- Shangnan County Hospital, Shangnan County, Shangluo City, China
Heterotopic ossification (HO) is the formation of abnormal mature lamellar bone in extra-skeletal sites, including soft tissues and joints, which result in high rates of disability. The understanding of the mechanism of HO is insufficient. The aim of this study was to explore biomarkers and pathological processes in HO+ samples. The gene expression profile GSE94683 was downloaded from the Gene Expression Omnibus database. Sixteen samples from nine HO- and seven HO+ subjects were analyzed. After data preprocessing, 3,529 genes were obtained for weighted gene co-expression network analysis. Highly correlated genes were divided into 13 modules. Finally, the cyan and purple modules were selected for further study. Gene ontology functional annotation and Kyoto Encyclopedia of Genes and Genomes pathway enrichment indicated that the cyan module was enriched in a variety of components, including protein binding, membrane, nucleoplasm, cytosol, poly(A) RNA binding, biosynthesis of antibiotics, carbon metabolism, endocytosis, citrate cycle, and metabolic pathways. In addition, the purple module was enriched in cytosol, mitochondrion, protein binding, structural constituent of ribosome, rRNA processing, oxidative phosphorylation, ribosome, and non-alcoholic fatty liver disease. Finally, 10 hub genes in the cyan module [actin related protein 3 (ACTR3), ADP ribosylation factor 4 (ARF4), progesterone receptor membrane component 1 (PGRMC1), ribosomal protein S23 (RPS23), mannose-6-phosphate receptor (M6PR), WD repeat domain 12 (WDR12), synaptosome associated protein 23 (SNAP23), actin related protein 2 (ACTR2), siah E3 ubiquitin protein ligase 1 (SIAH1), and glomulin (GLMN)] and 2 hub genes in the purple module [proteasome 20S subunit alpha 3 (PSMA3) and ribosomal protein S27 like (RPS27L)] were identified. Hub genes were validated through quantitative real-time polymerase chain reaction. In summary, 12 hub genes were identified in two modules that were associated with HO. These hub genes could provide new biomarkers, therapeutic ideas, and targets in HO.
Introduction
Heterotopic ossification (HO) is the formation of abnormal mature lamellar bone in extra-skeletal sites, including soft tissues and joints (1). HO was first described 1,000 years ago in the healing of fractures (2). HO is a frequent complication associated with arthroplasties [occurs in up to 40% of cases (3)], traumatic brain and spinal cord injuries [occurs in up to 50% of cases (3, 4)], extensive burns, severe trauma and combat-related extremity injuries (5). Other research reveals that up to 75% of HO cases are associated with trauma (6).
To date, the only available prophylactic treatments for advanced HO are nonsteroidal anti-inflammatory drug (NSAID) treatments and localized low-dose irradiation. However, their action is not against precise molecular biological and genetic targets (7). At later stages, surgical excision is the only effective procedure for treatment, which includes many risks, such as wound healing complications, delayed therapy, rehabilitation, and recurrence (5). Therefore, early diagnosis, and new and effective therapeutic strategies urgently need to be developed.
Due to the development of genetic testing technology in recent years, an increasing number of studies focus on the inhibition of local factors and signaling pathways, such as bone morphogenetic protein (BMP) inhibitors, like noggin (8), BMP type 1 receptor inhibitor (8, 9), and nuclear retinoid acid receptor-gamma (RARγ) agonists (10). These inhibitors catalyze ectopic bone formation to provide earlier diagnoses and to develop treatments that are more effectives (11). Weighted gene co-expression network analysis (WGCNA), a new systems biology method, has been developed to analyze gene expression microarray profiling data (12). WGCNA describes how genes work interactively and provides insights into the correlation between heterogeneous traits and genetics on the basis of genetic networks (12). It has been widely used in identifying biomarkers and candidate genes in complex diseases (13). Thus, based on expression profiling of array data, WGCNA was applied to analyze the GSE94683 datasets in the Gene Expression Omnibus (GEO) database to identify specific HO-related genes, and provided an opportunity to improve the understanding of HO.
Materials and Methods
Data Collection and Preprocessing
The expression profiling of the array dataset GSE94683, which is a transcriptome analysis of HO mesenchymal stromal cells (MSCs), was shared on the GEO database (https://www.ncbi.nlm.nih.gov/geo/query/acc.cgi?acc=GSE94683). The research was performed on 16 individuals (9 HO- and 7 HO+) based on the GPL10630 platform. Transcriptome analysis was performed on mesenchymal stromal cells from HOs and amplified in vitro after two passages. Agilent Whole Human Genome Oligo Microarrays were used to compare expression profiles of MSCs from HO- bone marrow of healthy donors. Series matrix files and the platform file were downloaded (https://www.ncbi.nlm.nih.gov/geo/query/acc.cgi?acc=GSE94683). The series matrix file was preprocessed to identify differentially expressed genes (DEGs) using GEO2R online (https://www.ncbi.nlm.nih.gov/geo/geo2r/?acc=GSE94683) (14, 15). The probe ID was converted into a gene symbol through the platform file and the series matrix file.
WGCNA on DEGs
The “WGCNA” package (12) in R software (version 4.0.0) was used for the network construction. Samples were corrected with limma package using the normalizeBetweenArrays function. A log2 transform was applied to the expression matrix of DEGs. Check missing values and filter (meanFPKM = 0.5) was conducted to evaluate the expression matrix file. Finally, a total of 3529 genes were obtained for subsequent analysis. WGCNA was used to cluster the DEGs into differently colored modules using the dissimilarity measure (1-topological overlap measure (TOM), TOM ≥ 0.15) (16). If the eigenvalue correlation coefficients of different modules were greater than 0.25, the different modules were integrated into one module (17). Module−trait relationships (p < 0.05) were used to determine HO-related modules. GeneTraitSignificance (GS) and geneModuleMembership (MM) were determined after relating modules to external clinical traits. All merged modules were exported for further research.
Gene Ontology (GO) and Pathway Enrichment Analysis of HO-Related Modules
HO-related modules were analyzed online using the database for annotation, visualization and integrated discovery (https://david.ncifcrf.gov/summary.jsp) (18) for GO functional annotation and Kyoto Encyclopedia of Genes and Genomes (KEGG) pathway enrichment analysis; p < 0.05 indicated significant differences. Then, GO was visualized using ggplot2 and the Cairo package in the R software, while KEGG was visualized using stringr and the Cairo package in the R software.
Protein–Protein Interaction (PPI) Network Construction
The online search tool for the retrieval of interacting genes (STRING; version 11.0; www.string-db.org) provides information for known and predicted PPIs (19). In this study, STRING was used to analyze the PPIs among HO-related modules. All genes in the HO-related modules were analyzed using the STRING online website. A confidence score >0.7 has been chosen to construct the PPI network in Cytoscape software (Version 3.7.2) (20). Genes with a node degree >10 are considered to be the key genes in the PPI network (15).
Identification of Hub Genes
The key genes in HO-related modules have been determined through an absolute value of the MM >0.8 and GS >0.2 (15). PPI network key genes were determined using a node degree >10. The genes that were common components between key genes in HO-related modules and key genes in the PPI network were considered to be hub genes and were visualized using Venn diagrams (results were reconstructed by Excel 2016) (21).
HO and Normal Sample Collection, Separating MSCs, Extraction of Total RNA, and Quantitative Real-time Polymerase Chain Reaction (qRT-PCR)
Four HO+ samples came from four HO patients (8 sample characteristic show in Table 1) surgically treated at the Shangnan County Hospital, Shangnan County, Shaanxi Province, China. Four normal (HO-) samples came from the bone marrow of four healthy donors at Shangnan County Hospital. After tissue harvested, the tissues of HO ample were separated into 1 mm3 and digested by collagenase 2. MSCs were separated by gradient centrifugation through Percoll (22, 23) (density 1.073 g/ml, Solarbio, China). MSCs were cultured in dulbecco’s modified eagle medium containing 10% fetal bovine serum (Bioind, Kibbutz Beit Haemek, Israel), 1% nonessential amino acid (Hyclone, Logan, UT, USA), 1% antibiotics (Sigma, St. Louis, MO, USA), 10 ng/ml epidermal growth factor (Gibco, Carslbad, CA, USA), 10 ng/ml fibroblast growth factor (Gibco, Carslbad, CA, USA) (24), and were trypsinized once confluent and propagated to 2 passage. After harvested MSCs were used to extract total RNA using the TRIzol™ reagent (Invitrogen, USA), according to the manufacturer’s protocol. Total RNA of all samples was stored in liquid nitrogen after extraction. For each sample, 1 μg of RNA was converted to cDNA using a First Strand cDNA Synthesis Kit (Takara Bio, Dalian, China). All primers (12 hub genes and β-actin) are shown in Supplementary Table 1, and have been designed and validated by the Takara Biological Company. Template cDNA (10 ng/rxn), SYBR Green fluorescent reagent (Tli RNaseH Plus, Takara Bio, Dalian, China) and a Roche LightCycler® 480 II (Roche, Switzerland) were utilized for qRT-PCR analysis. The reaction conditions were as follows: an initial denaturation at 95°C for 5 min, denaturation at 95°C for 30 s, annealing at 58°C for 30 s, extension at 72°C for 30 s, for a total of 45 cycles. Relative gene expression was calculated using the 2 −△△CT method with β-actin (ACTB) as the endogenous control gene. This study was approved by the ethics committee of Shangnan County Hospital. The study was conducted in accordance with the Declaration of Helsinki and all patients provided written informed consent before the study. All data were de-identified, presented as the mean ± standard deviation (SD), and analyzed using GraphPad Prism 8.01 software (GraphPad Software, USA). Statistical significance was determined using the Student’s t-test and p <0.05 was considered a significant difference.
Results
Processing of DEGs and Co-Expression Network Construction
The GEO2R online analysis tool was used to detect DEGs between HO+ and HO- samples, and then the adjusted p-value (adjusted using the Benjamini & Hochberg method) was calculated. Genes that met the cutoff criteria, adjusted p <0.05, were considered to be HO-related DEGs. Through check missing value and filter, a total of 3,529 genes were selected as HO-related DEGs. All HO-related DEGs were analyzed in R software through the WGCNA package. There are no sample outliers depicted in Figure 1. In this study, the soft-thresholding parameter has been determined as β = 9, where the curve first reached Rˆ2 = 0.9, to construct a weighted network based on a scale-free topology criterion (Figure 2) (15). As shown in Figure 3, 22 modules are detected by the dynamic tree cutting method, then 22 modules are merged into 13 new modules through eigenvalue correlation coefficients of different modules greater than 0.25 (17).
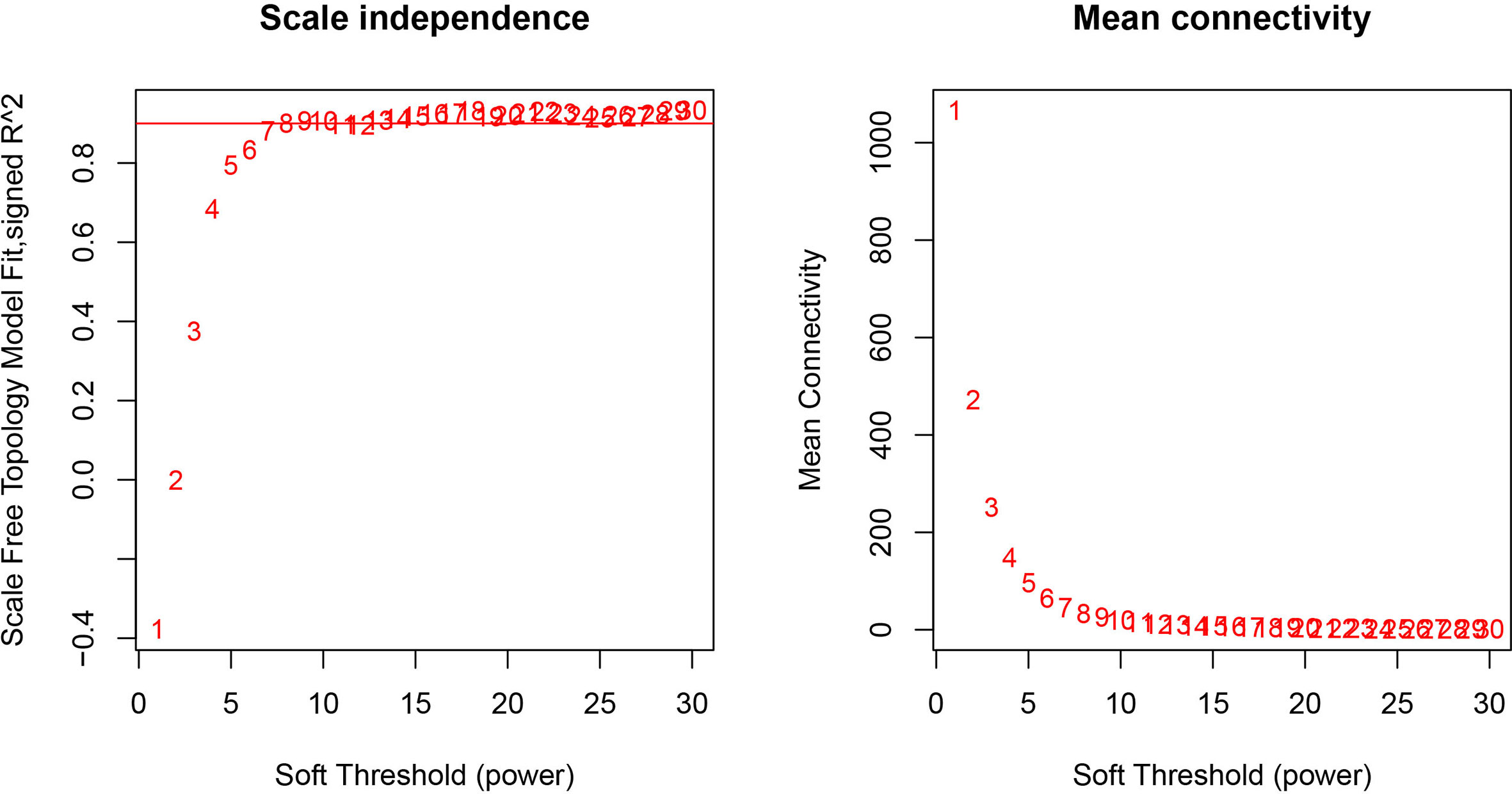
Figure 2 Analysis of network topology for various soft-thresholding powers. The left panel shows the scale-free fit index, signed Rˆ 2 (y-axis) and the soft threshold power (x-axis). β = 9 has been chosen for subsequent analysis. The right panel shows the mean connectivity (y-axis), which is a strictly decreasing function of the power β (x-axis).
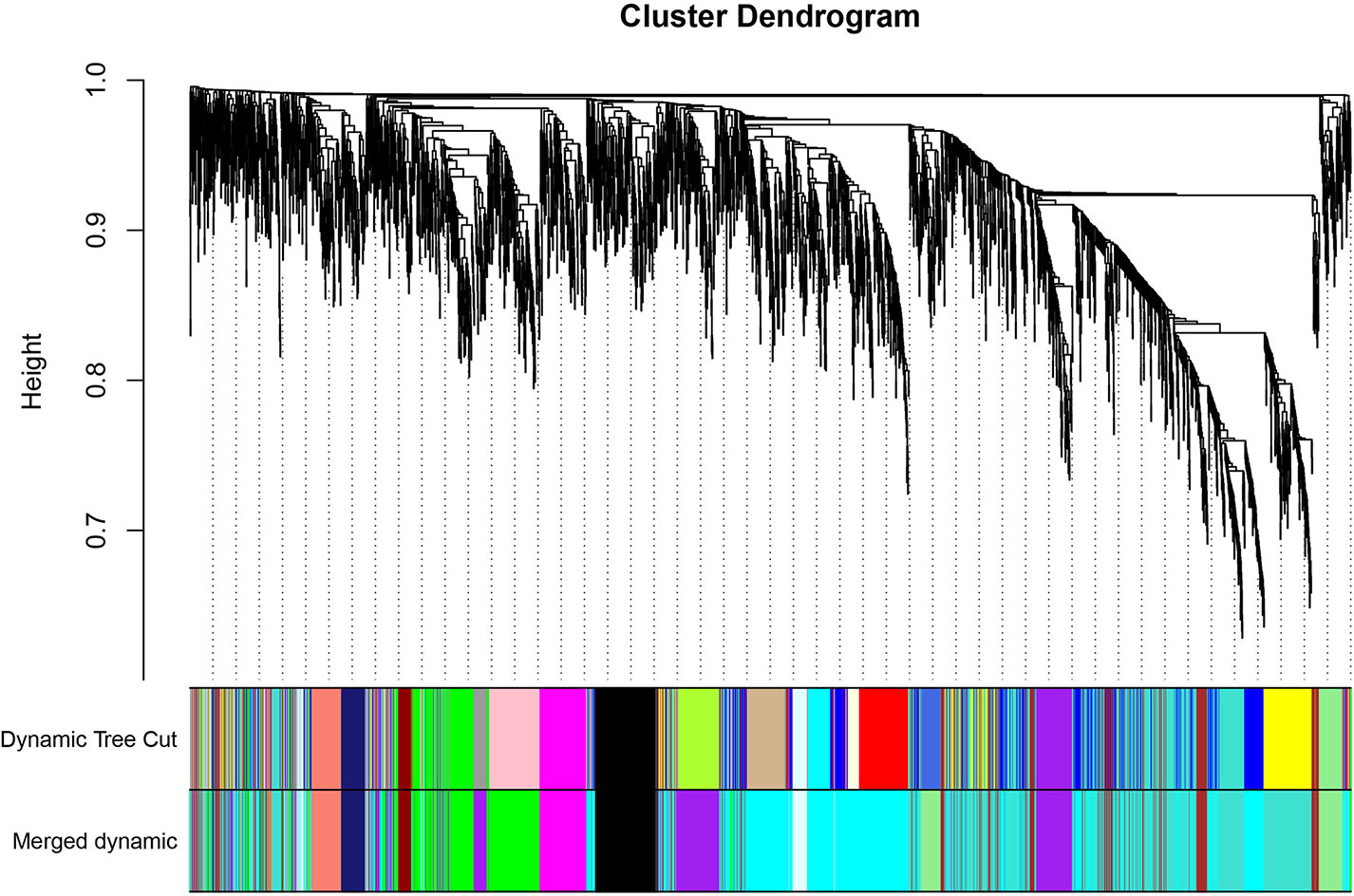
Figure 3 Clustering dendrogram of genes. The color bands provide a simple visual comparison of module (and merged dynamic) assignments (branch cuttings) based on the dynamic tree cutting method.
Identification of HO-Related Modules and Functional Annotation
After relating modules to traits, high correlations were observed in HO traits (Figure 4). Four modules (cyan r = 0.6, p = 0.01; magenta r = 0.56, p = 0.02; purple r = 0.74, p = 0.001; and brown r = 0.77, p = 5e−04) were selected as HO-related modules through the p < 0.05 criterion. Because the brown and magenta modules did not have a meaningful functional annotation analysis result, the brown and magenta modules were removed for subsequent analysis. Figure 5A shows that the cyan module is enriched in a variety of components, including protein binding, membrane, nucleoplasm, cytosol, and poly(A) RNA binding, based on GO analysis (p < 0.001). Figure 5B shows that the cyan module includes genes involved in the biosynthesis of antibiotics, carbon metabolism, endocytosis, citrate cycle (TCA cycle), and metabolic pathways, based on KEGG pathway analysis (p < 0.05). Figure 6A shows that the purple module is enriched in a variety of components, including cytosol, mitochondrion, protein binding, structural constituent of the ribosome, and rRNA processing, based on GO analysis (p < 0.001). Figure 6B shows that the purple module includes genes involved in oxidative phosphorylation, ribosome, non-alcoholic fatty liver disease (NAFLD), Huntington’s disease, and Alzheimer’s disease, based on KEGG pathway analysis (p < 0.05). Finally, the cyan module, with 887 genes, and the purple module, with 365 genes, were deemed to be clinically significant modules with HO associations.
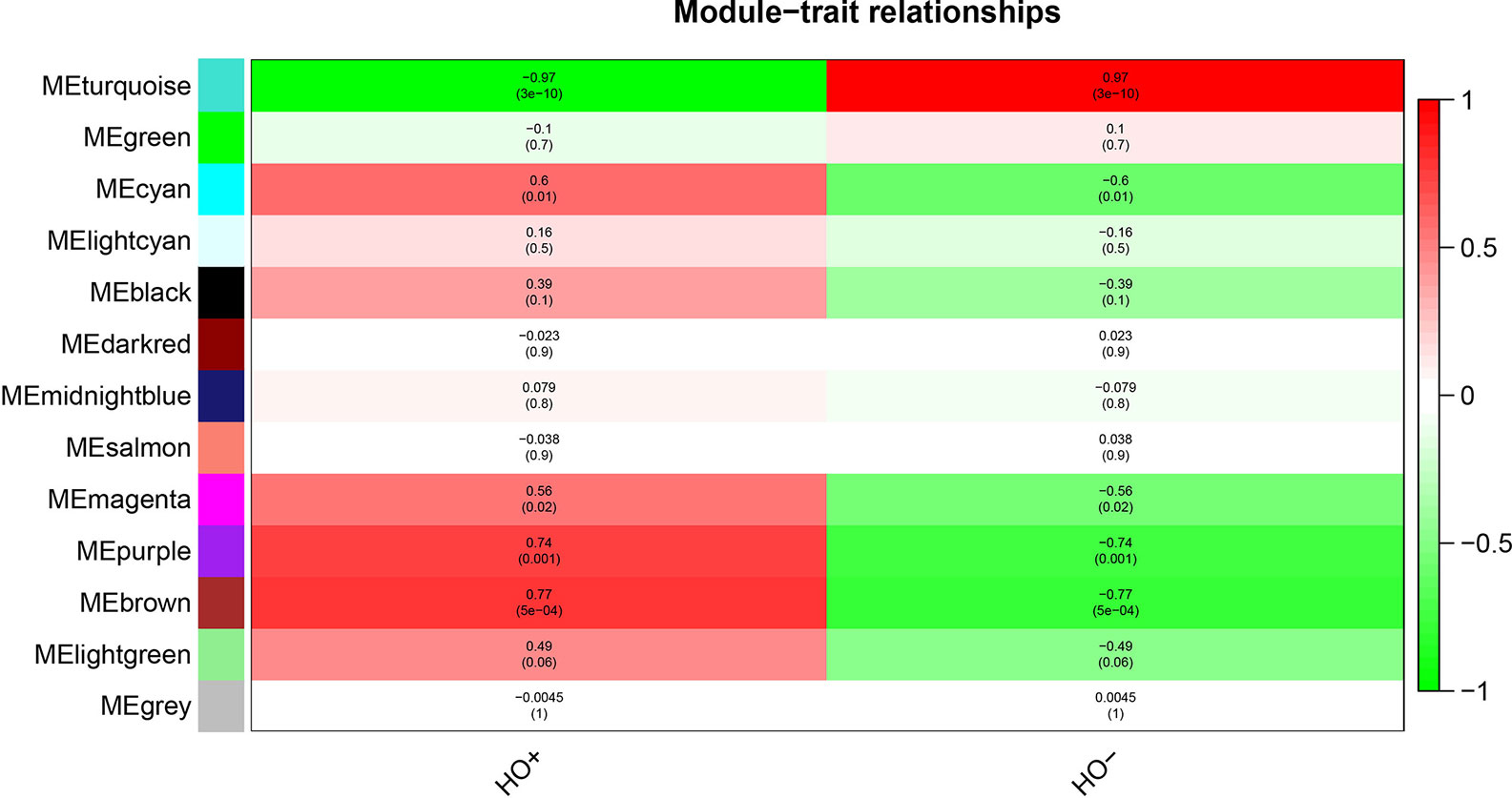
Figure 4 Module-trait relationships show that cyan, magenta, purple, and brown modules are related to HO.
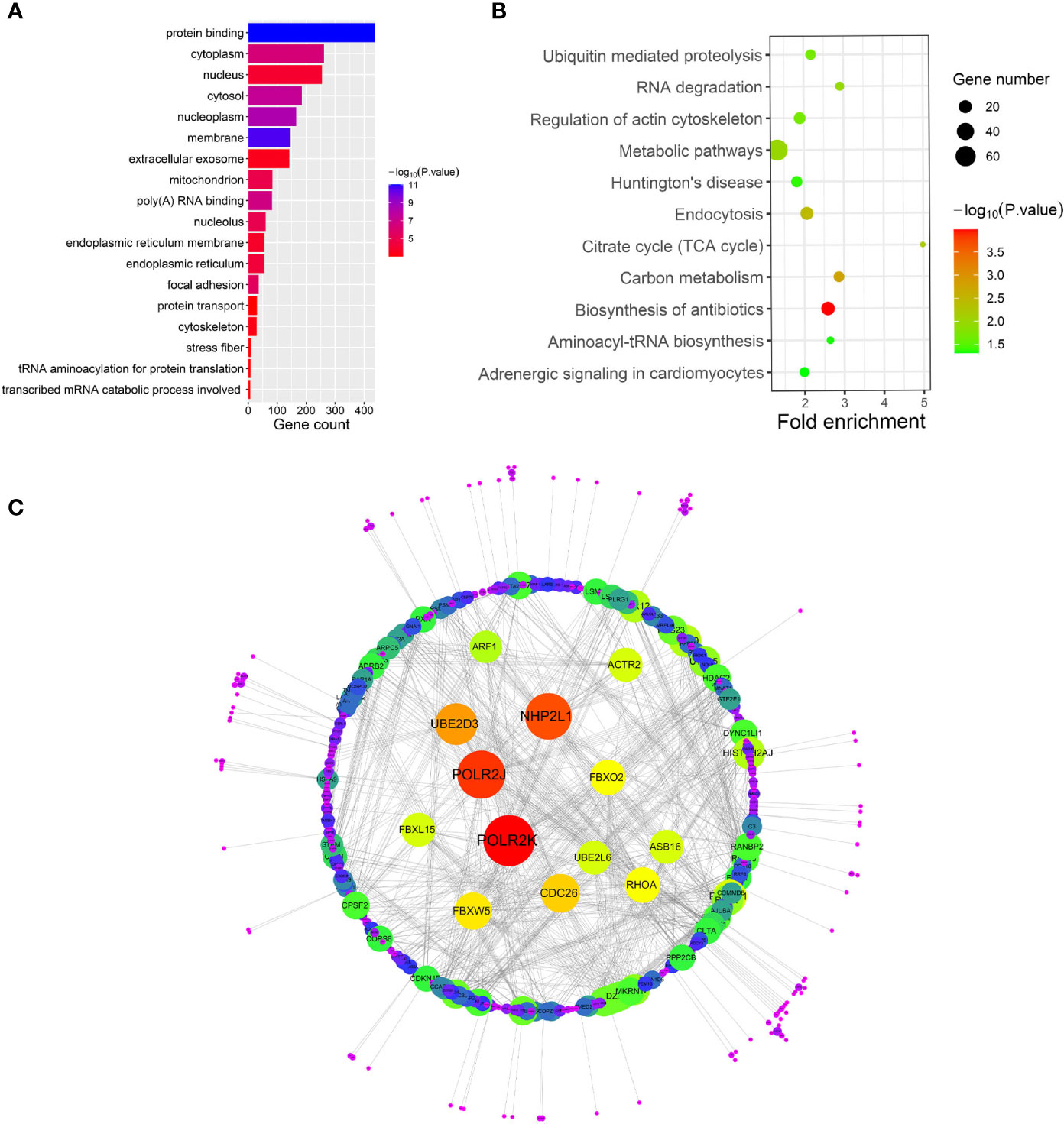
Figure 5 (A–C) show gene ontology (GO), Kyoto Encyclopedia of Genes and Genomes (KEGG) pathway and protein-protein interaction (PPI) analyses of the cyan module, respectively.
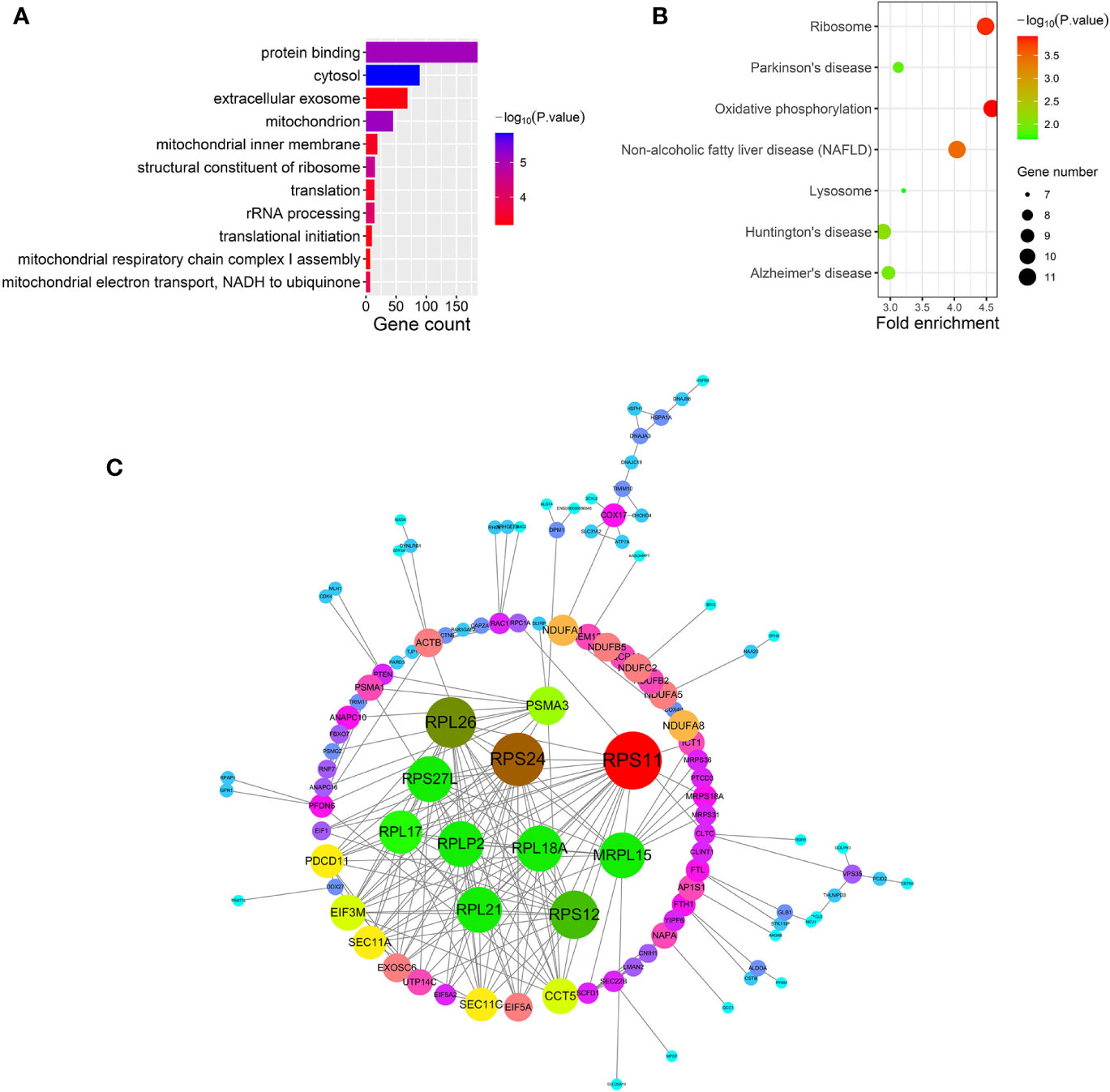
Figure 6 (A–C) show gene ontology (GO), Kyoto Encyclopedia of Genes and Genomes (KEGG) pathway and protein-protein interaction (PPI) analyses of the purple module, respectively.
PPI Network Analysis
To investigate the interactive relationships among DEGs, we submitted the DEGs to the STRING database with a combined score >0.7. Cytoscape software has been used to construct PPI networks (20). Nodes with a higher degree of connectivity tend to be more essential in the functional network (15). The genes with a node degree >10 were identified as key genes in the PPI analysis. The PPI network in the cyan module is shown in Figure 5C; there are 76 key genes in the cyan module by PPI analysis, as shown in Supplementary Table 2. The PPI network in the purple module is shown in Figure 6C; there are 13 key genes in the purple module using PPI analysis, as shown in Supplementary Table 3.
Identification of Hub Genes
Based on the absolute value of the MM >0.8 and GS >0.2, 130 key genes and 59 key genes were filtered from the cyan and purple modules, respectively (Supplementary Tables 4 and 5). The final hub genes are considered to be common genes between key genes in the cyan and purple modules and key genes in the cyan and purple PPI analysis (15). Through Venn diagram analysis, there were 10 hub genes [actin related protein 3 (ACTR3), ADP ribosylation factor 4 (ARF4), progesterone receptor membrane component 1 (PGRMC1), ribosomal protein S23 (RPS23), mannose-6-phosphate receptor (M6PR), WD repeat domain 12 (WDR12), synaptosome associated protein 23 (SNAP23), actin related protein 2 (ACTR2), siah E3 ubiquitin protein ligase 1 (SIAH1), and glomulin (GLMN)] in the cyan module (Figure 7A) and 2 hub genes in the purple module [proteasome 20S subunit alpha 3 (PSMA3) and ribosomal protein S27 like (RPS27L)] (Figure 7B).
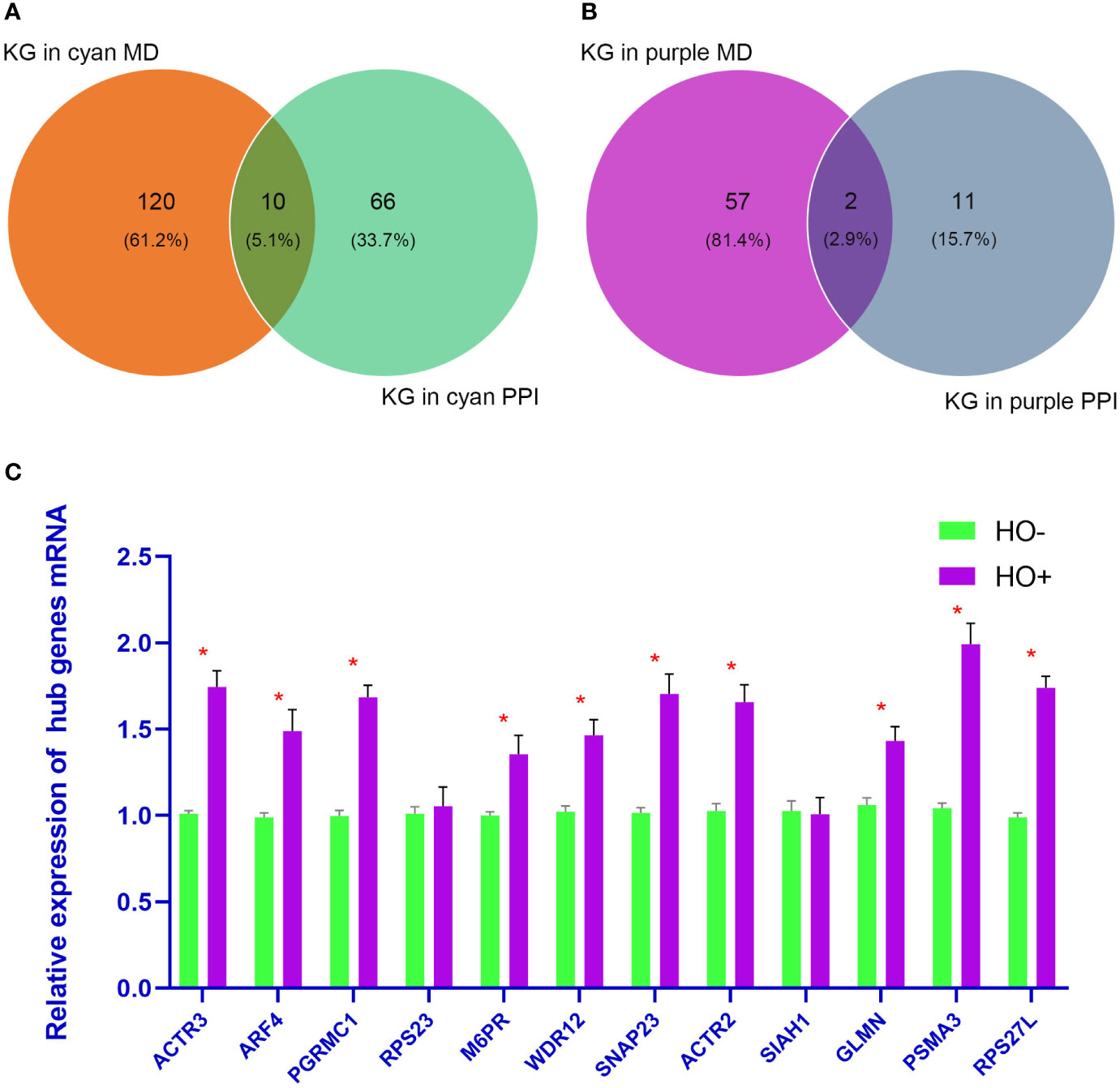
Figure 7 (A, B) show the Venn diagram analysis of the cyan and purple modules, respectively. (C) shows the quantitative real-time polymerase chain reaction analysis of 12 hub genes. p <0.05 is considered statistically significant. KG, Key genes; MD, modules.
Validation of Hub Genes through qRT-PCR
Four HO tissue samples and four normal samples were used to evaluate the expression levels of the 12 hub genes by qRT-PCR analysis. As shown in Figure 7C, mRNA levels of ACTR3, ARF4, PGRMC1, M6PR, WDR12, SNAP23, ACTR2, GLMN, PSMA3, and RPS27L were significantly upregulated in HO than in normal samples (p < 0.05). However, the other two genes (RPS23 and SIAH1) showed no significant difference.
Discussion
Because of the high incidence [up to 90% after certain types of hip arthroplasty or acetabular fractures (3, 25, 26)], low quality of life, and the resulting disability caused by HO, many studies have tried to identify causal factors from the mechanism of injury, management of wounds and complications, and the subsequent formation of HO (2, 27). The detailed molecular biological mechanism is not fully understood. The treatment of HO is restricted to the use of NSAIDs, radiation, and surgical excision (2). Although NSAIDs have demonstrated prophylactic efficacy against HO, it is confirmed that HO prophylaxis with indomethacin increases the risk of long bone nonunion (28, 29). The existing literature shows that rates of HO after hip arthroplasty decrease to 25% after radiation therapy compared with a range of approximately 5 to 90% before treatment (30, 31). However, radiation therapy has not been adequately studied at other joints than the hip (32). Additional side effects have been reported in a prospective, randomized study, including progressive soft-tissue contracture, delayed wound-healing, nonunion, inhibited ingrowth of press-fit hip implants, risk of malignancy, and others (32, 33). Surgical excision is also effective therapy for the treatment of previously existing HO, but has risks of delayed wound healing, infection, nerve injury, and recurrent contracture (34, 35). The investigation of pathological processes, clinical manifestation, diagnosis, treatment, and prevention is particularly essential and urgent.
In this study, HO-related biomarkers and pathological processes were explored using the WGCNA algorithm in R software. Through WGCNA analysis, it was found that both the cyan and purple modules were closely related to HO. Specifically, the cyan module containing 887 genes played a key role in protein binding, membrane, nucleoplasm, cytosol, and poly(A) RNA binding. The cyan module contained components enriched in biosynthesis of antibiotics, carbon metabolism, endocytosis, citrate cycle (TCA cycle), metabolic pathways, and signaling pathways. Ten hub genes (ACTR3, ARF4, PGRMC1, RPS23, M6PR, WDR12, SNAP23, ACTR2, SIAH1, and GLMN) were selected by combining the PPI network analysis with that of genes identified in the cyan module.
A previous study showed that the TCA cycle and metabolic pathways are significantly changed in mice HO modules (36). However, this has not been reported in humans. Another recent bioinformatics study showed that HO-related DEGs are mainly associated with metabolic processes in a mouse burn/tenotomy-induced HO model (37), but this has not been reported in humans. ACTR2 and ACTR3 are components of the seven-subunit Arp2/3 complex, which plays a key role in generating branched actin filament networks during many different cellular processes (38). In recent studies, ACTR3 has been confirmed to play key roles in the early immunodiagnosis of lung cancer and cholangiocarcinoma (39, 40); there is no evidence that ACTR2 and ACTR3 are related to HO. ARF4 is a component of osteogenic transcription factors, and is a necessary transcription factor for gene expression of bone matrix proteins (osteoblast markers), such as osteocalcin (41, 42). ARF4 plays key roles in the first and final stages of osteoblastic differentiation (42). Osteoblast differentiation is a key biological process for the occurrence and development of HO. Other research showed that ARF proteins control tumor proliferation and metastasis (43). Whether ARF4 protein plays a role in the proliferation of heterotopic ossification cells remains to be verified. Therefore, ARF4 may play key roles in HO development. PGRMC1/Sigma-2 receptor is confirmed to play key roles in the function of tumor proliferation (such as breast tumors, lung adenocarcinoma cells, and ovarian cancer) and chemoresistance (44–47). Recent research showed that PGRMC1 significantly suppresses the hydrogen peroxide-induced oxidative stress response in full-thickness fetal membrane explants and chorion cells (48). Another study showed that oxidative stress plays an important role in cancer bone demineralization, aortic valve mineralization, and HO in disease development (49). Thus, PGRMC1 may work in HO by regulating the oxidative stress response. RPS23 is involved in the protein synthesis processes and progression of disc degeneration (DD) (50), suggesting its potential use in the diagnosis and therapy of DD. Another study showed that RPS23 is a hub gene in gastric cancer (51). There is no evidence that RPS23 is related to HO. Similar to RPS23, it was found that M6PR, WDR12, SIAH1, and GLMN did not have a relationship with HO in previous studies. A previous study showed that SNAP23 expression is detected in osteoblastic cells (52). However, there was no evidence showing that SNAP23 influences the HO process.
The purple module had 365 genes that played key roles in cytosol, mitochondrion, protein binding, structural constituent of ribosome, and rRNA processing. The purple module also contained components enriched in oxidative phosphorylation, ribosome, NAFLD, lysosome, and signaling pathways. Two hub genes (PSMA3 and RPS27L) were selected by combining the PPI network analysis with genes identified in the purple module. A recent bioinformatics study showed that HO-related DEGs are mainly associated with oxidative phosphorylation in a mouse burn/tenotomy-induced HO model (34), but in humans, this has not been confirmed. An interesting study showed that RPS27L is significantly upregulated after irradiation in human peripheral blood (53). Another study showed that RPS27L is a physiological regulator of p53 that suppresses genomic instability and tumorigenesis (54). Radiation therapy is an effective method in the treatment of HO, possibly regulated by RPS27L. We speculate that regulating RPS27L expression may achieve the same effect as radiation therapy. Previous research has shown overexpression of RPS27L within the physiological inducible levels promoted, whereas siRNA silencing of RPS27L inhibited, apoptosis induced by etoposide (55). Another research has shown reduced apoptosis of osteoprogenitors may be responsible for increased osteogenesis in severely-injured patients (56). In a previous study, PSMA3 is not related to HO.
In this study, the qRT-PCR analysis showed that 10 hub genes (ACTR3, ARF4, PGRMC1, M6PR, WDR12, SNAP23, ACTR2, GLMN, PSMA3, and RPS27L) had significance between HO and normal groups, and further verifies the reliability of the WGCNA analysis.
It is worth noting that in this study, 8 (ACTR3, ARF4, PGRMC1, RPS23, WDR12, ACTR2, SIAH1, and RPS27L) of 12 hub genes are related to cancers, such as lung cancer, epithelial ovarian cancer, gastric cancer, glioblastoma, breast cancer, and esophageal cancer (51, 54, 57–63). A last year’s research showed pulmonary adenocarcinoma cells around the ossification expressed bone morphogenetic protein-2 and osteopontin, which generally induce and stimulate bone formation (64, 65). Another research showed overexpression of BMP-2 promote HO form in rectal cancer (66). Another research showed histogenesis of HO in this case of metastatic colon cancer is from the stromal cells in the tumoral microenvironment (67). HO occurs in a variety of tumors, thus, HO may have a similar molecular biology process as cancer.
There are several highlights of this study. First, this study is the first where MSCs were used for bioinformatics analysis of HO. Studying MSCs could contribute to a comprehensive understanding of HO. Second, WGCNA, which is used for the first time in HO analysis, has an advantage in processing gene expression datasets. This study not only confirmed the findings of previous studies, but also discovered new biomarkers for the further study of HO. However, the current study also has limitations. The sample size is small because there is no suitable data for analysis in the existing database, animal experimental verification has not been performed, and there are no additional clinical data (such as gender and age) in the original data. Future studies will further investigate HO in animal models. At last, we provide workflow showed in Figure 8.
Conclusions
In this study, the WGCNA algorithm was used to process gene expression datasets and identified a cyan module with 10 hub genes and a purple module with 2 hub genes associated with HO. Targeting these hub genes could improve the understanding of the pathological processes of HO and provide new therapeutic ideas and targets.
Data Availability Statement
The datasets presented in this article are available at https://www.ncbi.nlm.nih.gov/geo/query/acc.cgi?acc=GSE94683
Ethics Statement
The studies involving human participants were reviewed and approved by Ethics Committee of Shangnan County Hospital and the ethics committee Shangnan County Hospital is affiliated to Shangnan County Hospital. The patients/participants provided their written informed consent to participate in this study. Written informed consent was obtained from the individual(s) for the publication of any potentially identifiable images or data included in this article.
Author Contributions
JT, JW, SL, and LK conceived and designed the study. SW collected the data and wrote the manuscript. CS and JY performed the data analysis. LW and SW contributed to the language editing. All authors contributed to the article and approved the submitted version.
Conflict of Interest
The authors declare that the research was conducted in the absence of any commercial or financial relationships that could be construed as a potential conflict of interest.
Supplementary Material
The Supplementary Material for this article can be found online at: https://www.frontiersin.org/articles/10.3389/fendo.2020.581768/full#supplementary-material
References
1. Crowgey EL, Wyffels JT, Osborn PM, Wood TT, Edsberg LE. A Systems Biology Approach for Studying Heterotopic Ossification: Proteomic Analysis of Clinical Serum and Tissue Samples. Genomics Proteomics Bioinformatics (2018) 16(3):212–20. doi: 10.1016/j.gpb.2018.04.006
2. Edwards DS, Clasper JC. Heterotopic ossification: a systematic review. J R Army Med Corps (2015) 161(4):315–21. doi: 10.1136/jramc-2014-000277
3. Bedi A, Zbeda RM, Bueno VF, Downie B, Dolan M, Kelly BT. The incidence of heterotopic ossification after hip arthroscopy. Am J Sports Med (2012) 40(4):854–63. doi: 10.1177/0363546511434285
4. Forsberg JA, Pepek JM, Wagner S, Wilson K, Flint J, Andersen RC, et al. Heterotopic ossification in high-energy wartime extremity injuries: prevalence and risk factors. J Bone Joint Surg Am (2009) 91(5):1084–91. doi: 10.2106/JBJS.H.00792
5. Dey D, Wheatley BM, Cholok D, Agarwal S, Yu PB, Levi B, et al. The traumatic bone: trauma-induced heterotopic ossification. Transl Res (2017) 186:95–111. doi: 10.1016/j.trsl.2017.06.004
6. Nuovo MA, Norman A, Chumas J, Ackerman LV. Myositis ossificans with atypical clinical, radiographic, or pathologic findings: a review of 23 cases. Skeletal Radiol (1992) 21(2):87–101. doi: 10.1007/bf00241831
7. Legosz P, Drela K, Pulik L, Sarzynska S, Maldyk P. Challenges of heterotopic ossification-Molecular background and current treatment strategies. Clin Exp Pharmacol Physiol (2018) 45(12):1229–35. doi: 10.1111/1440-1681.13025
8. Hannallah D, Peng H, Young B, Usas A, Gearhart B, Huard J. Retroviral delivery of Noggin inhibits the formation of heterotopic ossification induced by BMP-4, demineralized bone matrix, and trauma in an animal model. J Bone Joint Surg Am (2004) 86(1):80–91. doi: 10.2106/00004623-200401000-00013
9. Pacifici M. Acquired and congenital forms of heterotopic ossification: new pathogenic insights and therapeutic opportunities. Curr Opin Pharmacol (2018) 40:51–8. doi: 10.1016/j.coph.2018.03.007
10. Shimono K, Tung WE, Macolino C, Chi AH, Didizian JH, Mundy C, et al. Potent inhibition of heterotopic ossification by nuclear retinoic acid receptor-γ agonists. Nat Med (2011) 17(4):454–60. doi: 10.1038/nm.2334
11. Ranganathan K, Loder S, Agarwal S, Wong VW, Forsberg J, Davis TA, et al. Heterotopic Ossification: Basic-Science Principles and Clinical Correlates. J Bone Joint Surg Am (2015) 97(13):1101–11. doi: 10.2106/JBJS.N.01056
12. Langfelder P, Horvath S. WGCNA: an R package for weighted correlation network analysis. BMC Bioinformatics (2008) 9:559. doi: 10.1186/1471-2105-9-559
13. Morrow JD, Zhou X, Lao T, Jiang Z, DeMeo DL, Cho MH, et al. Functional interactors of three genome-wide association study genes are differentially expressed in severe chronic obstructive pulmonary disease lung tissue. Sci Rep (2017) 7:44232. doi: 10.1038/srep44232
14. Xu Y, Shen K. Identification of potential key genes associated with ovarian clear cell carcinoma. Cancer Manag Res (2018) 10:5461–70. doi: 10.2147/CMAR.S187156
15. Mo X, Su Z, Yang B, Zeng Z, Lei S, Qiao H. Identification of key genes involved in the development and progression of early-onset colorectal cancer by co-expression network analysis. Oncol Lett (2020) 19(1):177–86. doi: 10.3892/ol.2019.11073
16. Kogelman LJ, Kadarmideen HN. Weighted Interaction SNP Hub (WISH) network method for building genetic networks for complex diseases and traits using whole genome genotype data. BMC Syst Biol (2014) 8(Suppl 2):S5. doi: 10.1186/1752-0509-8-s2-s5
17. Xiao KW, Li JL, Zeng ZH, Liu ZB, Hou ZQ, Yan X, et al. Monocytes affect bone mineral density in pre- and postmenopausal women through ribonucleoprotein complex biogenesis by integrative bioinformatics analysis. Sci Rep (2019) 9(1):17290. doi: 10.1038/s41598-019-53843-6
18. Huang da W, Sherman BT, Lempicki RA. Systematic and integrative analysis of large gene lists using DAVID bioinformatics resources. Nat Protoc (2009) 4(1):44–57. doi: 10.1038/nprot.2008.211
19. Szklarczyk D, Franceschini A, Wyder S, Forslund K, Heller D, Huerta-Cepas J, et al. STRING v10: protein-protein interaction networks, integrated over the tree of life. Nucleic Acids Res (2015) 43(Database issue):D447–52. doi: 10.1093/nar/gku1003
20. Shannon P, Markiel A, Ozier O, Baliga NS, Wang JT, Ramage D, et al. Cytoscape: a software environment for integrated models of biomolecular interaction networks. Genome Res (2003) 13(11):2498–504. doi: 10.1101/gr.1239303
21. Oliveros JC. Venny. An interactive tool for comparing lists with Venn’s diagrams. (2007-2015). https://bioinfogp.cnb.csic.es/tools/venny/index.html.
22. Collins JJ, Möbius MA, Thébaud B. Isolation of CD146+ Resident Lung Mesenchymal Stromal Cells from Rat Lungs. J Vis Exp (2016) 112). doi: 10.3791/53782
23. Zhao Y, Cai SX. [Isolation, culture and differentiation into endothelial-like cells from rat bone marrow mesenchymal stem cells in vitro]. Zhongguo Ying Yong Sheng Li Xue Za Zhi (2010) 26(1):60–5.
24. Cao X, Luo D, Li T, Huang Z, Zou W, Wang L, et al. MnTBAP inhibits bone loss in ovariectomized rats by reducing mitochondrial oxidative stress in osteoblasts. J Bone Miner Metab (2020) 38(1):27–37. doi: 10.1007/s00774-019-01038-4
25. Rath E, Sherman H, Sampson TG, Ben Tov T, Maman E, Amar E. The incidence of heterotopic ossification in hip arthroscopy. Arthroscopy (2013) 29(3):427–33. doi: 10.1016/j.arthro.2012.10.015
26. Chen HC, Yang JY, Chuang SS, Huang CY, Yang SY. Heterotopic ossification in burns: our experience and literature reviews. Burns (2009) 35(6):857–62. doi: 10.1016/j.burns.2008.03.002
27. Brown KV, Dharm-Datta S, Potter BK, Etherington J, Mistlin A, Hsu JR, et al. Comparison of development of heterotopic ossification in injured US and UK Armed Services personnel with combat-related amputations: preliminary findings and hypotheses regarding causality. J Trauma (2010) 69(Suppl 1):S116–22. doi: 10.1097/TA.0b013e3181e44cc7
28. Sagi HC, Jordan CJ, Barei DP, Serrano-Riera R, Steverson B. Indomethacin prophylaxis for heterotopic ossification after acetabular fracture surgery increases the risk for nonunion of the posterior wall. J Orthop Trauma (2014) 28(7):377–83. doi: 10.1097/bot.0000000000000049
29. Burd TA, Lowry KJ, Anglen JO. Indomethacin compared with localized irradiation for the prevention of heterotopic ossification following surgical treatment of acetabular fractures. J Bone Joint Surg Am (2001) 83(12):1783–8. doi: 10.2106/00004623-200112000-00003
30. Back DL, Smith JD, Dalziel RE, Young DA, Shimmin A. Incidence of heterotopic ossification after hip resurfacing. ANZ J Surg (2007) 77(8):642–7. doi: 10.1111/j.1445-2197.2007.04178.x
31. Popovic M, Agarwal A, Zhang L, Yip C, Kreder HJ, Nousiainen MT, et al. Radiotherapy for the prophylaxis of heterotopic ossification: a systematic review and meta-analysis of published data. Radiother Oncol (2014) 113(1):10–7. doi: 10.1016/j.radonc.2014.08.025
32. Ploumis A, Belbasis L, Ntzani E, Tsekeris P, Xenakis T. Radiotherapy for prevention of heterotopic ossification of the elbow: a systematic review of the literature. J Shoulder Elbow Surg (2013) 22(11):1580–8. doi: 10.1016/j.jse.2013.07.045
33. Lee A, Amin NP. “Radiation Therapy For Heterotopic Ossification Prophylaxis”. StatPearls (2020). Treasure Island (FL):StatPearls Publishing Copyright © 2020, StatPearls Publishing LLC.
34. Maender C, Sahajpal D, Wright TW. Treatment of heterotopic ossification of the elbow following burn injury: recommendations for surgical excision and perioperative prophylaxis using radiation therapy. J Shoulder Elbow Surg (2010) 19(8):1269–75. doi: 10.1016/j.jse.2010.05.029
35. Tsionos I, Leclercq C, Rochet JM. Heterotopic ossification of the elbow in patients with burns. Results after early excision. J Bone Joint Surg Br (2004) 86(3):396–403. doi: 10.1302/0301-620x.86b3.14480
36. Davis EL, Salisbury EA, Olmsted-Davis E, Davis AR. Anaplerotic Accumulation of Tricarboxylic Acid Cycle Intermediates as Well as Changes in Other Key Metabolites During Heterotopic Ossification. J Cell Biochem (2016) 117(4):1044–53. doi: 10.1002/jcb.25454
37. Zhang Q, Zhang Y, Yan M, Zhu K, Zhou D, Tan J. Bioinformatics Analysis of the Molecular Mechanism of Late-Stage Heterotopic Ossification. BioMed Res Int (2020) 2020:5097823. doi: 10.1155/2020/5097823
38. Abella JV, Galloni C, Pernier J, Barry DJ, Kjær S, Carlier MF, et al. Isoform diversity in the Arp2/3 complex determines actin filament dynamics. Nat Cell Biol (2016) 18(1):76–86. doi: 10.1038/ncb3286
39. Kong J, Shen S, Zhang Z, Wang W. Identification of hub genes and pathways in cholangiocarcinoma by coexpression analysis. Cancer Biomark (2020) 27(4):505–17. doi: 10.3233/cbm-190038
40. Jiang D, Wang Y, Liu M, Si Q, Wang T, Pei L, et al. A panel of autoantibodies against tumor-associated antigens in the early immunodiagnosis of lung cancer. Immunobiology (2020) 225(1):151848. doi: 10.1016/j.imbio.2019.09.007
41. Im BJ, Lee SC, Lee MH, Leesungbok R, Ahn SJ, Kang YG, et al. Promotion of osteoblastic differentiation and osteogenic transcription factor expression on a microgroove titanium surface with immobilized fibronectin or bone sialoprotein II. BioMed Mater (2016) 11(3):35020. doi: 10.1088/1748-6041/11/3/035020
42. Yang X, Matsuda K, Bialek P, Jacquot S, Masuoka HC, Schinke T, et al. ATF4 is a substrate of RSK2 and an essential regulator of osteoblast biology; implication for Coffin-Lowry Syndrome. Cell (2004) 117(3):387–98. doi: 10.1016/s0092-8674(04)00344-7
43. Boulay PL, Claing A. [ARF proteins: molecular switches controlling tumour proliferation and metastasis]. Med Sci (Paris) (2009) 25(10):783–5. doi: 10.1051/medsci/20092510783
44. Zhao Y, Ruan X. Identification of PGRMC1 as a Candidate Oncogene for Head and Neck Cancers and Its Involvement in Metabolic Activities. Front Bioeng Biotechnol (2019) 7:438. doi: 10.3389/fbioe.2019.00438
45. Ruan X, Cai G, Wei Y, Gu M, Zhang Y, Zhao Y, et al. Association of circulating Progesterone Receptor Membrane Component-1 (PGRMC1) with breast tumor characteristics and comparison with known tumor markers. Menopause (2020) 27(2):183–93. doi: 10.1097/gme.0000000000001436
46. Lin Y, Higashisaka K, Shintani T, Maki A, Hanamuro S, Haga Y, et al. Progesterone receptor membrane component 1 leads to erlotinib resistance, initiating crosstalk of Wnt/β-catenin and NF-κB pathways, in lung adenocarcinoma cells. Sci Rep (2020) 10(1):4748. doi: 10.1038/s41598-020-61727-3
47. Ponikwicka-Tyszko D, Chrusciel M, Stelmaszewska J, Bernaczyk P, Chrusciel P, Sztachelska M, et al. Molecular mechanisms underlying mifepristone’s agonistic action on ovarian cancer progression. EBioMedicine (2019) 47:170–83. doi: 10.1016/j.ebiom.2019.08.035
48. Feng L, Allen TK, Marinello WP, Murtha AP. Roles of Progesterone Receptor Membrane Component 1 in Oxidative Stress-Induced Aging in Chorion Cells. Reprod Sci (2019) 26(3):394–403. doi: 10.1177/1933719118776790
49. Anastassopoulou J, Kyriakidou M, Kyriazis S, Mavrogenis AF, Mamareli V, Mamarelis I, et al. Oxidative stress in ageing and disease development studied by FT-IR spectroscopy. Mech Ageing Dev (2018) 172:107–14. doi: 10.1016/j.mad.2017.11.009
50. Yang Z, Chen X, Zhang Q, Cai B, Chen K, Chen Z, et al. Dysregulated COL3A1 and RPL8, RPS16, and RPS23 in Disc Degeneration Revealed by Bioinformatics Methods. Spine (Phila Pa 1976) (2015) 40(13):E745–51. doi: 10.1097/brs.0000000000000939
51. Dong Z, Pei S, Zhao Y, Guo S, Wang Y. Identification of Hub Genes in Gastric Cancer with High Heterogeneity Based on Weighted Gene Co-Expression Network. Crit Rev Eukaryot Gene Expr (2020) 30(2):101–9. doi: 10.1615/CritRevEukaryotGeneExpr.2020028305
52. Prêle CM, Horton MA, Caterina P, Stenbeck G. Identification of the molecular mechanisms contributing to polarized trafficking in osteoblasts. Exp Cell Res (2003) 282(1):24–34. doi: 10.1006/excr.2002.5668
53. Li S, Lu X, Feng JB, Tian M, Wang J, Chen H, et al. Developing Gender-Specific Gene Expression Biodosimetry Using a Panel of Radiation-Responsive Genes for Determining Radiation Dose in Human Peripheral Blood. Radiat Res (2019) 192(4):399–409. doi: 10.1667/rr15355.1
54. Xiong X, Zhao Y, Tang F, Wei D, Thomas D, Wang X, et al. Ribosomal protein S27-like is a physiological regulator of p53 that suppresses genomic instability and tumorigenesis. Elife (2014) 3:e02236. doi: 10.7554/eLife.02236
55. He H, Sun Y. Ribosomal protein S27L is a direct p53 target that regulates apoptosis. Oncogene (2007) 26(19):2707–16. doi: 10.1038/sj.onc.1210073
56. Eid K, Labler L, Ertel W, Trentz O, Keel M. Systemic effects of severe trauma on the function and apoptosis of human skeletal cells. J Bone Joint Surg Br (2006) 88(10):1394–400. doi: 10.1302/0301-620x.88b10.17139
57. Pei L, Liu H, Ouyang S, Zhao C, Liu M, Wang T, et al. Discovering novel lung cancer associated antigens and the utilization of their autoantibodies in detection of lung cancer. Immunobiology (2020) 225(2):151891. doi: 10.1016/j.imbio.2019.11.026
58. Wu Q, Ren X, Zhang Y, Fu X, Li Y, Peng Y, et al. MiR-221-3p targets ARF4 and inhibits the proliferation and migration of epithelial ovarian cancer cells. Biochem Biophys Res Commun (2018) 497(4):1162–70. doi: 10.1016/j.bbrc.2017.01.002
59. Thejer BM, Adhikary PP, Kaur A, Teakel SL, Van Oosterum A, Seth I, et al. PGRMC1 phosphorylation affects cell shape, motility, glycolysis, mitochondrial form and function, and tumor growth. BMC Mol Cell Biol (2020) 21(1):24. doi: 10.1186/s12860-020-00256-3
60. Li JL, Chen C, Chen W, Zhao LF, Xu XK, Li Y, et al. Integrative genomic analyses identify WDR12 as a novel oncogene involved in glioblastoma. J Cell Physiol (2020) 235(10):7344–55. doi: 10.1002/jcp.29635
61. Desai K, Nair MG, Prabhu JS, Vinod A, Korlimarla A, Rajarajan S, et al. High expression of integrin β6 in association with the Rho-Rac pathway identifies a poor prognostic subgroup within HER2 amplified breast cancers. Cancer Med (2016) 5(8):2000–11. doi: 10.1002/cam4.756
62. Lim S, Cho HY, Kim DG, Roh Y, Son SY, Mushtaq AU, et al. Targeting the interaction of AIMP2-DX2 with HSP70 suppresses cancer development. Nat Chem Biol (2020) 16(1):31–41. doi: 10.1038/s41589-019-0415-2
63. Qiu BQ, Lin XH, Ye XD, Huang W, Pei X, Xiong D, et al. Long non-coding RNA PSMA3-AS1 promotes malignant phenotypes of esophageal cancer by modulating the miR-101/EZH2 axis as a ceRNA. Aging (Albany NY) (2020) 12(2):1843–56. doi: 10.18632/aging.102716
64. Suzuki J, Kanauchi N, Endo M, Hamada A, Watanabe H. [Pulmonary Adenocarcinoma with Heterotopic Ossification]. Kyobu Geka (2019) 72(5):363–6.
65. Kuribayashi H, Tsuta K, Mizutani E, Maeshima AM, Yoshida Y, Gemma A, et al. Clinicopathological analysis of primary lung carcinoma with heterotopic ossification. Lung Cancer (2009) 64(2):160–5. doi: 10.1016/j.lungcan.2008.08.007
66. Kypson AP, Morphew E, Jones R, Gottfried MR, Seigler HF. Heterotopic ossification in rectal cancer: Rare finding with a novel proposed mechanism. J Surg Oncol (2003) 82(2):132–6; disccussion 7. doi: 10.1002/jso.10181
Keywords: heterotopic ossification, biomarkers, WGCNA, pathological process, hub genes
Citation: Wang S, Tian J, Wang J, Liu S, Ke L, Shang C, Yang J and Wang L (2020) Identification of the Biomarkers and Pathological Process of Heterotopic Ossification: Weighted Gene Co-Expression Network Analysis. Front. Endocrinol. 11:581768. doi: 10.3389/fendo.2020.581768
Received: 14 August 2020; Accepted: 12 November 2020;
Published: 17 December 2020.
Edited by:
Giacomina Brunetti, University of Bari Aldo Moro, ItalyReviewed by:
Monica De Mattei, University of Ferrara, ItalyCarolina Figueroa, Maine Health, United States
Copyright © 2020 Wang, Tian, Wang, Liu, Ke, Shang, Yang and Wang. This is an open-access article distributed under the terms of the Creative Commons Attribution License (CC BY). The use, distribution or reproduction in other forums is permitted, provided the original author(s) and the copyright owner(s) are credited and that the original publication in this journal is cited, in accordance with accepted academic practice. No use, distribution or reproduction is permitted which does not comply with these terms.
*Correspondence: Jun Tian, dGlhbmp1bjE5NzMwNjA3QDE2My5jb20=