- Department of Medicine, University of Padova, Padova, Italy
Gestational diabetes mellitus (GDM) is the most common metabolic complication of pregnancy, with a prevalence that has increased significantly in the last decade, coming to affect 12–18% of all pregnancies. GDM is believed to be the result of a combination of genetic, epigenetic and environmental factors. Following the identification of susceptibility genes for type 2 diabetes by means of genome-wide association studies, an association has also been demonstrated between some type 2 diabetes susceptibility genes and GDM, suggesting a partial similarity of the genetic architecture behind the two forms of diabetes. More recent genome-wide association studies, focusing on maternal metabolism during pregnancy, have demonstrated an overlap in the genes associated with metabolic traits in gravid and non-gravid populations, as well as in genes apparently unique to pregnancy. Epigenetic changes—such as DNA methylation, histone modifications and microRNA gene silencing—have also been identified in GDM patients. Metabolomics has been used to profile the metabolic state of women during pregnancy, based on the measurement of numerous low-molecular-weight metabolites. Measuring amino acids and conventional metabolites has revealed changes in pregnant women with a higher insulin resistance and high blood glucose levels that resemble the changes seen in non-gravid, insulin-resistant populations. This would suggest similarities in the metabolic profiles typical of insulin resistance and hyperglycemia whether individuals are pregnant or not. Future studies combining data obtained using multiple technologies will enable an integrated systems biology approach to maternal metabolism during a pregnancy complicated by GDM. This review highlights the recent knowledge on the impact of genetics and epigenetics in the pathophysiology of GDM and the maternal and fetal complications associated with this pathology condition.
Introduction
Gestational diabetes mellitus (GDM) is defined as any degree of glucose intolerance developing or first recognized during pregnancy that is not clearly overt diabetes (1). GDM affects from 5–6% to as many as 15–20% of pregnancies worldwide, and its prevalence is increasing due mainly to rising rates of obesity, sedentary lifestyles, and women becoming pregnant at an older age (2, 3). Even if GDM is currently the most common medical complication of pregnancy, no single diagnostic modality to screen and diagnose this condition is universally applied. In this context it is difficult to assess its real frequency and related complications (3, 4).
The increase in placental hormones antagonizing the action of insulin in the second and third trimesters of pregnancy gives rise to a physiological increase in insulin resistance. The purpose of this mechanism is to provide the fetus with glucose, and a gradual increase in insulin secretion enables the woman’s normal glucose levels to be maintained (5). GDM develops when beta cell insulin secretion is unable to compensate for pregnancy-induced insulin resistance, and/or in conjunction with an impaired beta cell function (6–8).
Untreated GDM can lead to adverse outcomes for both the mother and her child during pregnancy and childbirth, including preeclampsia, cesarean delivery, birth trauma, macrosomia, and neonatal hypoglycemia and hyperbilirubinemia (9–11). GDM women have a 2 fold higher risk of developing type 2 diabetes later in life, as well as metabolic syndrome and cardiovascular disease. Furthermore children of these patients are at high risk to develop type 2 diabetes and obesity at an early age (earlier) (9–11). Therefore, greater efforts to reduce obesity and diabetes, especially among girls and young women through lifestyle modifications are necessary (4). Unfortunately, the participation of GDM women in postpartum follow-up programs is still unsatisfactory, emphasizing the importance to improve risk awareness utilizing more proactive patient-contacting attempts (4, 12).
The pathogenesis of GDM is not fully understood, but recent studies have suggested that it could be the result of a combination of genetic, epigenetic and environmental factors.
This review highlights the recent knowledge on the impact of genetics and epigenetics in the pathophysiology of GDM and the maternal and fetal complications associated with this pathology.
Genetic Studies
Two different approaches have been used to study the role of genes in maternal metabolism during pregnancy. Studies on candidate genes have examined the association between variants of selected genes and the risk of GDM. However, this approach has proved of limited use for the purpose of identifying the genes involved, due to small sample sizes and the inclusion of limited numbers of variants in the genetic loci. Using genome-wide association studies (in which genetic variants across the whole genome are tested for any association with a trait or disease), variants from many different loci have been associated with the risk of GDM. They have each shown only a modest effect, however, so large numbers of individuals need to be assessed to demonstrate a significant association.
Given that GDM, like type 2 diabetes, is characterized by insulin resistance, several genes known to be associated with a higher risk of developing type 2 diabetes have also been investigated in patients with GDM.
Kwak et al. (13). used the genome-wide association approach to compare 1399 women with GDM (cases) and 2025 non-diabetic women (controls). They employed 2,19 million genotyped or imputed markers, and selected 11 loci for further genotyping in stage 2 samples from 931 cases and 783 controls. The effect of genetic variants known to be associated with type 2 diabetes was also investigated in the women with GDM. A significant association emerged between known T2D variants and GDM. This association with GDM was particularly strong for the genetic variants in CDKAL (a type of variant affecting beta cell survival) and MTNR1B (a variant associated with high fasting plasma glucose and insulin levels). These results point to a shared genetic basis for GDM and T2D.
The association between certain genetic variants that raise the risk of T2D and GDM was subsequently studied by Huopio and coworkers in a sample of 533 GDM women and 407 normal pregnant controls, who were genotyped for 69 single-nucleotide polymorphisms (SNPs) (14). Interestingly, the MTNR1B variants rs10830963 and rs1387153 were found significantly associated not only with GDM, but also with impaired fasting plasma glucose levels and some insulin secretion indicators (the Matsuda index, the insulin/glucose AUC, and the AUC adjusted for the Matsuda index) measured in GDM patients after pregnancy (14). A genome-wide association study performed in East Asian population showed that some polimorhisms in KCNQ1 are associated with GDM (15). In 869 Korean GDM women and 632 non diabetic control subjects rs 2074196 and rs 2237892 were associated with the risk of GDM (OR 1.17, 95% CI 1.01–1.36, p=0.039; OR 1.24,95% CI 1.07–1.45, p=0.0049 respectively); furthermore rs 2237892 and rs 2237895 were associated with decreased insulinogenic index at the time of GDM diagnosis (p=0.025 and p=0.037) (15). Ren et al. tested MTNR1B for the association with diabetes related traits in 1.383 subjects at baseline and in 374 subjects over a 3–5 year follow up participating in the family-based Beta Gene study of the Mexican American (16). The rs 10830963 was associated cross-sectionally with fasting glucose (p=0.0069), acute insulin response (AIR, p=0.0013), disposition index (p=0.00078), glucose effectiveness (p=0.018), gestational diabetes (OR 1.48; p=0.012), the rate of change of fasting glucose (p=0.043), No association was found with OGTT 30 min and delta insulin (the difference between the 30 min and fasting glucose concentration), 30 min insulin based disposition index and the rate of change in beta cell compensation for insulin resistance. So the variation in MTNR1B contribute to determine the absolute level but not the rate of changes over time of insulin secretion. The differences in absolute insulin secretion and beta cell compensation could be a factor involved in TD2 and GDM development. This study makes an important contribution to the knowledge of the pathophysiological mechanisms determining type 2 diabetes and GDM.
The insulin receptor substrate 1 (IRS-1) expressed in tissues sensitive to insulin is crucial to glucose transporter 4 translocation (GLUT-4). IRS-1 polymorphism has been found related to insulin resistance, obesity and type 2 diabetes mellitus. In a study on GDM, the frequency of IRS-1 gene polymorphism was significantly higher in women with GDM than in pregnant women with a normal glucose tolerance, suggesting a role for this polymorphism in the onset of GDM as well as type 2 diabetes mellitus (17). The switch on IRS-1 of the amino acid GLY972 Arg (rs1801278) impairs insulin secretion, and a study on 1306 GDM patients and 1973 pregnant women without GDM found a significant association between the presence of this polymorphism and the risk of GDM (18).
Intriguing results were generated by a study on the genetic variants in GCK and TCF7L2, and their possible association with glucose levels on oral glucose tolerance tests (OGTTs) performed in pregnant women participating in the Hyperglycemia and Adverse Pregnancy Outcomes Study (HAPO) (19). This study involved 25000 pregnant women, and was designed to test the association between maternal glycemia and adverse outcomes of pregnancy. The OGTT results showed that the GCK variant was associated with high fasting, 1 h and 2 h glucose levels. In the European women’s offspring, the GCK variant was associated with a high birth weight, fat mass and skinfold thickness as well. Variants in GCK and TCF7L2 were also associated with a higher odds ratio for the onset of GDM (20).
Table 1 lists the genes responsible for insulin secretion, insulin resistance and glucose metabolism that have been found associated with the risk of GDM (13, 15, 18, 21–26).
During pregnancy, chronic low-grade inflammation have a role in causing insulin resistance, and several studies have found higher levels of inflammatory mediators in GDM patients and their newborn (27, 28). Using a candidate gene approach, some metabolism indicators and variants in 31 inflammatory pathway genes were examined in women taking part in the HAPO study. A significant association emerged between some maternal metabolism indicators (fasting and 1 h plasma glucose, C-peptide, HbA1c) and six of the genes investigated, suggesting that inflammation would contribute to the metabolic phenotype in pregnancy (Table 2) (29).
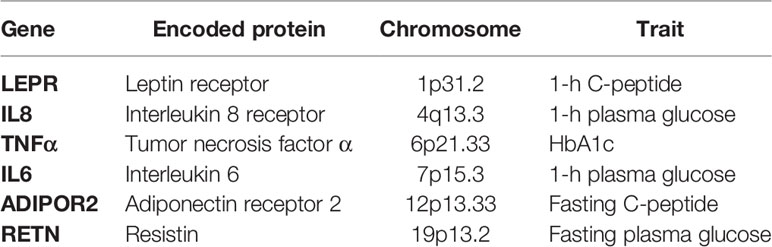
Table 2 Inflammatory pathway genes associated with maternal metabolism (29).
In order to evaluate the effect of preexisting maternal obesity and gestational diabetes on the expression of genes involved in adipokines regulation and lipid metabolism, human subcutaneous adipose tissues were analyzed by quantitative RT-PCR. The gene expression of the adipokines TNFalfa, IL-1beta, and leptin were increased in adipose tissue from obese (No.10) and GDM (No. 18) with respect to normal control women (No. 28). Furthermore, decreased gene expression was found for some transcription factors involved in lipid metabolism as LXRα, PPARα, PPARδ, PPARγ, RXRα and SREBP1c. These data underline the importance of the excess of substrate supply from the mother to the fetus in determining neonatal adiposity in obese and GDM pregnant women (30).
The genetic studies published until now show that several genes are involved in the physiophatology of GDM. However, the most of the studies have been conducted only in some populations (e.g. Asia, Korea) and this could explain the reason why they are not reproducible across population; furthermore, some studies are not adjusted for confounders (maternal age, diet, scolarity). Studies linking genetic variants to environmental factors are needed to understand the physiophatology of GDM.
Epigenetic Studies
Epigenetics is the study of changes in gene expression or phenotype prompted by mechanisms other than variations in DNA sequences. Examples of epigenetic changes include methylation, histone modifications, and messenger RNA (mRNA) binding by microRNAs (miRNAs).
Genes react to a pregnant woman’s metabolic status via epigenetic mechanisms, and studies have shown that even a slight increase in glycemia can be associated with epigenetic adaptations via the so-called “metabolic memory”. It is worth emphasizing here that metabolic memory does not only concern what happens in diabetic patients in the short term. It also has to do with long-term metabolic effects in the developing uterine environment. The concept of metabolic memory fits well with what Barker hypothesized in the 1980s about individuals being programmed to cope with nutritional thrift in intrauterine life in order to survive the damage caused by poor or over- nutrition after birth (31). Several studies have examined the association between methylation and GDM. Wu and coworkers described genome-wide DNA methylation changes in pregnant women’s blood before any GDM was diagnosed. They identified a series of differently methylated genes that the woman’s blood, umbilical cord and placenta shared, i.e. Hook Microtubule Tethering Protein 2 (HOOK2), Retinol Dehydrogenase 12 (RDH12), Constitutive photomorphogenic homolog subunit 8 (COPS8), Phosphoinositide-3-kinase, regulatory subunit 5(PIK3R5), 3-hydroxyanthranilate 3,4-dioxygenase (HAAO), Coiled-coil domain containing 124 (CCDC 124) and Chromosome 5 open reading frame 34 (C5orf 34). HOOK2 codes for a protein that mediates binding to organelles, and is involved in cilia morphogenesis and endocytosis. RDH12 encodes a retinal reductase involved in short-chain aldehyde metabolism. COPS8 is a regulator of multiple signaling pathways. PIK3R5 is involved in cell growth, proliferation, differentation, motility, survival and intracellular trafficking. HAAO catalyzes the sintesis of quinolonic acid (QUIN), which is an excitotoxin that may partecipate in the pathogenesis of neurologic and inflammatory disorders. CCDC 124 is involved in cell cycle and cell division, C5orf 34 is unknown, but sequence is conserved in chimpanzee, Rhesus monkey, dog, cow, mouse, rat, chicken and zebrafish (32).
Cardenas et al. (33) conducted an epigenome-wide association study (involving 850000 CpG sites) on samples of placenta and plasma glucose, correlating them with 2 h post-OGTT plasma glucose levels in 448 mother-and-infant pairs at 24–30 weeks of gestation. They found plasma glucose at 2 h OGTT positively associated with a lower DNA methylation of 4 CpG sites within the phosphodiesterase 4b gene. Then 3 other CpG sites in the TNFRSF1B, LDLR and BLM genes were found differentially methylated with respect to maternal glucose. TNFRSF1B is involved in apoptosis, the inhibition of cell fusion, and epithelial shedding. LDLR encodes a lipoprotein receptor that mediates LDL endocytosis in the cells, and is also expressed in the placenta. BLM is associated with genome stability and maintenance. When DNA methylation was correlated with the expression of its respective genes in placental tissue, it emerged that maternal glycemic levels during pregnancy were associated with placental DNA methylation of inflammatory genes, the expression of which depends on epigenetic changes. Bouchard and coworkers (34) found that lower DNA methylation levels in the ADIPOQ promoter on the fetal side of the placenta correlated with higher maternal glucose levels during the second trimester of pregnancy. Lower DNA methylation levels on the maternal side of the placenta were associated with a higher insulin resistance, according to the Homeostasis Model Assessment Method (HOMA), during the second and third trimesters of pregnancy; and higher circulating adiponectin levels throughout pregnancy. Given the insulin-sensitizing properties of adiponectin, the latter epigenetic modifications could prompt changes in glucose metabolism in the mother and offspring later in life.
A number of studies sought a link between epigenetic changes in GDM and the newborn’s development. Hajj et al. (35) tested the effect of GDM on the epigenome of the women’s offspring, analyzing cord blood and placental tissue from the newborn of women with GDM, 88 of them treated with dietary measures and 98 with insulin. The maternal imprinting MEST gene and the non-imprinting glucocorticoid receptor NR3C1 gene (both of which are associated with placental and fetal growth) showed significantly lower methylation levels in both tissues in both GDM groups compared with pregnant women without GDM. MEST methylation was also found significantly lower in the blood of adults with obesity than in normal-weight controls. These results indicate that intrauterine exposure to GDM has lasting effects on the epigenome of the offspring, and that epigenetic malprogramming of MEST can contribute to predisposing individuals to obesity later in life. Another study compared genome-wide methylation patterns in fetal cord blood between pregnant women with and without GDM. Using Illumina 450K methylation arrays, significant differences in methylation emerged between GDM patients and control pregnant women. These differences were more evident in GDM women on insulin than GDM on diet therapy and could be explained by more severe episodes of hypeglycemia in GDM requiring insulin treatment. Changes in methylation were seen for: ATPSA1, which encodes a subunit of mitochondrial ATP synthetase that prevents mitochondrial oxidation; MFAP4, which is involved in cell adhesion and intercellular interaction; PRKCH, which belongs to the protein C family and is involved in various signaling pathways; and SLC17A, an intestinal sodium/phosphate cotransporter associated with hypoxia. Interestingly, all these changes in methylation had a small effect size, but affected multiple genes/loci (36) (Table 3). Recently, methylation profiles were measured in peripheral blood of 93 GDM offspring and 945 controls from the Danish National Birth Cohort, aged 9–16 years, using an illumine Human Methylation 450 Bead Chip (37). Seventy six differentially methylated GpGs in GDM offspring compared to controls were identified (FDR P<0.05). Adjusting for offspring BMI did not change the association with GDM, even if most of the epigenetic changes resulted associated with maternal BMI, 13 methylated genes were independently associated with maternal GDM. This study supports the close association between GDM and pre-pregnancy BMI and strongly suggests that this association has an effect on offspring epigenetic profile involved in fetal metabolic programming.
Women with a history of GDM are at greater risk of developing type 2 diabetes after pregnancy. With this in mind, Michalczyk et al. (38) analyzed several epigenetic markers during and after pregnancy in: women with GDM who subsequently developed type 2 diabetes (No. 6); women with GDM who did not develop type 2 diabetes after delivery (No. 8); women with type 2 diabetes (No. 6); and non-diabetic women (No. 7). When the Authors calculated the proportion of total H3 histone that was methylated, it was: 50% lower for H3K27 at 8–10 and 20 weeks post-partum in the women with GDM who developed type 2 diabetes after pregnancy than in the non-diabetic women; and 75% lower for H3K4 at 8–10 weeks post-partum in the women with GDM who developed type 2 diabetes after pregnancy than in the women with GDM who did not. Although it was conducted on a small sample (No. 27), this study demonstrated that the proportion of histone methylation could be a useful predictor of type 2 diabetes in women with GDM.
Thus DNA methylation could be considered an interesting diagnostic and prognostic marker. However, the most of the studies published in the frame of GDM have limited sample size, do not correlate the markers with maternal and/or neonatal outcomes, furthermore different methods to measure DNA methylation have been utilized. These limitations make difficult to generalize the meaning of the studies.
MiRNAs are small non-coding RNAs that regulate gene expression and various cell functions by directing messenger RNA degradation or inhibiting its translation (39) (Table 3).
Some recently-identified miRNAs have been associated with insulin secretion, insulin resistance, and inflammation, and differences have emerged in some circulating miRNA levels between individuals with and without type 2 diabetes (40). Zhao and others (41) examined some miRNAs in pregnant women at 16–19 weeks of gestation (WG), finding a significantly lower expression of 3 miRNAs (miR-29a, miR-132 and miR222) in women who went on to develop GDM at 24-28 WG than in those who did not develop GDM. MiR-29 plays a part in glucose homeostasis: its overexpression inhibits insulin-stimulated glucose uptake and downregulates gluconeogenesis (42). MiR-132 targets the insulin-mediated regulation of cytochrome P450 (which is involved in hepatic metabolism), and it has a role in trophoblast expansion (its reduced expression impairs normal trophoblast development) (42, 43). MiR-222 is involved in regulating the cell cycle (controlling the cyclin-dependent kinase inhibitor).
Cao and coworkers (44) found miR16.5, miR17-5, and miR20a-5p the best predictors of GDM, with a ROC curve of 0.92, 0.88, and 0.74, respectively. MiR16.5 is involved in a series of processes related to insulin resistance, and it is upregulated in type 2 diabetes. MiR17-5 is involved in smooth muscle cell proliferation, and plays a part in the vascular complications of diabetes. MiR20a-5p is upregulated in preeclampsia, a condition often related to GDM.
Wander et al. (45). examined miRNA levels in pregnant women with GDM in an effort to see whether body mass index had any influence on its onset. MiR155-5p, and 21-3p were found positively associated with GDM, and an association between this condition and miR21-3p, and miR210-3p was only seen in overweight/obese women. MiR-155 and MiR21-3 have a role in pathways that regulate cell survival, such as apoptosis, cell cycle regulation, and response to inflammation. MiR210-3p is associated with angiogenesis (43).
Cao and coworkers looked into the role of miR-98 in placental tissues from GDM patients (46). MiR-98 is upregulated in the kidney in type 2 diabetes, and it is involved in embryo implantation. The results of the study indicate that miR-98 is upregulated and total DNA methylation levels are reduced in the placentas of GDM patients by comparison with those of normal pregnant women. MiR-98 targets Mecp2 and also regulates the Mecp2 target gene, and this could have important consequences for the fetal growth (Table 4).
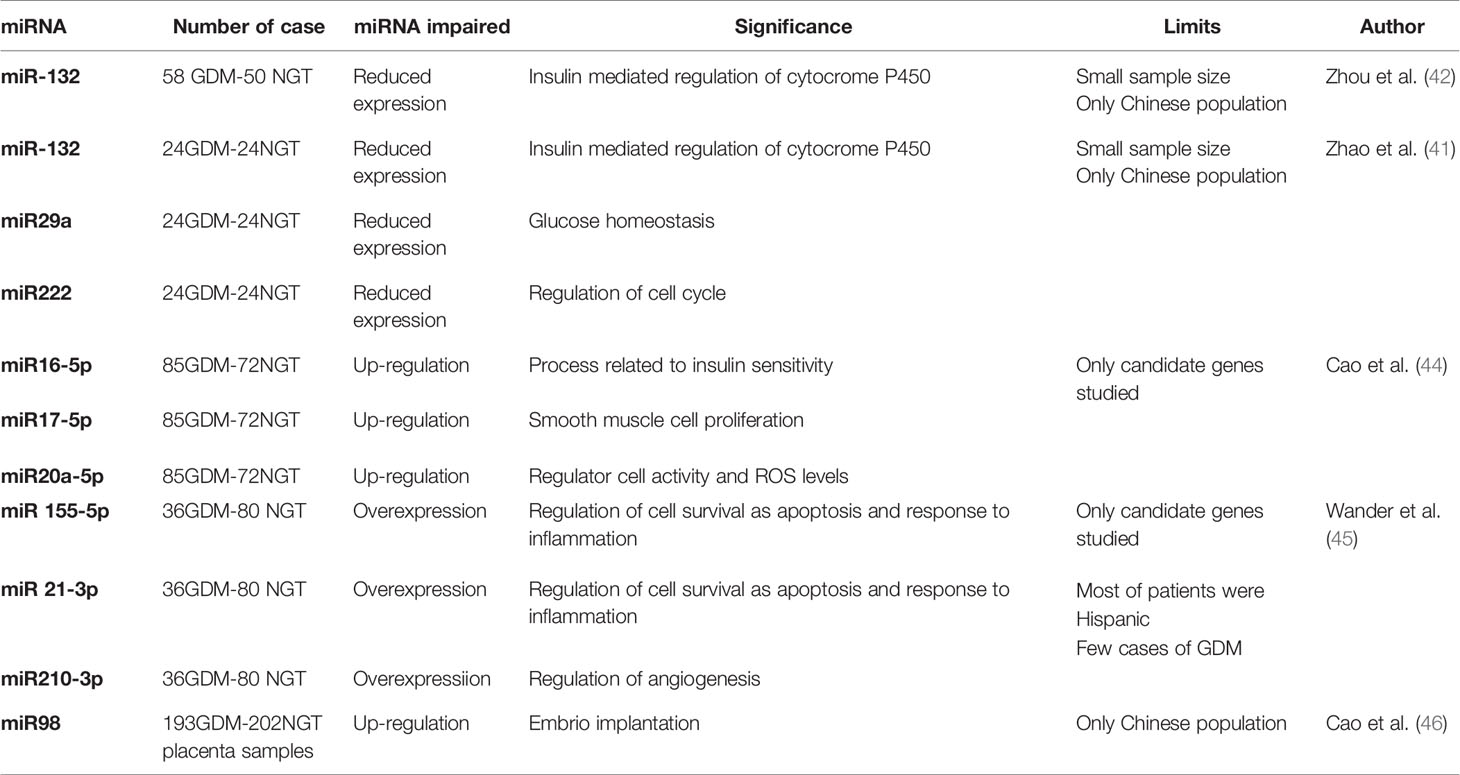
Table 4 Studies assessing the role of mRNAs in gestational diabetes (GDM) and Normal Glucose Tolerance (NGT).
Recent years have seen a few studies conducted to identify specific miRNAs as predictors of GDM, and of maternal and fetal outcomes. The results have been promising, but inconclusive for the time being. Further studies need to be performed, bearing in mind that miRNA expression in pregnancy varies with weeks of gestation, the sex of the fetus, the type of sample considered (blood, placenta, cord blood, and so on), and the mode and type of delivery (47).
New Methodological Approaches
Metabolomics is a relatively new-omic technology capable of shedding light on an individual’s metabolic status by measuring their low-molecular-weight metabolites, almost 4000 of which have been identified in human serum (48). Their identification was made possible thanks to the use of highly precise and accurate methods, such as magnetic resonance spectroscopy and mass spectrometry (49, 50).
In a study using magnetic resonance spectroscopy, the levels of some urinary metabolites—such as 3-hydroxyisovaleriate, 2-hydroxybutyrate, and choline—were found higher in GDM patients than in pregnant women without GDM (51). High 3-hydroxyisovaleriate levels reflect a reduction in biotin status. Levels of 2-hydroxybutyrate are reportedly associated with insulin resistance, and have been proposed as markers of the onset of type 2 diabetes (52).
Liu et al. (53) combined metabolic and genetic data relating to insulin sensitivity during pregnancy obtained from samples collected from women participating in the HAPO study, who underwent OGTT at 24–28 WG. Data on metabolites were obtained using targeted and untargeted mass spectrometric approaches, and the genetic information came from a genome-wide association study. Several metabolites were found associated with maternal insulin sensitivity, including amino acids, lipid metabolites, fatty acids and carbohydrates. The genome-wide association study identified 12 genetic variants in the GCKR locus significantly associated with insulin sensitivity, as measured with the insulin sensitivity index. Then the common GCKR variant rs1260326 was found significantly associated with a number of fasting and 1 h post-OGTT concentrations of metabolites such as triacylglycerol, 2-hydroxybutyrate, lactate, 2-ketoleucine, and palmitoleic acid. Mediation analysis suggested that 1 h palmitoleic acid concentrations contribute to the association between rs1260326 and insulin resistance. This study identified associations between metabolites, genetic markers and insulin sensitivity in pregnant women, shedding new light on the pathophysiology of GDM. A comprehensive analysis of metabolomic studies performed in China evaluating by mass spectrometry a series of urinary metabolites in GDM women has proposed a new view in the relationship between obesity and GDM (54). In particular the author propose that obesity determines the production of proinflammatory molecules that activates the tryptophan-kynurenine pathway and xanturenic acid synthesis so determining hyperglycemia. Hyperglycemia in its turn determines an increase of the nucleotide and acid uric synthesis. Supeoxide anions are produced as byproducts. The increased production of xanthurenic acid, uric acid and superoxide anions contributes to the development of GDM.
Proteomics (55) is an approach that uses mass spectrometry to identify any changes in protein expression due—in the case of interest here—to hyperglycemia and GDM. The method has been used mainly to study the placenta (56–60). It is well known that the placenta undergoes a number of structural and functional changes in diabetes because of the abnormal maternal milieu brought on by high glucose and insulin levels, which increase the production of inflammatory cytokines (56–58). In this setting, applying different mass spectrometry approaches—such as MALDI-MS and LC-MSE—to the study of placental samples from women with and without GDM generated evidence to show that, if well controlled, GDM induces only minor changes in the placental proteome (59, 60).
The studies published until now show differences in the metabolic profiles of mothers with GDM with respect to pregnant non diabetic women. This finding need to be confirmed by large studies that should also evaluate the relationships between the changes in metabolic profile and fetal outcomes.
Nutrigenetics in GDM
Nutrigenetics is the study of the effect of genetic variation on dietary response and of the implication of these interactions on the health status and on the diseases related to nutrition (61).
Recent evidence suggests that dietary factors can induce epigenetic changes, that in turn can affect genome stability and the expression of miRNAs and proteins involved in metabolism (62).
In a study that investigated the association between some clinical parameters and nine single nucleotide polymorphisms (SNPs) involved in nutrition and metabolism in women with and without GDM, the TCF7L2 gene variant rs7903146 showed a strong association with the risk of developing GDM (OR: 2.56; 95% CI: 1.24–5.29). A positive correlation was found between lipid parameters and polymorphisms of PPARG2 (p=0.03), APOA5 (p=0.02), MC4R (p=0.03), LDLR (p=0.04) and FTO (p=0.03). The rs17782313 variant, close to the MC4R gene, was associated with pre-pregnancy BMI (p=0.02) in the women with GDM. The significant associations between the GDM women’s nutritional parameters and some gene variants involved in nutrition and metabolism could be key to the development of effective tools to prevent GDM (63). In another study aiming to assess the role of novel nutrigenetic markers, alongside traditional parameters, in predicting early, subclinical atherosclerosis in 29 women with a history of GDM, the Authors measured 9 SNPs from 9 genes related to nutrition and metabolism, which were genotyped using high-resolution melting analysis. During a 3-year postpartum follow-up, all the women had an OGTT, their carotid artery intima-media thickness (cIMT) was measured, and their metabolic parameters were analyzed. Significant associations were found between the APOA5-CC genotype and cIMT, and between the CC-APOA5/CC-LDLR interaction and cIMT. This preliminary evidence suggests a role for lipid profile during pregnancy and some genetic variants in predicting cIMT, which is an early marker of cardiovascular disease (64).
Eating habits are a key factor influencing human health and fertility. A fetus’s adequate nutrition in utero ensures a normal hypothalamic programming with a balanced production of anorexigenic and orexigenic peptides, and this gives rise to normal feeding patterns in adult life. If a pregnant woman is not adequately nourished, an altered hypothalamic function develops in her offspring, with an exacerbation of the orexigenic state that causes hyperphagia, reduced satiety and metabolic syndrome later in life (Figure 1). In particular it has been shown that after periods of famine such as those of Dutch (65), China (66) and Austria (67) offspring born during and after show an increased risk to develop metabolic syndrome. Interestingly, in the exposed children of the Duch famine low levels of DNA methylation of the imprinting IGF2 gene have been reported (68). On the other side studies on rodents and primates mothers nourished with high fat diet determined insulin resistance, high blood pressure and metabolic syndrome in the offspring (69) due to epigenetic modifications of leptin and adiponectin gene expression (70).
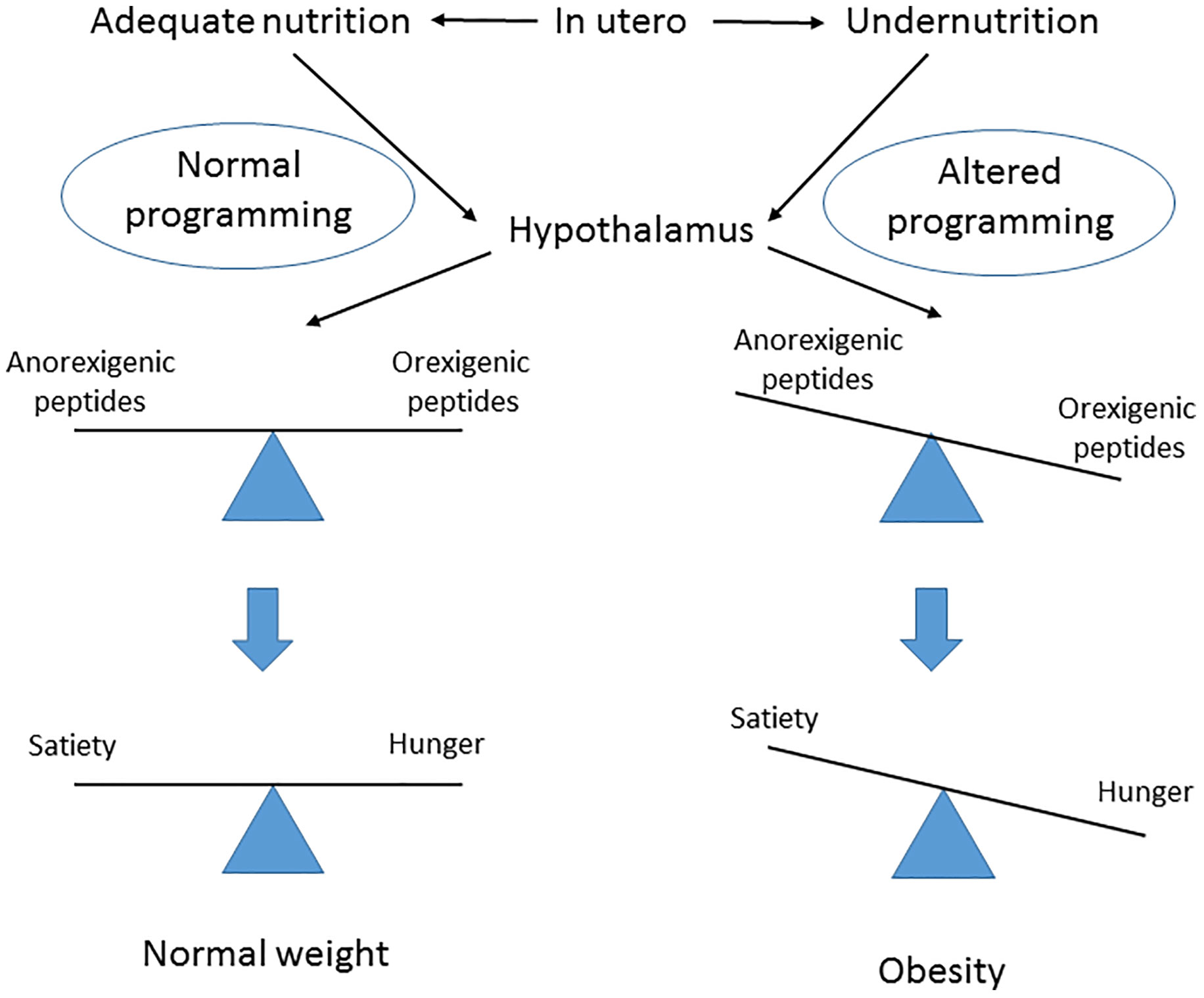
Figure 1 Postulated effect of fetal nutrition in utero on hypothalamus central regulator of obesity programming and subsequent risk later in life.
As for the offspring of women with GDM, an excessive intake of food during gestation and consequent excessive weight gain give rise to a lower adiponectin expression, higher leptin expression, and hypermethylation of adiponectin in adipose tissue of the fetus (71). A diet rich in lipids during gestation also induces hypermethylation in the offspring’s liver (72). These adverse effects carry a trangenerational risk of metabolic syndrome in the fetus that persists for three generations, even if subsequent pregnancies are characterized by a normal diet (73) (Figure 2).
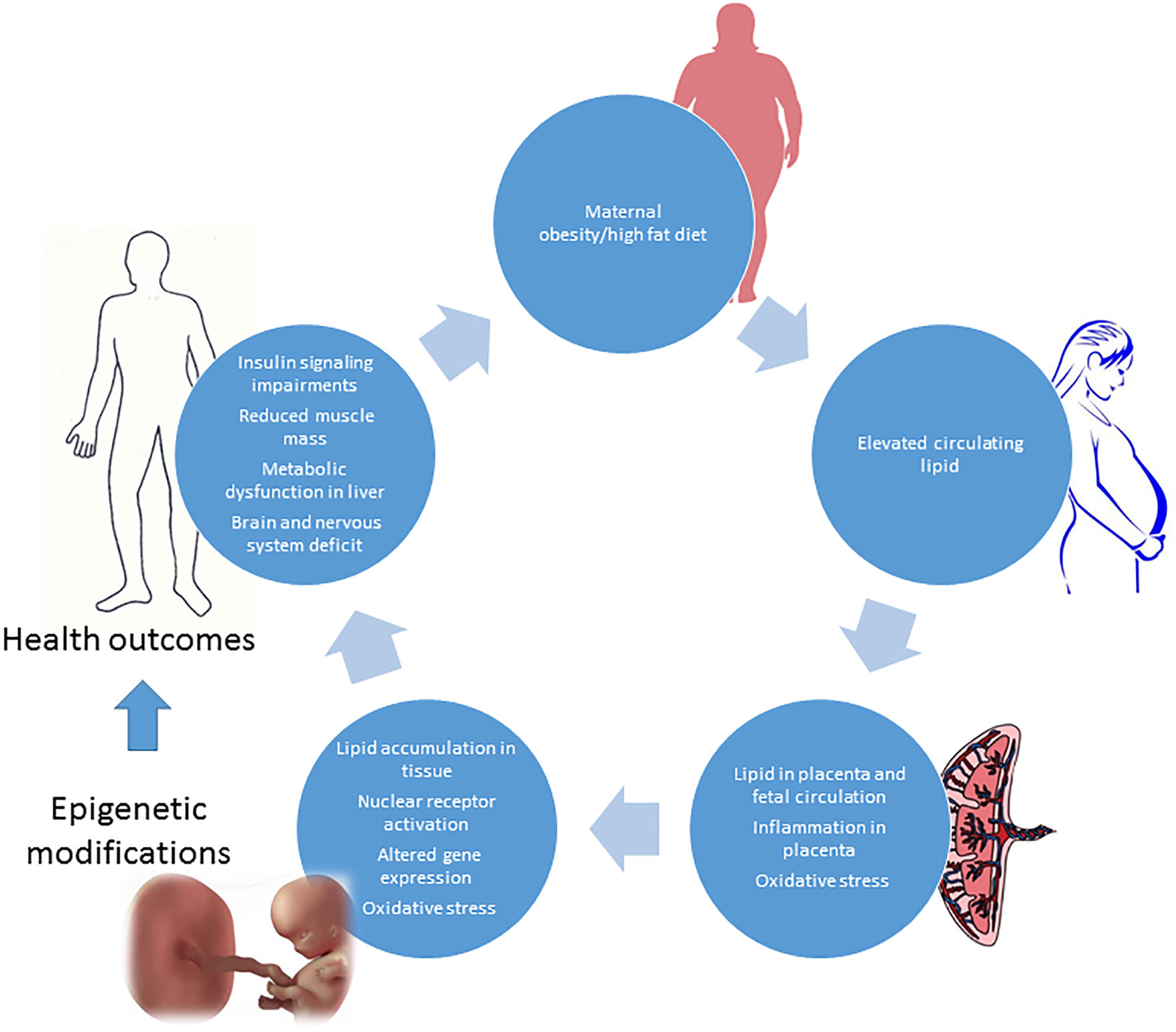
Figure 2 Obesity and high fat diet in pregnancy: impact on fetus development and adverse health outcomes in adult later in life.
In short, a correct lifestyle and diet are essential to avoid the negative effects of genetic and epigenetic changes. A study on rat pancreatic islets showed that the transcription factor Hhnf4a, which is involved in the onset of type 2 diabetes, is epigenetically regulated by a pregnant woman’s diet, establishing a link between mother nutrition and long-term health of offspring (74). Diet and exercise in obese fathers were able to recover an appropriate adiposity and insulin sensitivity in their female offspring (75). Finally, a protective role of bioactive dietary compounds—in terms of reducing epigenetic changes—has recently been suggested (76).
The connection of these genomic information along with high-specific “omic” technologies will enable the acquisition of new knowledge in order to obtain a better understanding of nutrient-gene interactions depending on the genotype and to develop personalized nutrition strategies for optimal health and disease prevention.
Conclusions
Interactions between genetic, epigenetic and environmental factors are a key factor in the onset of GDM. Genome-wide association studies first demonstrated that some T2D susceptibility genes are associated with GDM too, suggesting that the genetic architecture of type 2 diabetes and GDM are similar to some degree. Then more recent studies revealed that some genes are unique to pregnancy. Epigenetic changes, such as DNA methylation, histone modifications, and miRNA gene silencing, have also been identified in women with GDM. Study limitations are the small sample size, ethnic differences, and related type of foods. Larger studies, that include different ethnic group to differentiate the impact of ethnicity, lifestyle and nutritional habits on genetics/epigenetics expression are needed. For clinical application of epigenetic and genetic data it is necessary to reduce the number of genes involved to be analyzed. If it were possible to identify a limited number of measurable genes they could be considered in screening and diagnosis of GDM. As regards, on the other side, the relationship with maternal and fetal outcomes no data is available yet. Actually, in clinical practice, we can only give nutritional and lifestyle indications to GDM women in order to reduce the impact of genetics/epigenetics on mother and child health, considering that both nutrition and physical activity showed a positive effect on genetic/epigenetic modification. Future studies combining data from multiple technologies will give us a deeper understanding of the pathophysiology behind GDM. The roles of dietary patterns, nutrients, bioactive substances, and exercise in affecting the genome and epigenome need to be clarified, so that they can be used effectively and appropriately in cases of GDM to reduce the medium- and long-term complications for the mothers and their offspring.
Author Contributions
AL wrote the manuscript with support from MD, SB, and GD. MD and SB handled the reference resource. MD and GD realized tables and images. All authors contributed to the article and approved the submitted version.
Conflict of Interest
The authors declare that the research was conducted in the absence of any commercial or financial relationships that could be construed as a potential conflict of interest.
References
1. International Association of Diabetes and Pregnancy Study Groups Consensus Panel. International Association of Diabetes and Pregnancy Study Groups: Recommendations on the diagnosis and classification of hyperglycemia in pregnancy. Diabetes Care (2010) 33:676–68. doi: 10.2337/dc09-1848
2. Koning SH, Hoogenberg K, Lutgers HL, Van den Berg PP, Wolffenbuttel BHR. Gestational diabetes mellitus: current knowledge and unmet needs. J Diabetes (2016) 8(6):770–81. doi: 10.1111/1753-0407.12422
3. Lapolla A, Metzger BE. The post-HAPO situation with gestational diabetes: the bright and dark sides. Acta Diabetol (2018) 55(9):885–92. doi: 10.1007/s00592-018-1146-7
4. McIntyre DH, Catalano P, Zhang C, Desoye G, Mathiesen ER, Damm P. Gestational diabetes mellitus. Nat Rev (2019) 5:1–19. doi: 10.1038/s41572-019-0098-8
5. Ryan EA, Enns L. Role of gestational hormones in the induction of insulin resistance. J Clin Endocrinol Metab (1988) 67:341–47. doi: 10.1210/jcem-67-2-341
6. Kampmann U, Knorr S, Fuglsang J, Ovesen P. Determinants of maternal insulin resistance during pregnancy: an updated over review. J Diabetes Res (2019) 2019:5320156. doi: 10.1155/2019/5320156
7. Lain K, Catalano PM. Metabolic changes in pregnancy. Clin Obstet Gynaecol (2007) 50:938– 948. doi: 10.1097/GRF.0b013e31815a5494
8. Lapolla A, Dalfrà MG, Mello G, Parretti E, Cioni R, Marzari C, et al. Early detection of insulin sensitivity and beta-cell function with simple tests indicates future derangements in late pregnancy. J Clin Endocrinol Metab (2008) 93:876–80. doi: 10.1210/jc.2007-1363
9. Burlina S, Dalfrà MG, Lapolla A. Short- and long-term consequences for offspring exposed to maternal diabetes: a review. J Mater Fetal Neonatal Med (2019) 32(4):687–94. doi: 10.1080/14767058.2017.1387893
10. Lowe WL, Scholtens DM, Lowe LP, Kuang A, Nodzenky M, Talbot O, et al. Association of gestational diabetes with maternal disorders of glucose metabolism and childhood adiposity. JAMA (2018) 320(10):1005–16. doi: 10.1001/jama.2018.11628
11. Burlina S, Dalfrà MG, Lapolla A. Clinical and biochemical approach to predicting post-pregnancy metabolic decompensation. Diabetes Res Clin Pract (2018) 145:178–83. doi: 10.1016/j.diabres.2018.02.035
12. Dalfra MG, Burlina S, Del Vescovo GG, Anti F, Lapolla A. Adherence to a follow up program after gestational diabetes in an Italian population. Acta Diabetol (2020) 57(12):1473–80. doi: 10.1007/s00592-020-01564-y
13. Kwak SH, Kim SH, Cho YM, Cho YS, Choi SH, Moon NK, et al. A genome-wide association study of gestational diabetes mellitus in Korean women. Diabetes (2012) 61:531–41. doi: 10.2337/db11-1034
14. Huopio H, Cederberg H, Vangipurapu J, Hakkarainen H, Pääkkönen M, Kuulasmaa T, et al. Association of risk variants for type 2 diabetes and hyperglycemia with gestational diabetes. Eur J Endocrinol (2013) 169:291–7. doi: 10.1530/EJE-13-0286
15. Kwak SH, Kim TH, Cho YM, Choi SH, Jang HC, Park KS. Polymorphisms in KCNQ1 are associated with gestational diabetes in a Korean population. Horm Res Paediatr (2010) 74(5):333–8. doi: 10.1159/000313918
16. Ren J, Xiang AH, Trigo E, Takayanagi M, Beale E, Lawrence JM, et al. Genetic variation in MTNR1B is associated with gestational diabetes mellitus and contributes only to the absolute level of beta cell compensation in Mexican Americans. Diabetologia (2014) 57(7):1391–9. doi: 10.1007/s00125-014-3239-3
17. Fallucca F, Dalfrà MG, Sciullo E, Masin M, Buongiorno AM, Napoli A, et al. Polymorphism of insulin receptor substrate 1 and beta3-adrenergic receptor genes in gestational diabetes and normal pregnancy. Metabolism (2006) 55:1451–6. doi: 10.1016/j.metabol.2006.06.004
18. Zhang Y, Sun CM, Hu XQ, Zhao X. Relationship between melatonin receptor 1B and insulin receptor substrate 1 polymorphisms with gestational diabetes mellitus: a systematic review and meta-analysis. Sci Rep (2014) 4:6113–23. doi: 10.1038/srep06113
19. HAPO Study Cooperative Research Group. Hyperglycemia and adverse pregnancy outcomes. N Engl J Med (2008) 358:1991–2002. doi: 10.1056/NEJMoa0707943
20. Freathy RM, Hayes MG, Urbanek M, Lowe L, Lee H, Ackerman C, et al. Hyperglycemia and adverse pregnancy outcome (HAPO) study: common genetic variants in GCK and TCF7L2 are associated with fasting and post-challenge glucose levels in pregnancy and with the new consensus definition of gestational diabetes mellitus from the International Association of Diabetes and Pregnancy Study Group. Diabetes (2010) 59:2682–89. doi: 10.2337/db10-0177
21. Zhang C, Bao W, Rong Y, Yang H, Bowers K, Yeung E, et al. Genetic variants and the risk of gestational diabetes mellitus: a systematic review. Hum Reprod Update (2013) 19:376–90. doi: 10.1093/humupd/dmt013
22. Ding M, Chavarro J, Olsen S, Lin Y, Ley S, Bao W, et al. Genetic variants of gestational diabetes mellitus: a study of 112 SNPs among 8722 women in two independent populations. Diabetologia (2018) 6:1758–68. doi: 10.1007/s00125-018-4637-8
23. Lin Z, Wang Y, Zhang B, Jin Z. Association of type 2 diabetes susceptible genes GCKR, SLC308A7 and FTO polymorphisms with gestational diabetes mellitus risk: a meta-analysis. Endocrine (2018) 62:34–5. doi: 10.1007/s12020-018-1651-z
24. Kantimathi S, Chidambaram M, Bodini D, Liju S, Bhavatharini A, Uma R, et al. Association of recently-identified type 2 diabetes gene variants with gestational diabetes in Asian Indian population. Mol Genet Genomics (2017) 292:585–93. doi: 10.1007/s00438-017-1292-6
25. Whu L, Cui L, Tam WH, Ma RC, Wang CC. Genetic variants associated with gestational diabetes mellitus: a meta-analysis and subgroup analysis. Sci Rep (2016) 6:30539. doi: 10.1038/srep30539
26. Cho YM, Kim TH, Lim S, Choi SH, Shin HD, Lee HK, et al. Type 2 diabetes-associated genetic variants discovered in the recent genome-wide association studies are related to gestational diabetes mellitus in the Korean population. Diabetologia (2009) 52(2):253–61. doi: 10.1007/s00125-008-1196-4
27. Pantham P, Aye IL, Powell T. Inflammation in maternal obesity and gestational diabetes mellitus. Placenta (2015) 36:709–15. doi: 10.1016/j.placenta.2015.04.006
28. Lapolla A, Dalfrà MG, Sanzari M, Fedele D, Betterle C, Masin M, et al. Lymphocyte subsets and cytokines in women with gestational diabetes mellitus and their newborn. Cytochine (2005) 31:280–87. doi: 10.1016/j.cyto.2005.05.004
29. Urbanek M, Haynes MG, Lee H, Freathy RM, Lowe LP, Ackerman C, et al. The role of inflammatory pathway genetic variation on maternal metabolic phenotypes during pregnancy. PloS One (2012) 7:e32958. doi: 10.1371/journal.pone.0032958
30. Lappas M. Effect of pre-existing maternal obesity, gestational diabetes and adipokines on the expression of genes involved in lipid metabolism in adipose tissue. Metabolism (2014) 63:250–2. doi: 10.1016/j.metabol.2013.10.001
31. Barker DJ. The fetal and infant origins of adult disease. BMJ (1990) 301:1111–22. doi: 10.1136/bmj.301.6761.1111
32. Wu P, Farrel WE, Haworth KE, Emes RD, Kitchen MO, Glossop JR, et al. Maternal genome-wide DNA methylation profile in gestational diabetes shows distinctive disease-associated changes relative to matched healthy pregnancies. Epigenetics (2018) 13(2):122–8. doi: 10.1080/15592294.2016.1166321
33. Cardenas A, Gagnè-Quellet V, Allard C, Brisson D, Perron P, Bouchard L, et al. Placental DNA methylation adaptation to maternal glycemic response in pregnancy. Diabetes (2018) 67:1673–83. doi: 10.2337/db18-0123
34. Bouchard L, Hivert M, Guay SP, StPierre J, Perron P, Brisson D. Placental adiponectin gene DNA methylation levels are associated with mother’s blood glucose concentration. Diabetes (2012) 61:1272–80. doi: 10.2337/db11-1160
35. Hajj NE, Pliushch G, Schneider E, Dittrich M, Muller T, Korenov M, et al. Metabolic programming of MEST DNA methylation by intrauterine exposure to gestational diabetes mellitus. Diabetes (2013) 62:1320–8. doi: 10.2337/db12-0289
36. Haerle L, Haj N, Dittrich M, Muller T, Nanda I, Lehnen H. Epigenetic signatures of gestational diabetes mellitus on cord blood methylation. Clin Epigenet (2017) 9:28–38. doi: 10.1186/s13148-017-0329-3
37. Hjort L, Martino D, Grunnet LG, Naeem H, Maksimovic J, Olsson AH, et al. Gestational diabetes and maternal obesity are associated with epigenome-wide methylation changes in children. JCI Insight (2018) 3(17):1–14. doi: 10.1172/jci.insight.122572
38. Michalczyk AA, Dunbar JA, Janus ED, Best JD, Ebeling PR, Ackland MJ, et al. Epigenetic markers to predict conversion from gestational diabetes to type 2 diabetes. J Clin Endocrinol Metab (2016) 101:2396–404. doi: 10.1210/jc.2015-4206
40. Zhao H, Guan J, Lee HM, Sui Y, He L, Siu JJ, et al. Up-regulated pancreatic tissue micro RNA-375 associated with human type 2 diabetes through beta-cell deficit and islet amyloid deposition. Pancreas (2010) 39:843–46. doi: 10.1097/MPA.0b013e3181d12613
41. Zhao C, Dong J, Jiang T, Shi Z, Yu B, Zhu Y, et al. Early second trimester serum miRNA profiling predicts gestational diabetes mellitus. PloS One (2011) 6:23925–3. doi: 10.1371/journal.pone.0023925
42. Zhou X, Xiang C, Zheng X. MiR-132 serves as a diagnostic biomarker in gestational diabetes mellitus and its regulatory effect on trophoblast cell viability. Diagn Pathol (2019) 14:119–28. doi: 10.1186/s13000-019-0899-9
43. Zhu H, Leung SW. Identification of microRNA biomarkers in type 2 diabetes: a meta-analysis of controlled profiling studies. Diabetologia (2015) 58:900–11. doi: 10.1007/s00125-015-3510-2
44. Cao YL, Jia YJ, Xing BH, Shi DD, Dong XJ. Plasma microRNA-16-5p, 17-5p and 20a-5p: novel diagnostic biomarkers for gestational diabetes mellitus. Obstet Gynecol Res (2017) 43:974–81. doi: 10.1111/jog.13317
45. Wander PL, Boyko EJ, Hevner K, Parikin VJ, Tadesse MG, Sorensen TK, et al. Circulating early- and mid-pregnancy microRNAs and risk of gestational diabetes. Diabetes Res Clin Pract (2017) 132:1–9. doi: 10.1016/j.diabres.2017.07.024
46. Cao JL, Zhang L, Lin J, Tian S, Lv XD, Wang XQ, et al. Up-regulation of miR-98 and unravelling regulatory mechanisms in gestational diabetes mellitus. Sci Rep (2016) 6:32268–74. doi: 10.1038/srep32268
47. Ibarra A, Vega-Guedes B, Brito-Casillas Y, Wagner A. Diabetes in pregnancy and microRNAs:promises and limitations in their clinical applications. Non-coding RNA (2018) 4:1–250. doi: 10.3390/ncrna4040032
48. Psychogios N, Hau DD, Peng J, Guo AC, Mandal R, Bouatra S, et al. The human serum metabolome. PloS One (2011) 6:e16957. doi: 10.1371/journal.pone.0016957
49. Bain JR, Stevens RD, Wenner BR, Ilkayeva O, Muoio DM, Newgard CB. Metabolomics applied to diabetes research: moving from information to knowledge. Diabetes (2009) 58:2429–43. doi: 10.2337/db09-0580
50. Holmes E, Wilson ID, Nicholson JK. Metabolic phenotyping in health and disease. Cell (2008) 134:714–7. doi: 10.1016/j.cell.2008.08.026
51. Diaz SO, Pinto J, Graca G, Duarte IF, Barros AS, Galhano E, et al. Metabolic biomarkers of prenatal disorders: an exploratory NMR metabonomics study of second-trimester maternal urine and blood sample. J Proteome Res (2011) 10:3732–42. doi: 10.1021/pr200352m
52. Ferranini E, Natali A, Camastra S, Nannipieri M, Mari A, Adam KP, et al. Early metabolic markers of the development of disglycemia and type 2 diabetes and their physiological significance. Diabetes (2013) 62:1730–37. doi: 10.2337/db12-0707
53. Liu Y, Kuang A, Talbot O, Bain JR, Muehlbauer MJ, Hayes MG, et al. Metabolomic and genetic associations with insulin resistance in pregnancy. Diabetologia; (2020) 63(9):1783–95. doi: 10.1007/s00125-020-05198-1
54. Law KP, Zhang H. The pathogenesis and pathophysiology of gestational diabetes mellitus. Deduction from a three-part longitudinal metabolomics study in China. Clin Chim Acta (2017) 468:60–70. doi: 10.1016/jcca2017.02.008
55. Clauser KR, Baker P, Burlingame AL. Role of accurate mass measurement (10 ppm) in protein identification strategies employing MS or MS/MS and data base searching. Analyt Chem (1999) 71:2871–82. doi: 10.1021/ac9810516
56. Desoye G, Hauguel-de Mouzon S. The human placenta in gestational diabetes mellitus.The insulin and cytokine network. Diabetes Care (2007) 30(Supplement 2):S120–6. doi: 10.2337/dc07-s203
57. Hauguel-de Mouzon S, Desoye G. The placenta in diabetic pregnancy: new methodological approaches. In: Lapolla A, Metzger BE, editors. Gestational Diabetes: a decade after the HAPO study. Basel, Switzerland: Frontier in Diabetes Karger (2020). p. 145–54.
58. D’Aronco S, Crotti S, Agostini M, Traldi P, Chilelli NC, Lapolla A. The role of mass spectrometry in studies of glycation processes and diabetes management. Mass Spectrometry Rev (2018) 38(1):112–146. doi: 10.1002/mas.21576
59. Lapolla A, Porcu S, Roverso M, Desoye G, Cosma C, Nardelli GB, et al. A preliminary investigation on placenta protein profile reveals only modest changes in well-controlled gestational diabetes mellitus. Eur J Mass Spectrom (2013) 19:211–23. doi: 10.1255/ejms.1225
60. Burlina S, Banfi C, Brioschi M, Visentin S, Dalfrà MG, Traldi P, et al. Is the placenta proteome impaired in well-controlled gestational diabetes? J Mass Spectrom (2019) 54:359–65. doi: 10.1002/jms.4336
61. Simopoulos AP. Nutrigenetics/Nutrigenomics. Annu Rev Public Health (2010) 31:53–68. doi: 10.1146/annurev.publhealth.031809.130844
62. Franzago M, Santurbano D, Vitacolonna E, Stuppia L. Genes and diet in the prevention of chronic diseases in future generations. Int J Mol Sci (2020) 21(7):2633. doi: 10.3390/ijms21072633
63. Franzago M, Fraticelli F, Marchetti D, Celentano C, Liberati M, Stuppia L, et al. Nutrigenetic variants and cardio-metabolic risk in women with or without gestational diabetes. Diabetes Res Clin Pract (2018) 137:64–71. doi: 10.1016/j.diabres.2018.01.001
64. Franzago M, Fraticelli F, Di Nicola M, Bianco F, Marchetti D, Celentano C, et al. Early subclinical atherosclerosis in gestational diabetes: the predictive role of routine biomarkers and nutrigenetic variants. J Diabetes Res (2018) 2018:9242579. doi: 10.1155/2018/9242579
65. Schulz LC. The Duch hungher winter and the developmental origins of health and disease. Proc Natl Acad Sci USA (2010) 39:16757–8. doi: 10.1073/pnas.1012911107
66. Huang C, Li Z, Wang M, Martorell R. Early life exposure to the 1959-1961 Chinese famine has long-term heath consequences. J Nutr (2010) 140:1874–8. doi: 10.3945/jn.110.121293
67. Turner S, Klimek P, Szell M, Duftschmid G, Endel G, Kautzky-Willer A, et al. Quantification of excess risk for diabetes fro those born in times of hungher in an entire population of a nation, acoss a century. Proc Acad Sci USA (2013) 110:4703–7. doi: 10.1073/pnas.1215626110
68. Heijmans BT, Tobi EW, Stein AD, Putter H, Blauw GJ, Susser ES, et al. Persistent epigenetic differences associated with prenatal exposure to famine in humans. Proc Acad Sci USA (2008) 105:17046–9. doi: 10.1073/pnas.0806560105
69. Williams L, Seki Y, Vuguin PM, Charron MJ. Animal models of in utero exposition to an high fat diet: a review. Biochim Biophys Acta (2014) 1842:507–51. doi: 10.1016/j.bbadis.2013.07.006
70. Masuyama H, Hiramatsu Y. Effects of an high fat diet exposure in utero on the metabolic syndrome-like phenomenon in mouse offspring through epigenetic changes in adipocytochine gene expression. Endocrinology (2012) 153:2823–30. doi: 10.1210/en.2011-2161
71. Khalyfa A, Carreras A, Hakim F, Cunningham JM, Wang Y, Gozal D. Effects of late gestational high-fat diet on body weight, metabolic regulation and adipokine expression in offspring. Int J Obes (2013) 37(11):1481–9. doi: 10.1038/ijo.2013.12
72. Yu HL, Dong S, Gao LF, Li L, Xi YD, Ma WW, et al. Global DNA methylation was changed by a maternal high-lipid, high-energy diet during gestation and lactation in male adult mice liver. Br J Nutr (2015) 113(7):1032–9. doi: 10.1017/S0007114515000252
73. Masuyama H, Mitsui T, Nobumoto E, Hiramatsu Y. The effects of high-fat diet exposure in utero on the obesogenic and diabetogenic traits through epigenetic changes in adiponectin and leptin gene expression for multiple generations in female mice. Endocrinol (2015) 156(7):2482–91. doi: 10.1210/en.2014-2020
74. Sandovici I, Smith NH, Nitert MD, Ackers-Johnson M, Uribe-Lewis S, Ito Y, et al. Maternal diet and aging alter the epigenetic control of a promoter-enhancer interaction at the Hnf4a gene in rat pancreatic islets. Proc Natl Acad Sci U S A (2011) 108(13):5449–54. doi: 10.1073/pnas.1019007108
75. McPherson NO, Owens JA, Fullston T, Lane M. Preconception diet or exercise intervention in obese fathers normalizes sperm microRNA profile and metabolic syndrome in female offspring. Am J Physiol Endocrinol Metab (2015) 308(9):E805–21. doi: 10.1152/ajpendo.00013.2015
Keywords: obesity, genetic, epigenetic, gestational diabetes, mass spectrometry, nutrition
Citation: Dalfrà MG, Burlina S, Del Vescovo GG and Lapolla A (2020) Genetics and Epigenetics: New Insight on Gestational Diabetes Mellitus. Front. Endocrinol. 11:602477. doi: 10.3389/fendo.2020.602477
Received: 03 September 2020; Accepted: 02 November 2020;
Published: 01 December 2020.
Edited by:
Elena Succurro, University of Magna Graecia, ItalyReviewed by:
Andrea Mario Bolla, ASST Fatebenefratelli Sacco, ItalyMonique Francois, University of Wollongong, Australia
Copyright © 2020 Dalfrà, Burlina, Del Vescovo and Lapolla. This is an open-access article distributed under the terms of the Creative Commons Attribution License (CC BY). The use, distribution or reproduction in other forums is permitted, provided the original author(s) and the copyright owner(s) are credited and that the original publication in this journal is cited, in accordance with accepted academic practice. No use, distribution or reproduction is permitted which does not comply with these terms.
*Correspondence: Annunziata Lapolla, annunziata.lapolla@unipd.it