- 1Department of Pathology, Immunology and Laboratory Medicine, College of Medicine, University of Florida, Gainesville, FL, United States
- 2Department of Medical Science and Institute of Biotechnology, National Tsing Hua University, Hsinchu, Taiwan
Although first described over a hundred years ago, tissue optical clearing is undergoing renewed interest due to numerous advances in optical clearing methods, microscopy systems, and three-dimensional (3-D) image analysis programs. These advances are advantageous for intact mouse tissues or pieces of human tissues because samples sized several millimeters can be studied. Optical clearing methods are particularly useful for studies of the neuroanatomy of the central and peripheral nervous systems and tissue vasculature or lymphatic system. Using examples from solvent- and aqueous-based optical clearing methods, the mouse and human pancreatic structures and networks will be reviewed in 3-D for neuro-insular complexes, parasympathetic ganglia, and adipocyte infiltration as well as lymphatics in diabetes. Optical clearing with multiplex immunofluorescence microscopy provides new opportunities to examine the role of the nervous and circulatory systems in pancreatic and islet functions by defining their neurovascular anatomy in health and diabetes.
Introduction
Goals
Heterogeneity of the human pancreas is well accepted in terms of islet endocrine cell proportions and mass in healthy people and for lobularity in islet beta-cell losses and frequency of infiltrated islets in patients in type 1 diabetes (T1D) (1–7). Patients with type 2 diabetes (T2D) show similar heterogeneity in islet amyloidosis, fatty infiltration, fibrosis and inflammatory infiltrates (4, 8–10). Morphology-based studies of the human pancreas have been key to understanding regional heterogeneity yet examinations of the pancreas in its natural three-dimensional (3-D) configuration have been limited to laborious serial sectioning with subsequent reconstruction. Islets occupy only ~2% of the entire pancreas volume and sampling of multiple blocks is recommended to maximize islet analyzes by 2-D microscopy (11). Recent applications of optical clearing methods to the human and mouse pancreas provide new details for structure-function relationships in health and subsequent abnormalities in diabetes (12–15). This review provides an overview of recent optical clearing methods used in human and mouse pancreas studies and examples of pancreas optical clearing to define several components of the pancreas endocrine and exocrine compartments.
Techniques
Although basic optical clearing to render tissues transparent was first described by the German anatomist Walter Spalteholz over 100 years ago, the recent decade has seen a rapid growth in advanced clearing methods applicable for whole body or organ imaging to single cell resolution (16, 17). Optical clearing is readily accomplished in a standard laboratory and new procedures can be found nearly weekly in the literature for different organs and species (Table 1). Optical clearing methods are based on obtaining a high degree of tissue transparency and matching of the sample refractive index (RI) to that of the imaging media to remove light scattering (54). Most tissues are comprised of ~80% water (RI=1.33), 10% proteins (RI>1.44), and 10% lipids (RI>1.45) (55). Methods are broadly based on physical or chemical strategies with organic solvents or aqueous solutions used for the latter. Several excellent reviews are available that detail each optical clearing method advantages and disadvantages (45, 56–59). Early optical clearing methods used organic chemicals [e.g., benzyl alcohol–methyl salicylate, benzyl alcohol–benzyl benzoate (BABB), and solvents used in 3-D imaging of solvent-cleared organs (3DISCO)] (27). Generally these methods achieve high transparency within a few days by removing lipids and homogenizing refractive indices (RIs) of the samples, and they are compatible with whole-mount immunolabeling (48, 60). Solvent based clearing methods may use toxic chemicals and steps should be performed using a fume hood and suitable personal protective equipment. Recent methods have been named with acronyms such as immunolabeling-enabled imaging of solvent-cleared organs (iDISCO) (60), clear, unobstructed brain/body imaging cocktails and computational analysis (CUBIC) (17, 27, 61, 62), clear lipid-exchanged acrylamide-hybridized rigid imaging/immunostaining/in situ hybridization-compatible tissue hydrogel (CLARITY), and passive CLARITY technique (PACT) (38, 63–65).
The original CLARITY manuscript by Chung et al. (65) described four key steps: (1) hydrogel tissue embedding using a ratio of 4% acrylamide monomer to 0.05% bis-acrylamide followed by polymerization; (2) clearing secondary to lipid removal using 4% sodium dodecyl sulfate (SDS) detergent buffer within a custom built electrophoretic tissue clearing system (ETC); (3) immunostaining; and (4) tissue RI matching and imaging. Several modifications were subsequently published in favor of “passive clearing” without the use of ETC to avoid oxidative tissue artifacts with several variations in the amount of paraformaldehyde (0-4%), acrylamide monomer (1-4%) and bis-acrylamide in the hydrogel mixture depending on target organ (63, 66, 67).
Despite their differences in chemical and/or optical properties, the organic solvent, aqueous reagent, and electrophoresis-assisted clearing methods all extend our view of neurovascular networks (>>100 µm) with 3-D microscopy compared with the images acquired from classic IHC and H&E histology (3-5 µm in thickness). To apply tissue clearing, the key question is whether a specific clearing technique changes the sample chemical and/or cellular environment that causes artifacts in signal detection. For example, in CLARITY, the use of sodium dodecyl sulfate (SDS) treatment and electrophoresis to remove cellular membranes is likely to disturb membrane receptor proteins. Thus, studies of the nerve-receptor association in space will be better accepted if a passive aqueous-based clearing method (e.g., sugar reagent) is employed compared with CLARITY. Likewise, leukocytes and their vascular receptor association in space will be better examined in an aqueous environment, because disturbing the membranes for 3-D imaging may create false negative results in signal detection. However, we need to stress that the false negative result may also come from scattering in deep-tissue imaging. Thus, adding a positive control in the specimen (e.g., nuclear staining) can help investigators monitor the resolving power (e.g., resolving two adjacent nuclei in an islet) across the optical depth in deep-tissue pancreatic and islet imaging.
Volumetric microscopy has also paralleled optical clearing methods with advancements in confocal, multiphoton and lightsheet microscopes. Advantages of the lightsheet microscopes are faster scanning speeds, reduced photobleaching, and good resolution at high tissue penetration depths. When access to lightsheet microscopes is not feasible, confocal microscopy provides an excellent alternative with single-cell resolution. While any type of fluorescent microscope can be used to image optically-cleared tissue, the microscope imaging chamber must be able to accommodate the size of the sample and the stage configuration and imaging depth are dependent on the working distance of the objective. Most images can be obtained with regular air objectives between ×2 and ×20 magnifications. Specialized objectives for optically cleared samples over larger distances are available from multiple vendors such as Olympus and Zeiss. Users of optical clearing methods and advanced microscopy need also consider data storage requirements for both image acquisition and analysis. Additional storage space for the imaging microscope is required as well as additional memory and processing speed for image analysis workstations. The opensource software Fiji/ImageJ can be used for 2-D stitching and basic 3-D adjustments (68). Zeiss Zen software will also provide stitching and maximum intensity projections. Commercial software packages are available for advanced 3-D volume rendering and reconstruction such as Neurolucida360/Vesselucida (MBF), Arivis (FEI), and Imaris (Bitplane, Concord MA). Consideration of sample size and existing microscopes and image analysis software may thus dictate which optical clearing method is most suitable for a given laboratory. For those without sufficient local resources, the growing popularity of optical clearing methods generated several commercial sources services for clearing, microscopy, and analysis services (Visikol, ClearLight, LifeCanvas).
Challenges
Challenges related to optical clearing are relatively few. Acquisition of high-quality samples is important as for any down-stream application based on fixed samples. Fixation with 4% paraformaldehyde or 10% formalin is commonly employed in many laboratories. Cardiac perfusion is recommended for rodent studies in most part to remove red blood cells and their inherent high autofluorescence. However, immersion fixation is a reasonable alternative for rodents and the only method available for human biosamples. As for traditional immunolocalization, the duration of fixation is ideally kept to a minimum to avoid over-fixation of tissue antigens. Many primary antibodies utilized for formalin-fixed paraffin embedded samples work well for optical clearing and those tested in our laboratory are provided as a reference (Table 2). A pre-testing step is advised for new primary antibodies and can be accomplished with fixed frozen thick sections (40µm) utilizing similar conditions as those for permeabilization before immunostaining and optical clearing. Wide applicability of primary antibodies remains an issue, particularly for immune markers. While some methods have successfully employed preconjugated primary antibodies (43), the majority of optical clearing methods employ standard rounds of primary antibodies followed by secondary antibodies to promote antibody penetration. A reported benefit of CLARITY was the ability to reiteratively strip antibodies and reprobe a sample several times (65). In practice, we have been unsuccessful in fully stripping samples from human pancreas cleared using CLARITY and also found limited antigenicity and/or diffusion of subsequent primary antibodies (Campbell-Thompson, unpublished results). As such, our more recent studies employ single clearing methods with multiplex immunostaining on 500µm sections rather than several mm sized pieces to extend use of a given sample, particularly those from rare organ donors with diabetes.
Equipment utilized in optical clearing is generally found in any modern molecular pathology laboratory and include access to fume hoods, refrigeration or ovens, rocker plates, and other ancillary small equipment for immunostaining and clearing steps. Microscope and image analysis software are two aspects to be considered before conducting clearing studies as this will influence sample size and numbers of channels for multiplex staining and analysis. As costs for multiphoton and lightsheet microscopes decrease, access to these microscopes will increase whether through institutional shared resources, subcontract, or collaboration. Image analysis expertise is also limited and laboratory staff become proficient in the software available to them. Expense of commercial 3-D software analysis programs is high. Finally, the greatest hurdle may be the large image file sizes achieved by these methods. Here too, limiting the number of antigens needs to be balanced with the rarity of the sample since reiterative staining is difficult.
Optical Clearing Examples
This introduction on optical clearing and imaging provides a basic starting point for studies on human and mouse pancreas in health and diabetes. We will now show examples of application of different clearing methods to demonstrate the versatility of optical clearing in human and mouse pancreas for determination of normal states and changes found with diabetes.
Sample Processing
Human pancreata not suitable for clinical purposes were collected from nondiabetic, brain-dead organ donors after written informed consent from legal representative or next of kin and were processed by the Network for Pancreatic Organ donors with Diabetes (nPOD) program at the University of Florida (UF) Diabetes Institute using methods previously reported (69). The nPOD samples used in this specific study were approved as nonhuman by the UF IRB (IRB201902530). Collection and use of human pancreatectomy specimens were approved by the Institutional Review Board of National Taiwan University Hospital (201703131RIND). All UF animal studies were conducted using published guidelines and regulations of the National Institutes of Health for the care and use of laboratory animals. The protocol was approved by the UF Institutional Animal Care and Use Committee (IACUC 202009976). All animal studies conducted at the National Tsing Hua University were reviewed and approved by the institutional animal use review board.
Pancreatic Islet Schwann Cells
Passive CLARITY (PACT) provides for good tissue transparency and multiplex immunolabeling is quite feasible with image analysis such as using the open software Neurite tracer program in ImageJ (Figure 1) (15). Schwann cells are the peripheral counterpart to central nervous system oligodendrocytes and provide support to both myelinated and unmyelinated axons of motor and sensory neurons (70, 71). In addition to providing physical support, nonmyelinating Schwann cells are essential for maintenance and regeneration of damaged axons by production of neurotrophins and acting as “first responders” to injury (72, 73). Unlike the dense mesh-like network formed by islet Schwann cells in mouse islets, Schwann cells provide support for autonomic nerves in human islets in a loose formation (Figure 2) (70). Schwann cells are also of particular interest in T1D as they have a role in antigen presentation, interact with the complement system, and secrete factors involved in immune interactions (74, 75) and animal studies report reactive Schwann cells in diabetes and islet injury (23, 76–78). Furthermore, glial fibrillary acidic protein (GFAP) is expressed in peri-islet Schwann cells and is reported to be an autoantigen for T1D with potential use as a biomarker (79).
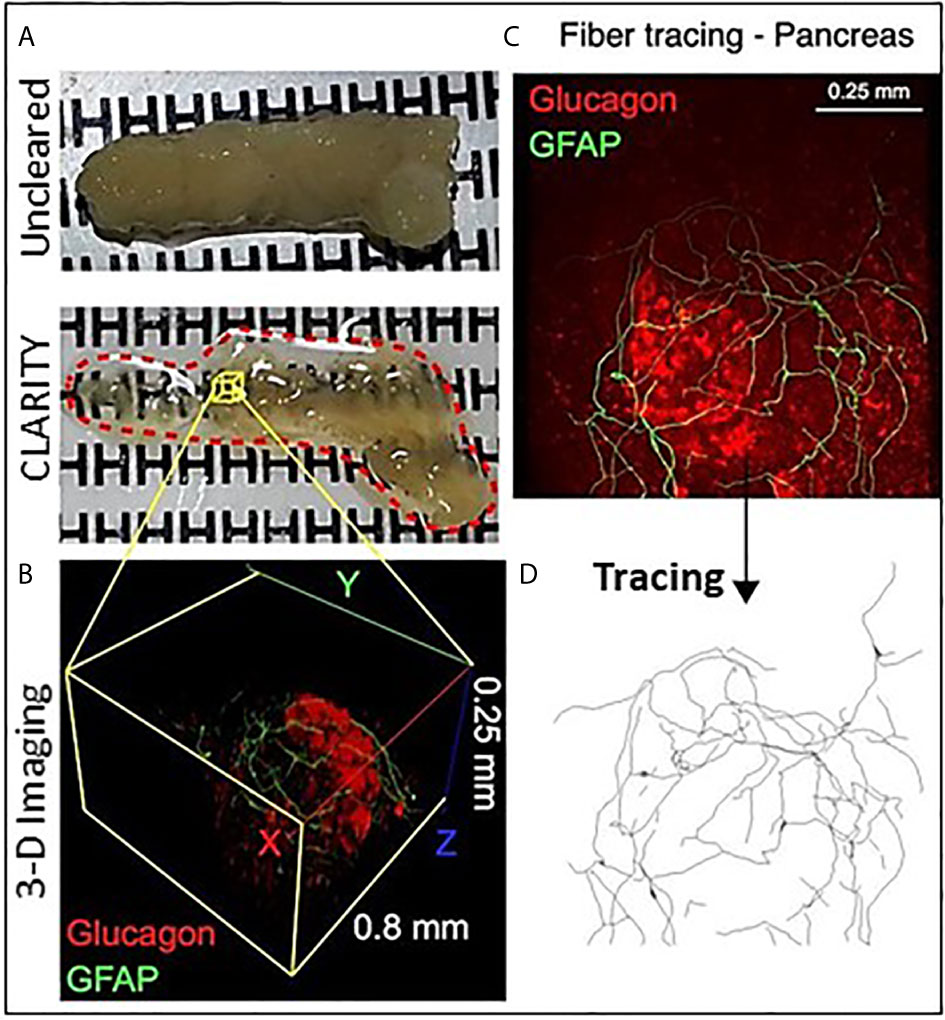
Figure 1 Optical clearing of human pancreas by PACT and iDISCO. (A) Fixed pancreas sample from a control donor before and after clearing using passive CLARITY (PACT) showing the degree of sample transparency achieved with this method. (B) Representative example of 2-photon imaging for a 0.8 mm x 0.8 mm x 0.25 mm region (X, Y, Z axes) containing an islet immunostained for glucagon (red) and glial fibrillary acidic protein (GFAP, green). (C) GFAP-stained Schwann cells overlay an islet and cell projects were analyzed using the ImageJ neurite tracer program. (D) The traced Schwann cells are shown by Neurite tracer skeleton diagram.
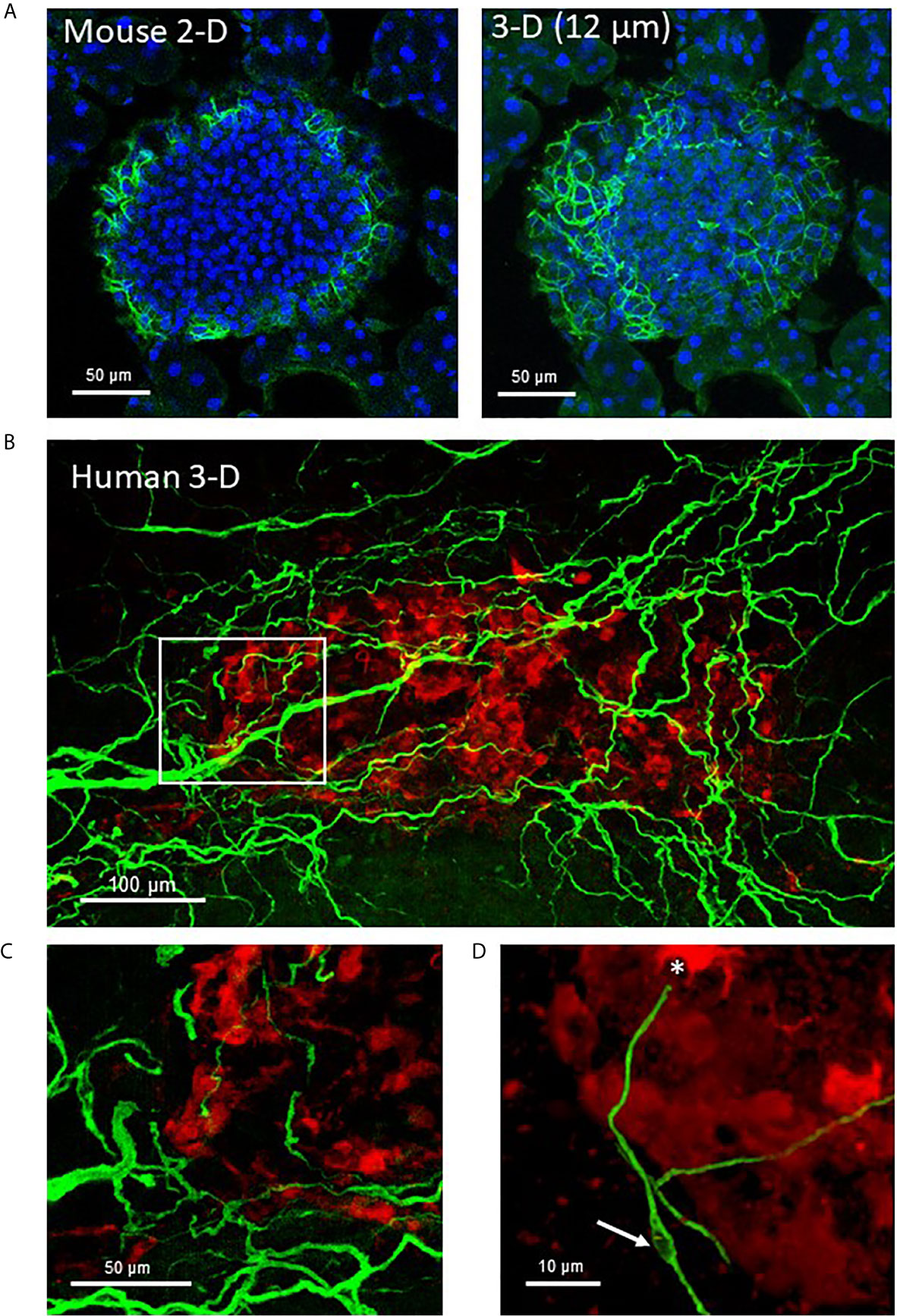
Figure 2 Mouse and human islet Schwann cells. (A) A fixed frozen section (40µm) from a C57BL/6 mouse pancreas was stained for GFAP (green) using whole mount staining. A single 2-D slice (7th of 13 slices) and the 3-D maximum intensity projection (MIP, 12 µm stack) are shown to demonstrate the increase in cellular information obtained with a z-stack. (B) A human control pancreas sample (~1 mm3) was cleared by iDISCO and immunolabeling with glucagon (red) and GFAP (green) before confocal 3-D imaging (maximum intensity projection 50 µm). (C) The region identified by white box in (A) shows Schwann cells at the periphery of the islet that extended along nerves to islet interiors traveling along afferent vessels. (D) A single Schwann cell shows a clear nuclear region (white arrow) and numerous extensions with a termination at an alpha-cell (asterisk). See also Supplementary Video 1 for (A).
Pancreatic Ganglia and Neuroinsular Complexes
Intrapancreatic ganglia represent the post-ganglionic neurons of the parasympathetic efferent network (80). They are widely distributed and in relatively low density throughout the human and rodent pancreas and thus optical clearing and 3-D imaging provides a greater opportunity to detect these ganglia (13). At low magnifications, interconnections of intrapancreatic ganglia and to islets are visualized in a human pancreas cleared using PACT (15) (Figures 3A, B). Such interconnections likely contribute to the synchronization of islet hormone secretions particularly during the cephalic phase of digestion (81). The intrapancreatic ganglia vary in numbers of neurons and also observed are small clusters of neurons (Figure 3C) or small clusters of neurons with islet β-cells and α-cells can also be observed, so-called neuroinsular complex type II (Figure 3D) (82). These type II structures have not been previously reported in adult human pancreas and were found only in fetal pancreas. The lack of detection in adults could be due to limitations of 2-D microscopy in finding small structures compared to 3-D microscopy as demonstrated here (83).
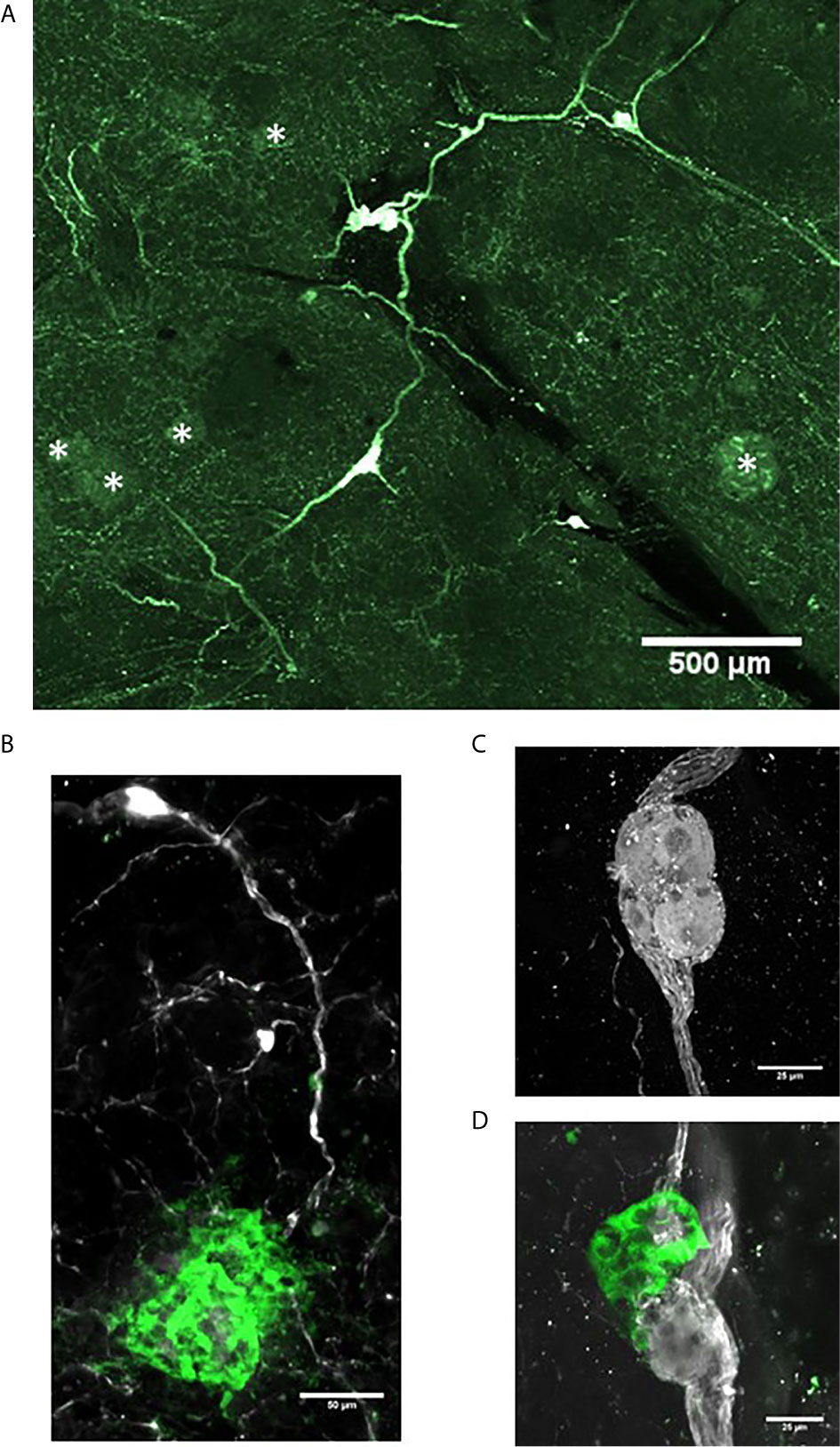
Figure 3 Intrapancreatic ganglia and neuroinsular complexes in adult human pancreas. Pancreas samples were studied in control donors using passive CLARITY (PACT) and immunostaining with primary antibodies for PGP9.5 (white) and GFAP (green) to delineate nerve fibers and supporting Schwann cells, respectively (Table 2). PGP9.5 also stained islet endocrine cells although with much less intensity (asterisks). Intrapancreatic ganglia represent post-ganglionic neurons and fibers of the parasympathetic efferent system and 3-D imaging shows how ganglia are interconnected (A) and also extend to islets (B). (C) Intrapancreatic ganglia contained varying numbers of neurons and small collections of neurons were also found widely scattered with efferent and afferent axons in both interlobular and intralobular regions. (D) Imaging for PGP9.5 and islet alpha-cells (GCG, green) demonstrate close association of clustered alpha-cells with neurons at a small ganglion, also known as a neuro-insular complex II. Scale bars: 500µm (A), 50µm (B), 25 µm (C, D). See also Supplementary Video 2 for (D).
Pancreatic Vasculature
The pancreatic vasculature in health and diabetes has been studied with newer studies showing detection of the Sars-CoV-2 receptor, ACE2, in pancreatic micovasculature, rather than islet endocrine cells, adding additional importance to understanding factors regulating islet blood flow in health and diabetes (84–87). The use of optical clearing provides an unprecedented opportunity to better examine structural-functional relationships of the islet microvasculature in the context of islet heterogeneity and inter-relationship to the surrounding acinar cells. Studies performed in rodents can be achieved by perfusion with fluorescent compounds including lectins or conjugated primary antibodies such as CD31. For human samples, the vasculature can be readily labeled using CD31 or CD34 followed by multiplex immunofluorescence with islet endocrine cell markers and the high vascular density can be appreciated throughout 3-D microscopy (Figure 4) (53).
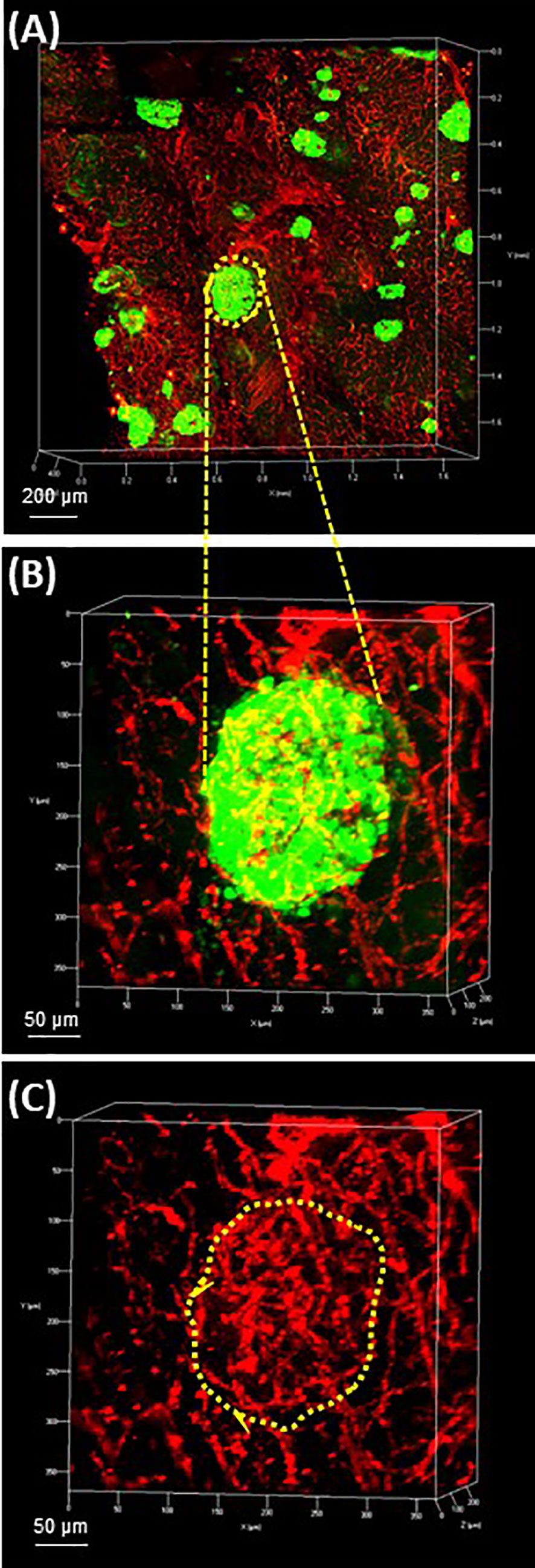
Figure 4 Human pancreas vasculature. 3-D extended projection of human pancreas exocrine and endocrine vasculature are shown with X, Y and Z axes in mm (Scale bar 200 µm). (A) The extensive nature of the human pancreas vascular system is demonstrated by immunolabeling with monoclonal anti-CD31 (red) and islets are shown stained with monoclonal anti-glucagon (green) antibodies. (B) An islet identified by yellow-dotted line in (A) is shown at higher resolution in (B) (Scale bar 50 µm). (C) The islet microvasculature is shown without the glucagon overlay (Scale bar 50 µm). See also Supplementary Video 3 for (A–C).
Pancreatic Acinar Ductal Metaplasia
Pancreatic cancer is one of the deadliest tumors and seminal studies showed that early lesions likely arise from acinar-ductal metaplasia forming so-called pancreatic intraductal neoplasia (PanIN) (88). Optical clearing studies in human and mice have shown characteristics of ductal lesions through 3-D imaging (31, 50). Cell culture models of human pancreatic cancer can also benefit from use of optical clearing and 3-D microscopy. Single cell details are apparent in an in vitro human primary acinar culture model showing duct formation following optical clearing using HISTO-M, a commercial product similar to iDISCO clearing (Figure 5) (Visikol) (T. Schmittgen, personal communication) (89).
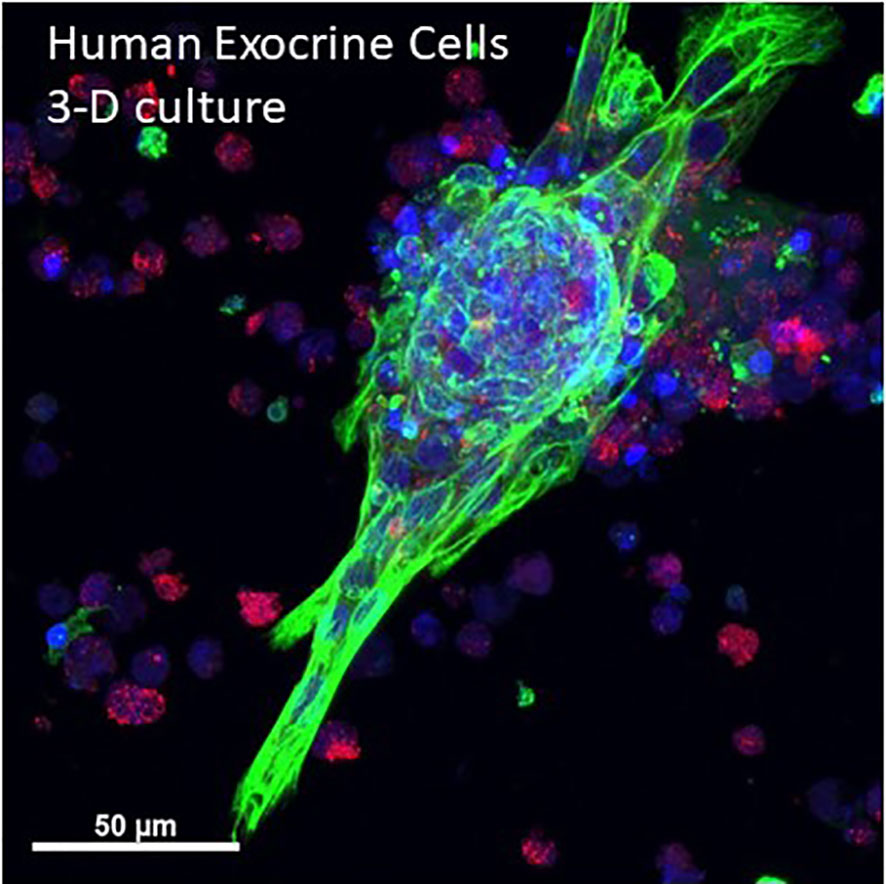
Figure 5 3-D projection human primary pancreas exocrine cells in culture. Isolated human exocrine cells were obtained from a pancreas donor non-islet fractions and following filtration to remove islets and clumps, exocrine cells were plated in 1:1 DMEM:F12 and Matrigel and grown for 6 days. A maximum projection image shows ductal cells (cytokeratin 19, green), acinar cells (amylase, red) and nuclei (blue). Cells were kindly provided by Dr. Thomas Schmittgen, College of Pharmacy, University of Florida. See also Supplementary Video 4 for entire 3-D z-stack (12 µm).
Pancreatic Fatty Infiltration
Unlike the fatty liver, in which lipid droplets accumulate in the cytoplasm in the hepatocytes, pancreatic fatty infiltration involves the fat cells (adipocytes) ectopically developing and accumulating in and around the pancreatic lobules alongside the exocrine and endocrine tissues. The fat content in the pancreas increases with age (90) and is detectable as early as in adolescence (91), and the degree is linked with obesity (92–94). In the progression from obesity to type 2 diabetes, the state of hyperinsulinemia is likely to accelerate the pancreatic fat accumulation due to the organ’s high insulin concentration. Insulin is a potent factor to induce adipogenesis, in which preadipocytes (e.g., fibroblasts and myofibroblasts in the pancreatic stroma) differentiate into adipocytes (95, 96), and stimulate the proliferation of adipocytes (97). Thus, it is not surprising that multiple studies documented the correlation between type 2 diabetes and pancreatic fats and implicated the negative influence of these fats on the islet microenvironment and function (98–100).
Clinically, magnetic resonance imaging (MRI) and computed tomography (CT) are the preferred imaging modalities to detect and quantify the pancreatic fats (90–92, 94, 98–102). While these two methods provide valuable in vivo information for cross-sectional or longitudinal studies, they cannot resolve the cellular structures of fats and the pancreatic exocrine and endocrine tissues. At the cellular level, the classic microtome-based histology with H&E staining can identify the adipocytes and their association with blood vessels, acini, ducts, and islets with µm-level resolution. However, due to the hydrophobicity of fats and their weak mechanical connection with the pancreatic lobules, both microtome slicing and dewaxing (xylene wash) of the paraffin-embedded pancreas may create artifacts on the locations of adipocytes, affecting the analysis of the peri- and/or intra-lobular adipocyte association.
Modern 3-D histology with aqueous-based optical clearing alleviates the abovementioned technical concern by maintaining the native hydrophilicity of the tissue and avoiding the microtome slicing in sample preparation (42). This is particularly important in examination of the type 2 diabetic pancreas and the surgical biopsy of pancreatic cancer [patient may have developed type 3c diabetes (103, 104)]. In both situations, investigators will likely encounter moderate-to-severe fatty infiltration, in which adipocytes generally or locally become a component of the pancreas (Figure 6). To investigate the pancreas in this condition, we advise careful comparison between the modern 3-D and the classic 2-D tissue images to confirm the adipocytes in and around the remodeled pancreatic lobules to avoid misrepresentation of the disease condition.
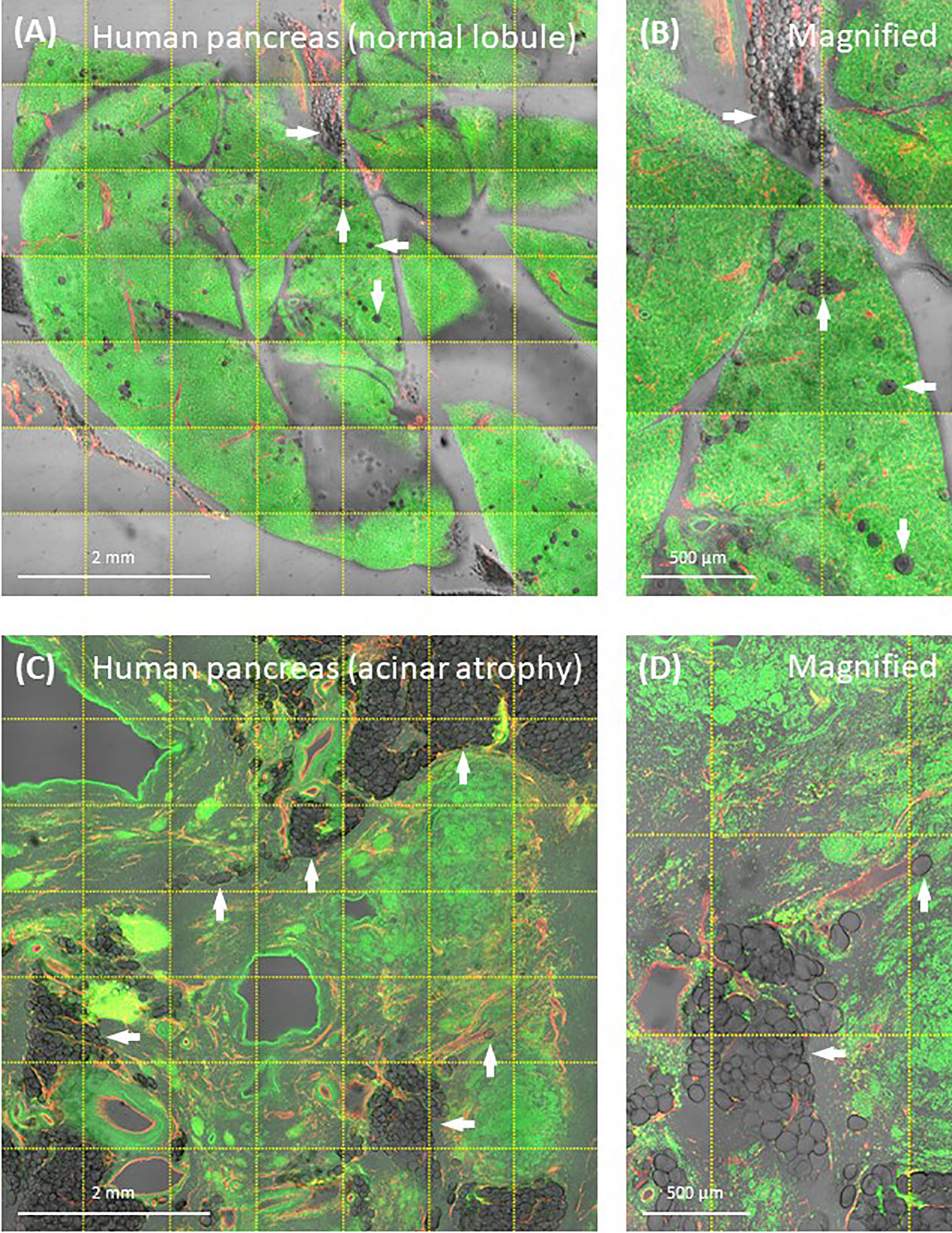
Figure 6 Human pancreatic fatty infiltration. Images were derived from tile scanning of optically cleared pancreatic specimens. (A, B) Normal lobule of human pancreas. Adipocytes are clearly seen around the blood vessel and inside the lobule (magnified, arrows). Green, nuclear staining; red, CD31. (C, D) Acinar atrophy of diseased lobule. This view was acquired 2-cm distal to the pancreatic ductal adenocarcinoma. Overlay of transmitted light and fluorescence signals identifies the fatty infiltration.
Pancreatic Lymphatic Network
The lymphatic drainage of pancreas is achieved by an intricate network of lymphatic vessels and nodes, in which the immune cells reside. The open-ended lymphatic network collects the interstitial fluids for water and lipid absorption and recycling (which balances the tissue osmatic pressure) and for immune surveillance (105). When pancreatic injury or disease occurs, the lymphatic system plays a central role in reaction to the exocrine [pancreatitis (44, 106)] and endocrine tissue inflammation. For example, in the nonobese diabetic (NOD) mice, the progression of type 1 diabetes features the migration of T lymphocytes from the circulatory system to the islet, attacking the β-cells (107, 108). In the process, the microtome-based 2-D histology has been used to evaluate the degree of islet inflammation, in which early, moderate, and severe insulitis are assigned to evaluate the progression of the disease (109). However, due to the dispersed nature of lymphatic vessels, the classic 2-D histology cannot provide a global assessment of lymphatic endothelial remodeling in response to insulitis. To understand the associated lymphatic and immune response to insulitis, panoramic and in-depth imaging of the pancreas is needed to characterize the lymphatic network and T-cell migration in a global and integrated fashion.
As can be seen in Figure 7, the optically cleared NOD mouse pancreas provides an experimental setting to investigate the lymphatic and T-lymphocyte association in insulitis. Using the panoramic image (Figure 7A), we can detect the CD3+ T lymphocytes in the lymph node (positive control) and around the islets. The latter provides a clear target for examination of the islet under immune attack, featuring vasodilation and the packing of T lymphocytes in the lymphatic vessels (Figures 7B, C). Overall, the quadruple signals of tissue microstructure (nuclear staining), vasculature (blood and lymphatic vessels), and CD3+ T lymphocytes in the transparent pancreas provide an optimized condition to visualize the islet vascular remodeling and immune attack in insulitis. They also demonstrate the different scales of tissue information (from interlobular to subcellular features of islets under immune attack) that can be acquired from optically cleared pancreas samples to investigate experimental insulitis.
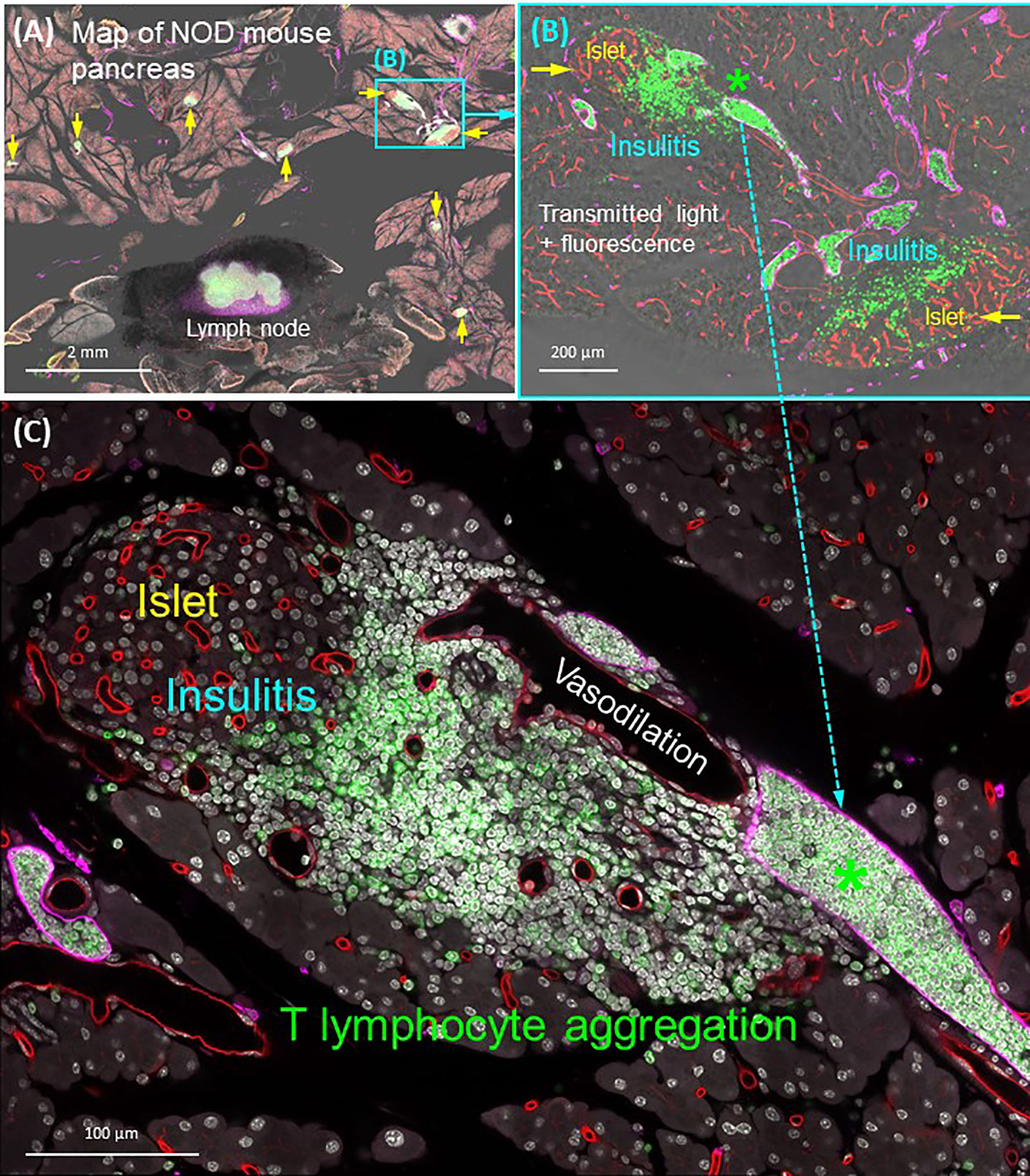
Figure 7 Panoramic and high-resolution images of optically cleared NOD mouse pancreas with insulitis. (A) Map of 8-week NOD mouse pancreas. Overlay of transmitted light and fluorescence signals identifies the Lyve1+ lymph node (filled with CD3+ T lymphocytes and surrounded by fats) and locations of insulitic islets (yellow arrows). Two islets (cyan box) are magnified in (B). (B) Islets with insulitis are shown with blood vessels (red), lymphatic vessels (magenta), and nuclei (white). CD3+ T lymphocytes are identified around the islets and congregated in the lymphatic vessels (asterisk; vascular compartment vs. extravascular domain). This feature is further magnified in (C). (C) Peri-islet aggregation of T lymphocytes and their vascular association shown as well as the peri-islet vasodilation and lymphocytic infiltration. See also Supplementary Video 5 for (B).
Conclusions
Both standard and modified optical clearing methods are well suited for studies of 3-D structure-function relationships for human and mouse pancreas and use readily available chemicals and imaging equipment. These methods are particularly advantageous for studies of diabetes due to known islet heterogeneity requiring examination of numerous islets and pancreas regions. Optical clearing methods can also be used in investigations of pancreatic cancer using patient or rodent samples or in vitro experiments examining acinar-ductal metaplasia. Advances in understanding failure of islet beta-cells in diabetes requires a wholistic examination of islets in their native environment as afforded by optical clearing and new findings are anticipated related to the role of the nervous, immune, and vascular systems in beta-cell biology from such studies.
Author Contributions
MC-T and S-CT designed the studies, performed experiments, prepared figures, and edited and revised the manuscript. All authors contributed to the article and approved the submitted version.
Funding
Funding provided by NIH 1R01DK122160, UC4 DK104155, U54 DK127823 and OT2 OD023861, Helmsley Charitable Trust 2015PG-T1D052 and JDRF 47-2014-1 and 2-SRA-2019-697-S-B to MC-T and Taiwan National Health Research Institutes (NHRI-EX109-10922EI) and Ministry of Science and Technology (MOST 108-2314-B-007-006-MY2) to S-CT.
Conflict of Interest
The authors declare that the research was conducted in the absence of any commercial or financial relationships that could be construed as a potential conflict of interest.
Acknowledgments
The authors thank past and current members of their laboratories for their assistance in optical clearing and microscopy. We thank the organ donor families for research organ donations. Organ Procurement Organizations (OPO) partnering with nPOD to provide research resources are listed at http://www.jdrfnpod.org/for-partners/npod-partners/. Human sample research was supported by nPOD (RRID : SCR_014641), a collaborative type 1 diabetes research project sponsored by JDRF (5-SRA-2018-557-Q-R) and the Leona M. & Harry B. Helmsley Charitable Trust (2018PG-T1D053). Imaging with the Zeiss 710 was performed with support from the UF Center for Immunology and Transplantation. Imaging with the Zeiss 800 was performed with support from Taiwan Ministry of Science and Technology (MOST 108-2731-M-007-001).
Supplementary Material
The Supplementary Material for this article can be found online at: https://www.frontiersin.org/articles/10.3389/fendo.2021.644826/full#supplementary-material
References
1. Campbell-Thompson M, Fu A, Kaddis JS, Wasserfall C, Schatz DA, Pugliese A, et al. Insulitis and β-Cell Mass in the Natural History of Type 1 Diabetes. Diabetes (2016) 65(3):719–31. doi: 10.2337/db15-0779
2. Dybala MP, Hara M. Heterogeneity of the Human Pancreatic Islet. Diabetes (2019) 68(6):1230–9. doi: 10.2337/db19-0072
3. Benninger RKP, Dorrell C, Hodson DJ, Rutter GA. The Impact of Pancreatic Beta Cell Heterogeneity on Type 1 Diabetes Pathogenesis. Curr Diabetes Rep (2018) 18(11):112. doi: 10.1007/s11892-018-1085-2
4. Nasteska D, Hodson DJ. The Role of Beta Cell Heterogeneity in Islet Function and Insulin Release. J Mol Endocrinol (2018) 61(1):R43–60. doi: 10.1530/JME-18-0011
5. Pipeleers D, De Mesmaeker I, Robert T, Van Hulle F. Heterogeneity in the Beta-Cell Population: A Guided Search Into its Significance in Pancreas and in Implants. Curr Diabetes Rep (2017) 17(10):86. doi: 10.1007/s11892-017-0925-9
6. Dorrell C, Schug J, Canaday PS, Russ HA, Tarlow BD, Grompe MT, et al. Human Islets Contain Four Distinct Subtypes of β Cells. Nat Commun (2016) 7:11756. doi: 10.1038/ncomms11756
7. Roscioni SS, Migliorini A, Gegg M, Lickert H. Impact of Islet Architecture on β-Cell Heterogeneity, Plasticity and Function. Nat Rev Endocrinol (2016) 12(12):695–709. doi: 10.1038/nrendo.2016.147
8. Hunter CS, Stein RW. Evidence for Loss in Identity, De-Differentiation, and Trans-Differentiation of Islet β-Cells in Type 2 Diabetes. Front Genet (2017) 8:35. doi: 10.3389/fgene.2017.00035
9. Aguayo-Mazzucato C, van Haaren M, Mruk M, Lee TB, Crawford C, Hollister-Lock J, et al. β Cell Aging Markers Have Heterogeneous Distribution and Are Induced by Insulin Resistance. Cell Metab (2017) 25(4):898–910.e5. doi: 10.1016/j.cmet.2017.03.015
10. Garcia TS, Rech TH, Leitão CB. Pancreatic Size and Fat Content in Diabetes: A Systematic Review and Meta-Analysis of Imaging Studies. PloS One (2017) 12(7):e0180911. doi: 10.1371/journal.pone.0180911
11. Poudel A, Fowler JL, Zielinski MC, Kilimnik G, Hara M. Stereological Analyses of the Whole Human Pancreas. Sci Rep (2016) 6:34049. doi: 10.1038/srep34049
12. Alvarsson A, Jimenez-Gonzalez M, Li R, Rosselot C, Tzavaras N, Wu Z, et al. A 3D Atlas of the Dynamic and Regional Variation of Pancreatic Innervation in Diabetes. Sci Adv (2020) 6(41):eaaz9124. doi: 10.1126/sciadv.aaz9124
13. Chien HJ, Chiang TC, Peng SJ, Chung MH, Chou YH, Lee CY, et al. Human Pancreatic Afferent and Efferent Nerves: Mapping and 3-D Illustration of Exocrine, Endocrine, and Adipose Innervation. Am J Physiol Gastrointest Liver Physiol (2019) 317(5):G694–706. doi: 10.1152/ajpgi.00116.2019
14. Tang SC, Jessup CF, Campbell-Thompson M. The Role of Accessory Cells in Islet Homeostasis. Curr Diabetes Rep (2018) 18(11):117. doi: 10.1007/s11892-018-1096-z
15. Butterworth E, Dickerson W, Vijay V, Weitzel K, Cooper J, Atkinson EW, et al. High Resolution 3d Imaging of the Human Pancreas Neuro-insular Network. J Vis Exp (2018) 131:56859–66. doi: 10.3791/56859
16. Azaripour A, Lagerweij T, Scharfbillig C, Jadczak AE, Willershausen B, Van Noorden CJ. A Survey of Clearing Techniques for 3D Imaging of Tissues With Special Reference to Connective Tissue. Prog Histochem Cytochem (2016) 51(2):9–23. doi: 10.1016/j.proghi.2016.04.001
17. Susaki EA, Shimizu C, Kuno A, Tainaka K, Li X, Nishi K, et al. Versatile Whole-Organ/Body Staining and Imaging Based on Electrolyte-Gel Properties of Biological Tissues. Nat Commun (2020) 11(1):1982. doi: 10.1038/s41467-020-15906-5
18. Kim A, Kilimnik G, Hara M. In Situ Quantification of Pancreatic Beta-Cell Mass in Mice. J Vis Exp (2010) 40:1970–3. doi: 10.3791/1970
19. Fu YY, Tang SC. At the Movies: 3-Dimensional Technology and Gastrointestinal Histology. Gastroenterology (2010) 139(4):1100–5, 5.e1. doi: 10.1053/j.gastro.2010.08.025
20. Fu YY, Lu CH, Lin CW, Juang JH, Enikolopov G, Sibley E, et al. Three-Dimensional Optical Method for Integrated Visualization of Mouse Islet Microstructure and Vascular Network With Subcellular-Level Resolution. J BioMed Opt (2010) 15(4):046018. doi: 10.1117/1.3470241
21. Li WC, Rukstalis JM, Nishimura W, Tchipashvili V, Habener JF, Sharma A, et al. Activation of Pancreatic-Duct-Derived Progenitor Cells During Pancreas Regeneration in Adult Rats. J Cell Sci (2010) 123(Pt 16):2792–802. doi: 10.1242/jcs.065268
22. Chiu YC, Hua TE, Fu YY, Pasricha PJ, Tang SC. 3-D Imaging and Illustration of the Perfusive Mouse Islet Sympathetic Innervation and its Remodelling in Injury. Diabetologia (2012) 55(12):3252–61. doi: 10.1007/s00125-012-2699-6
23. Tang SC, Chiu YC, Hsu CT, Peng SJ, Fu YY. Plasticity of Schwann Cells and Pericytes in Response to Islet Injury in Mice. Diabetologia (2013) 56(11):2424–34. doi: 10.1007/s00125-013-2977-y
24. Juang JH, Peng SJ, Kuo CH, Tang SC. Three-Dimensional Islet Graft Histology: Panoramic Imaging of Neural Plasticity in Sympathetic Reinnervation of Transplanted Islets Under the Kidney Capsule. Am J Physiol Endocrinol Metab (2014) 306(5):E559–70. doi: 10.1152/ajpendo.00515.2013
25. Tang SC, Peng SJ, Chien HJ. Imaging of the Islet Neural Network. Diabetes Obes Metab (2014) 16 Suppl 1:77–86. doi: 10.1111/dom.12342
26. Lee H, Park JH, Seo I, Park SH, Kim S. Improved Application of the Electrophoretic Tissue Clearing Technology, CLARITY, to Intact Solid Organs Including Brain, Pancreas, Liver, Kidney, Lung, and Intestine. BMC Dev Biol (2014) 14:48. doi: 10.1186/s12861-014-0048-3
27. Susaki EA, Tainaka K, Perrin D, Yukinaga H, Kuno A, Ueda HR. Advanced CUBIC Protocols for Whole-Brain and Whole-Body Clearing and Imaging. Nat Protoc (2015) 10(11):1709–27. doi: 10.1038/nprot.2015.085
28. Juang JH, Kuo CH, Peng SJ, Tang SC. 3-D Imaging Reveals Participation of Donor Islet Schwann Cells and Pericytes in Islet Transplantation and Graft Neurovascular Regeneration. EBioMedicine (2015) 2(2):109–19. doi: 10.1016/j.ebiom.2015.01.014
29. Treweek JB, Chan KY, Flytzanis NC, Yang B, Deverman BE, Greenbaum A, et al. Whole-Body Tissue Stabilization and Selective Extractions Via Tissue-Hydrogel Hybrids for High-Resolution Intact Circuit Mapping and Phenotyping. Nat Protoc (2015) 10(11):1860–96. doi: 10.1038/nprot.2015.122
30. Chien HJ, Peng SJ, Hua TE, Kuo CH, Juang JH, Tang SC. 3-D Imaging of Islets in Obesity: Formation of the Islet-Duct Complex and Neurovascular Remodeling in Young Hyperphagic Mice. Int J Obes (Lond) (2016) 40(4):685–97. doi: 10.1038/ijo.2015.224
31. Lin PY, Peng SJ, Shen CN, Pasricha PJ, Tang SC. PanIN-associated Pericyte, Glial, and Islet Remodeling in Mice Revealed by 3D Pancreatic Duct Lesion Histology. Am J Physiol Gastrointest Liver Physiol (2016) 311(3):G412–22. doi: 10.1152/ajpgi.00071.2016
32. Simon T, Pogu J, Remy S, Brau F, Pogu S, Maquigneau M, et al. Inhibition of Effector Antigen-Specific T Cells by Intradermal Administration of Heme Oxygenase-1 Inducers. J Autoimmun (2017) 81:44–55. doi: 10.1016/j.jaut.2017.03.005
33. Vlahos AE, Cober N, Sefton MV. Modular Tissue Engineering for the Vascularization of Subcutaneously Transplanted Pancreatic Islets. Proc Natl Acad Sci USA (2017) 114(35):9337–42. doi: 10.1073/pnas.1619216114
34. Wong HS, Yeung PKK, Lai HM, Lam KSL, Wutian W, Chung SK. Simple and Rapid Tissue Clearing Method for Three-Dimensional Histology of the Pancreas. Curr Protoc Cell Biol (2017) 77:19.20.1–19.20.10. doi: 10.1002/cpcb.34
35. Yamamoto J, Imai J, Izumi T, Takahashi H, Kawana Y, Takahashi K, et al. Neuronal Signals Regulate Obesity Induced β-Cell Proliferation by FoxM1 Dependent Mechanism. Nat Commun (2017) 8(1):1930. doi: 10.1038/s41467-017-01869-7
36. Pauerstein PT, Tellez K, Willmarth KB, Park KM, Hsueh B, Efsun Arda H, et al. A Radial Axis Defined by Semaphorin-to-Neuropilin Signaling Controls Pancreatic Islet Morphogenesis. Development (2017) 144(20):3744–54. doi: 10.1242/dev.148684
37. Chen L, Li G, Li Y, Li Y, Zhu H, Tang L, et al. Ubasm: An Effective Balanced Optical Clearing Method for Intact Biomedical Imaging. Sci Rep (2017) 7(1):12218. doi: 10.1038/s41598-017-12484-3
38. Hsueh B, Burns VM, Pauerstein P, Holzem K, Ye L, Engberg K, et al. Pathways to Clinical CLARITY: Volumetric Analysis of Irregular, Soft, and Heterogeneous Tissues in Development and Disease. Sci Rep (2017) 7(1):5899. doi: 10.1038/s41598-017-05614-4
39. Tang SC, Shen CN, Lin PY, Peng SJ, Chien HJ, Chou YH, et al. Pancreatic Neuro-Insular Network in Young Mice Revealed by 3D Panoramic Histology. Diabetologia (2018) 61(1):158–67. doi: 10.1007/s00125-017-4408-y
40. Nishimura W, Sakaue-Sawano A, Takahashi S, Miyawaki A, Yasuda K, Noda Y. Optical Clearing of the Pancreas for Visualization of Mature β-Cells and Vessels in Mice. Islets (2018) 10(3):e1451282. doi: 10.1080/19382014.2018.1451282
41. Noë M, Rezaee N, Asrani K, Skaro M, Groot VP, Wu PH, et al. Immunolabeling of Cleared Human Pancreata Provides Insights Into Three-Dimensional Pancreatic Anatomy and Pathology. Am J Pathol (2018) 188(7):1530–5. doi: 10.1016/j.ajpath.2018.04.002
42. Tang SC, Baeyens L, Shen CN, Peng SJ, Chien HJ, Scheel DW, et al. Human Pancreatic Neuro-Insular Network in Health and Fatty Infiltration. Diabetologia (2018) 61(1):168–81. doi: 10.1007/s00125-017-4409-x
43. Fowler JL, Lee SS, Wesner ZC, Olehnik SK, Kron SJ, Hara M. Three-Dimensional Analysis of the Human Pancreas. Endocrinology (2018) 159(3):1393–400. doi: 10.1210/en.2017-03076
44. Shen CN, Goh KS, Huang CR, Chiang TC, Lee CY, Jeng YM, et al. Lymphatic Vessel Remodeling and Invasion in Pancreatic Cancer Progression. EBioMedicine (2019) 47:98–113. doi: 10.1016/j.ebiom.2019.08.044
45. Hong SM, Noe M, Hruban CA, Thompson ED, Wood LD, Hruban RH. A “Clearer” View of Pancreatic Pathology: A Review of Tissue Clearing and Advanced Microscopy Techniques. Adv Anat Pathol (2019) 26(1):31–9. doi: 10.1097/PAP.0000000000000215
46. Tokumoto S, Yabe D, Tatsuoka H, Usui R, Fauzi M, Botagarova A, et al. Generation and Characterization of a Novel Mouse Model That Allows Spatiotemporal Quantification of Pancreatic β-Cell Proliferation. Diabetes (2020) 69(11):2340–51. doi: 10.2337/db20-0290
47. Hahn M, van Krieken PP, Nord C, Alanentalo T, Morini F, Xiong Y, et al. Topologically Selective Islet Vulnerability and Self-Sustained Downregulation of Markers for β-Cell Maturity in Streptozotocin-Induced Diabetes. Commun Biol (2020) 3(1):541. doi: 10.1038/s42003-020-01243-2
48. Maldonado M, Serrill JD, Shih HP. Painting the Pancreas in Three Dimensions: Whole-Mount Immunofluorescence Method. Methods Mol Biol (2020) 2155:193–200. doi: 10.1007/978-1-0716-0655-1_16
49. Roostalu U, Lercke Skytte J, Gravesen Salinas C, Klein T, Vrang N, Jelsing J, et al. 3D Quantification of Changes in Pancreatic Islets in Mouse Models of Diabetes Type I and II. Dis Model Mech (2020) 13(12):dmm045351. doi: 10.1242/dmm.045351
50. Hong SM, Jung D, Kiemen A, Gaida MM, Yoshizawa T, Braxton AM, et al. Three-Dimensional Visualization of Cleared Human Pancreas Cancer Reveals That Sustained Epithelial-to-Mesenchymal Transition is Not Required for Venous Invasion. Mod Pathol (2020) 33(4):639–47. doi: 10.1038/s41379-019-0409-3
51. Graham KD, Lopez SH, Sengupta R, Shenoy A, Schneider S, Wright CM, et al. Robust, 3-Dimensional Visualization of Human Colon Enteric Nervous System Without Tissue Sectioning. Gastroenterology (2020) 158(8):2221–35 e5. doi: 10.1053/j.gastro.2020.02.035
52. Chen J, Lippo L, Labella R, Tan SL, Marsden BD, Dustin ML, et al. Decreased Blood Vessel Density and Endothelial Cell Subset Dynamics During Ageing of the Endocrine System. EMBO J (2021) 40(1):e105242. doi: 10.15252/embj.2020105242
53. Campbell-Thompson M, Butterworth EA, Boatwright JL, Nair MA, Nasif LH, Nasif K, et al. Islet Sympathetic Innervation and Islet Neuropathology in Patients With Type 1 Diabetes. Sci Rep (2021) 11(1):6562–78. doi: 10.1038/s41598-021-85659-8
54. Costantini I, Cicchi R, Silvestri L, Vanzi F, Pavone FS. In-Vivo and Ex-Vivo Optical Clearing Methods for Biological Tissues: Review. BioMed Opt Express (2019) 10(10):5251–67. doi: 10.1364/BOE.10.005251
55. Schega Y, Flinner N, Hansmann ML. Quantitative Assessment of Optical Clearing Methods on Formalin-Fixed Human Lymphoid Tissue. Pathol Res Pract (2020) 216(11):153136. doi: 10.1016/j.prp.2020.153136
56. Richardson DS, Lichtman JW. Clarifying Tissue Clearing. Cell (2015) 162(2):246–57. doi: 10.1016/j.cell.2015.06.067
57. Orlich M, Kiefer F. A Qualitative Comparison of Ten Tissue Clearing Techniques. Histol Histopathol (2018) 33(2):181–99. doi: 10.14670/HH-11-903
58. Ariel P. A Beginner’s Guide to Tissue Clearing. Int J Biochem Cell Biol (2017) 84:35–9. doi: 10.1016/j.biocel.2016.12.009
59. Liu CY, Polk DB. Cellular Maps of Gastrointestinal Organs: Getting the Most From Tissue Clearing. Am J Physiol Gastrointest Liver Physiol (2020) 319(1):G1–G10. doi: 10.1152/ajpgi.00075.2020
60. Renier N, Wu Z, Simon DJ, Yang J, Ariel P, Tessier-Lavigne M. iDISCO: A Simple, Rapid Method to Immunolabel Large Tissue Samples for Volume Imaging. Cell (2014) 159(4):896–910. doi: 10.1016/j.cell.2014.10.010
61. Matsumoto K, Mitani TT, Horiguchi SA, Kaneshiro J, Murakami TC, Mano T, et al. Advanced CUBIC Tissue Clearing for Whole-Organ Cell Profiling. Nat Protoc (2019) 14(12):3506–37. doi: 10.1038/s41596-019-0240-9
62. Susaki EA, Tainaka K, Perrin D, Kishino F, Tawara T, Watanabe TM, et al. Whole-Brain Imaging With Single-Cell Resolution Using Chemical Cocktails and Computational Analysis. Cell (2014) 157(3):726–39. doi: 10.1016/j.cell.2014.03.042
63. Yang B, Treweek JB, Kulkarni RP, Deverman BE, Chen CK, Lubeck E, et al. Single-Cell Phenotyping Within Transparent Intact Tissue Through Whole-Body Clearing. Cell (2014) 158(4):945–58. doi: 10.1016/j.cell.2014.07.017
64. Tomer R, Ye L, Hsueh B, Deisseroth K. Advanced CLARITY for Rapid and High-Resolution Imaging of Intact Tissues. Nat Protoc (2014) 9(7):1682–97. doi: 10.1038/nprot.2014.123
65. Chung K, Deisseroth K. CLARITY for Mapping the Nervous System. Nat Methods (2013) 10(6):508–13. doi: 10.1038/nmeth.2481
66. Gradinaru V, Treweek J, Overton K, Deisseroth K. Hydrogel-Tissue Chemistry: Principles and Applications. Annu Rev Biophys (2018) 47:355–76. doi: 10.1146/annurev-biophys-070317-032905
67. Treweek JB, Gradinaru V. Extracting Structural and Functional Features of Widely Distributed Biological Circuits With Single Cell Resolution Via Tissue Clearing and Delivery Vectors. Curr Opin Biotechnol (2016) 40:193–207. doi: 10.1016/j.copbio.2016.03.012
68. Schindelin J, Arganda-Carreras I, Frise E, Kaynig V, Longair M, Pietzsch T, et al. Fiji: An Open-Source Platform for Biological-Image Analysis. Nat Methods (2012) 9(7):676–82. doi: 10.1038/nmeth.2019
69. Campbell-Thompson ML, Montgomery EL, Foss RM, Kolheffer KM, Phipps G, Schneider L, et al. Collection Protocol for Human Pancreas. J Vis Exp (2012) 63):e4039. doi: 10.3791/4039
70. Sunami E, Kanazawa H, Hashizume H, Takeda M, Hatakeyama K, Ushiki T. Morphological Characteristics of Schwann Cells in the Islets of Langerhans of the Murine Pancreas. Arch Histol Cytol (2001) 64(2):191–201. doi: 10.1679/aohc.64.191
71. Kidd GJ, Ohno N, Trapp BD. Biology of Schwann Cells. Handb Clin Neurol (2013) 115:55–79. doi: 10.1016/B978-0-444-52902-2.00005-9
72. Reinisch CM, Traxler H, Piringer S, Tangl S, Nader A, Tschachler E. Rarefaction of the Peripheral Nerve Network in Diabetic Patients is Associated With a Pronounced Reduction of Terminal Schwann Cells. Diabetes Care (2008) 31(6):1219–21. doi: 10.2337/dc07-1832
73. Griffin JW, Thompson WJ. Biology and Pathology of Nonmyelinating Schwann Cells. Glia (2008) 56(14):1518–31. doi: 10.1002/glia.20778
74. Wekerle H, Schwab M, Linington C, Meyermann R. Antigen Presentation in the Peripheral Nervous System: Schwann Cells Present Endogenous Myelin Autoantigens to Lymphocytes. Eur J Immunol (1986) 16(12):1551–7. doi: 10.1002/eji.1830161214
75. Tzekova N, Heinen A, Küry P. Molecules Involved in the Crosstalk Between Immune- and Peripheral Nerve Schwann Cells. J Clin Immunol (2014) 34 Suppl 1:S86–104. doi: 10.1007/s10875-014-0015-6
76. Tsui H, Chan Y, Tang L, Winer S, Cheung RK, Paltser G, et al. Targeting of Pancreatic Glia in Type 1 Diabetes. Diabetes (2008) 57(4):918–28. doi: 10.2337/db07-0226
77. Winer S, Tsui H, Lau A, Song A, Li X, Cheung RK, et al. Autoimmune Islet Destruction in Spontaneous Type 1 Diabetes is Not Beta-Cell Exclusive. Nat Med (2003) 9(2):198–205. doi: 10.1038/nm818
78. Teitelman G, Guz Y, Ivkovic S, Ehrlich M. Islet Injury Induces Neurotrophin Expression in Pancreatic Cells and Reactive Gliosis of Peri-Islet Schwann Cells. J Neurobiol (1998) 34(4):304–18. doi: 10.1002/(SICI)1097-4695(199803)34:4<304::AID-NEU2>3.0.CO;2-A
79. Pang Z, Kushiyama A, Sun J, Kikuchi T, Yamazaki H, Iwamoto Y, et al. Glial Fibrillary Acidic Protein (GFAP) is a Novel Biomarker for the Prediction of Autoimmune Diabetes. FASEB J (2017) 31(9):4053–63. doi: 10.1096/fj.201700110R
80. Li W, Yu G, Liu Y, Sha L. Intrapancreatic Ganglia and Neural Regulation of Pancreatic Endocrine Secretion. Front Neurosci (2019) 13:21. doi: 10.3389/fnins.2019.00021
81. Gylfe E, Tengholm A. Neurotransmitter Control of Islet Hormone Pulsatility. Diabetes Obes Metab (2014) 16 Suppl 1:102–10. doi: 10.1111/dom.12345
82. Serizawa Y, Kobayashi S, Fujita T. Neuro-Insular Complex Type I in the Mouse. Re-evaluation of the Pancreatic Islet as a Modified Ganglion. Arch Histol Jpn (1979) 42(3):389–94. doi: 10.1679/aohc1950.42.389
83. Proshchina AE, Krivova YS, Barabanov VM, Saveliev SV. Ontogeny of Neuro-Insular Complexes and Islets Innervation in the Human Pancreas. Front Endocrinol (Lausanne) (2014) 5:57. doi: 10.3389/fendo.2014.00057
84. Dybala MP, Kuznetsov A, Motobu M, Hendren-Santiago BK, Philipson LH, Chervonsky AV, et al. Integrated Pancreatic Blood Flow: Bi-Directional Microcirculation Between Endocrine and Exocrine Pancreas. Diabetes (2020) 69(7):1439–50. doi: 10.2337/db19-1034
85. Canzano JS, Nasif LH, Butterworth EA, Fu DA, Atkinson MA, Campbell-Thompson M. Islet Microvasculature Alterations With Loss of Beta-cells in Patients With Type 1 Diabetes. J Histochem Cytochem (2019) 67(1):41–52. doi: 10.1369/0022155418778546
86. Kusmartseva I, Wu W, Syed F, Van Der Heide V, Jorgensen M, Joseph P, et al. Expression of SARS-CoV-2 Entry Factors in the Pancreas of Normal Organ Donors and Individuals With COVID-19. Cell Metab (2020) 32(6):1041–51.e6. doi: 10.1016/j.cmet.2020.11.005
87. Coate KC, Cha J, Shrestha S, Wang W, Gonçalves LM, Almaça J, et al. SARS-Cov-2 Cell Entry Factors ACE2 and TMPRSS2 are Expressed in the Microvasculature and Ducts of Human Pancreas But Are Not Enriched in β Cells. Cell Metab (2020) 32(6):1028–40.e4. doi: 10.1016/j.cmet.2020.11.006
88. Abbruzzese JL, Andersen DK, Borrebaeck CAK, Chari ST, Costello E, Cruz-Monserrate Z, et al. The Interface of Pancreatic Cancer With Diabetes, Obesity, and Inflammation: Research Gaps and Opportunities: Summary of a National Institute of Diabetes and Digestive and Kidney Diseases Workshop. Pancreas (2018) 47(5):516–25. doi: 10.1097/MPA.0000000000001037
89. Bray JK, Elgamal OA, Jiang J, Wright LS, Sutaria DS, Badawi M, et al. Loss of RE-1 Silencing Transcription Factor Accelerates Exocrine Damage From Pancreatic Injury. Cell Death Dis (2020) 11(2):138. doi: 10.1038/s41419-020-2269-7
90. Saisho Y, Butler AE, Meier JJ, Monchamp T, Allen-Auerbach M, Rizza RA, et al. Pancreas Volumes in Humans From Birth to Age One Hundred Taking Into Account Sex, Obesity, and Presence of Type-2 Diabetes. Clin Anat (2007) 20(8):933–42. doi: 10.1002/ca.20543
91. Chiyanika C, Chan DFY, Hui SCN, So HK, Deng M, Yeung DKW, et al. The Relationship Between Pancreas Steatosis and the Risk of Metabolic Syndrome and Insulin Resistance in Chinese Adolescents With Concurrent Obesity and non-Alcoholic Fatty Liver Disease. Pediatr Obes (2020) 15(9):e12653. doi: 10.1111/ijpo.12653
92. Kovanlikaya A, Mittelman SD, Ward A, Geffner ME, Dorey F, Gilsanz V. Obesity and Fat Quantification in Lean Tissues Using Three-Point Dixon MR Imaging. Pediatr Radiol (2005) 35(6):601–7. doi: 10.1007/s00247-005-1413-y
93. Tirkes T, Jeon CY, Li L, Joon AY, Seltman TA, Sankar M, et al. Association of Pancreatic Steatosis With Chronic Pancreatitis, Obesity, and Type 2 Diabetes Mellitus. Pancreas (2019) 48(3):420–6. doi: 10.1097/MPA.0000000000001252
94. Pienkowska J, Brzeska B, Kaszubowski M, Kozak O, Jankowska A, Szurowska E. MRI Assessment of Ectopic Fat Accumulation in Pancreas, Liver and Skeletal Muscle in Patients With Obesity, Overweight and Normal BMI in Correlation With the Presence of Central Obesity and Metabolic Syndrome. Diabetes Metab Syndr Obes (2019) 12:623–36. doi: 10.2147/DMSO.S194690
95. Sorisky A. From Preadipocyte to Adipocyte: Differentiation-Directed Signals of Insulin From the Cell Surface to the Nucleus. Crit Rev Clin Lab Sci (1999) 36(1):1–34. doi: 10.1080/10408369991239169
96. Klemm DJ, Leitner JW, Watson P, Nesterova A, Reusch JE, Goalstone ML, et al. Insulin-Induced Adipocyte Differentiation. Activation of CREB Rescues Adipogenesis From the Arrest Caused by Inhibition of Prenylation. J Biol Chem (2001) 276(30):28430–5. doi: 10.1074/jbc.M103382200
97. Geloen A, Collet AJ, Guay G, Bukowiecki LJ. Insulin Stimulates In Vivo Cell Proliferation in White Adipose Tissue. Am J Physiol (1989) 256(1 Pt 1):C190–6. doi: 10.1152/ajpcell.1989.256.1.C190
98. Ishibashi C, Kozawa J, Hosakawa Y, Yoneda S, Kimura T, Fujita Y, et al. Pancreatic Fat is Related to the Longitudinal Decrease in the Increment of C-peptide in Glucagon Stimulation Test in Type 2 Diabetes Patients. J Diabetes Investig (2020) 11(1):80–7. doi: 10.1111/jdi.13108
99. Sakai NS, Taylor SA, Chouhan MD. Obesity, Metabolic Disease and the pancreas-Quantitative Imaging of Pancreatic Fat. Br J Radiol (2018) 91(1089):20180267. doi: 10.1259/bjr.20180267
100. Ou HY, Wang CY, Yang YC, Chen MF, Chang CJ. The Association Between Nonalcoholic Fatty Pancreas Disease and Diabetes. PloS One (2013) 8(5):e62561. doi: 10.1371/journal.pone.0062561
101. Tirkes T, Shah ZK, Takahashi N, Grajo JR, Chang ST, Venkatesh SK, et al. Reporting Standards for Chronic Pancreatitis by Using Ct, MRI, and MR Cholangiopancreatography: The Consortium for the Study of Chronic Pancreatitis, Diabetes, and Pancreatic Cancer. Radiology (2019) 290(1):207–15. doi: 10.1148/radiol.2018181353
102. Macauley M, Percival K, Thelwall PE, Hollingsworth KG, Taylor R. Altered Volume, Morphology and Composition of the Pancreas in Type 2 Diabetes. PloS One (2015) 10(5):e0126825. doi: 10.1371/journal.pone.0126825
103. Hart PA, Bellin MD, Andersen DK, Bradley D, Cruz-Monserrate Z, Forsmark CE, et al. Type 3c (Pancreatogenic) Diabetes Mellitus Secondary to Chronic Pancreatitis and Pancreatic Cancer. Lancet Gastroenterol Hepatol (2016) 1(3):226–37. doi: 10.1016/S2468-1253(16)30106-6
104. Molina-Montes E, Coscia C, Gomez-Rubio P, Fernandez A, Boenink R, Rava M, et al. Deciphering the Complex Interplay Between Pancreatic Cancer, Diabetes Mellitus Subtypes and Obesity/BMI Through Causal Inference and Mediation Analyses. Gut (2020) 70(2):319–29. doi: 10.1136/gutjnl-2019-319990
105. Santambrogio L. The Lymphatic Fluid. Int Rev Cell Mol Biol (2018) 337:111–33. doi: 10.1016/bs.ircmb.2017.12.002
106. Cesmebasi A, Malefant J, Patel SD, Du Plessis M, Renna S, Tubbs RS, et al. The Surgical Anatomy of the Lymphatic System of the Pancreas. Clin Anat (2015) 28(4):527–37. doi: 10.1002/ca.22461
107. Anderson MS, Bluestone JA. The NOD Mouse: A Model of Immune Dysregulation. Annu Rev Immunol (2005) 23:447–85. doi: 10.1146/annurev.immunol.23.021704.115643
108. Wallberg M, Cooke A. Immune Mechanisms in Type 1 Diabetes. Trends Immunol (2013) 34(12):583–91. doi: 10.1016/j.it.2013.08.005
Keywords: islet, autonomic (vegetative) nervous system, lightsheet microscopy, CLARITY, adipocyte, Schwann cell, confocal 3-D microscopy, organoid
Citation: Campbell-Thompson M and Tang S-C (2021) Pancreas Optical Clearing and 3-D Microscopy in Health and Diabetes. Front. Endocrinol. 12:644826. doi: 10.3389/fendo.2021.644826
Received: 21 December 2020; Accepted: 08 April 2021;
Published: 26 April 2021.
Edited by:
Vincent Poitout, Université de Montréal, CanadaReviewed by:
Holger Andreas Russ, University of Colorado Anschutz Medical Campus, United StatesSenta Georgia, Children’s Hospital of Los Angeles, United States
Rebecca Hull, University of Washington, United States
Copyright © 2021 Campbell-Thompson and Tang. This is an open-access article distributed under the terms of the Creative Commons Attribution License (CC BY). The use, distribution or reproduction in other forums is permitted, provided the original author(s) and the copyright owner(s) are credited and that the original publication in this journal is cited, in accordance with accepted academic practice. No use, distribution or reproduction is permitted which does not comply with these terms.
*Correspondence: Martha Campbell-Thompson, mct@ufl.edu; Shiue-Cheng Tang, sctang@life.nthu.edu.tw