- 1Department of General Surgery, Fourth Affiliated Hospital of China Medical University, Shenyang, China
- 2Department of Colorectal and Hernia Minimally Invasive Surgery, Shengjing Hospital of China Medical University, Shenyang, China
- 3Department of General Surgery, The Third Hospital of Shenyang Medical College, Shenyang, China
Background: As the incidence of nonalcoholic fatty liver disease (NAFLD) increases globally, nonalcoholic steatohepatitis (NASH) has become the second common cause of liver transplantation for liver diseases. Recent evidence shows that Roux-en-Y gastric bypass (RYGB) surgery obviously alleviates NASH. However, the mechanism underlying RYGB induced NASH improvement is still elusive.
Methods: We obtained datasets, including hepatic gene expression data and histologic NASH status, at baseline and 1 year after RYGB surgery. Differentially expressed genes (DEGs) were identified comparing gene expression before and after RYGB surgery in each dataset. Common DEGs were obtained between both datasets and further subjected to functional and pathway enrichment analysis. Protein–protein interaction (PPI) network was constructed, and key modules and hub genes were also identified.
Results: In the present study, GSE106737 and GSE83452 datasets were included. One hundred thirty common DEGs (29 up-regulated and 101 down-regulated) were identified between GSE106737 and GSE83452 datasets. KEGG analysis showed that mineral absorption, IL-17 signaling pathway, osteoclast differentiation, and TNF signaling pathway were significantly enriched. Based on the PPI network, IGF1, JUN, FOS, LDLR, TYROBP, DUSP1, CXCR4, ATF3, CXCL2, EGR1, SAA1, CTSS, and PPARA were identified as hub genes, and three functional modules were also extracted.
Conclusion: This study identifies the global gene expression change in the liver of NASH patients before and after RYGB surgery in a bioinformatic method. Our findings will contribute to the understanding of molecular biological changes underlying NASH improvement after RYGB surgery.
Introduction
In the past few decades, obesity and its comorbidities are becoming the leading causes of death around the world (1). Meanwhile, nonalcoholic fatty liver disease (NAFLD) has become the most prevalent chronic liver disease in the United States (2). NAFLD consists of a spectrum of pathological states ranging from simple steatosis to nonalcoholic steatohepatitis (NASH) (2). Epidemiological studies show that 59.1% of biopsy-proven NAFLD patients progress to NASH (2, 3). As the incidence of advanced liver diseases such as cirrhosis and hepatocarcinoma are significantly increased in NASH patients, NASH becomes the second common cause of liver transplantation for liver diseases and is still growing (2).
So far, bariatric surgery is the most effective approach to treat obesity, which can also alleviate its comorbidities, such as type II diabetes mellitus (T2DM), hypertension, and hyperlipidemia (4). Roux-en-Y gastric bypass (RYGB) and sleeve gastrectomy (SG) serve as the most prevalent bariatric procedures in the world (5). Now, RYGB is recognized as the gold standard bariatric procedure worldwide (6). Recently, a study showed that all NAFLD parameters improved after bariatric surgery. This effect is more significant in patients undergoing RYGB surgery than patients who undergo adjustable gastric banding (AGB) surgery (7). Evidence from the liver biopsy showed that hepatic steatosis, hepatocellular ballooning, lobular inflammation, fibrosis, and NAFLD score obviously improved 1 year after bariatric surgery (8). The remission rate of NASH is over 85% after bariatric surgery (8). However, the mechanism of bariatric surgery in NASH alleviation is still elusive.
RYGB surgery is thought to be the malabsorptive procedure that bypasses a great portion of the intestine leading to nutrient malabsorption and weight loss (9). In the past few decades, evidence shows that bariatric surgery contributes to weight loss at least partially through energy balance regulation, peripheral and central nervous system regulation, gastrointestinal absorption and secretion, and microbiota alteration (10). Although the therapeutic effect of bariatric surgery on NASH has been confirmed recently, the underlying mechanism is almost unknown.
Microarray technology is an essential tool to illustrate gene expression patterns in multiple diseases, which can help us understand the biology and molecular mechanisms of diseases more efficiently. Microarray technology has also been used in the liver of NASH patients and animal models to detect differentially expressed genes (DEGs), screen disease biomarkers, or find new therapeutic targets (11, 12). By searching the Gene Expression Omnibus (GEO), there exists only two human datasets comparing DEGs in the liver tissue of NASH patients before and after bariatric surgery (13, 14). As the bioinformation in these datasets have not been thoroughly mined. Thus, we performed a global transcriptome analysis using a bioinformatic approach to find pivotal genes which might mediate RYGB surgery induced NASH improvement. In addition, hub genes and functional modules were identified in the DEGs, and biological function and pathway annotation were also performed.
Material and Methods
Microarray Datasets Collection
The gene expression datasets were obtained from the Gene Expression Omnibus (GEO) database (http://www.ncbi.nlm.nih.gov/geo). Datasets must meet the main inclusion criteria: 1) Datasets assessed transcriptome information in the liver specimen of patients who undergone RYGB surgery; 2) Datasets included liver specimens during RYGB surgery and paired liver specimens collected through liver biopsy 1-year post-operation; 3) All cases should be pathological proven NASH before RYGB surgery and NASH regression 1 year post-operation; 4) Datasets must contain at least 10 cases and paired follow-ups. In the GSE106737 dataset, 21 cases matched the inclusion criteria. In the GSE83452 dataset, 16 cases matched the inclusion criteria. All microarray analysis in the GSE106737 and the GSE83452 were performed on the GPL16686 platform. All gene expression patterns were originated from the open-access GEO database, so our study did not require Ethics Committee approval.
Datasets Analysis
The Gene expression matrix and attached annotation document for GSE106737 and GSE83452 datasets were downloaded from the GEO database. GSE files were divided into Baseline (patients with pathological proven NASH during RYGB surgery) and Follow-up (same patients with NASH improvement 1 year after RYGB surgery) groups. All microarray data had already been corrected and normalized by the RMA method. Gene IDs in the matrix were annotated with gene symbols by R package through related annotation documents. Mean values were preserved if the gene symbols matched with multiple probes. The DEGs were detected between liver biopsies at baseline and 1 year after RYGB surgery in each microarray by limma (linear models for microarray) R package. In each dataset, |log fold change (FC)| > 0.5 and P-value < 0.05 were regarded as the threshold value to determine DEGs. Then, DEGs in each dataset were upload to Venn software online (http://bioinformatics.psb.ugent.be/webtools/Venn/) to get the common DEGs.
GO and KEGG Enrichment Analysis
Gene Ontology (GO) is a recognized method to annotate the function of genes detected through high throughput transcriptomic or genomic data. Kyoto Encyclopedia of Genes and Genomes (KEGG) is a database resource including genomes, diseases, biological pathways, and other bioinformation. In this study, Bioconductor clusterProfiler package was used to carried out GO and KEGG analysis for common DEGs (15). Biological process (BP), cellular component (CC), molecular function (MF), and pathways that were significantly enriched were screened out when the P-value < 0.05.
PPI Network Construction and Module Analysis
The Search Tool for the Retrieval of Interacting Genes/Proteins (STRING, https://string-db.org/) (version 11) is a frequently used software online to predict the interaction between proteins and proteins (16). In this study, the PPI network was predicted by STRING online database setting the cut-off value 0.04. Then, the PPI networks were visualized and analyzed with Cytoscape software (version 3.8.0). Hub genes were identified using CytoHubba plug-in APP in Cytoscape with the cut-off value degree value >10. Vital modules in the PPI network were clustered using the Molecular Complex Detection (MCODE) plug-in APP in Cytoscape (17). The cut-off value was MCODE score ≥ 5.
Results
Information of Selected Cases
Initially, GSE106737 and GSE83452 were included according to the inclusion criteria. However, to obtain high-quality data, we further screen both datasets to get cases that match inclusion criteria (details described in “Material and Methods”). There existed 37 Baseline (patients with pathological proven NASH during RYGB surgery) and 37 paired Follow-up (same patients with NASH improvement 1 year after RYGB surgery) for further analysis. The detailed information of included cases was shown in Table 1 and Supplementary Tables 1 and 2.
Identification of DEGs Between Baseline and Follow-Up
All selected cases in GSE106737 and GSE83452 datasets were standardized to eliminate individual differences. Each dataset was homogeneous after standardization. We screened out 132 DEGs in GSE106737. Among these DEGs, 31 genes were up-regulated, and 101 genes were down-regulated. In GSE83452, we identified 206 DEGs in which 38 genes were up-regulated, and 168 genes were down-regulated. The volcano plots and heatmaps of both GSE106737 and GSE83452 were shown in Figures 1 and 2, respectively.
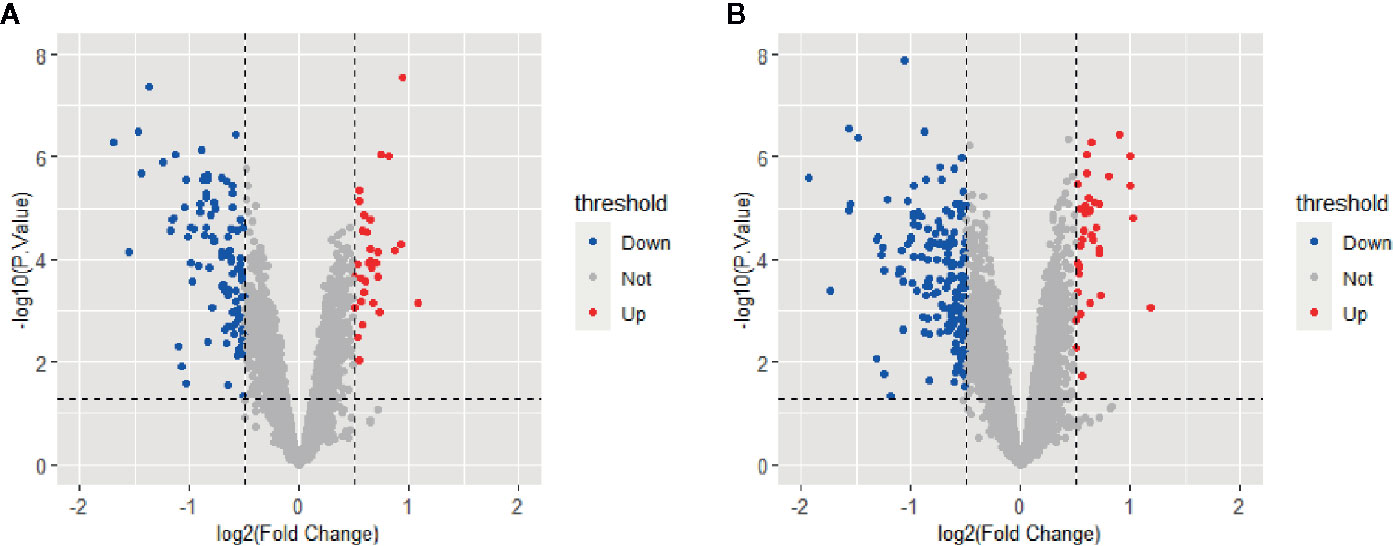
Figure 1 Volcano plots of gene expression in GSE106737 (A) and GSE83452 (B), with the threshold of P<0.05 and |log FC| > 0.5. Blue points represented down-regulated genes, red points represented up-regulated genes, and gray points represented genes with on significant difference.
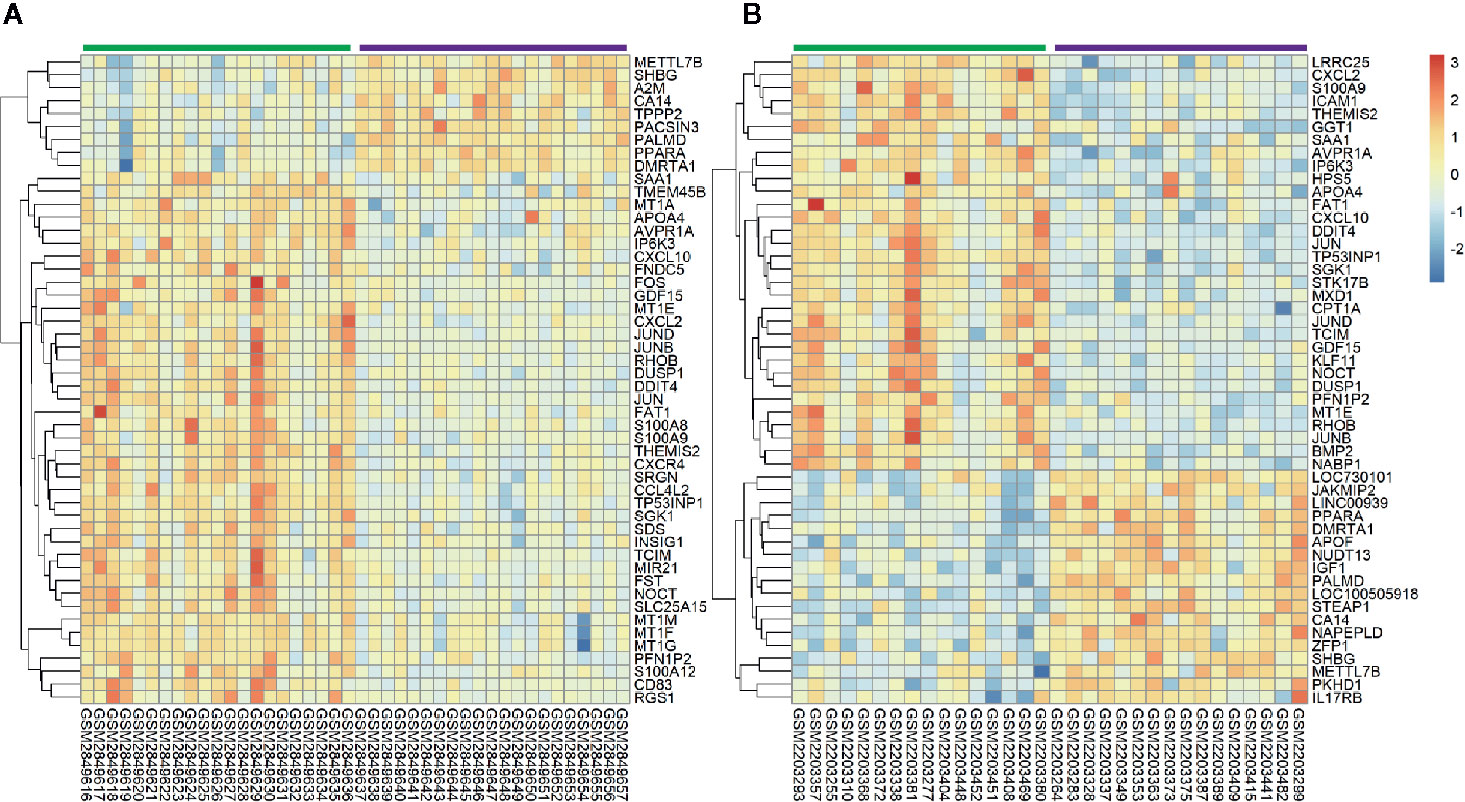
Figure 2 Heatmaps of top 50 genes expression in GSE106737 (A) and GSE83452 (B). Blue square represented down-regulated genes and red square represented up-regulated genes. The green bar represented baseline gene expression and the purple bar represented follow-up gene expression.
Identification of Common DEGs Between GSE106737 and GSE83452
DEGs in both GSE106737 and GSE83452 datasets were analyzed using the Venn diagram. We obtain 130 common DEGs between two datasets, in which 29 DEGs were up-regulated (logFC > 0) and 101 DEGs were down-regulated (logFC < 0). Venn diagram was shown in Figure 3, and the details of common DEGs were shown in Table 2.
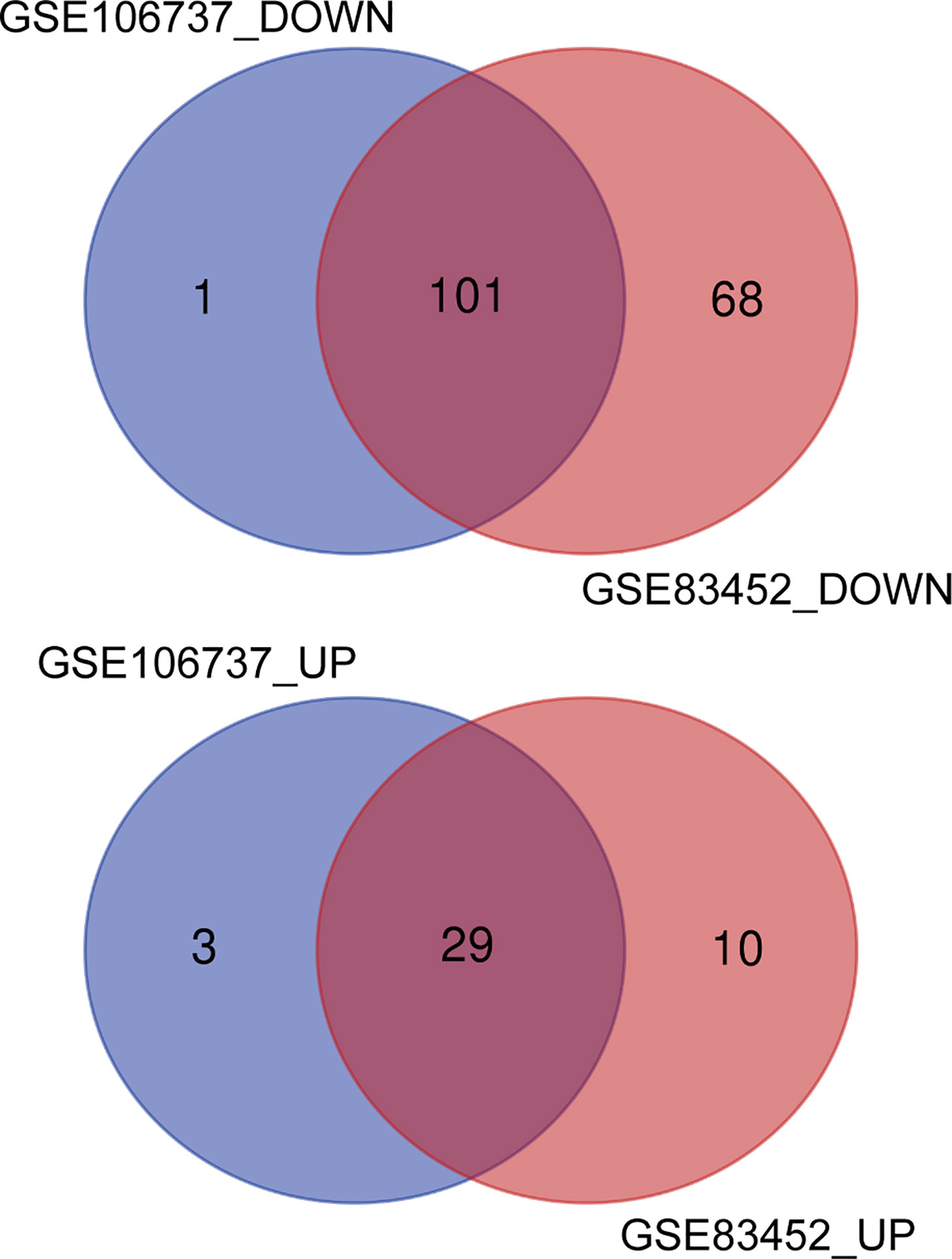
Figure 3 Identification of common DEGs from GSE106737 and GSE83452 using the Venn diagram. The cross section represented the common DEGs. DOWN, down-regulated DEGs; UP, up-regulated DEGs.

Table 2 130 common DEGs in NASH patients with paired liver specimens at baseline and 1-year post-operation.
GO and KEGG Enrichment Analysis
All common DEGs were analyzed by the clusterProfiler package to illustrate the biological functions and pathways related to DEGs. The results demonstrated that cellular zinc ion homeostasis process (P-value= 5.97E-13) was the most significantly enriched biological process (BP), followed by cellular response to cadmium ion process (P-value= 7.84E-13), zinc ion homeostasis process (P-value= 1.02E-12), and so on. Cell component (CC) and metabolic function (MF) of DEGs were mainly related to lipoprotein homeostasis and inflammatory signaling pathway, respectively. The detailed information of GO analysis was shown in Figure 4. KEGG pathway analysis indicated that DEGs are mainly related to mineral absorption pathway (P-value= 3.75E-08), followed by IL-17 signaling pathway (P-value= 1.11E-07), osteoclast differentiation (P-value= 1.23E-04), etc. The top 10 pathways of KEGG analysis were shown in Figure 4.
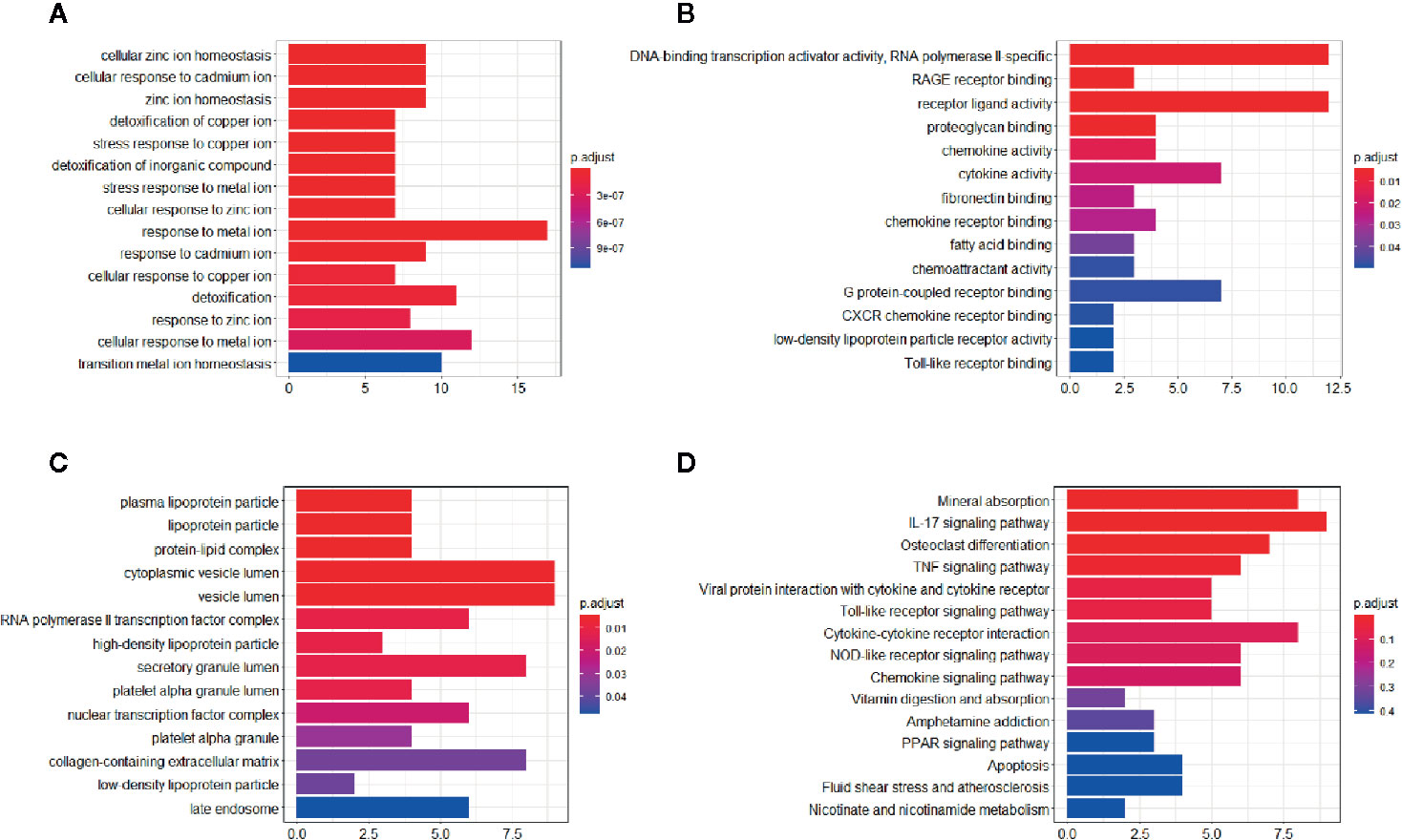
Figure 4 GO and KEGG enrichment analysis of the common DEGs. (A) Significant enriched biological process (BP) in GO terms. (B) Significant enriched cell component (CC) in GO terms. (C) Significant enriched molecular function (MF) in GO terms. (D) Significant enriched KEGG pathway in common DEGs.
PPI Network Construction and Hub Genes Identification
The PPI network of the common DEGs was constructed using the STRING online database and further analyzed and visualized using Cytoscape software. In the PPI network, there existed 93nodes, including 12 up-regulated genes and 81 down-regulated genes. Among 130 common DEGs, 37 genes including 17 up-regulated genes and 20 down-regulated genes were excluded from the PPI network. The term “degree” calculated using CytoHubba plug-in APP in Cytoscape indicated the number of interactions between genes or nodes. The PPI network was shown in Figure 5, the color depth positively correlated with the degree value. Setting the cut-off value of degree>10, IGF1, JUN, FOS, LDLR, ATF3, TYROBP, DUSP1, CXCR4, CXCL2, EGR1, SAA1, PPARA, and CTSS were identified as the hub genes. Among 13 hub genes, IGF1 and PPARA were up-regulated, and other genes were down-regulated.
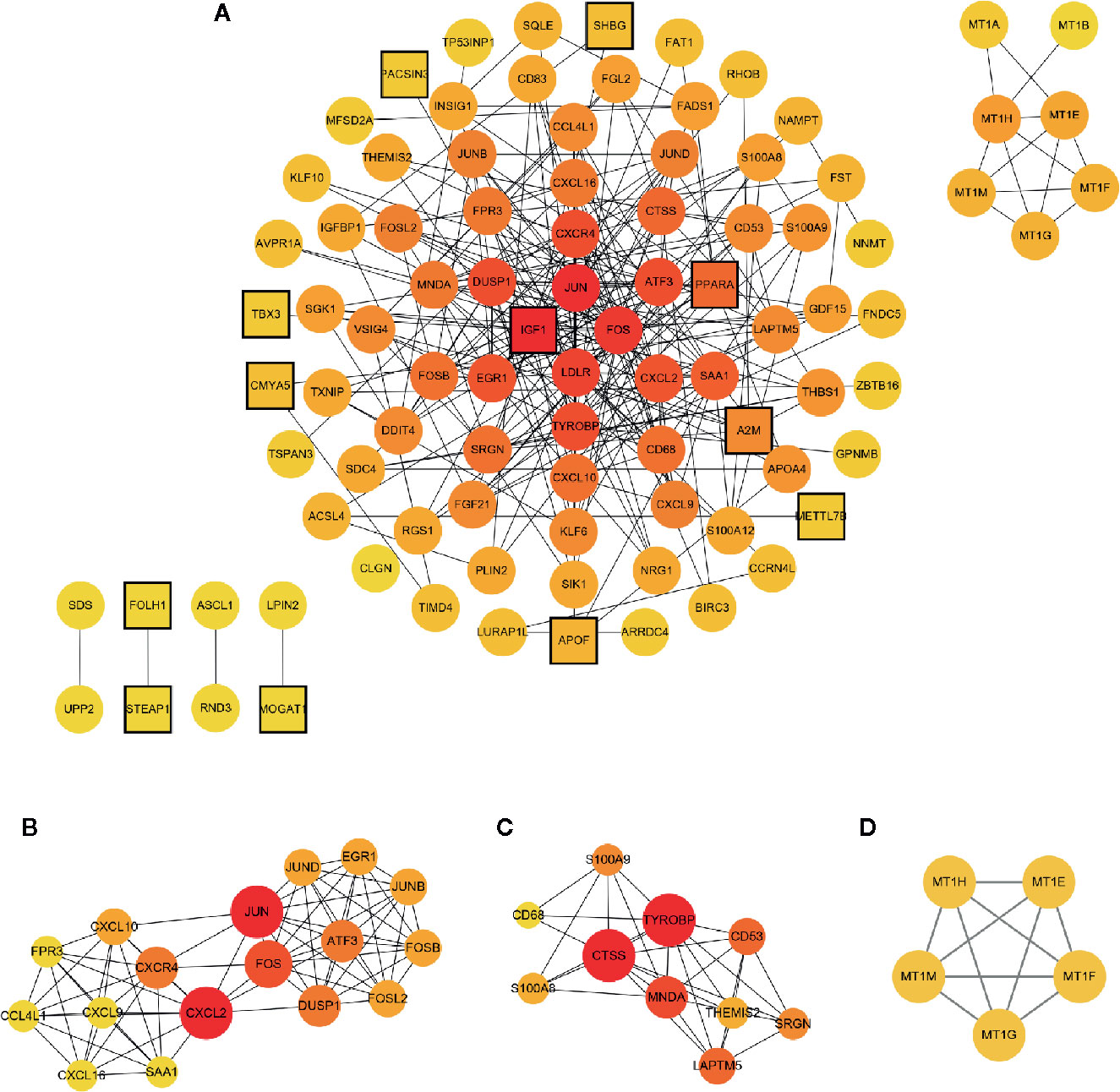
Figure 5 PPI network of the common DEGs and module exhibition. (A) The PPI network consisted of 93 genes. (B–D) Modules 1–3 which were extracted from the PPI network. The circle represented down-regulated genes, while the square with black border represented up-regulated genes. The depth of color correlated with the number of connections between nodes. In (B–D), the size of the circle correlated with the number of connections between nodes in each module.
Module Analysis of the PPI Network
Modules were extracted using MCODE plug-in APP in Cytoscape. Setting the threshold MCODE score ≥ 5, we obtained 3 modules from the PPI network, including Module 1 (MCODE Score=8.875, Nodes=17), Module 2 (MCODE Score=6.444, Nodes=10), and Module 3 (MCODE Score=5, Nodes=5). All genes belonged to these three modules were down-regulated common DEGs. Modules were shown in Figure 5. The genes in each module were analyzed using the clusterProfiler package to identify their GO and KEGG enrichment. Module 1 was mainly enriched in the IL-17 signaling pathway, osteoclast differentiation pathway, and chemokine signaling pathway. Module 2 was mainly involved in the lysosome pathway, IL-17 signaling pathway, and antigen processing and presentation pathway. Module 3 take part in the mineral absorption pathway. The detailed information was shown in Table 3.
Discussion
In the past few decades, NAFLD has become the most common chronic liver disease around the world (18, 19). Although NAFLD is a benign disease, NASH (a progressive stage of NAFLD) is the second common cause for liver transplantation and increases hepatocarcinoma progression (2). So far, bariatric surgery seems to be the most long-lasting effective method to treat NASH (7, 8). However, due to the safety issue, the American Association for the Study of Liver Diseases has not recommended bariatric surgery to specifically treat NASH (20). However, it can serve as an option for obese patients (BMI ≥35 kg/m2) with one or more complications remediable by weight loss, including NAFLD and NASH (21). Nowadays, less invasive and safer modern interventions are needed to replace invasive bariatric procedures. So, it is urgently needed to illustrate the mechanism underlying bariatric surgery in NASH improvement. Microarray technology is an efficient method to get transcriptomic bioinformation under various diseases. In this study, we included two microarray studies comparing gene expression profiles between Baseline (liver specimens obtained during RYGB surgery) and Follow-up (liver specimens obtained 1 year after RYGB surgery) to get DEGs. Furthermore, we perform functional annotation and construct PPI network to illustrate the biological function and involved pathways of the DEGs.
Although the GSE106737 dataset had been already used by others, their research was focused on the function of CD8 T cells in the progression and remission process in NASH (14). The study using the GSE83452 dataset included a wide range of patients with NASH or fibrosis and focused on single gene interpretation (13). The present research strictly selected cases which verified NASH improvement after RYGB surgery to interpret the hepatic global transcriptomic change underlying this process. In the present study, we obtained 130 common DEGs between GSE106737 and GSE83452 datasets (cases selected strictly matched inclusion criteria). Among these DEGs, 29 genes were up-regulated, and 101 genes were down-regulated. PPI network included 93 genes and most of them belonged to the down-regulated DEGs. In the PPI network, IGF1, JUN, FOS, LDLR, TYROBP, DUSP1, CXCR4, ATF3, CXCL2, EGR1, SAA1, CTSS, and PPARA were identified as the hub genes and ranked by the degree value using CytoHubba plug-in APP in Cytoscape. Among 13 hub genes, only IGF1 and PPARA were up-regulated, while others were down-regulated. In consistence with this conclusion, a previous study had demonstrated that PPARα activation might be the mechanism underlying NASH improvement after RYGB surgery (13, 22).
Furthermore, using the MCODE plug-in APP in Cytoscape, we got three modules in the PPI network. Seventeen genes were included in Module 1, among which, JUN, FOS, DUSP1, CXCR4, ATF3, CXCL2, EGR1, and SAA1 severed as hub genes. These genes participated in the IL-17 signaling pathway, osteoclast differentiation pathway, chemokine signaling pathway, viral protein interaction with cytokine and cytokine receptor pathway, etc. Activator protein 1 (AP-1), which was combined with Jun (c-Jun, JunB, and JunD), Fos (c-Fos, FosB, Fra-1, and Fra-2), activating transcription factor (Atf) and musculoaponeurotic fibrosarcoma (Maf) proteins to form homodimer or heterodimer is a dimeric leucine zipper (bZIP) transcription factor (23). Generally, the AP-1 complex played a pivotal role in acute stress response in the liver (24). Recent evidence showed that c-Jun/AP-1 overexpression might mediate the hepatic pathological alteration in NASH patients (25). Furthermore, AP-1 correlated with hepatic lipid metabolism and NASH progression through regulating PPARγ expression (26). CXCL and CCL are chemokines with chemotactic properties and CXCR is one type of G protein-coupled chemokine receptors (27). Chemokines participate in homeostatic or inflammatory regulation which mediates physiological or pathophysiological alteration in disease progression through binding corresponding receptors (28). CXCL2 and CXCL8 chemokines were mainly originated from activated Kupffer cells (29). CXCL2 and CXCL8 with neutrophil chemotactic properties recruit neutrophils, releasing reactive oxygen species (ROS) and proteases and therefore initiating hepatocyte necrosis (30). CXCL9, CXCL10, and CXCL 16 attract lymphocytes or natural killer (NK) cells involved in hepatic inflammatory pathogenesis and accelerate hepatic fibrosis progression (31). CXCR4 is the corresponding receptor of CXCL12. In the liver of NASH patients, the affinity of CXCR4 significantly increased which increased CD4+ T-cells deposition (32). DUSPs, namely, dual-specificity protein tyrosine phosphatases served as mitogen-activated protein kinases (MAPK) inactivator (33). DUSPs as MAPK phosphatases (MKPs) dephosphorylated the threonine and tyrosine residues of MAPK and played a pivotal role in hepatic metabolic regulation (34). MKP-1, a negative regulator of p38 MAPK and c-Jun NH2-terminal kinase 1/2 (JNK1/2), activated transcription factors regulating hepatic lipid homeostasis (35). Studies showed that MKP-1 deficient protected mice from diet-induced obesity and diet or gene induced hepatic steatosis (35, 36).EGR1, namely, early growth response 1, played an essential role in the pathophysiological process of inflammation and tissue repairment (37). A previous bioinformatic study identified that the lower expression of hepatic EGR1 might promote NAFLD development (38). Evidence from both in vivo and in vitro experiments also confirmed the function of EGR1 in hepatic insulin response and hepatic lipid metabolic regulation (37). SAA1, namely, serum amyloid A-1 protein belongs to the SAA family (39). As a classical acute-phase protein produced by hepatocytes, SAA mediated infection, injury, and inflammation response and could regulate toll-like receptor 4 (TLR4), which participated in obesity-induced insulin resistance (40). In progressive liver diseases, including NASH, the serum level of SAA could serve as a biomarker for inflammatory status (41). Moreover, SAA1 had the ability to stimulate NF-κBp65 protein transportation from the cytoplasm to the nucleus and activate the NF-κB pathway which mediated NASH progression (40).
Module 2 included TYROBP, CD68, THEMIS2, MNDA, S100A9, S100A8, LAPTM5, CD53, SRGN, and CTSS, among which TYROBP and CTSS served as the hub genes in the PPI network. TYROBP, namely, Tyrosine kinase binding protein could activate NK cells through binding a variety of receptors (42). Studies showed that TYROBP could synthesize lipopolysaccharide and activated pro-inflammatory cytokines production (43). After TYROBP gene deletion, the pro-inflammatory cytokines decreased obviously, which indicated that TYROBP might be involved in NASH progression (44). In Module 2, CTSS, LAPTM5, and CD68 were involved in the lysosome pathway. CTSS and CD68 were both recognized as the molecular biomarker of macrophages (45). Moreover, CTSS and CD68 positively correlated with hepatic macrophage infiltration in NAFLD mice (45). Meanwhile, a recent bioinformatic study identified hepatic CTSS and CD68 as major genes contributing to NAFLD (46). Furthermore, we identified S100A9 and S100A8 genes in Module 2, which were involved in the IL-17 signaling pathway. As members of the S100 proteins, S100A8 and S100A9 could be secreted by either neutrophils or monocytes (47). S100A8, S100A9, and S100A8/S100A9 (heterodimer formed by S100A8 and S100A9) were implicated in various inflammatory diseases and could serve as the biomarker for inflammatory activity monitoring (48). It was worth noting that S100A8 and S100A9 had a direct link with inflammatory and fibrotic status in NASH patients (47).
Module 3 included MT1E, MT1F, MT1G, MT1H, and MT1M. Though none of them was identified as the hub gene, they were involved in the mineral absorption pathway. Metallothioneins (MTs), including MT1, MT2, MT3, and MT4 isoforms, played a pivotal role in heavy metal toxicity protection, metal homeostasis, and oxidative stress regulation (49). In MT1, there existed MT1A, MT1B, MT1E, MT1F, MT1G, MT1H, MT1M, and MT1X isoforms (49). These proteins mainly regulated copper and zinc homeostasis in the liver and protected hepatocytes from oxidative damage (50). Thus, MT1 expression negatively correlated with the damage status of chronic liver diseases (51). Moreover, MT1 could stimulate damaged hepatocyte repair and regeneration (52). However, we could not ignore the fact that mineral deficiency was a common complication after RYGB surgery. Data from the American Society for Metabolic and Bariatric Surgery (ASMBS) Integrated Health Nutritional Guidelines showed that the prevalence of zinc deficiency occurred in 40% post-RYGB patients and copper deficiency occurred in 10%–20% post-RYGB patients (53). Thus, we speculated that copper and zinc deficiency might be caused by the low hepatic MT1 expression after RYGB surgery. On the contrary, as MT1 protein expression was positively regulated by zinc and copper, the zinc and copper deficiency further suppressed MT1 expression and formed a vicious circle (54, 55). So, the mineral condition should be inspected strictly and supplements should be administrated if necessary after RYGB surgery.
Though we got much transcriptomic bioinformation underlying RYGB induced NASH improvement, the causes of these hepatic changes had not been fully clarified. Due to the lack of conclusive evidence, we speculated about the reason for inflammatory related genes alteration after RYGB surgery: 1) The gastrointestinal anatomy was altered after RYGB surgery, so more bile acid (BA) reach ileum and stimulate enteric GLP-1 and PYY release through activating the local L-cells (56). Furthermore, the increased BA also stimulated TGR5 expression in Kupffer cells (57). These BA induced metabolic change may lead to a reduction in hepatic pro-inflammatory genes expression after RYGB surgery. 2) RYGB surgery manipulate a variety of adipocyte derived adipocytokines (adiponectin, leptin, TNFα, IL-6, and etc.), which have been proven to be involved in the hepatic inflammatory process (58). For instance, RYGB surgery could significantly increase adiponectin levels, which suppress Kupffer cells and hepatic stellate cells (HSC) activation and decrease hepatic inflammatory genes expression (58, 59). 3) The gut microbiota composition obviously shifted after RYGB surgery, as the rearrangement of the gastrointestinal tract created a more acidic and oxygen-rich environment (60). Recent evidence showed that specific gut microbiota was independently associated with the severity of NAFLD (61). We speculated that gut microbiota shift after RYGB surgery might alleviate NASH through modulating hepatic inflammatory gene expression.
There existed several limitations of the present study, which should be noted. First and foremost, due to the difficulty in collecting the hepatic specimens after RYGB surgery, we did not validate the genes in the pathway highlighted by the present study. This limited the strength of our results in interpreting the mechanism underlying RYGB surgery induced NASH alleviation. Second, we could not get enough demographic and clinical information for the patients included in our study. Considering body weight, body mass index (BMI), and obesity related comorbidities such as type 2 diabetes mellitus were all risk factors for NASH. There existed confounding factors in the analysis in the present study. Third, hepatic tissue was the heterogeneous mixture of hepatocytes and mesenchymal cells, and the cell composition might be influenced during specimen collection. Finally, the metabolic change in the liver after bariatric surgery is a dynamic process. Analysis at a single time point after surgery ignored the full picture of the hepatic remodeling process.
Conclusion
In conclusion, in the present study, we exhibit the global profile of DEGs and corresponding signaling pathways, which may mediate RYGB surgery induced NASH improvement. In the process of RYGB surgery induced NASH improvement, the possible key genes are IGF1, JUN, FOS, LDLR, TYROBP, DUSP1, CXCR4, ATF3, CXCL2, EGR1, SAA1, CTSS, and PPARA, and the possible involved pathways are IL-17 signaling pathway, osteoclast differentiation pathway, chemokine signaling pathway, viral protein interaction with cytokine and cytokine receptor pathway, Toll-like receptor signaling pathway, TNF signaling pathway and mineral absorption pathway. Most DEGs and enriched signaling pathways are involved in the inflammatory response, immunoreaction, and lipid homeostatic regulation. These results provide pivotal transcriptome information underlying RYGB surgery induced NASH alleviation.
Data Availability Statement
The datasets presented in this study can be found in online repositories. The names of the repository/repositories and accession number(s) can be found in the article/Supplementary Material.
Ethics Statement
Ethical review and approval was not required for the study on human participants in accordance with the local legislation and institutional requirements.
Author Contributions
All authors listed have made a substantial, direct, and intellectual contribution to the work and approved it for publication.
Funding
This work was supported by the Support Program for Young and Middle-aged Scientific and Technological Innovation Talent (Grant No. RC200607); 2020 “Double First Class”: Support Plan for the Iconic Achievement of Clinical Medicine (Grant No. 3110200345).
Conflict of Interest
The authors declare that the research was conducted in the absence of any commercial or financial relationships that could be construed as a potential conflict of interest.
Supplementary Material
The Supplementary Material for this article can be found online at: https://www.frontiersin.org/articles/10.3389/fendo.2020.611213/full#supplementary-material
References
1. Fan JG, Kim SU, Wong VW. New trends on obesity and NAFLD in Asia. J Hepatol (2017) 67(4):862–73. doi: 10.1016/j.jhep.2017.06.003
2. Younossi Z, Anstee QM, Marietti M, Hardy T, Henry L, Eslam M, et al. Global burden of NAFLD and NASH: trends, predictions, risk factors and prevention. Nat Rev Gastroenterol Hepatol (2018) 15(1):11–20. doi: 10.1038/nrgastro.2017.109
3. Younossi ZM, Koenig AB, Abdelatif D, Fazel Y, Henry L, Wymer M. Global epidemiology of nonalcoholic fatty liver disease-Meta-analytic assessment of prevalence, incidence, and outcomes. Hepatology (2016) 64:73–84. doi: 10.1002/hep.28431
4. Ponce de León-Ballesteros G, Sánchez-Aguilar HA, Velázquez-Fernández D, Nava-Ponce T, Herrera MF. Roux-en-Y Gastric Bypass in Patients >60 Years of Age: Morbidity and Short-Term Outcomes. Obes Surg (2020) 30(12):5033–5040. doi: 10.1007/s11695-020-04957-8
5. Wu Q, Zhang X, Zhong M, Han H, Liu S, Liu T, et al. Effects of Bariatric Surgery on Serum Bile Acid Composition and Conjugation in a Diabetic Rat Model. Obes Surg (2016) 26(10):2384–92. doi: 10.1007/s11695-016-2087-2
6. Misra S, Nandhini BD, Christinajoice S, Kumar SS, Prabhakaran S, Palanivelu C, et al. Is Laparoscopic Roux-en-Y Gastric Bypass Still the Gold Standard Procedure for Indians? Mid- to Long-Term Outcomes from a Tertiary Care Center. Obes Surg (2020) 30(11):4482–93. doi: 10.1007/s11695-020-04849-x
7. Caiazzo R, Lassailly G, Leteurtre E, Baud G, Verkindt H, Raverdy V, et al. Roux-en-Y gastric bypass versus adjustable gastric banding to reduce nonalcoholic fatty liver disease: a 5-year controlled longitudinal study. Ann Surg (2014) 260(5):893–8; discussion 898-9. doi: 10.1097/SLA.0000000000000945
8. Lassailly G, Caiazzo R, Buob D, Pigeyre M, Verkindt H, Labreuche J, et al. Bariatric Surgery Reduces Features of Nonalcoholic Steatohepatitis in Morbidly Obese Patients. Gastroenterology (2015) 149(2):379–88; quiz e15-6. doi: 10.1053/j.gastro.2015.04.014
9. Haluzík M. Bariatric surgery and the mechanism of diabetes remission: are we getting there. J Clin Endocrinol Metab (2013) 98(11):4336–8. doi: 10.1210/jc.2013-3698
10. Mulla CM, Middelbeek R, Patti ME. Mechanisms of weight loss and improved metabolism following bariatric surgery. Ann N Y Acad Sci (2018) 1411(1):53–64. doi: 10.1111/nyas.13409
11. Murphy SK, Yang H, Moylan CA, Pang H, Dellinger A, Abdelmalek MF, et al. Relationship between methylome and transcriptome in patients with nonalcoholic fatty liver disease. Gastroenterology (2013) 145(5):1076–87. doi: 10.1053/j.gastro.2013.07.047
12. Moylan CA, Pang H, Dellinger A, Suzuki A, Garrett ME, Guy CD, et al. Hepatic gene expression profiles differentiate presymptomatic patients with mild versus severe nonalcoholic fatty liver disease. Hepatology (2014) 59(2):471–82. doi: 10.1002/hep.26661
13. Lefebvre P, Lalloyer F, Baugé E, Pawlak M, Gheeraert C, Dehondt H, et al. Interspecies NASH disease activity whole-genome profiling identifies a fibrogenic role of PPARα-regulated dermatopontin. JCI Insight (2017) 2(13):e92264. doi: 10.1172/jci.insight.92264
14. Haas JT, Vonghia L, Mogilenko DA, Verrijken A, Molendi-Coste O, Fleury S, et al. Transcriptional Network Analysis Implicates Altered Hepatic Immune Function in NASH development and resolution. Nat Metab (2019) 1(6):604–14. doi: 10.1038/s42255-019-0076-1
15. Yu G, Wang LG, Han Y, He QY. clusterProfiler: an R package for comparing biological themes among gene clusters. OMICS (2012) 16(5):284–7. doi: 10.1089/omi.2011.0118
16. Szklarczyk D, Morris JH, Cook H, Kuhn M, Wyder S, Simonovic M, et al. The STRING database in 2017: quality-controlled protein-protein association networks, made broadly accessible. Nucleic Acids Res (2017) 45(D1):D362–8. doi: 10.1093/nar/gkw937
17. Lin Y, Li J, Wu D, Wang F, Fang Z, Shen G. Identification of Hub Genes in Type 2 Diabetes Mellitus Using Bioinformatics Analysis. Diabetes Metab Syndr Obes (2020) 13:1793–801. doi: 10.2147/DMSO.S245165
18. Jia X, Zhai T. Integrated Analysis of Multiple Microarray Studies to Identify Novel Gene Signatures in Non-alcoholic Fatty Liver Disease. Front Endocrinol (Lausanne) (2019) 10:599. doi: 10.3389/fendo.2019.00599
19. Chen F, Zhou Y, Yang K, Shen M, Wang Y. NPY stimulates cholesterol synthesis acutely by activating the SREBP2-HMGCR pathway through the Y1 and Y5 receptors in murine hepatocytes. Life Sci (2020) 262:118478. doi: 10.1016/j.lfs.2020.118478
20. Chalasani N, Younossi Z, Lavine JE, Charlton M, Cusi K, Rinella M, et al. The diagnosis and management of nonalcoholic fatty liver disease: Practice guidance from the American Association for the Study of Liver Diseases. Hepatology (2018) 67(1):328–57. doi: 10.1002/hep.29367
21. Mechanick JI, Apovian C, Brethauer S, Timothy Garvey W, Joffe AM, Kim J, et al. Clinical Practice Guidelines for the Perioperative Nutrition, Metabolic, and Nonsurgical Support of Patients Undergoing Bariatric Procedures - 2019 Update: Cosponsored by American Association of Clinical Endocrinologists/American College of Endocrinology, The Obesity Society, American Society for Metabolic and Bariatric Surgery, Obesity Medicine Association, and American Society of Anesthesiologists. Obesity (Silver Spring) (2020) 28(4):O1–O58. doi: 10.1002/oby.22719
22. Mazzini GS, Khoraki J, Dozmorov M, Browning MG, Wijesinghe D, Wolfe L, et al. Concomitant PPARα and FXR Activation as a Putative Mechanism of NASH Improvement after Gastric Bypass Surgery: a GEO Datasets Analysis. J Gastrointest Surg (2019) 23(1):51–7. doi: 10.1007/s11605-018-3938-z
23. Halazonetis TD, Georgopoulos K, Greenberg ME, Leder P. c-Jun dimerizes with itself and with c-Fos, forming complexes of different DNA binding affinities. Cell (1988) 55(5):917–24. doi: 10.1016/0092-8674(88)90147-X
24. Fuest M, Willim K, MacNelly S, Fellner N, Resch GP, Blum HE, et al. The transcription factor c-Jun protects against sustained hepatic endoplasmic reticulum stress thereby promoting hepatocyte survival. Hepatology (2012) 55(2):408–18. doi: 10.1002/hep.24699
25. Dorn C, Engelmann JC, Saugspier M, Koch A, Hartmann A, Müller M, et al. Increased expression of c-Jun in nonalcoholic fatty liver disease. Lab Invest (2014) 94(4):394–408. doi: 10.1038/labinvest.2014.3
26. Hasenfuss SC, Bakiri L, Thomsen MK, Williams EG, Auwerx J, Wagner EF. Regulation of steatohepatitis and PPARγ signaling by distinct AP-1 dimers. Cell Metab (2014) 19(1):84–95. doi: 10.1016/j.cmet.2013.11.018
27. Wu F, Sun C, Lu J. The Role of Chemokine Receptors in Renal Fibrosis. Rev Physiol Biochem Pharmacol (2020) 177:1–24. doi: 10.1007/112_2020_21
28. Huynh C, Dingemanse J, Meyer Zu Schwabedissen HE, Sidharta PN. Relevance of the CXCR4/CXCR7-CXCL12 axis and its effect in pathophysiological conditions. Pharmacol Res (2020) 161:105092. doi: 10.1016/j.phrs.2020.105092
29. Zimmermann HW, Tacke F. Modification of chemokine pathways and immune cell infiltration as a novel therapeutic approach in liver inflammation and fibrosis. Inflamm Allergy Drug Targets (2011) 10(6):509–36. doi: 10.2174/187152811798104890
30. Marra F, Tacke F. Roles for chemokines in liver disease. Gastroenterology (2014) 147(3):577–594.e1. doi: 10.1053/j.gastro.2014.06.043
31. Holt AP, Haughton EL, Lalor PF, Filer A, Buckley CD, Adams DH. Liver myofibroblasts regulate infiltration and positioning of lymphocytes in human liver. Gastroenterology (2009) 136(2):705–14. doi: 10.1053/j.gastro.2008.10.020
32. Boujedidi H, Robert O, Bignon A, Cassard-Doulcier AM, Renoud ML, Gary-Gouy H, et al. CXCR4 dysfunction in non-alcoholic steatohepatitis in mice and patients. Clin Sci (Lond) (2015) 128(4):257–67. doi: 10.1042/CS20130833
33. Boutros T, Chevet E, Metrakos P. Mitogen-activated protein (MAP) kinase/MAP kinase phosphatase regulation: roles in cell growth, death, and cancer. Pharmacol Rev (2008) 60(3):261–310. doi: 10.1124/pr.107.00106
34. Lawan A, Bennett AM. Mitogen-Activated Protein Kinase Regulation in Hepatic Metabolism. Trends Endocrinol Metab (2017) 28(12):868–78. doi: 10.1016/j.tem.2017.10.007
35. Flach RJ, Qin H, Zhang L, Bennett AM. Loss of mitogen-activated protein kinase phosphatase-1 protects from hepatic steatosis by repression of cell death-inducing DNA fragmentation factor A (DFFA)-like effector C (CIDEC)/fat-specific protein 27. J Biol Chem (2011) 286(25):22195–202. doi: 10.1074/jbc.M110.210237
36. Wu JJ, Roth RJ, Anderson EJ, Hong EG, Lee MK, Choi CS, et al. Mice lacking MAP kinase phosphatase-1 have enhanced MAP kinase activity and resistance to diet-induced obesity. Cell Metab (2006) 4(1):61–73. doi: 10.1016/j.cmet.2006.05.010
37. Magee YZ NS. Hepatocyte Early Growth Response 1 (EGR1) Regulates Lipid Metabolism in Nonalcoholic Fatty Liver Disease. FASEB J (2018) 32(S1):670.56.
38. Li Z, Yu P, Wu J, Tao F, Zhou J. Transcriptional Regulation of Early Growth Response Gene-1 (EGR1) is Associated with Progression of Nonalcoholic Fatty Liver Disease (NAFLD) in Patients with Insulin Resistance. Med Sci Monit (2019) 25:2293–3004. doi: 10.12659/MSM.914044
39. Chen B, Zheng Y, Liang Y. Analysis of Potential Genes and Pathways Involved in the Pathogenesis of Acne by Bioinformatics. BioMed Res Int (2019) 2019:3739086. doi: 10.1155/2019/3739086
40. Wang Y, Cao F, Wang Y, Yu G, Jia BL. Silencing of SAA1 inhibits palmitate- or high-fat diet induced insulin resistance through suppression of the NF-κB pathway. Mol Med (2019) 25(1):17. doi: 10.1186/s10020-019-0075-4
41. Yuan ZY, Zhang XX, Wu YJ, Zeng ZP, She WM, Chen SY, et al. Serum amyloid A levels in patients with liver diseases. World J Gastroenterol (2019) 25(43):6440–50. doi: 10.3748/wjg.v25.i43.6440
42. Tomasello E, Vivier E. KARAP/DAP12/TYROBP: three names and a multiplicity of biological functions. Eur J Immunol (2005) 35(6):1670–7. doi: 10.1002/eji.200425932
43. Dai W, Sun Y, Jiang Z, Du K, Xia N, Zhong G. Key genes associated with non-alcoholic fatty liver disease and acute myocardial infarction. Med Sci Monit (2020) 26:e922492. doi: 10.12659/MSM.922492
44. Turnbull IR, McDunn JE, Takai T. DAP12 (KARAP) amplifies inflammation and increases mortality from endotoxemia and septic peritonitis. J Exp Med (2005) 202(3):363–9. doi: 10.1084/jem.20050986
45. Stanton MC, Chen SC, Jackson JV, Rojas-Triana A, Kinsley D, Cui L, et al. Inflammatory Signals shift from adipose to liver during high fat feeding and influence the development of steatohepatitis in mice. J Inflammation (Lond) (2011) 8:8. doi: 10.1186/1476-9255-8-8
46. Hou C, Feng W, Wei S, Wang Y, Xu X, Wei J, et al. Bioinformatics Analysis of Key Differentially Expressed Genes in Nonalcoholic Fatty Liver Disease Mice Models. Gene Expr (2018) 19(1):25–35. doi: 10.3727/105221618X15341831737687
47. Serhal R, Hilal G, Boutros G, Sidaoui J, Wardi L, Ezzeddine S, et al. Nonalcoholic Steatohepatitis: Involvement of the Telomerase and Proinflammatory Mediators. BioMed Res Int (2015) 2015:850246. doi: 10.1155/2015/850246
48. Gebhardt C, Németh J, Angel P, Hess J. S100A8 and S100A9 in inflammation and cancer. Biochem Pharmacol (2006) 72(11):1622–31. doi: 10.1016/j.bcp.2006.05.017
49. Si M, Lang J. The roles of metallothioneins in carcinogenesis. J Hematol Oncol (2018) 11(1):107. doi: 10.1186/s13045-018-0645-x
50. Oliva RD L, Medici V. Metallothioneins and liver diseases. Metallothioneins (2008). doi: 10.1142/9789812778949_0014
51. Carrera G, Paternain JL, Carrere N, Folch J, Courtade-Saïdi M, Orfila C, et al. Hepatic metallothionein in patients with chronic hepatitis C: relationship with severity of liver disease and response to treatment. Am J Gastroenterol (2003) 98(5):1142–9. doi: 10.1111/j.1572-0241.2003.07403.x
52. Wang Y, Cheng M, Zhang B, Nie F, Jiang H. Dietary supplementation of blueberry juice enhances hepatic expression of metallothionein and attenuates liver fibrosis in rats. PLoS One (2013) 8(3):e58659. doi: 10.1371/journal.pone.0058659
53. Parrott J, Frank L, Rabena R, Craggs-Dino L, Isom KA, Greiman L. Asmbs Integrated Health Nutritional Guidelines For The Surgical Weight Loss Patient — 2016 Update: Micronutrients. Surg Obes Relat Dis (2017) 13(5):727–41. doi: 10.1016/j.soard.2016.12.018
54. Baltaci AK, Yuce K, Mogulkoc R. Zinc Metabolism and Metallothioneins. Biol Trace Elem Res (2018) 183:22–31. doi: 10.1007/s12011-017-1119-7
55. Krężel A, Maret W. The Functions of Metamorphic Metallothioneins in Zinc and Copper Metabolism. Int J Mol Sci (2017) 18(6):1237. doi: 10.3390/ijms18061237
56. Korner J, Inabnet W, Conwell IM, Taveras C, Daud A, Olivero-Rivera L, et al. Differential effects of gastric bypass and banding on circulating gut hormone and leptin levels. Obesity (Silver Spring) (2006) 14:1553–61. doi: 10.1038/oby.2006.179
57. Keitel V, Häussinger D. Perspective: TGR5 (Gpbar-1) in liver physiology and disease. Clin Res Hepatol Gastroenterol (2012) 36:412–9. doi: 10.1016/j.clinre.2012.03.008
58. Braunersreuther V, Viviani GL, Mach F, Montecucco F. Role of cytokines and chemokines in non-alcoholic fatty liver disease. World J Gastroenterol (2012) 18:727–35. doi: 10.3748/wjg.v18.i8.727
59. Trakhtenbroit MA, Leichman JG, Algahim MF, Miller CC 3rd, Moody FG, Lux TR, et al. Body weight, insulin resistance, and serum adipokine levels 2 years after 2 types of bariatric surgery. Am J Med (2009) 122:435–42. doi: 10.1016/j.amjmed.2008.10.035
60. Zhang H, DiBaise JK, Zuccolo A, Kudrna D, Braidotti M, Yu Y, et al. Human gut microbiota in obesity and after gastric bypass. Proc Natl Acad Sci USA (2009) 106:2365–70. doi: 10.1073/pnas.0812600106
Keywords: bariatric surgery, gastric bypass, nonalcoholic steatohepatitis, nonalcoholic fatty liver disease, microarray, differentially expressed genes
Citation: Chen F, Zhou Y, Wu Z, Li Y, Zhou W and Wang Y (2021) Integrated Analysis of Key Genes and Pathways Involved in Nonalcoholic Steatohepatitis Improvement After Roux-en-Y Gastric Bypass Surgery. Front. Endocrinol. 11:611213. doi: 10.3389/fendo.2020.611213
Received: 28 September 2020; Accepted: 14 December 2020;
Published: 02 February 2021.
Edited by:
Magdalene K. Montgomery, The University of Melbourne, AustraliaReviewed by:
Pablo Giraudi, Italian Liver Foundation ONLUS, ItalyMing Song, University of Louisville, United States
Copyright © 2021 Chen, Zhou, Wu, Li, Zhou and Wang. This is an open-access article distributed under the terms of the Creative Commons Attribution License (CC BY). The use, distribution or reproduction in other forums is permitted, provided the original author(s) and the copyright owner(s) are credited and that the original publication in this journal is cited, in accordance with accepted academic practice. No use, distribution or reproduction is permitted which does not comply with these terms.
*Correspondence: Yong Wang, d2FuZ3lvbmdAY211LmVkdS5jbg==