- 1Department of Preventive Medicine, Institute of Biomedical Informatics, Cell Signal Transduction Laboratory, Bioinformatics Center, School of Basic Medical Sciences, Henan University, Kaifeng, China
- 2Gomal Center of Biochemistry and Biotechnology, Gomal University, Dera Ismail Khan, Pakistan
- 3Department of Anesthesia, Stanford University, Stanford, CA, United States
Pheochromocytoma (PCC) is a rare neuroendocrine tumor of the adrenal gland with a high rate of mortality if diagnosed at a late stage. Common symptoms of pheochromocytoma include headache, anxiety, palpitation, and diaphoresis. Different treatments are under observation for PCC but there is still no effective treatment option. Recently, the gene expression profiling of various tumors has provided new subtype-specific options for targeted therapies. In this study, using data sets from TCGA and the GSE19422 cohorts, we identified two distinct PCC subtypes with distinct gene expression patterns. Genes enriched in Subtype I PCCs were involved in the dopaminergic synapse, nicotine addiction, and long-term depression pathways, while genes enriched in subtype II PCCs were involved in protein digestion and absorption, vascular smooth muscle contraction, and ECM receptor interaction pathways. We further identified subtype specific genes such as ALK, IGF1R, RET, and RSPO2 for subtype I and EGFR, ESR1, and SMO for subtype II, the overexpression of which led to cell invasion and tumorigenesis. These genes identified in the present research may serve as potential subtype-specific therapeutic targets to understand the underlying mechanisms of tumorigenesis. Our findings may further guide towards the development of targeted therapies and potential molecular biomarkers against PCC.
Introduction
Pheochromocytoma (PCC) is a type of tumor with catecholamine secretion derived from the chromaffin cells of the sympathoadrenal system (1–4). The majority of PCC arises within the adrenal medulla where the chromaffin cells are located in abundance (4). However, a small number of them are found in extra-adrenal sites (such as neck, mediastinum, abdomen, pelvis, and organ of Zuckerkandl) and are termed Paragangliomas (4, 5). The annual incidence rate of PCC is 1-4/106 of the population while the recurrence rate is 4.6-6.5% (5, 6). In females the tendency for PCC progression (55.2%) is slightly higher than in males (44.8%). PCC occurs most frequently in aged individuals around 40-50 years old (7, 8). The most common signs and symptoms include hypertension, palpitation, headache, pallor, and sweating because of excessive catecholamine secretion. While the less common signs and symptoms are fever, nausea, weight loss, constipation, flushing, and fatigue (9). The metastases rate is about 10-15%in pheochromocytoma patients (10), but only a few patients are suitable candidates for surgical resection of the tumor in this case (11). Although the survival advantage of surgical debunking is not proven, it can significantly reduce organ damage, catecholamine secretion, and the required dosage of alpha and beta blockades. The decreased tumor burden as a result of surgical resection can also assist in successive radiotherapy or chemotherapy (9). Different methodologies are under process for guiding the treatment of cancers including the recently developed gene expression profiling methods used against gastric cancer, breast cancer, and uterine carcinosarcomas (12–18). The successful categorization of cancers into different molecular subtypes help cancer patients to receive better diagnosis and get more effective therapy for cancers (19). Therefore, the characterization of PCC into molecular subgroups will provide a better understanding of the underlying mechanisms of disease and thus will lead to a better and more precise treatment for PCC in the future. In the current study, by using gene expression profiling method, we successfully defined two distinct solid subtypes of PCC with enriched different potential genes and pathways. Our findings will accelerate the understanding of PCC pathogenesis and provide opportunities for effective subtype-specific therapies.
Materials and Methods
Determination and Validation of Molecular Subtypes of PCC
TCGA and Gene Expression Omnibus (GEO) databases were checked to obtain the Expression profiling data of clinical PCC cases. Two datasets, including one dataset from TCGA (154 cases) and the other dataset of GSE19422 from GEO (63 samples), were collected and used to define the molecular subtypes of PCC. After filtering individual expression datasets with standard deviation, the transformation of the data was done by gene-based centering. To identify the molecular subtypes, both datasets were separately run on Consensus clustering (R package Consensus clustering Plus) (20) with a set of parameters, including 80% sample resampling, distance (1-Pearson correlation), 80% gene resampling, maximum evaluated k of 12, agglomerative hierarchical clustering algorithm, and 1000 iterations. Finally, the R package cluster (silhouette width) was used to determine the accuracy of subtype assignment from Consensus Clustering Plus (21).
Reproducibility Measurement of PCC Molecular Subtypes
Subclass Mapping (SubMap) implemented in Gene Pattern was used to determine the reproducibility of PCC molecular subtypes between TCGA and GSE19422 cohorts. SubMap analysis was achieved with parameters of (num. marker. genes=300, num.perm=1000 and num.per.fisher=1000) (22).
Gene Ontology and Gene Set Enrichment (GSEA) Analysis
Subtype-specific genes were identified by SAM (23) and SAMseq (24) with a false discovery rate of less than 0.05. GO and KEGG pathway analyses were performed using DAVID Bioinformatics resources online version 6.7 (https://david.ncifcrf.gov/). GSEA (25) analysis was carried out to examine the expression of gene patterns and pathways of each subtype. Furthermore, therapeutic genes of each PCC subtype were explored through the TARGET V2 database (http://www.broadinstitute.org/cancer/cga/target).
Statistical Analysis
For the evaluation of statistical significance between the clinical factors and subtypes of PCC, Fisher exact tests and chi-square test were applied and a p-value value less than 0.05 was considered to be significant. The survival curve was also calculated by log-rank test and Kaplan-Meier plot through Graphpad Prism 7 software.
Results
Consensus Clustering Identifies Two Different PCC Molecular Subtypes
PCC subtypes were identified using the gene expression profiling data of PCC with consensus clustering. Initially, the TCGA cohort (154 PCC samples) was revealed to have two optimal molecular subtypes based on the curve of empirical cumulative distribution (CDF) (Figures 1A–C). Subtype assignment was confirmed through silhouette width analysis. Out of 154 samples, 114 samples were found to have positive silhouette value, which was used for further analysis. In 114 samples, subtype I gathered 69 samples while 45 samples belonged to subtype II (Figure 1D).
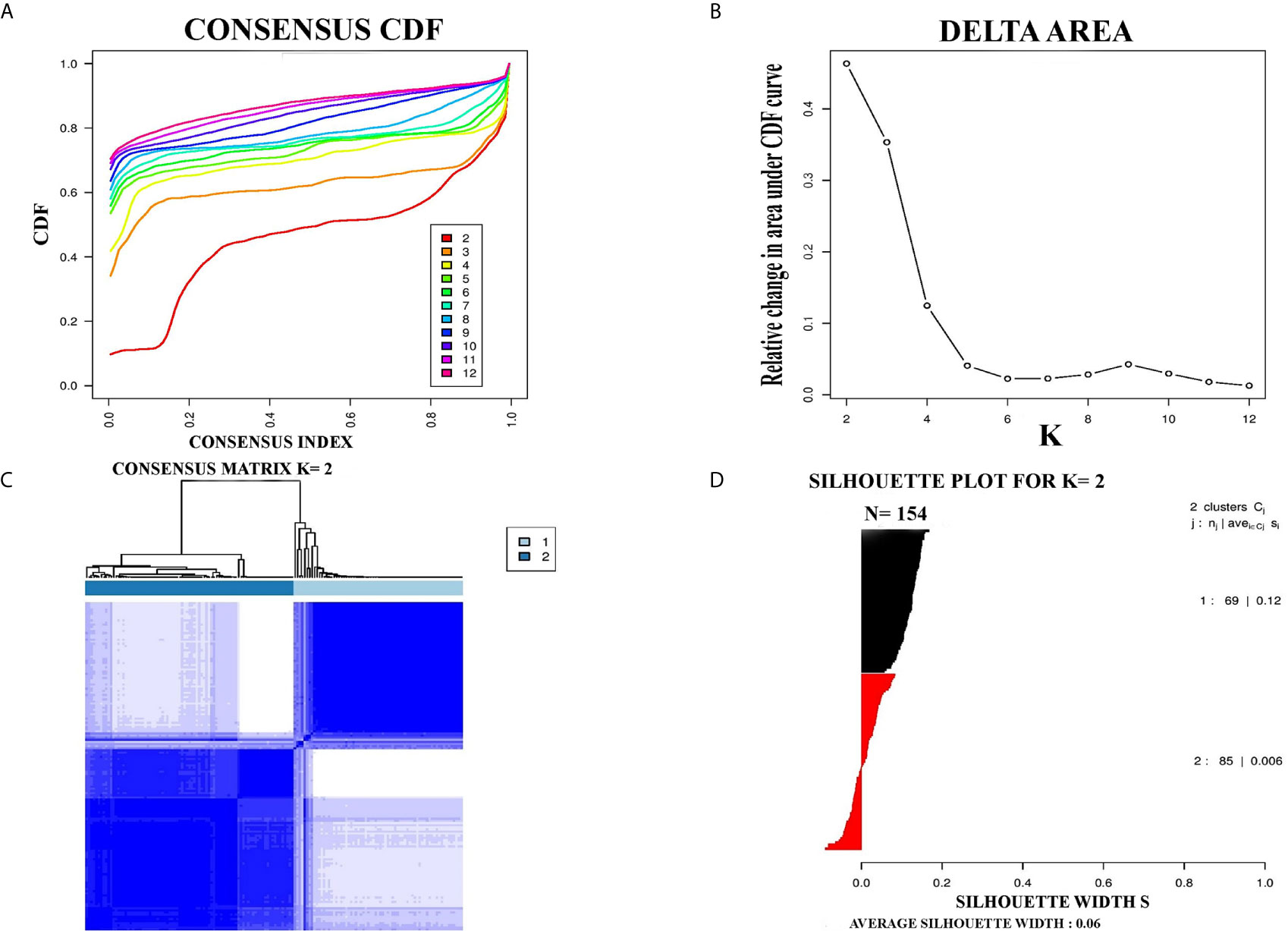
Figure 1 Represented two molecular subtypes in TCGA cohort of PCC. (A) The optimal number of PCC molecular subtypes was determined through Empirical cumulative distribution plot. (B) The increasement of area under the CDF curve with the increased expected number of molecular subtypes. (C) Consensus clustering matrix for the two distinct subtypes of PCC. (D) Silhouette plot based on Consensus clustering assignment.
Further Validation of PCC Molecular Subtypes in an Independent Dataset
For further confirmation of PCC subtypes identified in the TCGA cohort, a GEO dataset (GSE19422) with 63 PCC cases was analyzed. Consensus clustering identified two distinct molecular subtypes in the GSE19422 dataset as well (Figure 2). As in the TCGA dataset, positive silhouette cases were obtained and used for further analysis in GSE19422.
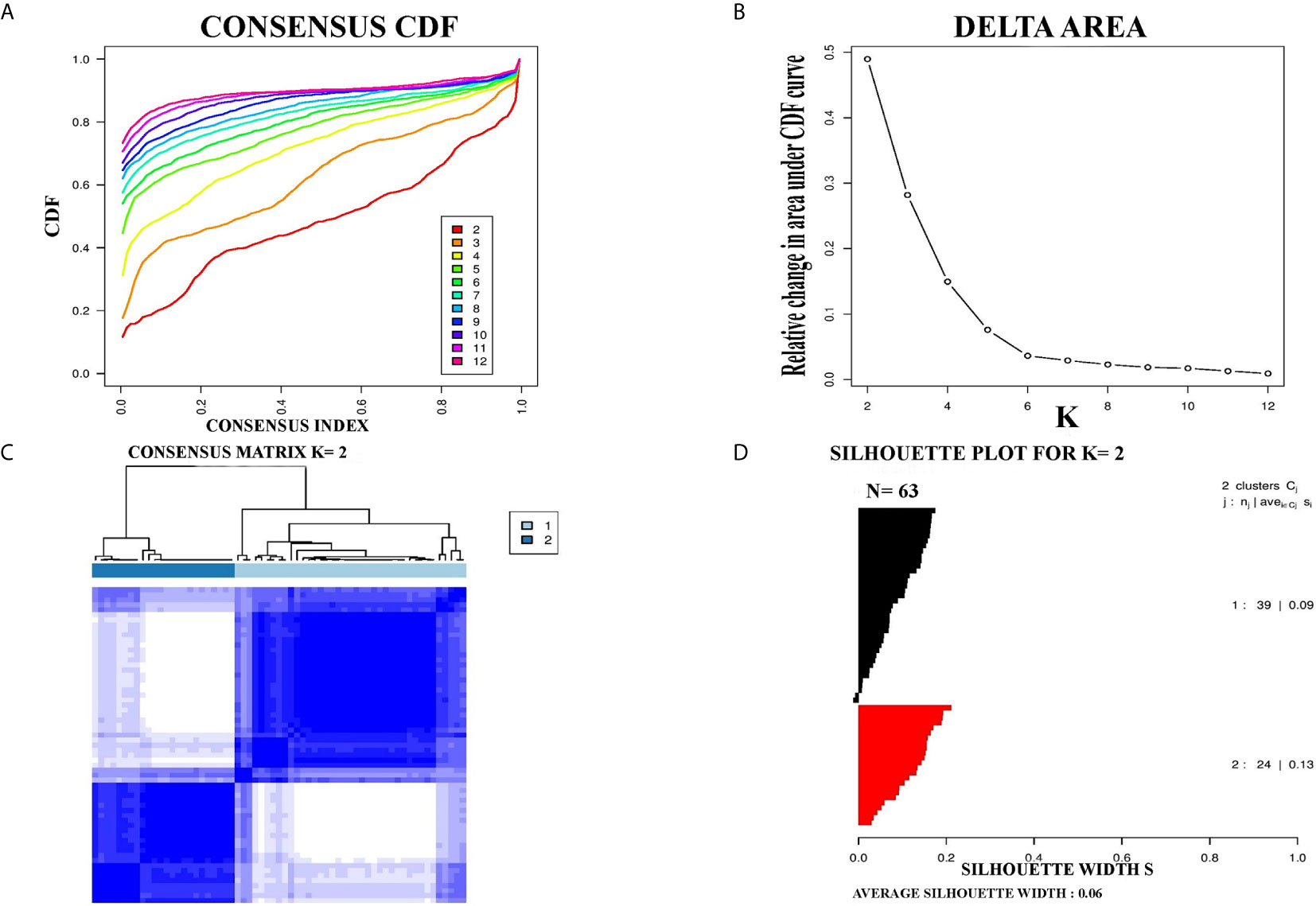
Figure 2 Identification of two molecular subtypes in GSE19422 cohort of PCC. (A) The optimal number of PCC molecular subtypes was defined through Empirical cumulative plot. (B) Comparative increase in the area under the CDF curve with the increasing expected number of molecular subtypes. (C) Consensus clustering matrix of the two PCC subtypes. (D) Silhouette plot of PCC samples based on Consensus clustering assignment.
Reproduced Molecular Subtypes in Independent PCC Cohorts by SubMap Analysis
The correlation of two distinct molecular subtypes of PCC in independent datasets was measured through SubMap analysis. The result of SubMap analysis revealed a significant correlation between A1-A2 subtypes of TCGA with the B1-B2 of GSE19422 (Figure 3), indicating the common and reproducible PCC molecular subtype across different cohorts.
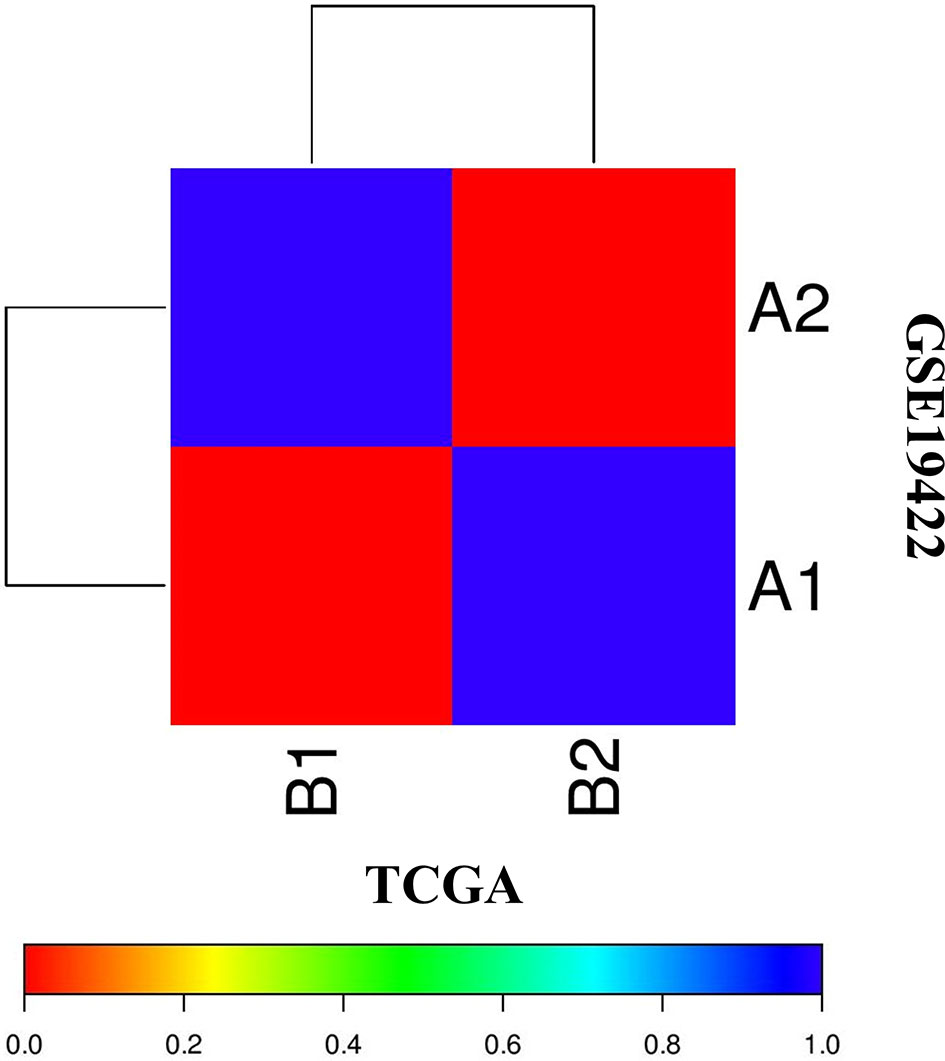
Figure 3 SubMap association between the two molecular subtypes from the two independent datasets of TCGA and GSE19422 presents a significant correlation. FDR-corrected p-value denoted the correlation significance.
Clinical Characteristics of PCC Molecular Subtypes
To understand the clinical characteristics of PCC molecular subtypes, the relationship between molecular subtypes and clinical factors was checked in the TCGA cohort. Notably, the laterality rate of subtype II PCC was found to be higher on the left side of patients (24/45) than subtype I PCC (34/69) (Supplementary Table S1, P = 0.2057). Furthermore, it is noteworthy to highlight that the detection rate of the disease during the initial screening was found to be higher in subtype I PCC patients (37/69) than in subtype II PCC patients (22/45) (P = 0.5883). The mean age at diagnosis was found to be higher in Subtype I PCCs (48.4 years) as compared to Subtype II PCCs (41.8 years) (P=0.02*). The median overall survival (OS) time in patients of Subtype I PCCs was recorded as 736 days, which was slightly shorter than the patients with Subtype II PCCs who had an OS time period of 944 days. However, survival curve analysis (Kaplan-Meier plots) showed no significant difference in survival between the two PCC subtypes. In addition to the survival rate, there was no significant difference found based on the patient’s sex among the two subtypes (P = .5248) as well as their success in primary therapy outcome (P = 0.6136) (Supplementary Table S1).
Functional Analysis of PCC Subtype-Specific Genes
SAMseq analysis was performed in the TCGA dataset to analyze differentially expressed genes between two PCC molecular subtypes. A total of 6813 genes were found to have differential expression between the subtypes, among which 2840 genes were overexpressed in subtype I while 3973 genes had higher expression in subtype II (Supplementary Table S2). KEGG and GO analyses were performed on the Top 1000 overexpressed genes from each PCC subtype to obtain further biological information about the subtypes. GO analysis revealed 187 biological processes enriched in subtype I, including Nervous system-associated genes (4.5%) (Supplementary Table S3). KEGG analysis of subtype I overexpressing genes revealed 23 different pathways that belonged to Neuroactive ligands receptor interaction, cAMP signaling pathways, and Calcium signaling pathways, (Figure 4A). Whereas 259 biological processes and 26 KEGG pathways were significantly enriched in subtype II PCCs. These pathways included Vascular Smooth Muscle Contraction, ECM Receptor Interaction, and Hedgehog Signaling Pathway. (Figure 4B). In addition, GSEA analysis in the TCGA cohort demonstrated gene sets enriched with significant biological pathways were found to be abundant only in subtype II. These pathways for subtype II include Hedgehog signaling pathways, Vascular smooth interaction, ECM interaction, and TGF Beta signaling pathway (Figure 5).
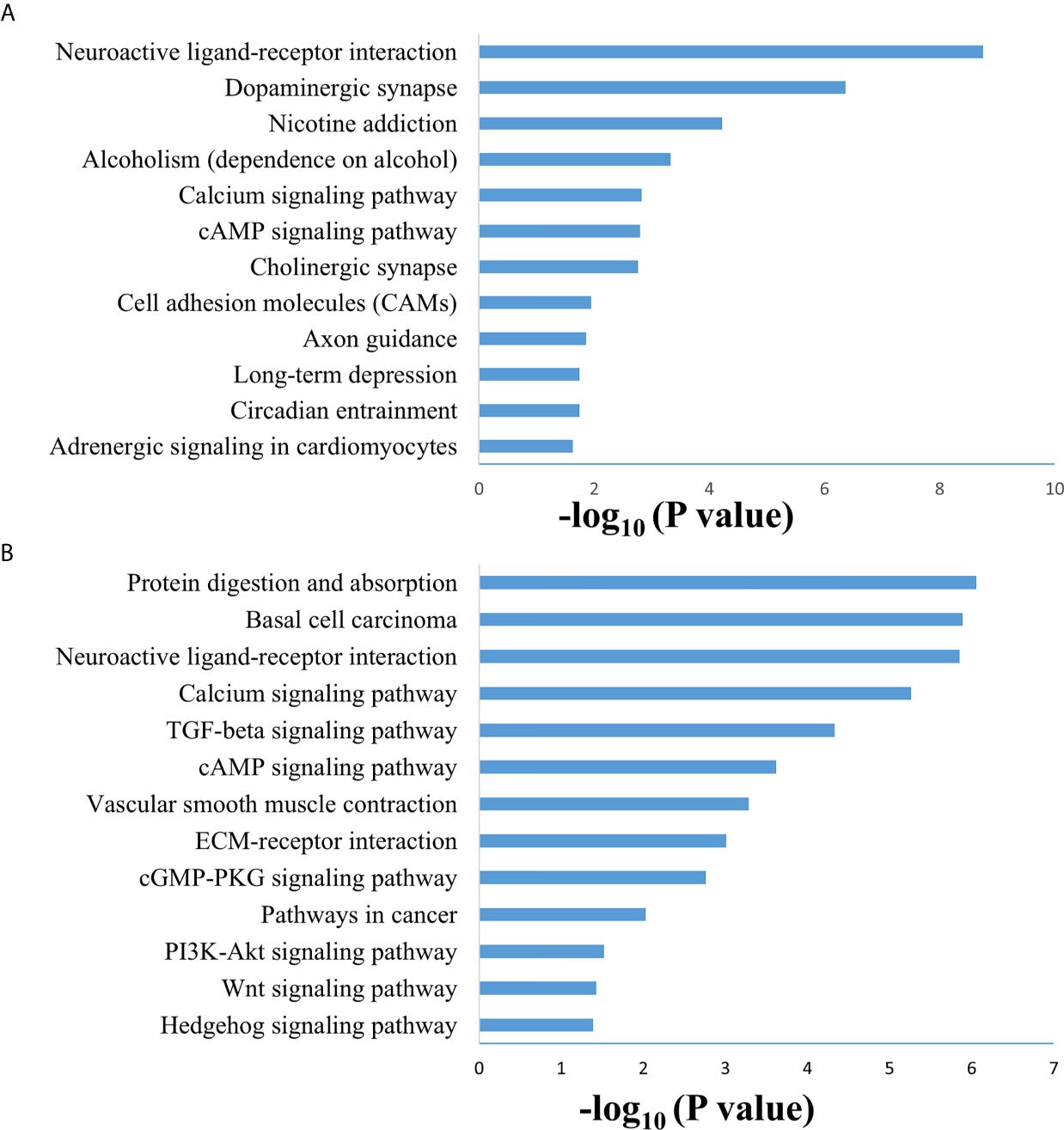
Figure 4 Enriched biological pathways found through the analysis of overexpression of genes individual PCC subtypes. (A) KEGG pathway through the gene overexpression profile analysis in subtype (I) (B) KEGG pathway through the gene overexpression profile analysis subtype II.
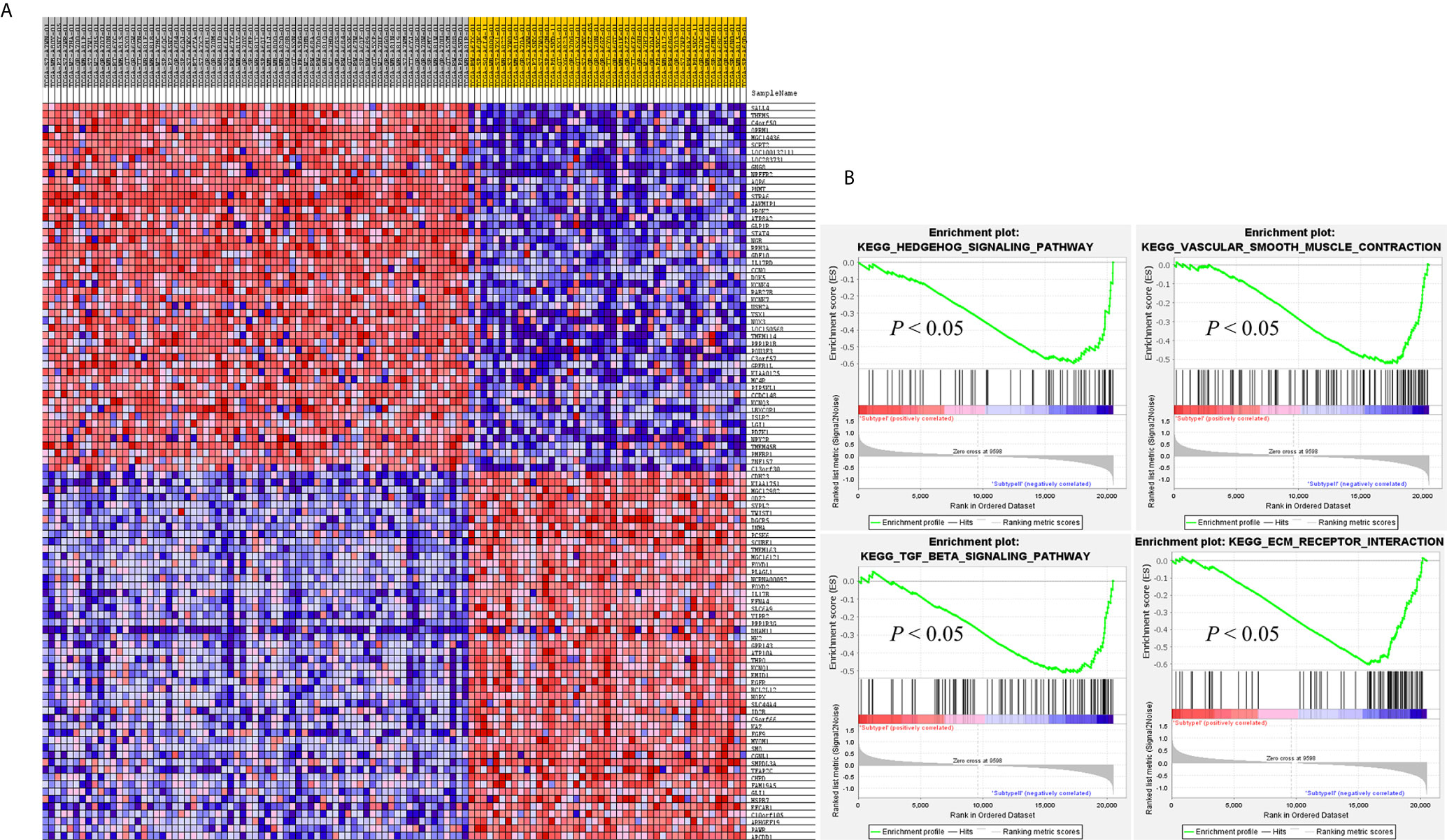
Figure 5 GSEA results validates the different gene expression patterns found in each PCC subtype. (A) Heatmap of gene expression of Top 100 genes in subtype I and subtype II. Over-expressed genes (Red) and down-expressed genes (blue). (B) GSEA enriched pathways in subtype II. GSEA results presented improved expression of vascular smooth muscle contraction and hedgehog signaling pathway. Distinct gene expression signatures are produced by the individual gene sets enriched in subtype I and subtype II. Permutation = 1000, p < 0.01.
Clinical Implication of PCC Subtyping
The purpose of molecular subtyping of PCC was to search and identify therapeutic routes and to employ those specified routes further in clinical studies and discourses. Overexpressed genes in each PCC subtype were obtained and compared with the target database (that contains target genes and functional inhibitors) for the determination of therapeutic molecules (26). Further studies may be carried out on the targeted genes to translate them into potential clinical stages (27–29).
We have found seven subtype-specific target genes which would give relative benefits to the PCC patients from distinct subtypes. Given in Table 1, subtype I PCCs benefit from four target genes, namely ALK, IGF1R, RET, and RSPO2, while subtype II contains three target genes: EGFR, ESR1, and SMO.
Discussion
PCC is a type of catecholamine-secreting neuroendocrine tumors, most of which arise from chromaffin cells of the adrenal medulla. About 15-20% of these PCC tumors belong to an extra-adrenal origin and are termed as paraganglioma (PGL) (30). Due to excessive secretion of catecholamines, both forms of tumors (adrenal and extra-adrenal) have similar clinical symptoms and are only distinguished based on potential differences in prognosis (31). Most of the PCCs are benign, although metastasis may develop in patients with specific backgrounds (32, 33). At present, different studies have debated PCC treatment. Patients at the same stage may still respond to treatment differently due to the molecular heterogeneity even if they were administered the identical treatment (34, 35). Molecular subtyping approaches based on gene expression profiling of tumors has greatly guided the medical community in introducing subtype specific diagnostic techniques and targeted therapies (20). The subtype-based targeted therapies in clinical trials of breast cancer is a good example of molecular prognostic and treatment of malignancies. The positive response of HER2-positive breast cancer patients towards subtype specific therapies is an example for the future directions of the current study (36). Using the gene expression profiling method, it is possible to get a better understanding of the heterogeneity of PCCs, and also provides the opportunity to develop subtype-specific therapeutic strategies.
In this study, we identified two molecular subtypes of PCC (also confirmed previously by Fishbian et al.) (37). The Gene set enrichment and Gene ontology analyses of the identified subtypes revealed the overexpression of certain genes and pathways specific to each subtype (Supplementary Table S4). The subtype I PCCs include the overexpressed genes involved in pathways of Dopaminergic synapse, Nicotine addiction, and Long-term depression. The SALL4 involved in the proliferation of cancer was also found to be overexpressed within subtype I (Figure 5). SALL4 gene is involved in the maintenance of pluripotency and self-renewal of embryonic stem cells (38, 39). Expression of SALL4 has been reported in various cancers such as precursor B-cell lymphoblastic lymphoma (40, 41), acute myeloid leukemia (42), myelodysplastic syndromes (43), breast cancer (44), chronic myeloid leukemia (45), lung cancer (46, 47), endometrial cancer (48), liver cancer (49, 50) gastrointestinal carcinoma (51–53), glioma (54), germ cell tumor, and yolk sac tumor (55, 56). In subtype II PCCs, enriched genes and pathways include the overexpression of smooth muscle-specific markers and the genes involved in the lymph node. Overexpressed pathways in subtype II include protein digestion and absorption pathway, Vascular smooth muscle contraction pathway, and ECM-receptor interaction pathway. The overexpressed gene in this subtype include Twist1 (Figure 5). The consistent significance of Twist1 gene in cancer biology involves its overexpression which is linked to metastasis, therapeutic failure, recurrence, and inferior prognosis (57). The role of Twist1 has already been shown to serve as a useful prognostic factor predicting poor outcome in breast cancer (58), nasopharyngeal cancer (59), ovarian cancer (60) and cervical cancer (61). Expression of these distinct genes and pathways in each subtype will provide a better way to understand PCC at the subtype level and to develop subtype-specific treatment.
After analyzing the overexpressed genes and pathways in each subtype, we further checked these overexpressed genes in the TARGET database and identified seven known target genes for each subtype. Subtype I PCCs have four overexpressed genes, namely ALK, IGF1R, RET, and RSPO2, while subtype II includes EGFR, ESR1, and SMO. In most types of cancers, overexpression of the IGF1R gene is found to be a typical hallmark (62). In addition, IGF1R has an important role in invasion, metastasis, and angiogenesis (63–65). Its overexpression has also been found in pheochromocytoma and paraganglioma with a high risk of metastasis (66).
Blocking the IGF1R via antisense therapy (67), anti IGF1R antibodies (68–70), dominant negative IGF1R (71), and small-molecule inhibitors has proven efficacious in the treatment of various cancers. A preclinical study found Cixutumumad effective against prostate cancer as it caused significant delaying of the androgen resistance by blocking IGF1R in disease (72, 73). Recent studies have suggested linsitinib (OSI-906) as a promising drug for PCC patients, when used alone or combined with mTOR inhibitors (74). Therefore, IGF1R inhibitors may play a significant role in subtype I of PCC.
The overexpression of EGFR has been observed to play a key role in tumorigenesis (75). Targeting EGFR using different approaches has proven effective in the treatment of various solid tumors such as head and neck, colorectal, pancreatic, and non-small lung cancer (NSCLS) (76–80). Gefitinib, the first FDA-approved anti-EGFR drug (81, 82), has been shown to prevent autophosphorylation of EGFR in many tumor cell lines and xenografts (83). It inhibits the cell growth in HER2-overexpressing breast cancer cells (84, 85). Similarly, Erlotinib is another FDA-approved drug that acts as an inhibitor of EGFR (86, 87) and has been proven effective in the treatment of NSCLC and metastatic pancreatic cancer when used in combination with gemcitabine (76, 80). Gene expression analysis revealed the role of the EGFR gene in subtype II patients of the PCC cohort. Based on the EGFR’s role in cancer and the availability of anti-EGFR inhibitors, patients of subtype II may benefit from anti-EGFR inhibitors.
In conclusion, we have characterized two distinct molecular subtypes of PCC in two independent cohorts. Differentially expressed genes found in the two subtypes provide an insight into the underlying mechanisms of tumorigenesis and progression in a subtype-specific manner. Targeted therapies against molecular targets identified in the present study may help better understand the disease prognosis and aid in developing specified therapies against individual subtypes of PCC.
Data Availability Statement
The datasets presented in this study can be found in online repositories. The names of the repository/repositories and accession number(s) can be found in the article/Supplementary Material.
Author Contributions
Term Definition Conceptualization: XG. Methodology: XG. Software: XG. Validation: US, XG. Formal analysis: US, XG, FW, ZL. Investigation: US, XG. Resources: XG, XJ, YL. Data Curation: US, XG. Writing - Original Draft: US, MA, WZ, XG. Writing - Review and Editing: US, MA, WZ, XG, XJ, LX, YA. Visualization: US. Supervision: XG, XJ, YL. Project administration: XG, XJ, YL. Funding acquisition: XG. All authors contributed to the article and approved the submitted version.
Funding
This work was supported by the program for Innovative Talents of Science and Technology in Henan Province (No. 18HASTIT048). The funding bodies were not involved in the study design, data collection, analysis and interpretation of data, or writing of this manuscript.
Conflict of Interest
The authors declare that the research was conducted in the absence of any commercial or financial relationships that could be construed as a potential conflict of interest.
Supplementary Material
The Supplementary Material for this article can be found online at: https://www.frontiersin.org/articles/10.3389/fendo.2021.605797/full#supplementary-material
References
1. Portela-Gomes GM, Stridsberg M, Grimelius L, Falkmer UG, Falkmer S. Expression of Chromogranins A, B, and C (Secretogranin II) in Human Adrenal Medulla and in Benign and Malignant Pheochromocytomas. An Immunohistochemical Study With Region-Specific Antibodies. APMIS (2004) 112:663–73. doi: 10.1111/j.1600-0463.2004.t01-1-apm1121005.x
2. Allibhai Z, Rodrigues G, Brecevic E, Neumann HP, Winquist E. Malignant Pheochromocytoma Associated With Germline Mutation of the SDHB Gene. J Urol (2004) 172:1409 –10. doi: 10.1097/01.ju.0000137892.89141.6a
3. De Toma G, Letizia C, Cavallaro G, Cavallaro G, Giacchino V, Mosiello G, et al. Malignant Pheochromocytoma. Personal Experience and Review of the Literature. Ann Ital Chir (2002) 73:413– 8.
4. Thompson LD. Pheochromocytoma of the Adrenal Gland Scaled Score (PASS) to Separate Benign From Malignant Neoplasms. A Clinicopathologic and Immunophenotypic Study of 100 Cases. Am J Surg Pathol (2002) 26:551–66. doi: 10.1097/00000478-200205000-00002
5. Adler JT, Meyer-Rochow GY, Chen H, Benn DE, Robinson BG, Sippel RS, et al. Pheochromocytoma: Current Approaches and Future Directions. Oncologist (2008) 13:779–93. doi: 10.1634/theoncologist.2008-0043
6. Tang SH, Chen A, Lee CT, Yu DS, Chang SY, Sun GH. Remote Recurrence of Malignant Pheochromocytoma 14 Years After Primary Operation. J Urol (2003) 169:269. doi: 10.1097/00005392-200301000-00066
7. Lenders JW, Eisenhofer G, Mannelli M, Pacak K. Phaeochromocytoma. Lancet (2005) 366:665–75. doi: 10.1016/S0140-6736(05)67139-5
8. Adas M, Koc B, Adas G, Yalcin O, Celik S, Kemik O. Pitfalls in the Diagnosis of Pheochromocytoma: A Case Series and Review of the Literature. J Epidemiol Res (2016) 2:49–55. doi: 10.5430/jer.v2n2p49
9. Chen H, Sippel RS, O’Dorisio MS, Vinik AI, Lloyd RV, Pacak K. North American Neuroendocrine Tumor Society (Nanets). The North American Neuroendocrine Tumor Society Consensus Guideline for the Diagnosis and Management of Neuroendocrine Tumors: Pheochromocytoma, Paraganglioma, and Medullary Thyroid Cancer. Pancreas (2010) 39:775–83. doi: 10.1097/MPA.0b013e3181ebb4f0
10. Pacak K, Wimalawansa SJ. Pheochromocytoma and Paraganglioma. Endocri Pract (2015) 21:406–12. doi: 10.4158/EP14481.RA
11. Pacak K, Linehan WM, Eisenhofer G, Walther MM, Goldstein DS. Recent Advances in Genetics, Diagnosis, Localization, and Treatment of Pheochromocytoma. Ann Intern Med (2001a) 134:315–29. doi: 10.7326/0003-4819-134-4-200102200-00016
12. Bertucci F, Finetti P, Rougemont J, Charafe–Jauffret E, Cervera N, Tarpin C, et al. Gene Expression Profiling Identifies Molecular Subtypes of Inflammatory Breast Cancer. Cancer Res (2005) 65(6):2170–8. doi: 10.1158/0008-5472.CAN-04-4115
13. Sorlie T, Perou CM, Tibshirani R, Aas T, Geisler S, Johnsen H, et al. Gene Expression Patterns of Breast Carcinomas Distinguish Tumor Subclasses With Clinical Implications. Proc Natl Acad Sci U.S.A. (2001) 98(19):10869–74. doi: 10.1073/pnas.191367098
14. Lei Z, Tan IB, Das K, Deng N, Zouridis H, Pattison S, et al. Identification of Molecular Subtypes of Gastric Cancer With Different Responses to PI3-Kinase Inhibitors and 5-Fluorouracil. Gastroenterology (2013) 145(3):554–65. doi: 10.1053/j.gastro.2013.05.010
15. Cristescu R, Lee J, Nebozhyn M, Kim KM, Ting JC, Wong SS, et al. Molecular Analysis of Gastric Cancer Identifies Subtypes Associated With Distinct Clinical Outcomes. Nat Med (2015) 21(5):449–56. doi: 10.1038/nm.3850
16. Saddozai UAK, Wang F, Cheng Y, Lu Z, Akbar MU, Zhu W, et al. Gene Expression Profile Identifies Distinct Molecular Subtypes and Potential Therapeutic Genes in Merkel Cell Carcinoma. Trans Oncol (2020) 13(11):100816. doi: 10.1016/j.tranon.2020.100816
17. Wang F, Zhongyi Y, Jiajia L, Junfang X, Yifang D, Xiaoxiao S. Gene Expression Profiling Reveals Distinct Molecular Subtypes of Oesophageal Squamous Cell Carcinoma in Asian Population. Neoplasia (2019) 21:571–81. doi: 10.1016/j.neo.2019.03.013
18. Flynn A, Dwight T, Harris J, Benn D, Zhou L, Hogg A, et al. Pheo-Type: A Diagnostic Gene-expression Assay for the Classification of Pheochromocytoma and Paraganglioma. J Clin Endocrinol Metab (2016) Mar101(3):1034–43. doi: 10.1210/jc.2015-3889
19. Goldhirsch A, Wood WC, Coates AS, Gelber RD, Thürlimann B, Senn HJ. Strategies for Subtypes–Dealing With the Diversity of Breast Cancer: Highlights of the St. Gallen International Expert Consensus on the Primary Therapy of Early Breast Cancer. Ann Oncol (2011) 22(8):1736–47. doi: 10.1093/annonc/mdr304
20. Wilkerson MD, Hayes DN. Consensus Cluster Plus; a Class Discovery Tool With Confidence Assessments and Item Tracking. Bioinformatics (2010) 26:1572–3. doi: 10.1093/bioinformatics/btq170
21. Rousseeuw PJ. Silhouettes; a Graphical Aid to the Interpretation and Validation of Cluster Analysis. J Comput Appl Math (1987) 20:53–65. doi: 10.1016/0377-0427(87)90125-7
22. Hoshida Y, Brunet JP, Tamayo P, Golub TR, Mesirov JP. Subclass Mapping; Identifying Common Subtypes in Independent Disease Data Sets. PloS One (2007) 2:e1195. doi: 10.1371/journal.pone.0001195
23. Tusher VG, Tibshirani R, Chu G. Significance Analysis of Microarrays Applied to the Ionizing Radiation Response. Proc Natl Acad Sci USA (2001) 98:5116–21. doi: 10.1073/pnas.091062498
24. Li J, Tibshirani R. Finding Consistent Patterns; a Nonparametric Approach for Identifying Differential Expression in RNA-Seq Data. Stat Methods Med Res (2013) 22:519–36. doi: 10.1177/0962280211428386
25. Subramanian A, Tamayo P, Mootha VK, Mukherjee S, Ebert BL, Gillette MA. Gene Set Enrichment Analysis; a Knowledge-Based Approach for Interpreting Genome-Wide Expression Profiles. Proc Natl Acad Sci (2005) 102:15545–50. doi: 10.1073/pnas.0506580102
26. Van Allen EM, Wagle N, Stojanov P, Perrin DL, Cibulskis K, Marlow S. Whole-Exome Sequencing and Clinical Interpretation of Formalin Fixed, Paraffin-Embedded Tumor Samples to Guide Precision Cancer Medicine. Nat Med (2014) 20:682–8. doi: 10.1038/nm.3559
27. Moroni M, Veronese S, Benvenuti S, Marrapese G, Sartore-Bianchi A, Di-Nicolantonio F. Gene Copy Number for Epidermal Growth Factor Receptor (EGFR) and Clinical Response to Anti-EGFR Treatment in Colorectal Cancer; a Cohort Study. Lancet Oncol (2005) 6:279–86. doi: 10.1016/S1470-2045(05)70102-9
28. Lehmann BD, Bauer JA, Chen X, Sanders ME, Chakravarthy AB, Shyr Y. Identification of Human Triple-Negative Breast Cancer Subtypes and Preclinical Models for Selection of Targeted Therapies. J Clin Invest (2011) 121:2750–67. doi: 10.1172/JCI45014
29. Paez JG, Jänne PA, Lee JC, Tracy S, Greulich H, Gabriel S. EGFR Mutations in Lung Cancer; Correlation With Clinical Response to Gefitinib Therapy. Science (2004) 304:1497–500. doi: 10.1126/science.1099314
30. Lenders JW, Eisenhofer G, Mannelli M, Pacak K. Phaeochromocytoma. Lancet (2005) 366(9486):665–75. doi: 10.1016/S0140-6736(05)67139-5
31. Omura M, Saito J, Yamaguchi K, Kakuta Y, Nishikawa T. Prospective Study on the Prevalence of Secondary Hypertension Among Hypertensive Patients Visiting a General Outpatient Clinic in Japan. Hypertens Res (2004) 27(3):193–202. doi: 10.1291/hypres.27.193
32. Ayala-Ramirez M, Feng L, Johnson MM, Ejaz S, Habra MA, Rich T, et al. Clinical Risk Factors for Malignancy and Overall Survival in Patients With Pheochromocytomas and Sympathetic Paragangliomas: Primary Tumor Size and Primary Tumor Location as Prognostic Indicators. J Clin Endocrinol Metab (2011) 96:717–25. doi: 10.1210/jc.2010-1946
33. Eisenhofer G, Lenders JW, Siegert G, Bornstein SR, Friberg P, Milosevic D, et al. Plasma Methoxytyramine: A Novel Biomarker of Metastatic Pheochromocytoma and Paraganglioma in Relation to Established Risk Factors of Tumour Size, Location and SDHB Mutation Status. Eur J Cancer (2012) 48:1739–49. doi: 10.1016/j.ejca.2011.07.016
34. Gerlinger M, Rowan AJ, Horswell S, Math M, Larkin J, Endesfelderm D, et al. Intratumor Heterogeneity and Branched Evolution Revealed by Multiregion Sequencing. N Engl J Med (2012) 366:883–92. doi: 10.3410/f.14001976.792252863
35. Navin N, Kendall J, Troge J, Andrews P, Rodgers L, McIndoo J, et al. Tumor Evolution Inferred by Single Cell Sequencing. Nature (2011) 472:90–4. doi: 10.1038/nature09807
36. Piccart-Gebhart MJ, Procter M, Leyland-Jones B. Trastuzumab After Adjuvant Chemotherapy in HER2- Positive Breast Cancer. N Engl J Med (2005) 353:1659–72. doi: 10.1056/NEJMoa052306
37. Fishbein L, Leshchiner I, Walter V, Danilova L, Robertson AG, Johnson AR, et al. Comprehensive Molecular Characterization of Pheochromocytoma and Paraganglioma. Cancer Cell (2017) 2:181–93. doi: 10.1016/j.ccell.2017.01.001
38. Yang L, Chai TC, Fowles Z, Alipio Z, Xu D, Fink LM, et al. Genome-Wide Analysis Reveals Sall4 to be a Major Regulator of Pluripotency in Murineembryonic Stem Cells, Proc. Natl Acad Sci USA (2008) 105:19756–61. doi: 10.1073/pnas.0809321105
39. Zhang WL, Tam GQ, Tong Q, Wu Q, Chan HY, Soh BS, et al. SALL4 Modulates Embryonic Stem Cell Pluripotency and Early Embryonic Development by the Transcriptional Regulation of Pou5f1. Nat Cell Biol (2006) 8:1114–23. doi: 10.1038/ncb1481
40. Ueno J, Lu J, He J, Li A, Zhang X, Ritz J, et al. Aberrant Expression of SALL4 in Acute B Cell Lymphoblastic Leukemia: Mechanism, Function, and Implication for a Potential Novel Therapeutic Target. Exp Hematol (2014) 42(308):307–16. doi: 10.1016/j.exphem.2014.01.005
41. Cui W, Kong NR, Ma Y, Amin HM, Lai R, Chai L. Differential Expression of the Novel Oncogene, SALL4, in Lymphoma, Plasma Cell Myeloma, and Acute Lymphoblastic Leukemia. Mod Pathol (2006) 19:1585–92. doi: 10.1038/modpathol.3800694
42. Ma Y, Cui W, Yang J, Qu J, Di C, Amin HM, et al. SALL4, a Novel Oncogene, Is Constitutively Expressed in Human Acutemyeloid Leukemia (AML) and Induces AML in Transgenic Mice. Blood (2006) 108:2726–35. doi: 10.1182/blood-2006-02-001594
43. Wang F, Guo Y, Chen Q, Yang Z, Ning N, Zhang Y, et al. Stem Cell Factor SALL4, a Potential Prognostic Marker Formyelodysplastic Syndromes. J Hematol Oncol (2013) 6:73. doi: 10.1186/1756-8722-6-73
44. Kobayashi D, Kuribayshi K, Tanaka M, Watanabe N. SALL4 Is Essential for Cancer Cell Proliferation and is Overexpressed at Early Clinical Stages in Breast Cancer. Int J Oncol (2011) 38:933–9. doi: 10.3892/ijo.2011.929
45. Lu J Y, Ma Y, Kong N, Alipio Z, Gao C, Krause DS, et al. Dissecting the Role of SALL4, a Newly Identified Stem Cell Factor, in Chronic Myelogenous Leukemia. Leukemia (2011) 25:1211–3. doi: 10.1038/leu.2011.65
46. Kobayashi D, Kuribayashi K, Tanaka M, Watanabe N. Overexpression of SALL4 in Lung Cancer and Its Importance in Cell Proliferation. Oncol Rep (2011) 26:965–70. doi: 10.3892/or.2011.1374
47. Fujimoto M, Sumiyoshi S, Yoshizawa A, Sonobe M, Kobayashi M, Moriyoshi K, et al. SALL4 Immunohistochemistry in non-Small-Cell Lung Carcinomas. Histopathology (2014) 64:309–11. doi: 10.1111/his.12241
48. Li A, Jiao Y, Yong KJ, Wang F, Gao C, Yan B, et al. SALL4 Is a New Target in Endometrial Cancer. Oncogene (2013) 34(1):63–72. doi: 10.1038/onc.2013.529
49. Oikawa T, Kamiya A, Zeniya M, Chikada H, Hyuck AD, Yamazaki Y, et al. Sal-Like Protein 4 (SALL4), a Stem Cell Biomarker in Liver Cancers. Hepatology (2013) 57:1469–83. doi: 10.1002/hep.26159
50. Zeng SS, Yamashita T, Kondo M, Nio K, Hayashi T, Hara Y, et al. The Transcription Factor SALL4 Regulates Stemness of EpCAM-Positive Hepatocellular Carcinoma. J Hepatol (2014) 60:127–34. doi: 10.1016/j.jhep.2013.08.024
51. Zhang L, Xu Z, Xu X, Zhang B, Wu H, Wang M, et al. SALL4, a Novel Marker for Human Gastric Carcinogenesis and Metastasis. Oncogene (2013) 33(48):5491–500. doi: 10.1038/onc.2013.495
52. Rigter LS, Schaapveld M, Janus CPM, Krol ADG, van der Maazen RWM, Roesink J, et al. Overall and Disease-Specific Survival of Hodgkin Lymphoma Survivors Who Subsequently Developed Gastrointestinal Cancer. Cancer Med (2018) 8(1):190–9. doi: 10.1002/cam4.1922
53. Forghanifard MM, Moghbeli M, Raeisossadati R, Tavassoli A, Mallak AJ, Boroumand–Noughabi S, et al. Role of SALL4 in the Progression and Metastasis of Colorectal Cancer. J Biomed Sci (2013) 20:6. doi: 10.1186/1423-0127-20-6
54. Zhang L, Yan Y, Jiang Y, Cui Y, Zou Y, Qian J, et al. The Expression of SALL4 in Patients With Gliomas: High Level of SALL4 Expression Is Correlated With Poor Outcome. J Neurooncol (2014) 121(2):261–8. doi: 10.1007/s11060-014-1646-4
55. Miettinen M, Wang Z, McCue M, Sarlomo–Rikala M, Rys J, Biernat W, et al. SALL4 Expression in Germ Cell and Non-Germ Cell Tumors: A Systematic Immunohistochemical Study of 3215 Cases. Am J Surg Pathol (2014) 38:410–20. doi: 10.1097/PAS.0000000000000116
56. Wang F, Liu A, Peng Y, Rakheja D, Wei L, Xue D, et al. Diagnostic Utility of SALL4 in Extragonadal Yolk Sac Tumors: An Immunohistochemical Study of 59 Cases With Comparison to Placental-Like Alkaline Phosphatase, Alpha-Fetoprotein, and Glypican-3. Am J Surg Pathol (2009) 33:1529–39. doi: 10.1097/PAS.0b013e3181ad25d5
57. Ansieau S, Morel AP, Hinkal G, Bastid J, Puisieux A. Twisting an Embryonic Transcription Factor Into an Oncoprotein. Oncogene (2010) 29:3173–84. doi: 10.1038/onc.2010.92
58. Martin TA, Goyal A, Watkins G, Jiang WG. Expression of the Transcription Factors Snail, Slug, and Twist and Their Clinical Significance in Human Breast Cancer. Ann Surg Oncol (2005) 12:488–96. doi: 10.1245/ASO.2005.04.010
59. Song LB, Liao WT, Mai HQ, Zhang HZ, Zhang L, Li MZ, et al. The Clinical Significance of Twist Expression in Nasopharyngeal Carcinoma. Cancer Lett (2006) 242:258–65. doi: 10.1016/j.canlet.2005.11.013
60. Kajiyama H, Hosono S, Terauchi M, Shibata K, Ino K, Yamamoto E, et al. Twist Expression Predicts Poor Clinical Outcome of Patients With Clear Cell Carcinoma of the Ovary. Oncology (2006) 71:394– 401. doi: 10.1159/000107108
61. Shibata K, Kajiyama H, Ino K, Terauchi M, Yamamoto E, Nawa A, et al. Twist Expression in Patients With Cervical Cancer Is Associated With Poor Disease Outcome. Ann Oncol (2008) 19:81–5. doi: 10.1093/annonc/mdm344
62. Werner H. Tumor Suppressors Govern Insulin-Like Growth Factor Signaling Pathways: Implications in Metabolism and Cancer. Oncogene (2012) 31:2703–14. doi: 10.1038/onc.2011.447
63. Baserga R. The IGF-I Receptor in Cancer Research. Exp Cell Res (1999) 253:1–6. doi: 10.1006/excr.1999.4667
64. Raju U, Molkentine D, Valdecanas DR, Deorukhkar A, Mason KA, Buchholz TA, et al. Inhibition of EGFR or IGF-1R Signaling Enhances Radiation Response in Head and Neck Cancer Models But Concurrent Inhibition has No Added Benefit. Cancer Med (2014) 4:65–74. doi: 10.1002/cam4.345
65. Samani AA, Yakar S, LeRoith D, Brodt P. The Role of the IGF System in Cancer Growth and Metastasis: Overview and Recent Insights. Endocrine Rev (2007) 28:20–47. doi: 10.1210/er.2006-0001
66. Fernandez MC, Martin A, Venara M, Calcagno MdeL, Sansó G, Quintana S, et al. Overexpression of the Insulin-Like Growth Factor 1 Receptor (IGF-1R) Is Associated With Malignancy in Familial Pheochromocytomas and Paragangliomas. Clin Endocrinol (2013) 79:623–30. doi: 10.1111/cen.12205
67. Resnicoff M, Tjuvajev J, Rotman HL, Abraham D, Curtis M, Aiken R, et al. Regression of C6 Rat Brain Tumors by Cells Expressing an Antisense Insulinlike Growth Factor I Receptor RNA. J Exp Ther Oncol (1996) 1:385–9.
68. Cohen BD, Baker DA, Soderstrom C, Tkalcevic G, Rossie AM, Miller P, et al. Combination Therapy Enhances the Inhibition of Tumor Growth With the Fully Human Anti-Type 1 Insulin-Like Growth Factor Receptor Monoclonal Antibody CP-751,871. Clin Cancer Res (2005) 11:2063–73. doi: 10.1158/1078-0432.CCR-04-1070
69. Beadling C, Patterson J, Justusson E, Nelson D, Pantaleo MA, Hornick JL, et al. Gene Expression of the IGF Pathway Family Distinguishes Subsets of Gastrointestinal Stromal Tumors Wild Type for KIT and PDGFRA. Cancer Med (2013) 2:21–31. doi: 10.1002/cam4.57
70. Wang Y, Hailey J, Williams D, Wang Y, Lipari P, Malkowski M, et al. Inhibition of Insulin-Like Growth Factor-I Receptor (IGF-IR) Signaling and Tumor Cell Growth by a Fully Human Neutralizing anti-IGF-IR Antibody. Mol Cancer Ther (2005) 4:1214–21. doi: 10.1158/1535-7163.MCT-05-0048
71. Sachdev D, Hartell JS, Lee AV, Zhang X, Yee D. A Dominant Negative Type I Insulin-Like Growth Factor Receptor Inhibits Metastasis of Human Cancer Cells. J Biol Chem (2004) 279:5017–24. doi: 10.1074/jbc.M305403200
72. de Bono JS, Attard G, Adjei A, Pollak MN, Fong PC, Haluska P, et al. Potential Applications for Circulating Tumor Cells Expressing the Insulin-Like Growth Factor-I Receptor. Clin Cancer Res (2007) 13:3611–6. doi: 10.1158/1078-0432.CCR-07-0268
73. Plymate SR, Haugk K, Coleman I, Woodke L, Vessella R, Nelson P, et al. An Antibody Targeting the Type I Insulin-Like Growth Factor Receptor Enhances the Castration-Induced Response in Androgen-Dependent Prostate Cancer. Clin Cancer Res (2007) 13:6429–39. doi: 10.1158/1078-0432.CCR-07-0648
74. De Martino MC, Richard A, Feelders Dogan F, Van–koetsveld P, Krijger RD, Janssen J, et al. Expression of IGF/mTOR Pathway Components in Human Pheochromocytomas and In Vitro Inhibition of PC12 Rat Pheochromocytoma Cell Growth by mTOR Inhibitors Alone and in Combination With the Dual IGFI-R/INS-R Antagonist OSI-906. Endocrine Abstracts (2014) 35:P62. doi: 10.1530/endoabs.35.P62
75. Wells A. EGF Receptor. Int J Biochem Cell Biol (1999) 31:637–43. doi: 10.1016/S1357-2725(99)00015-1
76. Bareschino MA, Schettino C, Troiani T, Martinelli E, Morgillo F, Ciardiello F. Erlotinib in Cancer Treatment. Ann Oncol (2007) 18:35–41. doi: 10.1093/annonc/mdm222
77. Giaccone G, Gonzalez-Larriba JL, Van Oosterom AT, Alfonso R, Smit EF, Martens M, et al. Combination Therapy With Gefitinib, an Epidermal Growth Factor Receptor Tyrosine Kinase Inhibitor, Gemcitabine and Cisplatin in Patients With Advanced Solid Tumors. Ann Oncol (2004) 15:831–8. doi: 10.1093/annonc/mdh188
78. Petrelli F, Borgonovo K, Cabiddu M, Ghilardi M, Barni S. Cetuximab and Panitumumab in KRAS Wild-Type Colorectal Cancer: A Meta-Analysis. Int J Colorectal Dis (2011) 26:823–33. doi: 10.1007/s00384-011-1149-0
79. Petrelli F, Barni S. Anti-EGFR—targeting Agents in Recurrent or Metastatic Head and Neck Carcinoma: A Meta-Analysis. Head Neck (2011) 34(11):1657–64. doi: 10.1002/hed.21858
80. Rocha-Lima CM, Soares HP, Raez LE, Singal R. EGFR Targeting of Solid Tumors. Cancer. Contr (2007) 14:295–304. doi: 10.1177/107327480701400313
81. Fukuoka M, Yano S, Giaccone G, Tamura T, Nakagawa K, Douillard JY, et al. Multi-Institutional Randomized Phase II Trial of Gefitinib for Previously Treated Patients With Advanced Non-Small-Cell Lung Cancer (the IDEAL 1 Trial). J Clin Oncol (2003) 21:2237–46. doi: 10.1200/JCO.2003.10.038
82. Kris MG, Natale RB, Herbst RS, Lynch TJ Jr, Prager D, Belani CP, et al. Efficacy of Gefitinib, an Inhibitor of the Epidermal Growth Factor Receptor Tyrosine Kinase, in Symptomatic Patients With Non-Small Cell Lung Cancer: A Randomized Trial. JAMA (2003) 290:2149–58. doi: 10.1001/jama.290.16.2149
83. Wakeling AE, Barker AJ, Davies DH, Brown DS, Green LR, Cartlidge SA, et al. Specific Inhibition of Epidermal Growth Factor Receptor Tyrosine Kinase by 4-Anilinoquinazolines. Breast Cancer Res Treat (1996) 38:67–73. doi: 10.1007/BF01803785
84. Moulder SL, Yakes FM, Muthuswamy SK, Bianco R, Simpson JF, Arteaga CL. Epidermal Growth Factor Receptor (HER1) Tyrosine Kinase Inhibitor ZD1839 (Iressa) Inhibits HER2/neu (erbB2)-Overexpressing Breast Cancer Cells In Vitro and In Vivo. Cancer Res (2001) 61:8887–95.
85. Normanno N, Maiello MR, De LA. Epidermal Growth Factor Receptor Tyrosine Kinase Inhibitors (EGFR-TKIs): Simple Drugs With a Complex Mechanism of Action? J Cell Physiol (2003) 194:13–9. doi: 10.1002/jcp.10194
86. Moyer JD, Barbacci EG, Iwata KK, Arnold L, Boman B, Cunningham A, et al. Induction of Apoptosis and Cell Cycle Arrest by CP-358,774, an Inhibitor of Epidermal Growth Factor Receptor Tyrosine Kinase. Cancer Res (1997) 57:4838–48.
Keywords: pheochromocytoma, prognosis, molecular subtype, mutation, subtype specific treatment
Citation: Saddozai UAK, Wang F, Akbar MU, Zhang L, An Y, Zhu W, Xie L, Li Y, Ji X and Guo X (2021) Identification of Clinical Relevant Molecular Subtypes of Pheochromocytoma. Front. Endocrinol. 12:605797. doi: 10.3389/fendo.2021.605797
Received: 13 September 2020; Accepted: 10 May 2021;
Published: 21 June 2021.
Edited by:
Alfred King-yin Lam, Griffith University, AustraliaReviewed by:
David Vaudry, Institut National de la Santé et de la Recherche Médicale (INSERM), FranceMd Farhadul Islam, Griffith University, Australia
Copyright © 2021 Saddozai, Wang, Akbar, Zhang, An, Zhu, Xie, Li, Ji and Guo. This is an open-access article distributed under the terms of the Creative Commons Attribution License (CC BY). The use, distribution or reproduction in other forums is permitted, provided the original author(s) and the copyright owner(s) are credited and that the original publication in this journal is cited, in accordance with accepted academic practice. No use, distribution or reproduction is permitted which does not comply with these terms.
*Correspondence: Yongqiang Li, liyongqiang@vip.henu.edu.cn; Xinying Ji, 10190096@vip.henu.edu.cn; Xiangqian Guo, xqguo@henu.edu.cn