases facilitating the development of targeted therapies (112).
The versatility of mouse models has enabled pre-clinical human studies to progress toward drug development. The effectiveness and specificity of the candidate compound, together with the biodistribution within tissues, as well as clearance and safety can be tested in mice (113). Drugs can be administered through different routes – systemic or local (114). Moreover, mice are suitable for testing different types of drugs, including small molecules, antibodies and RNA interference (siRNA, shRNA and miRNA).
There are however limitations hindering the use of mice for drug discovery. The main pitfalls remain the limited number of compounds that can be tested due to the expenses related to mouse purchasing and housing, the quantity of drugs used, and post-treatment analyses (113). Overall, these represent essential features for translating a potential therapy in the clinical setting to treat patients. Importantly, most of the discussed aspects can only be partially tested in other experimental models, including in vitro and non-vertebrate models, thus making the mouse an indispensable tool in the drug discovery pipeline.
Another crucial aspect to be considered when using in vivo animal models in drug discovery is the drug response exhibited by mice and humans. Hits that prove to be effective in animal models may fail to show effectiveness in human clinical trials. Several factors can impact the translational power of experimental therapies, including the non-reproducibility of the pre-clinical studies, the technical unfeasibility, and the poor prediction efficacy of animal models for drug safety in humans (113, 115). Although several compounds have already been tested using these approaches, only a very small number of them have thus far cleared their way to clinical trials.
An important issue to be addressed during a drug development program is the dose conversion from mice to humans (116). In fact, extrapolation of the dose from the animal model in question to humans is a crucial step that has to take into account different aspects such as varied pharmaco- kinetic and dynamics, in body weight and surface, and in the overall physiology existing between the organisms. To overcome this, different approaches for dose conversion have been described (117). In particular, the US Food and Drug Administration released guidelines for industry based on the dose conversion approach namely “dose by factor” that represents an empirical method based on the use of the No Observed Adverse Effect Levels to derive the maximum recommended starting dose for a clinical trial (118). Other approaches are specifically applied for targeted model systems. For example in the case of mouse-human drug conversion, these are based on the comparison, the similarity, or they can be pharmacokinetics-guided (118).
Based on these aspects, we therefore propose a three-step functional study and pharmacological testing pipeline (Figure 3). Step-1 requires identification of a potentially causal gene or gene variant that could act as a putative drug target. This will be used in step-2, where complementary functional studies will be performed by mouse (A), in vitro cell culture (B), and zebrafish (C) labs to gather data. These model systems are commonly tested sequentially following the identification of potential in silico hits, starting with cells followed by animal models including teleost or bony fish (zebrafish and medaka) and mammals. However, their parallel use will increase output and validation prior to testing in higher animal models and human clinical trials. If the putative target shows compelling data in multiple model systems, step-3 will be performed to discover new compounds that could act on the putative target or its pathway. In vitro cultured cells and zebrafish will act as primary complementary testing platforms for therapeutic discovery using compound(s) that could hypothetically act on the candidate protein or tractable candidates within the signaling pathway. These lists of compounds could be derived from in silico drug docking of synthetic chemical structures (e.g., by using 3D protein crystal structure), literature-based drug repurposing, or pre-made commercially available chemical libraries predicted to act on the signaling pathway. Both zebrafish (larvae or ex vivo cultured scales) and in vitro systems allow testing of these ‘low-confidence’ candidates on a (semi-)high throughput scale. When a sub-set of these compounds shows a pre-defined effect (validation) in both systems, these ‘higher confidence’ compounds with an osteogenic potential can then proceed to stage-2 where they can be further validated and tested in the terrestrial mouse system and/or adult zebrafish (Figure 3). This pipeline requires cross-discipline collaboration between several laboratories exercising their expertise. This will speed-up novel drug discovery, limit false-positive findings (since multiple labs will validate the findings independently), and reduce unnecessary animal use.
The path from discovery of the underlying genetic cause of a disease through to the development of a clinical therapy is a long and winding road, but some success stories have been seen during the recent years in the field of rare bone diseases, such as targeted therapies for HPP (119), X-linked hypophosphatemic rickets (120) and achondroplasia (121).
In the field of bone mass disorders, an example of promising treatment discovery is represented by the OPTA2, as described above, a rare monogenic disease characterized by bone fragility despite increased bone mass (122). The phenotype of OPTA2 has been characterized since the beginning of the 20th century, then in the 2000s, mutations in the CLCN7 gene were associated with the disease (123). The in vitro characterization of the pathogenic variants revealed that CLCN7 mutations negatively affected osteoclast function, impairing their ability to resorb bone matrix. Although the number of osteoclasts is normal or even increased, they were not able to properly acidify the resorption lacuna and remove mineralized bone matrix (122, 124). The first animal model for OPTA2 carrying the mouse homolog of the most frequent and well characterized human CLCN7 mutation (p.G215R) was created in 2013 (125). Interestingly, the mouse model not only recapitulated the bone defect but also allowed deep phenotyping of the disease, revealing its complex multiorgan involvement (126). To date, there has been no disease-specific therapy and patients receive only symptomatic management. Interferon gamma (Actimmune), a promising non-targeted therapeutic approach, was tested in a phase 2 clinical trial (NCT02584608). Unfortunately, despite the encouraging results obtained from the animal model (127), the clinical trial in OPTA2 patients was not successful (128). However, availability of reliable in vitro and in vivo models, and the known dominant negative nature of the disease-causing mutation, allowed the development of another innovative experimental OPTA2 targeted therapy, based on the systemic delivery of mutation-specific siRNA (73). This experimental approach has proven to be effective in pre-clinical studies, rescuing both the skeletal and extra-skeletal defects with no safety issues (73, 126). This therapy’s clinical development is expected in the coming years. Therefore, the OPTA2 story is an example of a “canonical” pipeline in the study of a monogenic condition that started from the discovery of the disease causing-mutation and hopefully will end with the clinical development of targeted therapy.
Unravelling the pathogenic mechanisms underlying rare monogenic conditions can result in novel treatment options not only for the specific rare disease but also for more common disorders. Clear illustrative examples of this are sclerosteosis and van Buchem disease, two related conditions arising from mutations affecting the SOST gene which codes the protein sclerostin (24–27, 129, 130). Sclerostin presented as an interesting drug target for osteoporosis, not only based on its function but also because of its expression pattern, being almost exclusive to bone. Furthermore, it became clear that modulation of sclerostin concentration and activity affects bone mass, as indicated by the fact that SOST SNVs are associated with BMD (131) and that heterozygous carriers for mutations causing sclerosteosis and van Buchem disease also show an increased bone mass (132). Clinical trials with a humanized monoclonal antibody that targets sclerostin (romosozumab) have shown that the drug increases bone formation and decreases bone resorption in postmenopausal women with low BMD. In 2019, romosozumab was approved as an important anabolic treatment for severe osteoporosis (133). Yet, no long-term data on romosozumab treatment efficacy or safety is available (134). Concerns have indeed been raised regarding the increased risk of cardiovascular events following romosozumab treatment in older women (135). This is not surprising as canonical WNT signaling plays a dominant role also in other pathologies, including heart disease such as myocardial infraction, cardiovascular remodeling, and congestive heart failure (136). Elevated sclerostin levels have been positively associated with increased arterial calcification, a physiological adaptation to inhibit atherosclerosis development (137). The effect of sclerostin on vascular pathophysiology has been reviewed in detail elsewhere (138). However, this brings to light a crucial caveat in romosozumab treatment that requires further attention and consideration.
These two examples illustrate that knowledge on pathogenic mechanisms underlying very rare conditions is crucial for significant advances in patient care in common disease. Only hypotheses based on scientific evidence can move the field forward, as has been demonstrated by the development of targeted therapies for HPP (119), X-linked hypophosphatemic rickets (120) and achondroplasia (121). The recent advances in genetic and molecular studies, and access to animal models are likely to decrease the time interval from disease characterization to development of targeted therapies. Importantly, the knowledge obtained from rare monogenic disorders of low and high bone mass can be used to advance therapeutic discoveries for more common disorders, especially osteoporosis.
High-throughput sequencing technologies have led to a significant increase in knowledge in the area of rare hereditary diseases (139). At the same time, methodological developments have revolutionized biological science by allowing large-scale cost-efficient sequencing, frequently integrated with proteomics, transcriptomic and other omics in a multi-omics approach. On the other hand, such data can be merged with clinical data, phenotyping information, imaging data, family history details, and biochemical results in a multi-source highly integrated data analysis process (140, 141). Combining all the available data, bioinformatics methods and Artificial Intelligence approaches with machine learning, has the potential of creating a paradigm shift in the gene discovery and consequently, in the entire diagnostic pathway (142–144).
Studies of rare conditions, more than any other disease, need structures for data integration between academia and clinical institutions in order to collect a sufficient number of patients from which patterns can emerge to underpin valid research questions. Success in such a collaborative multi-center approach relies on proper phenotyping with standardized disease classification, unified and reliable data capture, merging of data, analyses processes, and storage methods (145, 146). In addition to this, the quality of machine learning outputs and bioinformatics methods strictly depends on the quality and consistency of input data. Considering all the mentioned points, a goal of the GEMSTONE Working Group 3 is to create a roadmap for gene discoveries in the context of rare bone diseases, which requires an established process in close collaboration with all other GEMSTONE Working Groups (Figure 4) to assure data quality and impact.
Although individually rare, genetic disorders of the skeleton are of clinical relevance because of their combined overall frequency and impact. A correct diagnosis has important implications in genetic counselling when determining the mode of inheritance and the recurrence risk in affected families. Furthermore, a specific diagnosis is helpful in the follow-up and treatment of the patient, and in predicting long-term outcomes of the disorder. As highlighted in this review, the clinical and genetic spectrum of rare disorders of low and high bone mass are still inadequately understood and novel monogenic disorders still remain to be discovered. Such disorders have the potential of providing novel information about molecular pathways regulating the numerous and only partially known aspects of bone metabolism. New gene discoveries need to be extensively validated and explored in various in vitro and in vivo experiments. All this work has the potential to lead to significant scientific advancements that can be translated into improved patient care for individuals affected by rare bone diseases, and also more widely for the millions of individuals affected by osteoporosis.
Susanna Balcells, J. H. Duncan Bassett, Dylan J. M. Bergen, Eveline Boudin, Maria Luisa Brandi, Alper Cebi, Jannie Dahl Hald, Emma Duncan, Melissa M. Formosa (WG3 Leader), Natalia Garcia-Giralt, Celia L. Gregson, Daniel Grinberg, Gretl Hendrickx, Wolfgang Högler, Anders Kämpe, Douglas Kiel, Outi Mäkitie (WG3 Leader), Antonio Maurizi, Georgina McDonald, Maria Carolina Medina Gomez, Evangelia Ntzani, Diana Ovejero Crespo, Jose A. Riancho, Luca Sangiorgi, Uunur Styrkársdóttir, Anna Teti, Wim Van Hul, Graham R. Williams, Angela Xuereb-Anastasi, Wei Zhou, M Carola Zillikens
OM and MMF initiated the manuscript. DB, AK, MMF, and SB generated the figures for the manuscript. All authors contributed to the article and approved the submitted version.
Funding was obtained from the GEMSTONE COST Action (CA18139). MMF has received research funds from The University of Malta Research, Innovation and Development Trust and The Malta Community Chest Fund, and the Research Excellence Programme (REP-2020-011; Project GeOM financed by the Malta Council for Science & Technology, for and on behalf of the Foundation for Science and Technology, through the Research Excellence Programme). DB is funded by the Foundation Fellowship from Versus Arthritis (Grant no. 22044). NG-G and DC are supported by CIBERFES (CB16/10/00245 [ISCIII]), FEIOMM2019 and European Regional Development Fund (ERDF). WZ is funded by the Jaap Schouten Foundation (The Netherlands). WVH received a Methusalem-OEC grant for the project “GENOMED” and a Research Fund of the University of Antwerp (FFB190208). MB has received funding from La Fondazione Italiana Ricerca sulle Malattie dell’Osso. The Origins of Bone and Cartilage Disease Programme analysed the skeletal phenotype of knockout mice generated by the International Mouse Phenotyping Consortium (IMPC) and was funded by a Wellcome Trust Strategic Award (101123) to GW and JB. OM is funded by the Sigrid Jusélius Foundation. DG and SB declare that this study received funding from MINECO (SAF2016-75948R and PID2019-107188RB-C21). The funder was not involved in the study design, collection, analysis, interpretation of data, the writing of this article or the decision to submit it for publication.
MZ has received honoraria in the past for lectures or advice from Alexion, Amgen, Eli Lilly, Kyowa Kirin, Shire and UCB, unrelated to the current work. WH has received research support by Alexion, Kyowa Kirin, Ultragenyx and Internis Pharma. AM is co-inventors of the patented siRNA treatment for the ADO2 therapy mentioned in the article. MB has received honoraria from Amgen, Bruno Farmaceutici, Calcilytix, Kyowa Kirin; Academic grants and/or speaker: Abiogen, Alexion, Amgen, Bruno Farmaceutici, Echolight, Eli Lilly, Kyowa Kirin, MSD, NPS, Servier, Shire, SPA, Theramex, and acts as a consultant for Alexion, Bruno Farmaceutici, Kyowa Kirin, Servier, Shire, unrelated to the current work.
The remaining authors declare that the research was conducted in the absence of any commercial or financial relationships that could be construed as a potential conflict of interest.
All claims expressed in this article are solely those of the authors and do not necessarily represent those of their affiliated organizations, or those of the publisher, the editors and the reviewers. Any product that may be evaluated in this article, or claim that may be made by its manufacturer, is not guaranteed or endorsed by the publisher.
This publication is based upon work from COST Action GEMSTONE, supported by COST (European Cooperation in Science and Technology). COST is a funding agency for research and innovation networks. Our Actions help connect research initiatives across Europe and enable scientists to grow their ideas by sharing them with their peers. This boosts their research, career and innovation (www.cost.eu).
1. Mortier GR, Cohn DH, Cormier-Daire V, Hall C, Krakow D, Mundlos S, et al. Nosology and Classification of Genetic Skeletal Disorders: 2019 Revision. Am J Med Genet A (2019) 179:2393–419. doi: 10.1002/ajmg.a.61366
2. Krakow D, Rimoin DL. The Skeletal Dysplasias. Genet Med (2010) 12:327–41. doi: 10.1097/GIM.0b013e3181daae9b
4. Sabir AH, Cole T. The Evolving Therapeutic Landscape of Genetic Skeletal Disorders. Orphanet J rare Dis (2019) 14:300. doi: 10.1186/s13023-019-1222-2
5. Paccou J, Michou L, Kolta S, Debiais F, Cortet B, Guggenbuhl P. High Bone Mass in Adults. Joint Bone Spine (2018) 85:693–9. doi: 10.1016/j.jbspin.2018.01.007
6. Robinson ME, Rauch F. Mendelian Bone Fragility Disorders. Bone (2019) 126:11–7. doi: 10.1016/j.bone.2019.04.021
7. Rivadeneira F, Mäkitie O. Osteoporosis and Bone Mass Disorders: From Gene Pathways to Treatments. Trends Endocrinol Metab (2016) 27:262–81. doi: 10.1016/j.tem.2016.03.006
8. Koromani F, Alonso N, Alves I, Brandi ML, Foessl I, Formosa MM, et al. Genomics of Musculoskeletal Traits Translational Network” (GEMSTONE): Origins, Rationale, Organization and Prospects. Front Endocrinol.
9. Koromani F, Trajanoska K, Rivadeneira F, Oei L. Recent Advances in the Genetics of Fractures in Osteoporosis. Front Endocrinol (Lausanne) (2019) 10:337. doi: 10.3389/fendo.2019.00337
10. McInerney-Leo AM, Duncan EL. Massively Parallel Sequencing for Rare Genetic Disorders: Potential and Pitfalls. Front Endocrinol (2021) 11:1–10. doi: 10.3389/fendo.2020.628946
11. Marini JC, Forlino A, Bächinger HP, Bishop NJ, Byers PH, Paepe A, et al. Osteogenesis Imperfecta. Nat Rev Dis Primers (2017) 3:17052. doi: 10.1038/nrdp.2017.52
12. Marom R, Rabenhorst BM, Morello R. Osteogenesis Imperfecta: An Update on Clinical Features and Therapies. Eur J Endocrinol (2020) 183:R95–106. doi: 10.1530/eje-20-0299
13. Jovanovic M, Guterman-Ram G, Marini JC. Osteogenesis Imperfecta: Mechanisms and Signaling Pathways Connecting Classical and Rare OI Types. Endocr Rev (2021). doi: 10.1210/endrev/bnab017
14. Chetty M, Roomaney IA, Beighton P. The Evolution of the Nosology of Osteogenesis Imperfecta. Clin Genet (2021) 99:42–52. doi: 10.1111/cge.13846
15. El-Gazzar A, Högler W. Mechanisms of Bone Fragility: From Osteogenesis Imperfecta to Secondary Osteoporosis. Int J Mol Sci (2021) 22:625–43. doi: 10.3390/ijms22020625
16. Bonafe L, Cormier-Daire V, Hall C, Lachman R, Mortier G, Mundlos S, et al. Nosology and Classification of Genetic Skeletal Disorders: 2015 Revision. Am J Med Genet A (2015) 167a:2869–92. doi: 10.1002/ajmg.a.37365
17. Caetano-Lopes J, Lessard SG, Hann S, Espinoza K, Kang KS, Lim KE, et al. Clcn7(F318L/+) as a New Mouse Model of Albers-Schönberg Disease. Bone (2017) 105:253–61. doi: 10.1016/j.bone.2017.09.007
18. Bénichou OD, Laredo JD, de Vernejoul MC. Type II Autosomal Dominant Osteopetrosis (Albers-Schönberg Disease): Clinical and Radiological Manifestations in 42 Patients. Bone (2000) 26:87–93. doi: 10.1016/s8756-3282(99)00244-6
19. Motyckova G, Fisher DE. Pycnodysostosis: Role and Regulation of Cathepsin K in Osteoclast Function and Human Disease. Curr Mol Med (2002) 2:407–21. doi: 10.2174/1566524023362401
20. Donnarumma M, Regis S, Tappino B, Rosano C, Assereto S, Corsolini F, et al. Molecular Analysis and Characterization of Nine Novel CTSK Mutations in Twelve Patients Affected by Pycnodysostosis. Mutation in Brief 961. Online. Hum Mutat (2007) 28:524. doi: 10.1002/humu.9490
21. Whyte MP. Sclerosing Bone Disorders. In: Rosen C, editor. Primer on the Metabolic Bone Diseases and Disorders of Mineral Metabolism: American Society for Bone and Mineral Research. Washington, USA: The Sheridan Press (2008). p. 412–23.
22. Brömme D, Panwar P, Turan S. Cathepsin K Osteoporosis Trials, Pycnodysostosis and Mouse Deficiency Models: Commonalities and Differences. Expert Opin Drug Discovery (2016) 11:457–72. doi: 10.1517/17460441.2016.1160884
23. Eisman JA, Bone HG, Hosking DJ, McClung MR, Reid IR, Rizzoli R, et al. Odanacatib in the Treatment of Postmenopausal Women With Low Bone Mineral Density: Three-Year Continued Therapy and Resolution of Effect. J Bone Miner Res (2011) 26:242–51. doi: 10.1002/jbmr.212
24. Balemans W, Ebeling M, Patel N, Van Hul E, Olson P, Dioszegi M, et al. Increased Bone Density in Sclerosteosis Is Due to the Deficiency of a Novel Secreted Protein (SOST). Hum Mol Genet (2001) 10:537–43. doi: 10.1093/hmg/10.5.537
25. Balemans W, Patel N, Ebeling M, Van Hul E, Wuyts W, Lacza C, et al. Identification of a 52 Kb Deletion Downstream of the SOST Gene in Patients With Van Buchem Disease. J Med Genet (2002) 39:91–7. doi: 10.1136/jmg.39.2.91
26. Staehling-Hampton K, Proll S, Paeper BW, Zhao L, Charmley P, Brown A, et al. A 52-Kb Deletion in the SOST-MEOX1 Intergenic Region on 17q12-Q21 Is Associated With Van Buchem Disease in the Dutch Population. Am J Med Genet (2002) 110:144–52. doi: 10.1002/ajmg.10401
27. Hamersma H, Gardner J, Beighton P. The Natural History of Sclerosteosis. Clin Genet (2003) 63:192–7. doi: 10.1034/j.1399-0004.2003.00036.x
28. Stephen LX, Hamersma H, Gardner J, Beighton P. Dental and Oral Manifestations of Sclerosteosis. Int Dent J (2001) 51:287–90. doi: 10.1002/j.1875-595x.2001.tb00840.x
29. Delgado-Calle J, Sato AY, Bellido T. Role and Mechanism of Action of Sclerostin in Bone. Bone (2017) 96:29–37. doi: 10.1016/j.bone.2016.10.007
30. Whyte MP, McAlister WH, Zhang F, Bijanki VN, Nenninger A, Gottesman GS, et al. New Explanation for Autosomal Dominant High Bone Mass: Mutation of Low-Density Lipoprotein Receptor-Related Protein 6. Bone (2019) 127:228–43. doi: 10.1016/j.bone.2019.05.003
31. Gregson CL, Bergen DJM, Leo P, Sessions RB, Wheeler L, Hartley A, et al. A Rare Mutation in SMAD9 Associated With High Bone Mass Identifies the SMAD-Dependent BMP Signaling Pathway as a Potential Anabolic Target for Osteoporosis. J Bone Miner Res (2020) 35:92–105. doi: 10.1002/jbmr.3875
32. Foessl I, Bassett D, BjØrmerem Å, Busse B, Calado Â, Chavassieux P, et al. Translational Approaches to Musculoskeletal Phenotyping Across Humans and Animal Models – Concept Paper of Working Group 2 of the EU Cost Action GEMSTONE (“Genomics of Musculoskeletal Traits Translational Network”). Front Endocrinol.
33. Richards S, Aziz N, Bale S, Bick D, Das S, Gastier-Foster J, et al. Standards and Guidelines for the Interpretation of Sequence Variants: A Joint Consensus Recommendation of the American College of Medical Genetics and Genomics and the Association for Molecular Pathology. Genet Med (2015) 17:405–24. doi: 10.1038/gim.2015.30
34. Karczewski KJ, Francioli LC, Tiao G, Cummings BB, Alföldi J, Wang Q, et al. The Mutational Constraint Spectrum Quantified From Variation in 141,456 Humans. Nature (2020) 581:434–43. doi: 10.1038/s41586-020-2308-7
35. McLaren W, Gil L, Hunt SE, Riat HS, Ritchie GR, Thormann A, et al. The Ensembl Variant Effect Predictor. Genome Biol (2016) 17:122. doi: 10.1186/s13059-016-0974-4
36. Kishikawa T, Momozawa Y, Ozeki T, Mushiroda T, Inohara H, Kamatani Y, et al. Empirical Evaluation of Variant Calling Accuracy Using Ultra-Deep Whole-Genome Sequencing Data. Sci Rep (2019) 9:1784. doi: 10.1038/s41598-018-38346-0
37. Ng PC, Henikoff S. SIFT: Predicting Amino Acid Changes That Affect Protein Function. Nucleic Acids Res (2003) 31:3812–4. doi: 10.1093/nar/gkg509
38. Adzhubei I, Jordan DM, Sunyaev SR. Predicting Functional Effect of Human Missense Mutations Using Polyphen-2. Curr Protoc Hum Genet (2013) 1-7.20.41. doi: 10.1002/0471142905.hg0720s76
39. Rentzsch P, Witten D, Cooper GM, Shendure J, Kircher M. CADD: Predicting the Deleteriousness of Variants Throughout the Human Genome. Nucleic Acids Res (2019) 47:D886–94. doi: 10.1093/nar/gky1016
40. Smedley D, Jacobsen JO, Jäger M, Köhler S, Holtgrewe M, Schubach M, et al. Next-Generation Diagnostics and Disease-Gene Discovery With the Exomiser. Nat Protoc (2015) 10:1–7.20.41. doi: 10.1038/nprot.2015.124
41. Sobreira N, Schiettecatte F, Valle D, Hamosh A. Genematcher: A Matching Tool for Connecting Investigators With an Interest in the Same Gene. Hum Mutat (2015) 36:928–30. doi: 10.1002/humu.22844
42. Kiel DP, Kemp JP, Rivadeneira F, Westendorf JJ, Karasik D, Duncan E, et al. The Musculoskeletal Knowledge Portal: Making Omics Data Useful to the Broader Scientific Community. J Bone Miner Res (2020) 35:1626–33. doi: 10.1002/jbmr.4147
43. Muñoz-Fuentes V, Cacheiro P, Meehan TF, Aguilar-Pimentel JA, Brown SDM, Flenniken AM, et al. The International Mouse Phenotyping Consortium (IMPC): A Functional Catalogue of the Mammalian Genome That Informs Conservation. Conserv Genet (2018) 19:995–1005. doi: 10.1007/s10592-018-1072-9
44. Lindstrand A, Eisfeldt J, Pettersson M, Carvalho CMB, Kvarnung M, Grigelioniene G, et al. From Cytogenetics to Cytogenomics: Whole-Genome Sequencing as a First-Line Test Comprehensively Captures the Diverse Spectrum of Disease-Causing Genetic Variation Underlying Intellectual Disability. Genome Med (2019) 11:68. doi: 10.1186/s13073-019-0675-1
45. Grigelioniene G, Suzuki HI, Taylan F, Mirzamohammadi F, Borochowitz ZU, Ayturk UM, et al. Gain-of-Function Mutation of Microrna-140 in Human Skeletal Dysplasia. Nat Med (2019) 25:583–90. doi: 10.1038/s41591-019-0353-2
46. Morris JA, Kemp JP, Youlten SE, Laurent L, Logan JG, Chai RC, et al. An Atlas of Genetic Influences on Osteoporosis in Humans and Mice. Nat Genet (2019) 51:258–66. doi: 10.1038/s41588-018-0302-x
47. Gong Y, Slee RB, Fukai N, Rawadi G, Roman-Roman S, Reginato AM, et al. LDL Receptor-Related Protein 5 (LRP5) Affects Bone Accrual and Eye Development. Cell (2001) 107:513–23. doi: 10.1016/s0092-8674(01)00571-2
48. Little RD, Carulli JP, Del Mastro RG, Dupuis J, Osborne M, Folz C, et al. A Mutation in the LDL Receptor-Related Protein 5 Gene Results in the Autosomal Dominant High-Bone-Mass Trait. Am J Hum Genet (2002) 70:11–9. doi: 10.1086/338450
49. Sugrue LP, Desikan RS. What Are Polygenic Scores and Why Are They Important? Jama (2019) 321:1820–1. doi: 10.1001/jama.2019.3893
50. Farber CR, Reich A, Barnes AM, Becerra P, Rauch F, Cabral WA, et al. A Novel IFITM5 Mutation in Severe Atypical Osteogenesis Imperfecta Type VI Impairs Osteoblast Production of Pigment Epithelium-Derived Factor. J Bone Miner Res (2014) 29:1402–11. doi: 10.1002/jbmr.2173
51. Liu T, Li B, Zheng XF, Jiang SD, Zhou ZZ, Xu WN, et al. Chordin-Like 1 Improves Osteogenesis of Bone Marrow Mesenchymal Stem Cells Through Enhancing BMP4-SMAD Pathway. Front Endocrinol (Lausanne) (2019) 10:360. doi: 10.3389/fendo.2019.00360
52. Liu J, Cui Y, Shi L, Luan J, Zhou X, Han J. A Cellular Model for Wilson’s Disease Using Patient-Derived Induced Pluripotent Stem Cells Revealed Aberrant β-Catenin Pathway During Osteogenesis. Biochem Biophys Res Commun (2019) 513:386–91. doi: 10.1016/j.bbrc.2019.04.013
53. Csobonyeiova M, Polak S, Zamborsky R, Danisovic L. iPs Cell Technologies and Their Prospect for Bone Regeneration and Disease Modeling: A Mini Review. J Adv Res (2017) 8:321–7. doi: 10.1016/j.jare.2017.02.004
54. Wu Q, Yang B, Hu K, Cao C, Man Y, Wang P. Deriving Osteogenic Cells From Induced Pluripotent Stem Cells for Bone Tissue Engineering. Tissue Eng Part B Rev (2017) 23:1–8. doi: 10.1089/ten.TEB.2015.0559
55. Roca-Ayats N, Ng PY, Garcia-Giralt N, Falcó-Mascaró M, Cozar M, Abril JF, et al. Functional Characterization of a GGPPS Variant Identified in Atypical Femoral Fracture Patients and Delineation of the Role of GGPPS in Bone-Relevant Cell Types. J Bone Miner Res (2018) 33:2091–8. doi: 10.1002/jbmr.3580
56. Matsuda M, Yamanaka Y, Uemura M, Osawa M, Saito MK, Nagahashi A, et al. Recapitulating the Human Segmentation Clock With Pluripotent Stem Cells. Nature (2020) 580:124–9. doi: 10.1038/s41586-020-2144-9
57. Collet C, Ostertag A, Ricquebourg M, Delecourt M, Tueur G, Isidor B, et al. Primary Osteoporosis in Young Adults: Genetic Basis and Identification of Novel Variants in Causal Genes. JBMR Plus (2018) 2:12–21. doi: 10.1002/jbm4.10020
58. BinEssa HA, Zou M, Al-Enezi AF, Alomrani B, Al-Faham MSA, Al-Rijjal RA, et al. Functional Analysis of 22 Splice-Site Mutations in the PHEX, the Causative Gene in X-Linked Dominant Hypophosphatemic Rickets. Bone (2019) 125:186–93. doi: 10.1016/j.bone.2019.05.017
59. Huang J, Bao X, Xia W, Zhu L, Zhang J, Ma J, et al. Functional Analysis of a De Novo Mutation C.1692 Del a of the PHEX Gene in a Chinese Family With X-Linked Hypophosphataemic Rickets. Bone Joint Res (2019) 8:405–13. doi: 10.1302/2046-3758.88.Bjr-2018-0276.R1
60. Duan X, Liu J, Zheng X, Wang Z, Zhang Y, Hao Y, et al. Deficiency of ATP6V1H Causes Bone Loss by Inhibiting Bone Resorption and Bone Formation Through the TGF-β1 Pathway. Theranostics (2016) 6:2183–95. doi: 10.7150/thno.17140
61. Uday S, Matsumura T, Saraff V, Saito S, Orimo H, Högler W. Tissue non-Specific Alkaline Phosphatase Activity and Mineralization Capacity of Bi-Allelic Mutations From Severe Perinatal and Asymptomatic Hypophosphatasia Phenotypes: Results From an In Vitro Mutagenesis Model. Bone (2019) 127:9–16. doi: 10.1016/j.bone.2019.05.031
62. Neugebauer J, Heilig J, Hosseinibarkooie S, Ross BC, Mendoza-Ferreira N, Nolte F, et al. Plastin 3 Influences Bone Homeostasis Through Regulation of Osteoclast Activity. Hum Mol Genet (2018) 27:4249–62. doi: 10.1093/hmg/ddy318
63. Justice MJ, Dhillon P. Using the Mouse to Model Human Disease: Increasing Validity and Reproducibility. Dis Model Mech (2016) 9:101–3. doi: 10.1242/dmm.024547
64. von Scheidt M, Zhao Y, Kurt Z, Pan C, Zeng L, Yang X, et al. Applications and Limitations of Mouse Models for Understanding Human Atherosclerosis. Cell Metab (2017) 25:248–61. doi: 10.1016/j.cmet.2016.11.001
65. O’Brien CA, Morello R. Modeling Rare Bone Diseases in Animals. Curr Osteoporos Rep (2018) 16:458–65. doi: 10.1007/s11914-018-0452-x
66. Luther J, Yorgan TA, Rolvien T, Ulsamer L, Koehne T, Liao N, et al. Wnt1 Is an Lrp5-Independent Bone-Anabolic Wnt Ligand. Sci Transl Med (2018) 10:eaau7137. doi: 10.1126/scitranslmed.aau7137
67. Cox TC. Utility and Limitations of Animal Models for the Functional Validation of Human Sequence Variants. Mol Genet Genomic Med (2015) 3:375–82. doi: 10.1002/mgg3.167
68. Gurumurthy CB, O’Brien AR, Quadros RM, Adams J Jr., Alcaide P, Ayabe S, et al. Reproducibility of CRISPR-Cas9 Methods for Generation of Conditional Mouse Alleles: A Multi-Center Evaluation. Genome Biol (2019) 20:171. doi: 10.1186/s13059-019-1776-2
69. Bouxsein ML, Boyd SK, Christiansen BA, Guldberg RE, Jepsen KJ, Müller R. Guidelines for Assessment of Bone Microstructure in Rodents Using Micro-Computed Tomography. J Bone Miner Res (2010) 25:1468–86. doi: 10.1002/jbmr.141
70. Parfitt AM, Drezner MK, Glorieux FH, Kanis JA, Malluche H, Meunier PJ, et al. Bone Histomorphometry: Standardization of Nomenclature, Symbols, and Units. Report of the ASBMR Histomorphometry Nomenclature Committee. J Bone Miner Res (1987) 2:595–610. doi: 10.1002/jbmr.5650020617
71. Shetty S, Kapoor N, Bondu JD, Thomas N, Paul TV. Bone Turnover Markers: Emerging Tool in the Management of Osteoporosis. Indian J Endocrinol Metab (2016) 20:846–52. doi: 10.4103/2230-8210.192914
72. Bateman JF, Sampurno L, Maurizi A, Lamandé SR, Sims NA, Cheng TL, et al. Effect of Rapamycin on Bone Mass and Strength in the α2(I)-G610C Mouse Model of Osteogenesis Imperfecta. J Cell Mol Med (2019) 23:1735–45. doi: 10.1111/jcmm.14072
73. Maurizi A, Capulli M, Patel R, Curle A, Rucci N, Teti A. RNA Interference Therapy for Autosomal Dominant Osteopetrosis Type 2. Towards the Preclinical Development. Bone (2018) 110:343–54. doi: 10.1016/j.bone.2018.02.031
74. Rauner M, Foessl I, Formosa MM, Kague E, Prijatelj V, Alonso Lopez N, et al. Functional Validation for Skeletal Genetic Disease Using Cellular, Molecular and Animal-Modeling Techniques: A GEMSTONE Consortium Mission Statement. Front Endocrinol.
75. Howe K, Clark MD, Torroja CF, Torrance J, Berthelot C, Muffato M, et al. The Zebrafish Reference Genome Sequence and Its Relationship to the Human Genome. Nature (2013) 496:498–503. doi: 10.1038/nature12111
76. Chatani M, Takano Y, Kudo A. Osteoclasts in Bone Modeling, as Revealed by In Vivo Imaging, Are Essential for Organogenesis in Fish. Dev Biol (2011) 360:96–109. doi: 10.1016/j.ydbio.2011.09.013
77. Bergen DJM, Kague E, Hammond CL. Zebrafish as an Emerging Model for Osteoporosis: A Primary Testing Platform for Screening New Osteo-Active Compounds. Front Endocrinol (Lausanne) (2019) 10:6. doi: 10.3389/fendo.2019.00006
78. Bedell VM, Wang Y, Campbell JM, Poshusta TL, Starker CG, Krug RG 2nd, et al. In Vivo Genome Editing Using a High-Efficiency TALEN System. Nature (2012) 491:114–8. doi: 10.1038/nature11537
79. Kok FO, Shin M, Ni CW, Gupta A, Grosse AS, van Impel A, et al. Reverse Genetic Screening Reveals Poor Correlation Between Morpholino-Induced and Mutant Phenotypes in Zebrafish. Dev Cell (2015) 32:97–108. doi: 10.1016/j.devcel.2014.11.018
80. Witten PE, Harris MP, Huysseune A, Winkler C. Small Teleost Fish Provide New Insights Into Human Skeletal Diseases. Methods Cell Biol (2017) 138:321–46. doi: 10.1016/bs.mcb.2016.09.001
81. Hammond CL, Moro E. Using Transgenic Reporters to Visualize Bone and Cartilage Signaling During Development In Vivo. Front Endocrinol (Lausanne) (2012) 3:91. doi: 10.3389/fendo.2012.00091
82. Kwon RY, Watson CJ, Karasik D. Using Zebrafish to Study Skeletal Genomics. Bone (2019) 126:37–50. doi: 10.1016/j.bone.2019.02.009
83. Aker M, Rouvinski A, Hashavia S, Ta-Shma A, Shaag A, Zenvirt S, et al. An SNX10 Mutation Causes Malignant Osteopetrosis of Infancy. J Med Genet (2012) 49:221–6. doi: 10.1136/jmedgenet-2011-100520
84. Bergemann D, Massoz L, Bourdouxhe J, Carril Pardo CA, Voz ML, Peers B, et al. Nifurpirinol: A More Potent and Reliable Substrate Compared to Metronidazole for Nitroreductase-Mediated Cell Ablations. Wound Repair Regener (2018) 26:238–44. doi: 10.1111/wrr.12633
85. Busse B, Galloway JL, Gray RS, Harris MP, Kwon RY. Zebrafish: An Emerging Model for Orthopedic Research. J Orthop Res (2020) 38:925–36. doi: 10.1002/jor.24539
86. Carnovali M, Luzi L, Terruzzi I, Banfi G, Mariotti M. Metabolic and Bone Effects of High-Fat Diet in Adult Zebrafish. Endocrine (2018) 61:317–26. doi: 10.1007/s12020-017-1494-z
87. Carnovali M, Ottria R, Pasqualetti S, Banfi G, Ciuffreda P, Mariotti M. Effects of Bioactive Fatty Acid Amide Derivatives in Zebrafish Scale Model of Bone Metabolism and Disease. Pharmacol Res (2016) 104:1–8. doi: 10.1016/j.phrs.2015.12.009
88. Chen B, Yan YL, Liu C, Bo L, Li GF, Wang H, et al. Therapeutic Effect of Deferoxamine on Iron Overload-Induced Inhibition of Osteogenesis in a Zebrafish Model. Calcif Tissue Int (2014) 94:353–60. doi: 10.1007/s00223-013-9817-4
89. Suniaga S, Rolvien T, Vom Scheidt A, Fiedler IAK, Bale HA, Huysseune A, et al. Increased Mechanical Loading Through Controlled Swimming Exercise Induces Bone Formation and Mineralization in Adult Zebrafish. Sci Rep (2018) 8:3646. doi: 10.1038/s41598-018-21776-1
90. Tarasco M, Laizé V, Cardeira J, Cancela ML, Gavaia PJ. The Zebrafish Operculum: A Powerful System to Assess Osteogenic Bioactivities of Molecules With Pharmacological and Toxicological Relevance. Comp Biochem Physiol C Toxicol Pharmacol (2017) 197:45–52. doi: 10.1016/j.cbpc.2017.04.006
91. Bergen DJM, Tong Q, Shukla A, Newman E, Zethof J, Lundberg M, et al. The Transcriptome of Regenerating Zebrafish Scales Identifies Genes Involved in Human Bone Disease. bioRxiv (2020) 2020:1–35. doi: 10.1101/2020.10.08.331561
92. Kobayashi-Sun J, Yamamori S, Kondo M, Kuroda J, Ikegame M, Suzuki N, et al. Uptake of Osteoblast-Derived Extracellular Vesicles Promotes the Differentiation of Osteoclasts in the Zebrafish Scale. Commun Biol (2020) 3:190. doi: 10.1038/s42003-020-0925-1
93. Tomecka MJ, Ethiraj LP, Sánchez LM, Roehl HH, Carney TJ. Clinical Pathologies of Bone Fracture Modelled in Zebrafish. Dis Model Mech (2019) 12(9):dmm037630. doi: 10.1242/dmm.037630
94. de Vrieze E, Zethof J, Schulte-Merker S, Flik G, Metz JR. Identification of Novel Osteogenic Compounds by an Ex-Vivo Sp7:Luciferase Zebrafish Scale Assay. Bone (2015) 74:106–13. doi: 10.1016/j.bone.2015.01.006
95. Fiedler IAK, Schmidt FN, Wölfel EM, Plumeyer C, Milovanovic P, Gioia R, et al. Severely Impaired Bone Material Quality in Chihuahua Zebrafish Resembles Classical Dominant Human Osteogenesis Imperfecta. J Bone Miner Res (2018) 33:1489–99. doi: 10.1002/jbmr.3445
96. Lleras-Forero L, Winkler C, Schulte-Merker S. Zebrafish and Medaka as Models for Biomedical Research of Bone Diseases. Dev Biol (2020) 457:191–205. doi: 10.1016/j.ydbio.2019.07.009
97. Henke K, Daane JM, Hawkins MB, Dooley CM, Busch-Nentwich EM, Stemple DL, et al. Genetic Screen for Postembryonic Development in the Zebrafish (Danio Rerio): Dominant Mutations Affecting Adult Form. Genetics (2017) 207:609–23. doi: 10.1534/genetics.117.300187
98. Aksoy YA, Nguyen DT, Chow S, Chung RS, Guillemin GJ, Cole NJ, et al. Chemical Reprogramming Enhances Homology-Directed Genome Editing in Zebrafish Embryos. Commun Biol (2019) 2:198. doi: 10.1038/s42003-019-0444-0
99. Boel A, De Saffel H, Steyaert W, Callewaert B, De Paepe A, Coucke PJ, et al. CRISPR/Cas9-Mediated Homology-Directed Repair by Ssodns in Zebrafish Induces Complex Mutational Patterns Resulting From Genomic Integration of Repair-Template Fragments. Dis Model Mech (2018) 11(10):dmm035352. doi: 10.1242/dmm.035352
100. Prieto-Martínez FD, López-López E, Eurídice Juárez-Mercado K, Medina-Franco JL. Chapter 2 - Computational Drug Design Methods—Current and Future Perspectives. In: Roy K, editor. Silico Drug Design. London, United Kingdom: Elsevier: Academic Press (2019). p. 19–44. doi: 10.1016/b978-0-12-816125-8.00002-x
101. Li P, Zhao Z, Wang L, Jin X, Shen Y, Nan C, et al. Minimally Effective Concentration of Zoledronic Acid to Suppress Osteoclasts In Vitro. Exp Ther Med (2018) 15:5330–6. doi: 10.3892/etm.2018.6120
102. Pirapaharan DC, Søe K, Panwar P, Madsen JS, Bergmann ML, Overgaard M, et al. A Mild Inhibition of Cathepsin K Paradoxically Stimulates the Resorptive Activity of Osteoclasts in Culture. Calcif Tissue Int (2019) 104:92–101. doi: 10.1007/s00223-018-0472-7
103. Garcia GR, Noyes PD, Tanguay RL. Advancements in Zebrafish Applications for 21st Century Toxicology. Pharmacol Ther (2016) 161:11–21. doi: 10.1016/j.pharmthera.2016.03.009
104. Yu PB, Hong CC, Sachidanandan C, Babitt JL, Deng DY, Hoyng SA, et al. Dorsomorphin Inhibits BMP Signals Required for Embryogenesis and Iron Metabolism. Nat Chem Biol (2008) 4:33–41. doi: 10.1038/nchembio.2007.54
105. de Vrieze E, van Kessel MA, Peters HM, Spanings FA, Flik G, Metz JR. Prednisolone Induces Osteoporosis-Like Phenotype in Regenerating Zebrafish Scales. Osteoporos Int (2014) 25:567–78. doi: 10.1007/s00198-013-2441-3
106. Pasqualetti S, Congiu T, Banfi G, Mariotti M. Alendronate Rescued Osteoporotic Phenotype in a Model of Glucocorticoid-Induced Osteoporosis in Adult Zebrafish Scale. Int J Exp Pathol (2015) 96:11–20. doi: 10.1111/iep.12106
107. Gioia R, Tonelli F, Ceppi I, Biggiogera M, Leikin S, Fisher S, et al. The Chaperone Activity of 4PBA Ameliorates the Skeletal Phenotype of Chihuahua, a Zebrafish Model for Dominant Osteogenesis Imperfecta. Hum Mol Genet (2017) 26:2897–911. doi: 10.1093/hmg/ddx171
108. Asharani PV, Keupp K, Semler O, Wang W, Li Y, Thiele H, et al. Attenuated BMP1 Function Compromises Osteogenesis, Leading to Bone Fragility in Humans and Zebrafish. Am J Hum Genet (2012) 90:661–74. doi: 10.1016/j.ajhg.2012.02.026
109. Williams CH, Hong CC. Zebrafish Small Molecule Screens: Taking the Phenotypic Plunge. Comput Struct Biotechnol J (2016) 14:350–6. doi: 10.1016/j.csbj.2016.09.001
110. Cosman F. Anabolic and Antiresorptive Therapy for Osteoporosis: Combination and Sequential Approaches. Curr Osteoporos Rep (2014) 12:385–95. doi: 10.1007/s11914-014-0237-9
111. Sophocleous A, Idris AI. Rodent Models of Osteoporosis. Bonekey Rep (2014) 3:614. doi: 10.1038/bonekey.2014.109
112. Lee H. Genetically Engineered Mouse Models for Drug Development and Preclinical Trials. Biomol Ther (Seoul) (2014) 22:267–74. doi: 10.4062/biomolther.2014.074
113. Zuberi A, Lutz C. Mouse Models for Drug Discovery. Can New Tools and Technology Improve Translational Power? Ilar J (2016) 57:178–85. doi: 10.1093/ilar/ilw021
114. Turner PV, Brabb T, Pekow C, Vasbinder MA. Administration of Substances to Laboratory Animals: Routes of Administration and Factors to Consider. J Am Assoc Lab Anim Sci (2011) 50:600–13.
115. Van Norman GA. Limitations of Animal Studies for Predicting Toxicity in Clinical Trials: Is It Time to Rethink Our Current Approach? JACC: Basic to Trans Sci (2019) 4:845–54. doi: 10.1016/j.jacbts.2019.10.008
116. Reagan-Shaw S, Nihal M, Ahmad N. Dose Translation From Animal to Human Studies Revisited. FASEB Journal: Off Publ Fed Am Societies Exp Biol (2008) 22:659–61. doi: 10.1096/fj.07-9574LSF
117. Nair AB, Jacob S. A Simple Practice Guide for Dose Conversion Between Animals and Human. J Basic Clin Pharm (2016) 7:27–31. doi: 10.4103/0976-0105.177703
118. US Food and Drug Administration’s Centre for Drug Evaluation and Research (CDER). Guidance for Industry: Estimating the Maximum Safe Starting Dose in Initial Clinical Trials for Therapeutics in Adult Healthy Volunteer. Rockwell, MD (2005).
119. Whyte MP, Greenberg CR, Salman NJ, Bober MB, McAlister WH, Wenkert D, et al. Enzyme-Replacement Therapy in Life-Threatening Hypophosphatasia. N Engl J Med (2012) 366:904–13. doi: 10.1056/NEJMoa1106173
120. Carpenter TO, Whyte MP, Imel EA, Boot AM, Högler W, Linglart A, et al. Burosumab Therapy in Children With X-Linked Hypophosphatemia. N Engl J Med (2018) 378:1987–98. doi: 10.1056/NEJMoa1714641
121. Savarirayan R, Irving M, Bacino CA, Bostwick B, Charrow J, Cormier-Daire V, et al. C-Type Natriuretic Peptide Analogue Therapy in Children With Achondroplasia. N Engl J Med (2019) 381:25–35. doi: 10.1056/NEJMoa1813446
122. Maurizi A, Teti A. Osteopetrosis. In: Bilezikian T, Martin J, Clemens TL, Rosen CJ, editors. Principles of Bone Biology, 4th ed, London, United Kingdom: Elsevier: Academic Press 1553–68 (2019).
123. Cleiren E, Bénichou O, Van Hul E, Gram J, Bollerslev J, Singer FR, et al. Albers-Schönberg Disease (Autosomal Dominant Osteopetrosis, Type II) Results From Mutations in the Clcn7 Chloride Channel Gene. Hum Mol Genet (2001) 10:2861–7. doi: 10.1093/hmg/10.25.2861
124. Bollerslev J, Henriksen K, Nielsen MF, Brixen K, Van Hul W. Autosomal Dominant Osteopetrosis Revisited: Lessons From Recent Studies. Eur J Endocrinol (2013) 169:R39–57. doi: 10.1530/eje-13-0136
125. Alam I, Gray AK, Chu K, Ichikawa S, Mohammad KS, Capannolo M, et al. Generation of the First Autosomal Dominant Osteopetrosis Type II (ADO2) Disease Models. Bone (2014) 59:66–75. doi: 10.1016/j.bone.2013.10.021
126. Maurizi A, Capulli M, Curle A, Patel R, Ucci A, Côrtes JA, et al. Extra-Skeletal Manifestations in Mice Affected by Clcn7-Dependent Autosomal Dominant Osteopetrosis Type 2 Clinical and Therapeutic Implications. Bone Res (2019) 7:17. doi: 10.1038/s41413-019-0055-x
127. Alam I, Gray AK, Acton D, Gerard-O’Riley RL, Reilly AM, Econs MJ. Interferon Gamma, But Not Calcitriol Improves the Osteopetrotic Phenotypes in ADO2 Mice. J Bone Miner Res (2015) 30:2005–13. doi: 10.1002/jbmr.2545
128. Imel EA, Liu Z, Acton D, Coffman M, Gebregziabher N, Tong Y, et al. Interferon Gamma-1b Does Not Increase Markers of Bone Resorption in Autosomal Dominant Osteopetrosis. J Bone Miner Res (2019) 34:1436–45. doi: 10.1002/jbmr.3715
129. Beighton P, Barnard A, Hamersma H, van der Wouden A. The Syndromic Status of Sclerosteosis and Van Buchem Disease. Clin Genet (1984) 25:175–81. doi: 10.1111/j.1399-0004.1984.tb00481.x
130. Baron R, Kneissel M. WNT Signaling in Bone Homeostasis and Disease: From Human Mutations to Treatments. Nat Med (2013) 19:179–92. doi: 10.1038/nm.3074
131. Uitterlinden AG, Arp PP, Paeper BW, Charmley P, Proll S, Rivadeneira F, et al. Polymorphisms in the Sclerosteosis/Van Buchem Disease Gene (SOST) Region Are Associated With Bone-Mineral Density in Elderly Whites. Am J Hum Genet (2004) 75:1032–45. doi: 10.1086/426458
132. van Lierop AH, Hamdy NA, Hamersma H, van Bezooijen RL, Power J, Loveridge N, et al. Patients With Sclerosteosis and Disease Carriers: Human Models of the Effect of Sclerostin on Bone Turnover. J Bone Miner Res (2011) 26:2804–11. doi: 10.1002/jbmr.474
133. Cosman F, Crittenden DB, Adachi JD, Binkley N, Czerwinski E, Ferrari S, et al. Romosozumab Treatment in Postmenopausal Women With Osteoporosis. N Engl J Med (2016) 375:1532–43. doi: 10.1056/NEJMoa1607948
134. Khosla S. Bone Diseases: Romosozumab - on Track or Derailed? Nat Rev Endocrinol (2017) 13:697–8. doi: 10.1038/nrendo.2017.136
135. Tominaga A, Wada K, Okazaki K, Nishi H, Terayama Y, Kato Y. Early Clinical Effects, Safety, and Predictors of the Effects of Romosozumab Treatment in Osteoporosis Patients: One-Year Study. Osteoporosis International: J Established as Result Cooperation Between Eur Foundation Osteoporosis Natl Osteoporosis Foundation USA. Osteoporos Int (2021) 1–11. doi: 10.1007/s00198-021-05925-3
136. Gay A, Towler DA. Wnt Signaling in Cardiovascular Disease: Opportunities and Challenges. Curr Opin Lipidol (2017) 28:387–96. doi: 10.1097/MOL.0000000000000445
137. Krishna SM, Seto SW, Jose RJ, Li J, Morton SK, Biros E, et al. Wnt Signaling Pathway Inhibitor Sclerostin Inhibits Angiotensin II-Induced Aortic Aneurysm and Atherosclerosis. Arterioscler Thromb Vasc Biol (2017) 37:553–66. doi: 10.1161/atvbaha.116.308723
138. Catalano A, Bellone F, Morabito N, Corica F. Sclerostin and Vascular Pathophysiology. Int J Mol Sci (2020) 21:4779. doi: 10.3390/ijms21134779
139. Boycott KM, Vanstone MR, Bulman DE, MacKenzie AE. Rare-Disease Genetics in the Era of Next-Generation Sequencing: Discovery to Translation. Nat Rev Genet (2013) 14:681–91. doi: 10.1038/nrg3555
140. Hasin Y, Seldin M, Lusis A. Multi-Omics Approaches to Disease. Genome Biol (2017) 18:83. doi: 10.1186/s13059-017-1215-1
141. Kerr K, McAneney H, McKnight AJ. Protocol for a Scoping Review of Multi-Omic Analysis for Rare Diseases. BMJ Open (2019) 9:e026278. doi: 10.1136/bmjopen-2018-026278
142. de Marvao A, Dawes TJW, O’Regan DP. Artificial Intelligence for Cardiac Imaging-Genetics Research. Front Cardiovasc Med (2019) 6:195. doi: 10.3389/fcvm.2019.00195
143. Lee S, Liang X, Woods M, Reiner AS, Concannon P, Bernstein L, et al. Machine Learning on Genome-Wide Association Studies to Predict the Risk of Radiation-Associated Contralateral Breast Cancer in the WECARE Study. PloS One (2020) 15:e0226157. doi: 10.1371/journal.pone.0226157
144. Nicholls HL, John CR, Watson DS, Munroe PB, Barnes MR, Cabrera CP. Reaching the End-Game for GWAS: Machine Learning Approaches for the Prioritization of Complex Disease Loci. Front Genet (2020) 11:350. doi: 10.3389/fgene.2020.00350
145. Haneef R, Delnord M, Vernay M, Bauchet E, Gaidelyte R, Van Oyen H, et al. Innovative Use of Data Sources: A Cross-Sectional Study of Data Linkage and Artificial Intelligence Practices Across European Countries. Arch Public Health (2020) 78:55. doi: 10.1186/s13690-020-00436-9
Keywords: bone mass, monogenic bone disorders, gene variants, functional validation, drug discovery, GEMSTONE, skeletal dysplasia
Citation: Formosa MM, Bergen DJM, Gregson CL, Maurizi A, Kämpe A, Garcia-Giralt N, Zhou W, Grinberg D, Ovejero Crespo D, Zillikens MC, Williams GR, Bassett JHD, Brandi ML, Sangiorgi L, Balcells S, Högler W, Van Hul W and Mäkitie O (2021) A Roadmap to Gene Discoveries and Novel Therapies in Monogenic Low and High Bone Mass Disorders. Front. Endocrinol. 12:709711. doi: 10.3389/fendo.2021.709711
Received: 14 May 2021; Accepted: 12 July 2021;
Published: 13 August 2021.
Edited by:
Gudrun Stenbeck, Brunel University London, United KingdomReviewed by:
Mark Johnson, University of Missouri–Kansas City, United StatesCopyright © 2021 Formosa, Bergen, Gregson, Maurizi, Kämpe, Garcia-Giralt, Zhou, Grinberg, Ovejero Crespo, Zillikens, Williams, Bassett, Brandi, Sangiorgi, Balcells, Högler, Van Hul and Mäkitie. This is an open-access article distributed under the terms of the Creative Commons Attribution License (CC BY). The use, distribution or reproduction in other forums is permitted, provided the original author(s) and the copyright owner(s) are credited and that the original publication in this journal is cited, in accordance with accepted academic practice. No use, distribution or reproduction is permitted which does not comply with these terms.
*Correspondence: Outi Mäkitie, b3V0aS5tYWtpdGllQGhlbHNpbmtpLmZp
Disclaimer: All claims expressed in this article are solely those of the authors and do not necessarily represent those of their affiliated organizations, or those of the publisher, the editors and the reviewers. Any product that may be evaluated in this article or claim that may be made by its manufacturer is not guaranteed or endorsed by the publisher.
Research integrity at Frontiers
Learn more about the work of our research integrity team to safeguard the quality of each article we publish.