- 1ULB Center for Diabetes Research, Medical Faculty, Université Libre de Bruxelles (ULB), Brussels, Belgium
- 2Welbio, Medical Faculty, Université Libre de Bruxelles (ULB), Brussels, Belgium
- 3Indiana Biosciences Research Institute, Indianapolis, IN, United States
Type 1 diabetes (T1D) is a chronic disease caused by the selective destruction of the insulin-producing pancreatic beta cells by infiltrating immune cells. We presently evaluated the transcriptomic signature observed in beta cells in early T1D and compared it with the signatures observed following in vitro exposure of human islets to inflammatory or metabolic stresses, with the aim of identifying “footprints” of the immune assault in the target beta cells. We detected similarities between the beta cell signatures induced by cytokines present at different moments of the disease, i.e., interferon-α (early disease) and interleukin-1β plus interferon-γ (later stages) and the beta cells from T1D patients, identifying biological process and signaling pathways activated during early and late stages of the disease. Among the first responses triggered on beta cells was an enrichment in antiviral responses, pattern recognition receptors activation, protein modification and MHC class I antigen presentation. During putative later stages of insulitis the processes were dominated by T-cell recruitment and activation and attempts of beta cells to defend themselves through the activation of anti-inflammatory pathways (i.e., IL10, IL4/13) and immune check-point proteins (i.e., PDL1 and HLA-E). Finally, we mined the beta cell signature in islets from T1D patients using the Connectivity Map, a large database of chemical compounds/drugs, and identified interesting candidates to potentially revert the effects of insulitis on beta cells.
Introduction
Pancreatic beta cell stress and death are central components of type 1 diabetes (T1D) and also contribute in a decisive way to T2D (1, 2). Gertrude Stein once wrote in her poem “Sacred Emily” that a “rose is a rose is a rose is a rose.” But is “beta cell stress a beta cell stress a beta cell stress?” In other words, are all forms of beta cell stress the same and, more importantly, does it matter, as the outcome may be the same, namely relative or absolute reduction in insulin production?
Pancreatic beta cells are highly specialized endocrine cells that have as central tasks to sense circulating nutrients and respond to their circulating levels by releasing insulin in adequate amounts to assure their proper uptake and use by different organs; this maintains circulating levels of nutrients, such as glucose, inside narrow limits in spite of the wide variations in food intake observed in most mammalian species. Pancreatic beta cells in humans are very long lived, and our “beta cell patrimony” is probably established by early adulthood (3). These cells will thus need to cope with decades of varying insulin production – not a trivial task, considering that under stimulated conditions insulin synthesis increases 10-fold compared to basal level, approaching 50% of the total protein synthesis.
An important question is how beta cells react when exposed to mediators of autoimmune damage, such as pro-inflammatory cytokines released at the islet vicinity during the early [e.g., type 1 interferons (IFNs), such as IFNα] or late [e.g., interleukin-1β (IL1β) and interferon-γ (IFNγ)] stages of insulitis, or to saturated free fatty acids, such as palmitate, that contribute to metabolic stress in T2D (1, 2)? Available data suggest that beta cells trigger different adaptive responses that involve a decrease in its most differentiated functions, i.e., insulin synthesis and release, and the up-regulation of complex cellular responses, such as the unfolded protein response [UPR; (4)]. These adaptive responses are at least in part determined by the stress inducing them – for instance, beta cells exposed to pro-inflammatory cytokines trigger branches of the UPR that are different from the ones triggered in response to palmitate (2, 5, 6). These responses to diverse stresses will thus leave gene expression/molecular footprints that can be detected by omics techniques such as global RNA sequencing. Exam of these footprints may allow us to detect the nature of the beta cell stress causing them and, by comparing the molecular footprints induced by in vitro stresses with those present in beta cells isolated from patients affected by T1D, enable us to define the best experimental models to study the human disease. Furthermore, and of particular relevance for the discovery of novel therapies for T1D, comparisons of the different beta cells molecular footprints against large databases of cells exposed to different drugs, such as the recently updated Connectivity MAP database of cellular signatures, including > 1.3M profiles of human cells responses to chemical and genetic perturbations (7), can identify agents that antagonize particular gene signatures that may contribute to beta cell demise. Some of these agents, such as for instance the JAK inhibitor baricitinib, are already in use for other autoimmune diseases (8, 9) and can then be re-purposed for T1D therapy (10) (see below). We have recently published two comprehensive review articles focusing on beta cell fate in T1D (2, 11), and will focus here on the available studies characterizing the footprints left by immune or metabolic stresses on human beta cells.
In recent years RNA sequencing analysis has been done by us and others on human islets exposed to IL1β + IFNγ (12), IFNα (10) and palmitate (13) and of purified human beta cells or whole islets obtained from the pancreata of patients with T1D (14) or T2D (15); all these valuable datasets have been deposited on public access sites, such as the Gene Expression Omnibus repository (GEO). We have presently re-analyzed the most informative of these datasets, using the same pipeline [i.e., Salmon, GENCODE v31, DESeq2 (16–18)] to allow adequate comparisons between them, aiming to answer the following questions:
- How similar are the molecular footprints left on human islets by IL1β + IFNγ (12), IFNα (10) and palmitate (13)?
- Are these footprints representative of the patterns observed in beta cells obtained from patients affected by T1D?
- Can we obtain relevant indications for new therapies by mining these molecular footprints against available drug-induced footprints in other cell types?
Methods
For the present review and analysis we have selected available RNA-seq datasets of pancreatic human islets or FACS-purified human beta cells exposed to different pro-inflammatory stimuli (10, 12), metabolic stressors (13) or to the local environment present during T1D development (insulitis) (14) that are publicly available from the GEO repository (www.ncbi.nlm.nih.gov/geo). For the search we have used the following terms combinations: (1) “pancreatic endocrine cells” [All Fields] OR “pancreatic beta cells” [All Fields] OR “human islets” [All Fields] AND “type 1 diabetes” [All Fields] AND (“Homo sapiens” [Organism] AND “Expression profiling by high throughput sequencing”[Filter]); (2) “pancreatic endocrine cells” [All Fields] OR “pancreatic beta cells” [All Fields] OR “human islets” [All Fields] AND “cytokines” [All Fields] AND (“Homo sapiens” [Organism] AND “Expression profiling by high throughput sequencing” [Filter]); (3) “pancreatic endocrine cells” [All Fields] OR “pancreatic beta cells” [All Fields] OR “human islets” [All Fields] AND “palmitate” [All Fields] AND (“Homo sapiens” [Organism] AND “Expression profiling by high throughput sequencing” [Filter]). We also searched the Pubmed using the same criteria and mined online sources for unpublished data. Since the present analysis focus on beta cell transcript (isoforms) expression, we excluded articles having insufficient reads coverage (<20 million reads per sample, n = 3) and depleted of beta cells (<500 transcripts per million (TPM) of insulin, n = 1). The PRISM flow diagram (19) describing the search strategies is represented in Figure 1. Table 1 provides a detailed description of each dataset including their GEO reference number.
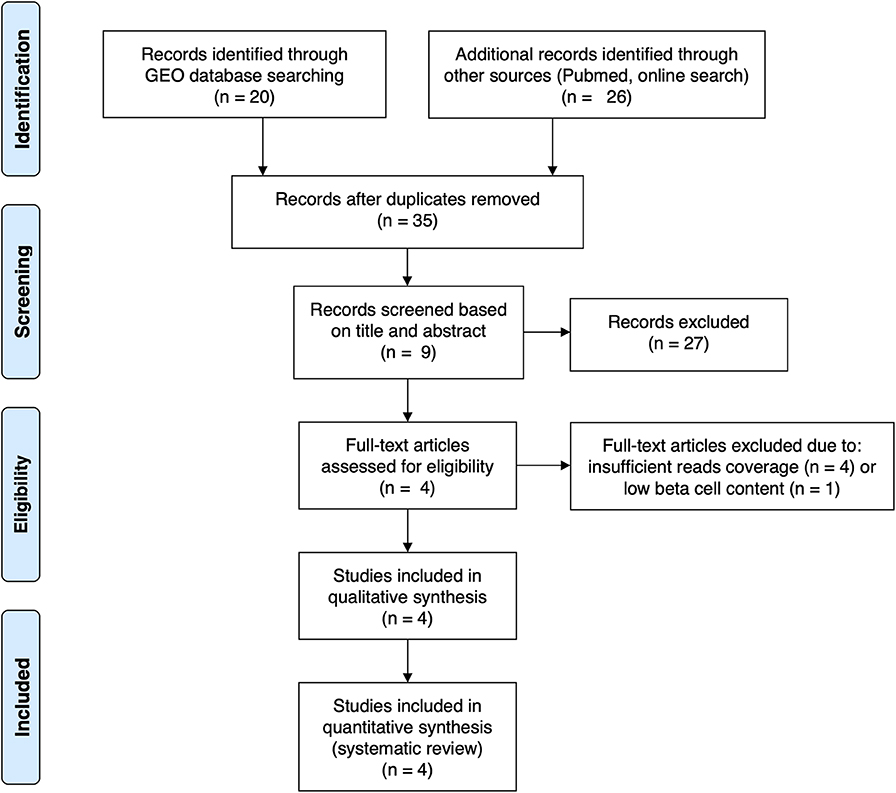
Figure 1. PRISMA flow diagram (19) describing the search strategy used to identify the analyzed studies.
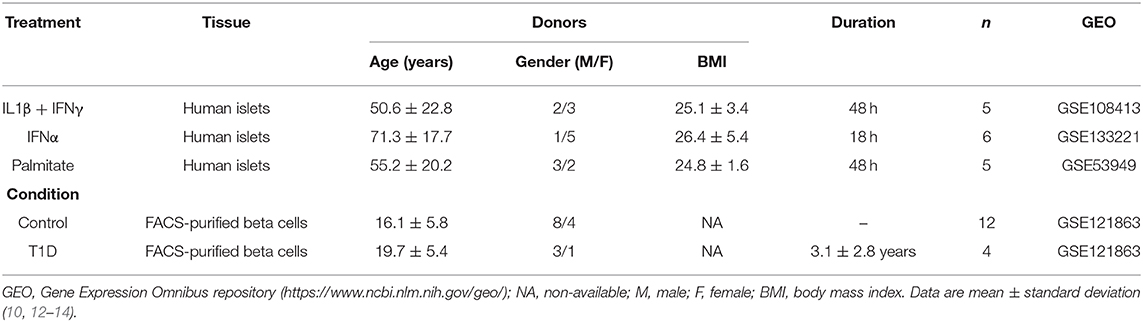
Table 1. Description of the datasets of pancreatic human islets and purified beta cells exposed to different stimuli and conditions presently evaluated.
After downloading the raw data, we used Salmon version 0.13.2 (16) to map the reads to the human reference transcriptome [GENCODE version 31 (GRCh38) (17)] using the quasi-alignment model. The transcript abundance is represented in Transcripts Per Million (TPM).
Differential analysis was performed using the R package DESeq2 version 1.24.0 (18). The estimated number of reads obtained from Salmon were used as input for DESeq2. Briefly, DESeq2 normalizes samples according to per-sample sequencing depth and accounting for intra-sample variability. Then, it fits data to a negative binomial generalized linear model (GLM) and calculates the Wald statistic. Finally, the raw p-values are corrected using the false discovery rate (FDR) for multiple testing by the Benjamini-Hochberg method. Transcripts with an FDR <0.05 were considered differentially modified.
To compare the different signatures present in each dataset, we performed Rank-Rank Hypergeometric Overlap (RRHO) mapping (20). For this goal, we have generated lists of transcripts ranked by their -log10 p-values from the differential expression analysis. In a RRHO map the hypergeometric p-value for enrichment of k overlapping genes is calculated for all possible threshold pairs for each condition, generating a matrix where the indices are the current rank in each condition. The log-transformed hypergeometric p-values are then plotted in a heatmap indicating the degree of statistically significant overlap between the two ranked lists on that position of the map. We have applied adjustment for multiple comparison using the Benjamini-Yekutieli correction.
To evaluate the similarities between datasets we used the R package FactoExtra version 1.0.6 (https://github.com/kassambara/factoextra) considering as (dis)similarity (distance) measure the Pearson correlation between samples (1 – correlation) using the 300 most variable transcripts (i.e., median absolute deviation). Next, hierarchical clustering was performed based on the average of the pairwise (dis)similarities (distances) between samples.
For functional enrichment analysis the R/ Bioconductor package ClusterProfiler version 3.12 (21) was used in combination with gene sets from the Molecular Signatures Database (22). All the transcripts presenting a TPM > 1 in at least half of the samples were considered as background and a Benjamini–Hochberg FDR threshold of 0.05 was defined as significant enrichment.
The top 150 up-regulated transcripts in the RNA-seq of FACS-purified beta cells from T1D individuals (14) were identified from the differential expression analysis. This list of transcripts was used to query the Connectivity Map dataset of L1000 cellular signatures, which has transcriptional responses of human cells to different chemical and genetic perturbations, using the CLUE platform (https://clue.io) (7). To identify compounds potentially reverting the effects induced by insulitis on beta cells we focused on perturbagens promoting signatures that were opposite (negative tau score) to our query list. Only perturbagens having a median tau score < −60 were considered for further evaluation.
Results
The Footprints of in vitro Cytokine, but Not Palmitate, Exposure Are Similar to the Ones Observed in Beta Cells From T1D Patients
In order to obtain in vitro inflammatory and metabolic footprints of beta cells, we analyzed previously generated RNA-sequencing of pancreatic human islets exposed to pro-inflammatory cytokines [IL1β + IFNγ (12) or IFNα (10)] or to a metabolic stressor [palmitate (13)] (Figure 1). Furthermore, recent advances in techniques to purify beta cells and the establishment of collaborative networks between different research groups have allowed for the first time the generation of RNA-sequencing data of human beta cells from T1D individuals (14). This database offers a unique opportunity to validate the in vitro models by comparing them against the in vivo situation present during T1D development. For this purpose, we first evaluated the similarities between the signatures of transcripts induced by the different stimuli (inflammatory and metabolic) in pancreatic islets and by the local environment of insulitis that beta cells are exposed to in T1D (Figure 2). The analysis was performed at the transcript (isoform) level, since we have previously observed that exposure of beta cell to pro-inflammatory cytokines promotes major changes in alternative splicing (AS), leading to a high number of different splicing events (10, 23–25). This is particularly relevant since AS is a cell-type- and context-dependent mechanism. In line with this, several RNA-binding proteins that regulate gene splicing are significantly modified in beta cells isolated from individuals affected by T1D (Supplementary Table 1). This analysis indicated that the pro-inflammatory cytokines trigger a profile of transcripts that generate clusters of similar samples (positive Pearson correlation), while the metabolic stressor palmitate generates different groups of transcripts that cluster separately and with an opposite profile as compared to cytokines (negative Pearson correlation) (Figure 2). Interestingly, the samples obtained from T1D individuals (indicated by red color) clustered together with the two in vitro models of pancreatic islet inflammation; this similarity was slightly higher (represented by darker red color boxes) with the signature of the pro-inflammatory cytokines IL1β + IFNγ (orange) than with IFNα (yellow) (Figure 2). This is probably due to the fact that the beta cell samples were obtained from four patients 5 months, 2, 3, and 7 years after diagnosis of T1D, a period when a full adaptive immune response against the beta cells is in place, including exposure of islets to the cytokines IL1β + IFNγ, while IFNα may play a more relevant role during the early and more “innate-immunity related” phases of the disease (26).
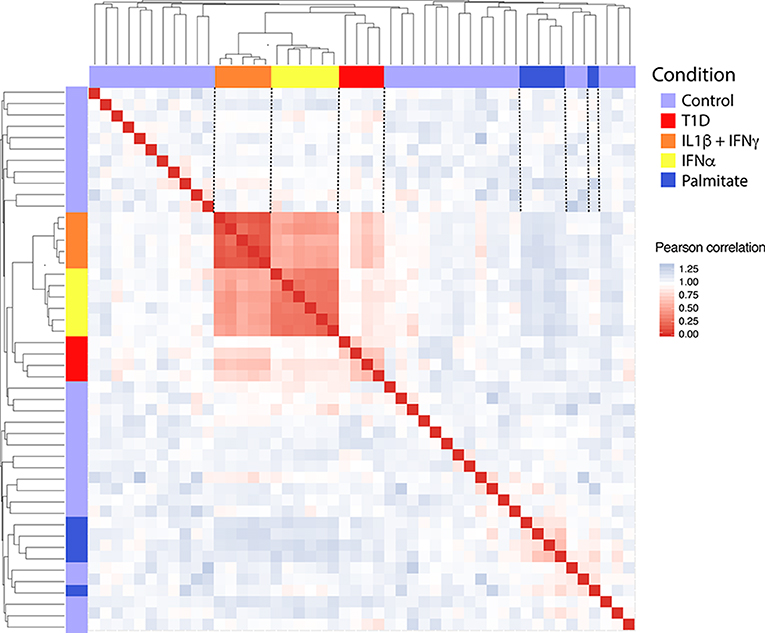
Figure 2. Correlation between the transcripts expressed in human islets exposed to IL1β + IFNγ, IFNα or palmitate and the transcripts expressed in human beta cells of individuals affected by T1D. Data were obtained by RNA sequencing (10, 12–14). The Pearson correlation (1 – correlation) was used to evaluate the (dis)similarities (distance) among the 300 most variable transcripts in the RNA-seq datasets. Red squares represent a positive correlation (similarity), blue squares a negative correlation (dissimilarity) and white squares an absence of correlation between each pair of observations. Next, the hierarchical clustering was performed considering the average of the dissimilarity (distance) between samples. The resulting dendrogram is shown in the upper and lateral part of the matrix.
Next, to identify the transcripts that are analogously modulated in beta cells from individuals affected by T1D and in pancreatic islets exposed to the different stressful stimuli, we performed a Rank-Rank hypergeometric overlap (RRHO) analysis (20) which evaluates the (dis)similarities between two ranked lists. For this comparison, ranked lists of transcripts based on the -log10 p-values from the differential expression analysis (T1D or stimuli vs. controls) were generated. The RRHO mapping demonstrated a significant intersection of similarly up-regulated transcripts in T1D beta cells and in human islets exposed to both IFNα (Figure 3A) and IL1β + IFNγ (Figure 3B). In agreement with the distance matrix findings (Figure 2), the significance of this intersection was more pronounced for the late cytokines, i.e., IL1β + IFNγ (Figure 3B), particularly related to down-regulated genes. This concordance in down-regulated genes may be due to the fact that IL1β + IFNγ but not IFNα, trigger a more severe beta cell stress, eventually leading to apoptosis (10, 23, 27). On the other hand, there was no statistically significant correlation between the T1D beta cells signature and the one induced by the metabolic stressor palmitate in human islets (Figure 3C). In line with this, we have previously shown that there is no clear correlation between human islets exposed to IFNα (10) or to IL1β + IFNγ (2) and human islets obtained from patients affected by type 2 diabetes.
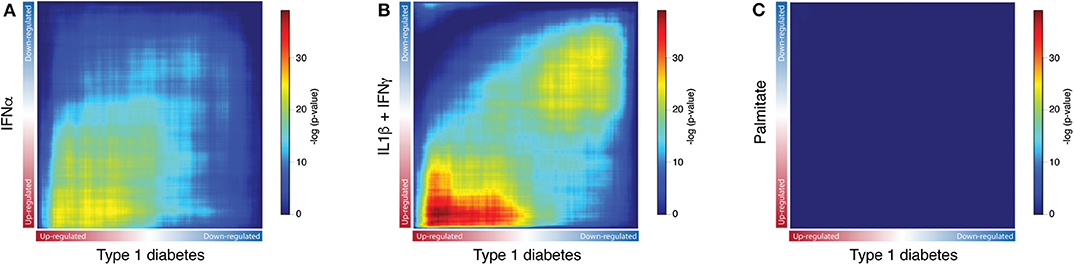
Figure 3. Exposure of human islets to pro-inflammatory cytokines, but not to palmitate, induce a similar transcriptomic profile as observed in islets isolated from patients affected by type 1 diabetes. (A–C) Rank-Rank Hypergeometric Overlap (RRHO) map comparing the transcriptional expression profile of human islets exposed to IFNα (A), IL1β + IFNγ (B) or palmitate (C) to the one present in primary beta cells from individuals affected by T1D, as identified by RNA-seq. Ranked lists of transcripts based on the -log10 p-values from the differential expression analysis of human islets exposed to IFNα (A), IL1β + IFNγ (B) or palmitate (C) were compared to a similarly ranked-list from beta cells obtained from patients with T1D.
To gain further insight into the biological processes and pathways triggered at the different stages of T1D development, we next performed enrichment analysis of the up-regulated transcripts present in the areas of significant intersection between the RNA-seq datasets from islets obtained from patients affected by T1D as compared to cytokine-treated human islets (Figure 4). Figure 4A outlines the three intersection areas evaluated, while Figure 4B shows transcripts up-regulated only in the intersection between the INFα and T1D datasets, reflecting most likely the early changes induced in beta cells during the evolution of diabetes. Figure 4C shows transcripts up-regulated only in the intersection between the IL1β + INFγ and T1D datasets, probably mirroring the changes present at later stage of T1D progression. Finally, Figure 4D shows transcripts up-regulated in the intersection between all datasets (INFα, IL1β + IFNγ, and T1D), which may represent alterations common to different phases of the disease. These putative early and late changes observed in the islets in the course of T1D are discussed in more detail below.
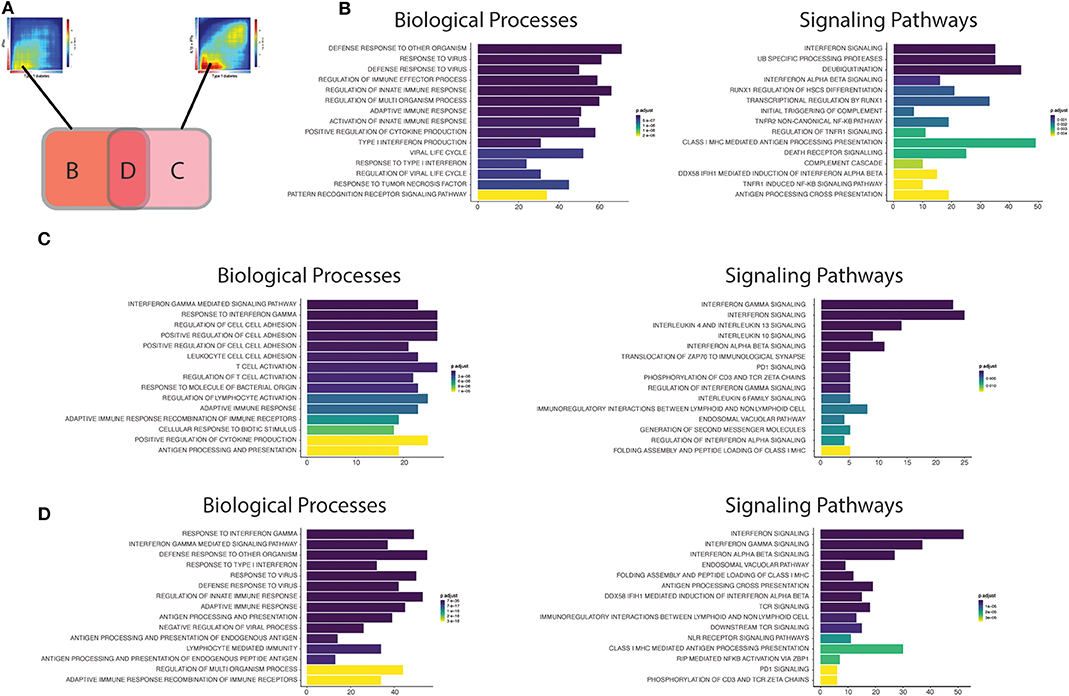
Figure 4. Functional analysis of the transcripts overlapping beta cell datasets from T1D patients' islets or cytokine-exposed human islets. (A) First, the up-regulated transcripts present in the intersection of RRHO maps comparing the T1D signature in beta cells against the IFNα (left) and IL1β + IFNγ (right) signatures in human islets were identified. Next, these transcripts were divided in three different groups: (B) transcripts only present in the intersection between T1D vs. IFNα, (C) transcripts only present in the intersection between T1D vs IL1β + IFNγ, (D) transcripts present in the intersection of all the three datasets (T1D, IFNα, IL1β + IFNγ). (B–D) Biological processes (Gene Ontology) and signaling pathways (Reactome) enriched among the overlapping regions of the RRHO maps described above [(B) only T1D vs IFNα; (C) only T1D vs. IL1β + IFNγ; (D) T1D, IFNα and IL1β + IFNγ]. The y axis shows the 15 most significant genesets, while the x axis represents the total number of transcripts identified in each geneset.
Early Pancreatic Islets Changes
The biological processes (Gene Ontology) regulated by the transcripts present in area B (Figure 4B; only T1D vs. IFNα) recapitulate well the initial events putatively present during T1D development (26). In brief, innate immune responses are probably triggered after activation of pattern recognition receptors (PRRs) (including Toll-like receptors (TLRs), and RIG-I-like receptors) by endogenous “danger signals” or exogenous ligands, such as nucleic acids (e.g., double stranded RNA) produced during viral infections, which are putative environmental factors associated with T1D (28). The activation of PRRs on beta cells promotes an increased local production of antiviral type I interferons, such as IFNα, by the beta cells themselves and by other cells present in the islets, and the recruitment of immune cells to the pancreatic islets. In support to this model, rare variants in the RIG-I-like receptor MDA5 that lead to decreased function are associated with protection against T1D (29). In contrast, a genetic variant of MDA5 that leads to a partial gain-of-function significantly increases the risk for T1D (30). In both cases, the impact on T1D development is dependent on, respectively, a decreased or an exacerbated production of type I IFNs. These locally produced cytokines activate several signaling pathways that include key pathways observed in early human T1D, such as MHC class I antigen presentation (31) and beta cell death (26) (Figure 4B). We have recently demonstrated that IFNα up-regulates different mechanisms involved in post-transcriptional regulation of gene expression, especially alternative splicing (AS) (10) and endoplasmic reticulum (ER) stress (10, 32), which can potentially generate beta cells neoantigens. This, combined with the overexpression of HLA class I, may facilitate auto-immunity progression by activation of autoreactive T-cells that may have escaped thymic selection (11). Of note, a similar phenomenon has been observed for the pro-inflammatory cytokines IL1β + IFNγ which increase the expression of an isoform of secretogranin V, SCG55-009, that is recognized by auto-reactive CD8+ T-cells present in the pancreas of T1D individuals (12). It has also been described that beta cells undergoing endoplasmic reticulum stress can modify the insulin translation initiation site, producing a highly immunogenic polypeptide capable of activating T-cells from T1D individuals with the potential for killing human beta cells (33).
Macrophages are among the main immune cell populations present in the human pancreas (34), and their density is increased in the vicinity of the pancreatic islets (<20 μM) of individuals with recent-onset T1D in comparison with non-diabetic individuals (34). This is compatible with findings from different animal models showing that macrophages are among the first activated immune cells present in the pancreas in autoimmune diabetes (35, 36) and the main cell type responsible for the TNF production in pancreatic islets (37). Furthermore, we identified “activation of TNF receptor subunits” and its main downstream transcription factor nuclear factor-κB (NF- κB) as important pathways activated in early human T1D (Figure 4B). This is particularly relevant, since differently from other cell types in which NF-κB has mainly a pro-survival role (38), NF-κB activation in beta cells is mostly pro-apoptotic (39).
Finally, pathways involved in the regulation of protein ubiquitination were also overrepresented in our analysis of common effects observed in early T1D and following human islet exposure to IFNα (Figure 4B). The signal transduction downstream of the PRRs relies on their association with specific adaptors, which in many cases require ubiquitin-specific modifications to became active (40). Ubiquitination is counteracted by deubiquitinases, a group of enzymes that remove these modifications and thus provide a negative feedback on the signaling cascade (41). Interestingly, single-nucleotide polymorphisms (SNPs) that decrease activity of the ubiquitin-modifying enzyme TNFAIP3 (A20) (A20 provides a negative feedback on NF-κB activation by stimulating degradation of some of its components) are associated with a higher risk for development of T1D and other autoimmune diseases (42). Moreover, rare mutations leading to TNFAIP3 loss-of-function cause a systemic autoinflammatory disease (43). The affected individuals present a type I interferon (IFN) signature which correlates with the disease activity and predicts their response to treatment with janus kinase (JAK) inhibitors (JAK1 is a key kinase for type I interferon signaling) (44). In agreement with this, we have shown that the JAK 1 and 2 inhibitors ruxolitinib (45) and baricitinib (10) prevent IFNα-induced MHC class I and chemokine up-regulation in human islets, besides inhibiting IFNα + IL1β-induced beta cell apoptosis; another drug from this family was shown to prevent diabetes in NOD mice (46). Baricitinib is already in clinical use for other autoimmune diseases (8, 9) and may be eventually re-purposed for the early therapy of T1D. This demonstrates the utility of beta cell signature characterizations for the identification of new therapeutic targets in T1D (see below).
Advanced Pancreatic Islets Changes
In the case the local pro-inflammatory environment described above is maintained, the increased homing of different immune cells to the pancreatic islets promotes the transition to a scenario dominated by adaptive immune responses. The intersection area C may be representative of the findings observed during these late stages of insulitis (Figure 4C). The biological processes (GO) are now enriched in IFNγ responses, reflecting the increased number of T-cells present in the islets. A critical step for the immune cells to reach the inflamed tissue is their adhesion and crawling on the endothelium (47). In line with this, several processes (GO) involved in cell adhesion are induced in pancreatic islets from T1D individuals (Figure 4C). During this process, activated T-cells expressing high-affinity integrins bind to the endothelial cells via cellular adhesion molecules (CAMs) (48). Deficiency of the vascular adhesion molecule adhesion intercellular adhesion molecule 1 (ICAM1) (49) or its receptor (50), lymphocyte function-associated antigen 1 (LFA1), prevents the development/progression of autoimmune diabetes in NOD mice. Of interest, a genetic risk variant associated to T1D (rs657152) (51) is also associated with the circulating levels of soluble ICAM1 (52).
Other potentially important pathways identified by the present analysis were the “Immunoregulatory interactions between lymphoid and non-lymphoid cells” and “PD1 signaling” (Figure 4C). We have recently shown that during insulitis, in addition to pro-inflammatory stimuli, beta cells also express immune checkpoint proteins, including programmed death-ligand 1 (PDL1) and HLA-E, possibly in an attempt to down-regulate the immune responses and thus avoid further tissue damage (10, 53). In line with these observations, individuals receiving immunotherapy based on PDL1/PD1 blockers for cancer treatment have a higher risk of developing T1D (54) and other autoimmune diseases (55). The induction of these checkpoint proteins in human beta cells is mainly mediated by type I and II IFNs under the control of the transcription factor interferon regulatory factor 1 (IRF1) (53). The protective role for these co-inhibitory molecules in beta cells is reinforced by the facts that beta cells surviving the immune assault in NOD mice express high levels of PDL1 (56), that transgenic PDL1 overexpression in beta cells decreases diabetes prevalence in NOD mice (57), and that that both PDL1 (53) and HLA-E (10) are absent in pancreatic islets from T1D individuals depleted of insulin.
Another group of immunomodulatory molecules presenting activation of their signaling pathways were anti-inflammatory cytokines, including interleukin-10 (IL10) and interleukin 4/13 (IL4/13) (Figure 4D). In line with this, systemic delivery of IL10 via adenovirus-associated gene therapy prevented diabetes recurrence after syngeneic islets transplantation in NOD mice (58). In the same animal model, oral administration of a probiotic (Lactococcus lactis) expressing IL10 and the autoantigen GAD65, in combination with low dose of anti-CD3, reversed autoimmune diabetes (59). The second class of cytokines include IL4 and IL13, which exerts their actions through three different combinations of shared receptors (60). These cytokines can trigger phenotypes that range from allergy, including asthma, to anti-helminthic responses. Interestingly, helminthic infections are associated with protection against immune-mediated diseases, such as T1D (61). The systemic administration of IL4 (62) or IL13 (63) was shown to prevent the development of diabetes in NOD mice. This effect is at least in part mediated via their direct action on beta cells, since beta cells express all the required IL4/13 receptor subunits (64) and their in vitro exposure to IL4 or IL13 protects them against pro-inflammatory cytokine-induced apoptosis (64, 65). This cytoprotection is associated with the activation of signal transducer and activator of transcription 6 (STAT6) in beta cells, leading to the up-regulation of anti-apoptotic proteins such as myeloid leukemia-1 (MCL-1) and B cell lymphoma-extra large (BCL-XL) (65).
Finally, analysis of the biological processes and the signaling pathways controlled by genes commonly up-regulated in all the three datasets (IFNα, IL1β + IFNγ and T1D) (Figure 4D) indicate that they summarize many findings present at the different stages of the disease, including regulation of antiviral responses, responses to type I (IFNα/β) and II (IFNγ) interferons, MHC class I antigen presentation, lymphocyte activation, interaction between lymphoid and non-lymphoid cells and PD1 signaling. This suggest that signatures of both innate and adaptive immunity remain present in the islets as the disease evolves, which is supported by histological and RNA-seq findings in whole islets from T1D patients (66, 67). Analysis of transcripts that are only modified in beta cells of T1D individuals, but not after exposure of these cells to cytokines (Supplementary Figure 1A), indicates pathways that are less dependent on these inflammatory mediators (Supplementary Figure 1B). Changes in genes involved in digestion and absorption were upregulated in samples from T1D only, and among these genes we identified CTRB1 and CTRB2. Polymorphisms in these genes have been associated to higher risk for T1D development (68, 69), and mild hyperglycemia induces their upregulation and that of other genes identified in the same pathway (70). There was also activation of matrix metalloproteinases, which is a potentially relevant mechanism in T1D since matrix metalloproteinases can cleave membrane-bound PDL1 present on the cell surface and thus regulate T-cell responses (71).
Mining Beta Cell Molecular Footprints for Drug Re-purposing in T1D
Up to now there is no treatment available to prevent the development of T1D in individuals at risk. This, and the worldwide increase in T1D incidence observed in recent decades (72, 73), makes T1D a major area of interest for drug discovery. We have presently mined the RNA-sequencing of FACS-purified beta of T1D individuals (14) using the recently updated version of the Connectivity Map (CMap) database (7). To avoid potential off-target findings caused by focusing on individual compounds in the analysis, we focused instead on classes of drugs that promote an opposite signature to the one present in beta cells of T1D individuals. We identified several classes of drugs/compounds that could potentially revert the inflammatory signatures present in beta cells during T1D, including bile acids, bromodomain inhibitors, leucine-rich repeat kinase (LRRK) inhibitors and vitamin D receptor agonists (Figure 5).
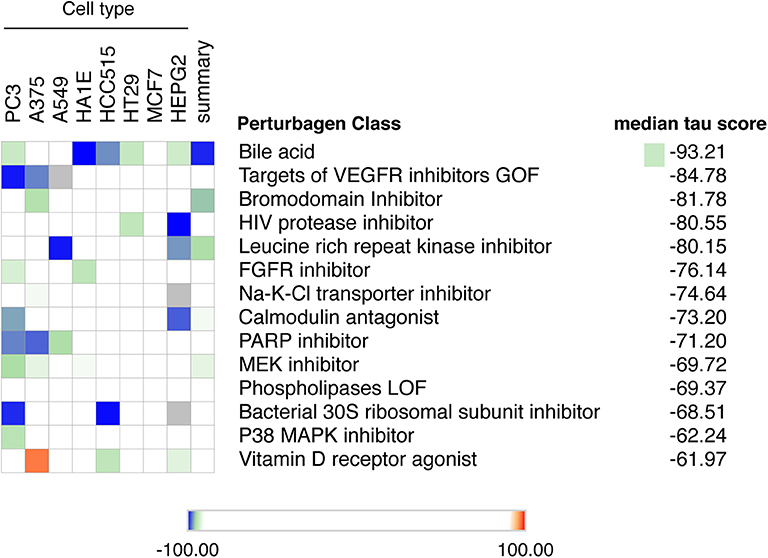
Figure 5. Mining the beta cell signature in T1D to identify potential new therapeutic targets. The top 150 up-regulated transcripts identified in the RNA-seq of beta cells of T1D individuals (14) were used to query the Connectivity Map database of cellular signatures (7). The top Connectivity Map classes of perturbagens that promote an opposite signature (negative tau scores) to the one present in beta cells of T1D individuals are represented.
Among the top classes of compounds identified were bile acids. This is an interesting finding, since TUDCA - a conjugated bile acid with chaperone properties - has been shown to prevent autoimmune diabetes in NOD mice (74). This protection was mediated via the restoration of a defective unfolded protein response (UPR) observed in beta cells during insulitis. In vitro studies demonstrated that TUDCA also inhibits IFNα-induced ER stress and its subsequent UPR activation in human beta cells (75). Finally, there is an ongoing phase 2 clinical trial evaluating the potential translational impact of these findings in individuals with recent-onset T1D (ClinicalTrials.gov Identifier: NCT02218619).
Another class of compounds identified were bromodomain inhibitors. The bromodomain (BRD) proteins are “readers” of histone acetylation that, associated with other components of chromatin-remodeling complexes, promote transcriptional activation. Bromodomain and extra-terminal domain (BET) proteins are the most studied members of the BRD family. Inhibitors of BET proteins have protective effects in different animal models of autoimmunity (76, 77), including in the NOD mouse model of autoimmune diabetes (78). We have recently demonstrated that two bromodomain inhibitors, namely JQ1 and I-BET-151, partially prevent the deleterious effects of IFNα on human beta cells (10).
We also identified leucine-rich repeat kinase inhibitors (LRRK) as a potential perturbagen in T1D (Figure 5). The currently available LRRK inhibitors mainly target LRRK2. The LRRK2 protein has two domains with catalytic activity; a GTPase domain of the Ras of complex (ROC) protein family, and a kinase domain of the tyrosine kinase like (TKL) family (79). Genome-wide association studies have linked kinase-activating mutations in LRRK2 with an increased risk for both Parkinson disease (80) and inflammatory bowel diseases (IBD) (81). In vivo and in vitro studies indicate that these LRRK2 risk variants act mainly by promoting exacerbated responses to pro-inflammatory stimuli (82–84). In line with this, LRRK2 inhibition prevented microglial inflammatory responses triggered by TLR activation (85). Of concern, systemic LRRK2 knockout (KO) prevented the phosphorylation of Rab10, a crucial step for insulin-mediated glucose transporter type 4 (GLUT4) translocation to cell surface (86). GLUT4 is a key glucose transporter in peripheral tissues, and this inhibitory impact on its cellular localization may lead to insulin resistance. This highlights the importance of reviewing the whole body of evidence before selecting the best potential therapeutic targets.
Several studies have demonstrated the role of vitamin D and its analogs as anti-inflammatory and immunomodulatory agents. In line with this, exposure of human pancreatic islets to the active form of vitamin D, calcitriol, increases expression of the protective candidate gene TNFAIP3 and reduces pro-inflammatory cytokine (IL1β + TNF + IFNγ)-induced MHC class I expression, IL6 production and nitric oxide synthesis (87, 88). In vivo studies on NOD mice support these findings, with a decrease in insulitis and diabetes prevalence observed when calcitriol was administrated early in life (89). Furthermore, two large birth cohorts of genetically at risk individuals demonstrated that lower levels of 25(OH)-vitamin D in early infancy are associated with a higher incidence of islet autoimmunity (90, 91) and T1D development (90). This effect was modified by polymorphisms in the vitamin D receptor (VDR) (91). However, data from clinical trials using vitamin D and its analogies in humans have provided conflicting results. This may be caused by different factors: (1) the genetic background of the affected individuals (such as genetic variants in the candidate gene TNFAIP3 and polymorphisms in the VDR) may modify their responses to vitamin D; (2) insufficient doses of vitamin D to modulate the immune system are often used, due to concerns regarding hypercalcemia; (3) too late introduction of the treatment; (4) the need to use vitamin D analogs in combination with other agents to address the complexity of T1D pathogenesis.
Discussion
Despite the continued work performed by the research community there is still no treatment capable of preventing T1D development in individuals at risk or reverting disease after its outbreak. At the very best, use of anti-CD3, a class of drugs targeting exclusively the immune system, delayed by around 2 years the progression to T1D in individuals at high-risk for the disease (92). A similarly transient benefit on beta cell function was observed when treating patients with clinical T1D using anti-CD3 (93) or anti-CD20 (94).
In order to better understand and treat T1D, we may need to move on from an immune cell-centered view of the disease to a scenario that considers the disease as the product of a dynamic interaction between the killing immune cells and the target beta cells (2, 11, 26). To add information on the impact of insulitis on the target beta cells, we have presently characterized the human beta cell responses during T1D and after exposure to different immune-mediated stimuli. We observed that the in vivo beta cell responses can be closely recapitulated using an in vitro system biology approach that combines exposure to cytokines putatively present at different stages of the disease and high coverage RNA-sequencing. This may be further improved by the parallel evaluation of cytokine-induced changes in human beta cells protein expression (10, 95, 96) and chromatin status (10, 95). The validation of these models is an important finding, as the access to high quality beta cells from patients affected by T1D for multi-omics analysis is extremely difficult. Finally, the present integrated analysis identified important biological processes and signaling pathways activated during T1D progression, leading to the identification of potentially new therapeutic targets to be considered for future clinical trials.
Recent studies have suggested a role for aging and senescence-associated secretory profile (SASP) in beta cell disfunction and death in experimental models for both T1D and T2D (97, 98). We have searched for eventual changes in SASP-related genes (i.e., ARNTL, CDKN1A, ICAM1, ID2, LIMS1, MAP2K1, MAPK14, MIF, PRKCD, SERPINE1, TBX2, ULK3, ZMIZ1 etc.) in the present RNA-seq of beta cells from T1D individuals, but did not observe significant changes in any of these transcripts as compared to non-diabetic donors (data not shown).
Weakness of the present study, which may hamper extrapolations to most T1D patients, include the limited number of samples analyzed (there were only 4 preparations from T1D patients available in the literature that reached the proposed criteria (see Methods) for inclusion in our analysis) and the disparate age of islet/beta cell donors when comparing for instance T1D patients (mean age 19.7 years) and IFNα-treated islets (mean age 71.3 years) (Table 1). It is remarkable that, in spite of these limitations, there remained major and consistent similarities between the gene expression observed in beta cells from different patients affected by T1D and the gene expression present in human islets exposed to pro-inflammatory cytokines.
To further expand the present information and better define the role of each cell type present in the islets of Langerhans or in its vicinity during insulitis, single cells analysis will be required (99); up to now, however, this has been technically challenging due to the very limited number of beta cells retrieved from diabetic individuals (100). This approach has been already performed in another autoimmune disease, systemic lupus erythematosus, in which single cell analysis of lupus nephritis identified cell specific signatures that led to the recognition of key signaling pathways suitable for specific therapeutic targeting (101).
The recent technical and scientific advances allowing the generation of beta cells derived from inducible pluripotent stem cells (iPSC) that show similar responses to pro-inflammatory cytokines as adult human beta cells (102), opens the possibility to differentiate beta cells from individuals with specific genetic variants that modulate the different stages of insulitis (2). Notably, these iPSC-derived cells could also be used to generate organoids simulating the pancreas environment, which would allow co-culture with relevant immune cells to better define beta cell-immune system crosstalk in the context of specific genetic backgrounds, and then to use this system for drug screening.
T1D is a complex disease, and a sustained therapeutic response will only be achieved by combining compounds that contribute to “re-educate” the immune system and to protect/regenerate beta cells. An interesting approach to be pursued at the beta cell level would be to down-regulate HLA class I antigen presentation while increasing signaling of the immune check-point proteins (PDL1 and HLA-E); this may be feasible, as suggested by the observation that inhibiting the transcription factor STAT2 decreases HLA class I expression while preserving/increasing PDL1 expression (53). Importantly, these interventions should be safe and started as early as possible to prevent irreversible loss of functional beta cell mass.
Data Availability Statement
Publicly available datasets were analyzed in this study. This data can be found at: Gene Expression Omnibus (GEO) repository (https://www.ncbi.nlm.nih.gov/geo/) under the accession numbers: GSE108413, GSE133221, GSE53949, GSE121863, GSE121863.
Author Contributions
MC and DE conceived, designed, supervised the study, wrote the manuscript, and all authors revised it. MC retrieved data, performed analysis, as such have full access to all the data in the study, take responsibility for the integrity of the data, and the accuracy of the data analysis. MC and FS performed bioinformatic analyses. All authors contributed to the article and approved the submitted version.
Funding
This work was supported by grants from the Fonds National de la Recherche Scientifique (FNRS), Welbio CR-2015A-06 and CR-2019C-04, Belgium; and Innovate2CureType1 - Dutch Diabetes Research Fundation (DDRF). DE have also received funding from the Innovative Medicines Initiative 2 Joint Undertaking under grant agreements No. 115797 (INNODIA) and No. 945268 (INNODIA HARVEST). These Joint Undertakings receive support from the European Union's Horizon 2020 research and innovation programme and EFPIA, JDRF, and The Leona M. and Harry B. Helmsley Charitable Trust.
Conflict of Interest
The authors declare that the research was conducted in the absence of any commercial or financial relationships that could be construed as a potential conflict of interest.
Supplementary Material
The Supplementary Material for this article can be found online at: https://www.frontiersin.org/articles/10.3389/fendo.2020.568446/full#supplementary-material
References
1. Cnop M, Welsh N, Jonas JC, Jorns A, Lenzen S, Eizirik DL. Mechanisms of pancreatic β-cell death in type 1 and type 2 diabetes: many differences, few similarities. Diabetes. (2005) 54(Suppl. 2):S97–107. doi: 10.2337/diabetes.54.suppl_2.S97
2. Eizirik DL, Pasquali L, Cnop M. Pancreatic β-cells in type 1 and type 2 diabetes mellitus: different pathways to failure. Nat Rev Endocrinol. (2020) 16:349–62. doi: 10.1038/s41574-020-0355-7
3. Cnop M, Igoillo-Esteve M, Hughes SJ, Walker JN, Cnop I, Clark A. Longevity of human islet α- and β-cells. Diabetes Obes Metab. (2011) 13(Suppl. 1):39–46. doi: 10.1111/j.1463-1326.2011.01443.x
4. Eizirik DL, Miani M, Cardozo AK. Signalling danger: endoplasmic reticulum stress and the unfolded protein response in pancreatic islet inflammation. Diabetologia. (2013) 56:234–41. doi: 10.1007/s00125-012-2762-3
5. Brozzi F, Eizirik DL. ER stress and the decline and fall of pancreatic β cells in type 1 diabetes. Ups J Med Sci. (2016) 121:133–9. doi: 10.3109/03009734.2015.1135217
6. Cnop M, Toivonen S, Igoillo-Esteve M, Salpea P. Endoplasmic reticulum stress and eIF2α phosphorylation: the achilles heel of pancreatic β cells. Mol Metab. (2017) 6:1024–39. doi: 10.1016/j.molmet.2017.06.001
7. Subramanian A, Narayan R, Corsello SM, Peck DD, Natoli TE, Lu X, et al. A next generation connectivity map: L1000 platform and the first 1,000,000 profiles. Cell. (2017) 171:1437–52.e17. doi: 10.1016/j.cell.2017.10.049
8. Genovese MC, Kremer J, Zamani O, Ludivico C, Krogulec M, Xie L, et al. Baricitinib in patients with refractory rheumatoid arthritis. N Engl J Med. (2016) 374:1243–52. doi: 10.1056/NEJMoa1507247
9. Wallace DJ, Furie RA, Tanaka Y, Kalunian KC, Mosca M, Petri MA, et al. Baricitinib for systemic lupus erythematosus: a double-blind, randomised, placebo-controlled, phase 2 trial. Lancet. (2018) 392:222–31. doi: 10.1016/S0140-6736(18)31363-1
10. Colli ML, Ramos-Rodriguez M, Nakayasu ES, Alvelos MI, Lopes M, Hill JLE, et al. An integrated multi-omics approach identifies the landscape of interferon-a-mediated responses of human pancreatic β cells. Nat Commun. (2020) 11:2584. doi: 10.1038/s41467-020-16327-0
11. Mallone R, Eizirik DL. Presumption of innocence in type 1 diabetes: why are β cells such vulnerable autoimmune targets? Diabetologia. (2020). [Epub ahead of print].
12. Gonzalez-Duque S, Azoury ME, Colli ML, Afonso G, Turatsinze JV, Nigi L, et al. Conventional and neo-antigenic peptides presented by β cells are targeted by circulating naive CD8+ T cells in type 1 diabetic and healthy donors. Cell Metab. (2018) 28:946–60.e6. doi: 10.1016/j.cmet.2018.07.007
13. Cnop M, Abdulkarim B, Bottu G, Cunha DA, Igoillo-Esteve M, Masini M, et al. RNA sequencing identifies dysregulation of the human pancreatic islet transcriptome by the saturated fatty acid palmitate. Diabetes. (2014) 63:1978–93. doi: 10.2337/db13-1383
14. Russell MA, Redick SD, Blodgett DM, Richardson SJ, Leete P, Krogvold L, et al. HLA class II antigen processing and presentation pathway components demonstrated by transcriptome and protein analyses of islet β-cells from donors with type 1 diabetes. Diabetes. (2019) 68:988–1001. doi: 10.2337/db18-0686
15. Xin Y, Kim J, Okamoto H, Ni M, Wei Y, Adler C, et al. RNA sequencing of single human islet cells reveals Type 2 diabetes genes. Cell Metab. (2016) 24:608–15. doi: 10.1016/j.cmet.2016.08.018
16. Patro R, Duggal G, Love MI, Irizarry RA, Kingsford C. Salmon provides fast and bias-aware quantification of transcript expression. Nat Methods. (2017) 14:417–9. doi: 10.1038/nmeth.4197
17. Frankish A, Diekhans M, Ferreira AM, Johnson R, Jungreis I, Loveland J, et al. GENCODE reference annotation for the human and mouse genomes. Nucleic Acids Res. (2019) 47:D766–73. doi: 10.1093/nar/gky955
18. Love MI, Huber W, Anders S. Moderated estimation of fold change and dispersion for RNA-seq data with DESeq2. Genome Biol. (2014) 15:550. doi: 10.1186/s13059-014-0550-8
19. Moher D, Liberati A, Tetzlaff J, Altman DG, Group P. Preferred reporting items for systematic reviews and meta-analyses: the PRISMA statement. PLoS Med. (2009) 6:e1000097. doi: 10.1371/journal.pmed.1000097
20. Plaisier SB, Taschereau R, Wong JA, Graeber TG. Rank-rank hypergeometric overlap: identification of statistically significant overlap between gene-expression signatures. Nucleic Acids Res. (2010) 38:e169. doi: 10.1093/nar/gkq636
21. Yu G, Wang LG, Han Y, He QY. clusterProfiler: an R package for comparing biological themes among gene clusters. OMICS. (2012) 16:284–7. doi: 10.1089/omi.2011.0118
22. Subramanian A, Tamayo P, Mootha VK, Mukherjee S, Ebert BL, Gillette MA, et al. Gene set enrichment analysis: a knowledge-based approach for interpreting genome-wide expression profiles. Proc Natl Acad Sci USA. (2005) 102:15545–50. doi: 10.1073/pnas.0506580102
23. Eizirik DL, Sammeth M, Bouckenooghe T, Bottu G, Sisino G, Igoillo-Esteve M, et al. The human pancreatic islet transcriptome: expression of candidate genes for type 1 diabetes and the impact of pro-inflammatory cytokines. PLoS Genet. (2012) 8:e1002552. doi: 10.1371/journal.pgen.1002552
24. Alvelos MI, Juan-Mateu J, Colli ML, Turatsinze JV, Eizirik DL. When one becomes many-Alternative splicing in β-cell function and failure. Diabetes Obes Metab. (2018) 20(Suppl. 2):77–87. doi: 10.1111/dom.13388
25. Juan-Mateu J, Rech TH, Villate O, Lizarraga-Mollinedo E, Wendt A, Turatsinze JV, et al. Neuron-enriched RNA-binding proteins regulate pancreatic β cell function and survival. J Biol Chem. (2017) 292:3466–80. doi: 10.1074/jbc.M116.748335
26. Eizirik DL, Colli ML, Ortis F. The role of inflammation in insulitis and β-cell loss in type 1 diabetes. Nat Rev Endocrinol. (2009) 5:219–26. doi: 10.1038/nrendo.2009.21
27. Santin I, Moore F, Grieco FA, Marchetti P, Brancolini C, Eizirik DL. USP18 is a key regulator of the interferon-driven gene network modulating pancreatic β cell inflammation and apoptosis. Cell Death Dis. (2012) 3:e419. doi: 10.1038/cddis.2012.158
28. Op de Beeck A, Eizirik DL. Viral infections in type 1 diabetes mellitus–why the β cells? Nat Rev Endocrinol. (2016) 12:263–73. doi: 10.1038/nrendo.2016.30
29. Nejentsev S, Walker N, Riches D, Egholm M, Todd JA. Rare variants of IFIH1, a gene implicated in antiviral responses, protect against type 1 diabetes. Science. (2009) 324:387–9. doi: 10.1126/science.1167728
30. Gorman JA, Hundhausen C, Errett JS, Stone AE, Allenspach EJ, Ge Y, et al. The A946T variant of the RNA sensor IFIH1 mediates an interferon program that limits viral infection but increases the risk for autoimmunity. Nat Immunol. (2017) 18:744–52. doi: 10.1038/ni.3766
31. Richardson SJ, Rodriguez-Calvo T, Gerling IC, Mathews CE, Kaddis JS, Russell MA, et al. Islet cell hyperexpression of HLA class I antigens: a defining feature in type 1 diabetes. Diabetologia. (2016) 59:2448–58. doi: 10.1007/s00125-016-4067-4
32. Marroqui L, Dos Santos RS, Op de Beeck A, Coomans de Brachene A, Marselli L, Marchetti P, et al. Interferon-α mediates human β cell HLA class I overexpression, endoplasmic reticulum stress and apoptosis, three hallmarks of early human type 1 diabetes. Diabetologia. (2017) 60:656–67. doi: 10.1007/s00125-016-4201-3
33. Kracht MJ, van Lummel M, Nikolic T, Joosten AM, Laban S, van der Slik AR, et al. Autoimmunity against a defective ribosomal insulin gene product in type 1 diabetes. Nat Med. (2017) 23:501–7. doi: 10.1038/nm.4289
34. Wang YJ, Traum D, Schug J, Gao L, Liu C, Consortium H, et al. Multiplexed in situ imaging mass cytometry analysis of the human endocrine pancreas and immune system in type 1 diabetes. Cell Metab. (2019) 29:769–83.e4. doi: 10.1016/j.cmet.2019.01.003
35. Lehuen A, Diana J, Zaccone P, Cooke A. Immune cell crosstalk in type 1 diabetes. Nat Rev Immunol. (2010) 10:501–13. doi: 10.1038/nri2787
36. Eizirik DL, Mandrup-Poulsen T. A choice of death–the signal-transduction of immune-mediated β-cell apoptosis. Diabetologia. (2001) 44:2115–33. doi: 10.1007/s001250100021
37. Dahlen E, Dawe K, Ohlsson L, Hedlund G. Dendritic cells and macrophages are the first and major producers of TNF-α in pancreatic islets in the nonobese diabetic mouse. J Immunol. (1998) 160:3585–93.
38. Luo JL, Kamata H, Karin M. IKK/NF-kappaB signaling: balancing life and death–a new approach to cancer therapy. J Clin Invest. (2005) 115:2625–32. doi: 10.1172/JCI26322
39. Eldor R, Yeffet A, Baum K, Doviner V, Amar D, Ben-Neriah Y, et al. Conditional and specific NF-kappaB blockade protects pancreatic β cells from diabetogenic agents. Proc Natl Acad Sci USA. (2006) 103:5072–7. doi: 10.1073/pnas.0508166103
40. Hu H, Sun SC. Ubiquitin signaling in immune responses. Cell Res. (2016) 26:457–83. doi: 10.1038/cr.2016.40
41. Ruan J, Schluter D, Wang X. Deubiquitinating enzymes (DUBs): DoUBle-edged swords in CNS autoimmunity. J Neuroinflammation. (2020) 17:102. doi: 10.1186/s12974-020-01783-8
42. Ma A, Malynn BA. A20: linking a complex regulator of ubiquitylation to immunity and human disease. Nat Rev Immunol. (2012) 12:774–85. doi: 10.1038/nri3313
43. Zhou Q, Wang H, Schwartz DM, Stoffels M, Park YH, Zhang Y, et al. Loss-of-function mutations in TNFAIP3 leading to A20 haploinsufficiency cause an early-onset autoinflammatory disease. Nat Genet. (2016) 48:67–73. doi: 10.1038/ng.3459
44. Schwartz DM, Blackstone SA, Sampaio-Moura N, Rosenzweig S, Burma AM, Stone D, et al. Type I interferon signature predicts response to JAK inhibition in haploinsufficiency of A20. Ann Rheum Dis. (2020) 79:429–31. doi: 10.1136/annrheumdis-2019-215918
45. Coomans de Brachene A, Dos Santos RS, Marroqui L, Colli ML, Marselli L, Mirmira RG, et al. IFN-α induces a preferential long-lasting expression of MHC class I in human pancreatic β cells. Diabetologia. (2018) 61:636–40. doi: 10.1007/s00125-017-4536-4
46. Trivedi PM, Graham KL, Scott NA, Jenkins MR, Majaw S, Sutherland RM, et al. Repurposed JAK1/JAK2 inhibitor reverses established autoimmune insulitis in NOD mice. Diabetes. (2017) 66:1650–60. doi: 10.2337/db16-1250
47. Sandor AM, Jacobelli J, Friedman RS. Immune cell trafficking to the islets during type 1 diabetes. Clin Exp Immunol. (2019) 198:314–25. doi: 10.1111/cei.13353
48. Ley K, Laudanna C, Cybulsky MI, Nourshargh S. Getting to the site of inflammation: the leukocyte adhesion cascade updated. Nat Rev Immunol. (2007) 7:678–89. doi: 10.1038/nri2156
49. Martin S, van den Engel NK, Vinke A, Heidenthal E, Schulte B, Kolb H. Dominant role of intercellular adhesion molecule-1 in the pathogenesis of autoimmune diabetes in non-obese diabetic mice. J Autoimmun. (2001) 17:109–17. doi: 10.1006/jaut.2001.0526
50. Glawe JD, Patrick DR, Huang M, Sharp CD, Barlow SC, Kevil CG. Genetic deficiency of Itgb2 or ItgaL prevents autoimmune diabetes through distinctly different mechanisms in NOD/LtJ mice. Diabetes. (2009) 58:1292–301. doi: 10.2337/db08-0804
51. Plagnol V, Howson JM, Smyth DJ, Walker N, Hafler JP, Wallace C, et al. Genome-wide association analysis of autoantibody positivity in type 1 diabetes cases. PLoS Genet. (2011) 7:e1002216. doi: 10.1371/journal.pgen.1002216
52. Pare G, Chasman DI, Kellogg M, Zee RY, Rifai N, Badola S, et al. Novel association of ABO histo-blood group antigen with soluble ICAM-1: results of a genome-wide association study of 6,578 women. PLoS Genet. (2008) 4:e1000118. doi: 10.1371/journal.pgen.1000118
53. Colli ML, Hill JLE, Marroqui L, Chaffey J, Dos Santos RS, Leete P, et al. PDL1 is expressed in the islets of people with type 1 diabetes and is up-regulated by interferons-α and-gamma via IRF1 induction. EBioMedicine. (2018) 36:367–75. doi: 10.1016/j.ebiom.2018.09.040
54. Stamatouli AM, Quandt Z, Perdigoto AL, Clark PL, Kluger H, Weiss SA, et al. Collateral damage: insulin-dependent diabetes induced with checkpoint inhibitors. Diabetes. (2018) 67:1471–80. doi: 10.2337/dbi18-0002
55. Wang PF, Chen Y, Song SY, Wang TJ, Ji WJ, Li SW, et al. Immune-related adverse events associated with anti-PD-1/PD-L1 treatment for malignancies: a meta-analysis. Front Pharmacol. (2017) 8:730. doi: 10.3389/fphar.2017.00730
56. Rui J, Deng S, Arazi A, Perdigoto AL, Liu Z, Herold KC. β cells that resist immunological attack develop during progression of autoimmune diabetes in NOD mice. Cell Metab. (2017) 25:727–38. doi: 10.1016/j.cmet.2017.01.005
57. Wang CJ, Chou FC, Chu CH, Wu JC, Lin SH, Chang DM, et al. Protective role of programmed death 1 ligand 1 (PD-L1)in nonobese diabetic mice: the paradox in transgenic models. Diabetes. (2008) 57:1861–9. doi: 10.2337/db07-1260
58. Zhang YC, Pileggi A, Agarwal A, Molano RD, Powers M, Brusko T, et al. Adeno-associated virus-mediated IL-10 gene therapy inhibits diabetes recurrence in syngeneic islet cell transplantation of NOD mice. Diabetes. (2003) 52:708–16. doi: 10.2337/diabetes.52.3.708
59. Robert S, Gysemans C, Takiishi T, Korf H, Spagnuolo I, Sebastiani G, et al. Oral delivery of glutamic acid decarboxylase (GAD)-65 and IL10 by Lactococcus lactis reverses diabetes in recent-onset NOD mice. Diabetes. (2014) 63:2876–87. doi: 10.2337/db13-1236
60. LaPorte SL, Juo ZS, Vaclavikova J, Colf LA, Qi X, Heller NM, et al. Molecular and structural basis of cytokine receptor pleiotropy in the interleukin-4/13 system. Cell. (2008) 132:259–72. doi: 10.1016/j.cell.2007.12.030
61. Wammes LJ, Mpairwe H, Elliott AM, Yazdanbakhsh M. Helminth therapy or elimination: epidemiological, immunological, and clinical considerations. Lancet Infect Dis. (2014) 14:1150–62. doi: 10.1016/S1473-3099(14)70771-6
62. Mi QS, Ly D, Zucker P, McGarry M, Delovitch TL. Interleukin-4 but not interleukin-10 protects against spontaneous and recurrent type 1 diabetes by activated CD1d-restricted invariant natural killer T-cells. Diabetes. (2004) 53:1303–10. doi: 10.2337/diabetes.53.5.1303
63. Zaccone P, Phillips J, Conget I, Gomis R, Haskins K, Minty A, et al. Interleukin-13 prevents autoimmune diabetes in NOD mice. Diabetes. (1999) 48:1522–8. doi: 10.2337/diabetes.48.8.1522
64. Russell MA, Cooper AC, Dhayal S, Morgan NG. Differential effects of interleukin-13 and interleukin-6 on Jak/STAT signaling and cell viability in pancreatic β-cells. Islets. (2013) 5:95–105. doi: 10.4161/isl.24249
65. Leslie KA, Russell MA, Taniguchi K, Richardson SJ, Morgan NG. The transcription factor STAT6 plays a critical role in promoting β cell viability and is depleted in islets of individuals with type 1 diabetes. Diabetologia. (2019) 62:87–98. doi: 10.1007/s00125-018-4750-8
66. Mastracci TL, Turatsinze JV, Book BK, Restrepo IA, Pugia MJ, Wiebke EA, et al. Distinct gene expression pathways in islets from individuals with short- and long-duration type 1 diabetes. Diabetes Obes Metab. (2018) 20:1859–67. doi: 10.1111/dom.13298
67. Martino L, Masini M, Bugliani M, Marselli L, Suleiman M, Boggi U, et al. Mast cells infiltrate pancreatic islets in human type 1 diabetes. Diabetologia. (2015) 58:2554–62. doi: 10.1007/s00125-015-3734-1
68. t Hart LM, Fritsche A, Nijpels G, van Leeuwen N, Donnelly LA, Dekker JM, et al. The CTRB1/2 locus affects diabetes susceptibility and treatment via the incretin pathway. Diabetes. (2013) 62:3275–81. doi: 10.2337/db13-0227
69. Barrett JC, Clayton DG, Concannon P, Akolkar B, Cooper JD, Erlich HA, et al. Genome-wide association study and meta-analysis find that over 40 loci affect risk of type 1 diabetes. Nat Genet. (2009) 41:703–7. doi: 10.1038/ng.381
70. Ebrahimi AG, Hollister-Lock J, Sullivan BA, Tsuchida R, Bonner-Weir S, Weir GC. cell identity changes with mild hyperglycemia: implications for function, growth, and vulnerability. Mol Metab. (2020) 35:100959. doi: 10.1016/j.molmet.2020.02.002
71. Aguirre JE, Beswick EJ, Grim C, Uribe G, Tafoya M, Chacon Palma G, et al. Matrix metalloproteinases cleave membrane-bound PD-L1 on CD90+ (myo-)fibroblasts in Crohn's disease and regulate Th1/Th17 cell responses. Int Immunol. (2020) 32:57–68. doi: 10.1093/intimm/dxz060
72. Patterson CC, Dahlquist GG, Gyurus E, Green A, Soltesz G, Group ES. Incidence trends for childhood type 1 diabetes in Europe during 1989-2003 and predicted new cases 2005-20: a multicentre prospective registration study. Lancet. (2009) 373:2027–33. doi: 10.1016/S0140-6736(09)60568-7
73. Mayer-Davis EJ, Dabelea D, Lawrence JM. Incidence trends of type 1 and type 2 diabetes among youths, 2002-2012. N Engl J Med. (2017) 377:301. doi: 10.1056/NEJMc1706291
74. Engin F, Yermalovich A, Nguyen T, Hummasti S, Fu W, Eizirik DL, et al. Restoration of the unfolded protein response in pancreatic β cells protects mice against type 1 diabetes. Sci Transl Med. (2013) 5:211ra156. doi: 10.1126/scitranslmed.3006534
75. Lombardi A, Tomer Y. Interferon α impairs insulin production in human β cells via endoplasmic reticulum stress. J Autoimmun. (2017) 80:48–55. doi: 10.1016/j.jaut.2017.02.002
76. Mele DA, Salmeron A, Ghosh S, Huang HR, Bryant BM, Lora JM. BET bromodomain inhibition suppresses TH17-mediated pathology. J Exp Med. (2013) 210:2181–90. doi: 10.1084/jem.20130376
77. Wei S, Sun Y, Sha H. Therapeutic targeting of BET protein BRD4 delays murine lupus. Int Immunopharmacol. (2015) 29:314–9. doi: 10.1016/j.intimp.2015.10.036
78. Fu W, Farache J, Clardy SM, Hattori K, Mander P, Lee K, et al. Epigenetic modulation of type-1 diabetes via a dual effect on pancreatic macrophages and β cells. Elife. (2014) 3:e04631. doi: 10.7554/eLife.04631
79. Ahmadi Rastegar D, Dzamko N. Leucine rich repeat kinase 2 and innate immunity. Front Neurosci. (2020) 14:193. doi: 10.3389/fnins.2020.00193
80. West AB, Moore DJ, Biskup S, Bugayenko A, Smith WW, Ross CA, et al. Parkinson's disease-associated mutations in leucine-rich repeat kinase 2 augment kinase activity. Proc Natl Acad Sci USA. (2005) 102:16842–7. doi: 10.1073/pnas.0507360102
81. Hui KY, Fernandez-Hernandez H, Hu J, Schaffner A, Pankratz N, Hsu NY, et al. Functional variants in the LRRK2 gene confer shared effects on risk for Crohn's disease and Parkinson's disease. Sci Transl Med. (2018) 10:aai7795. doi: 10.1126/scitranslmed.aai7795
82. Han KA, Yoo L, Sung JY, Chung SA, Um JW, Kim H, et al. Leucine-rich repeat kinase 2 (LRRK2) stimulates IL-1β-mediated inflammatory signaling through phosphorylation of RCAN1. Front Cell Neurosci. (2017) 11:125. doi: 10.3389/fncel.2017.00125
83. Kozina E, Sadasivan S, Jiao Y, Dou Y, Ma Z, Tan H, et al. Mutant LRRK2 mediates peripheral and central immune responses leading to neurodegeneration in vivo. rain. (2018) 141:1753–69. doi: 10.1093/brain/awy077
84. Liu W, Liu X, Li Y, Zhao J, Liu Z, Hu Z, et al. LRRK2 promotes the activation of NLRC4 inflammasome during Salmonella Typhimurium infection. J Exp Med. (2017) 214:3051–66. doi: 10.1084/jem.20170014
85. Moehle MS, Webber PJ, Tse T, Sukar N, Standaert DG, DeSilva TM, et al. LRRK2 inhibition attenuates microglial inflammatory responses. J Neurosci. (2012) 32:1602–11. doi: 10.1523/JNEUROSCI.5601-11.2012
86. Funk N, Munz M, Ott T, Brockmann K, Wenninger-Weinzierl A, Kuhn R, et al. The Parkinson's disease-linked Leucine-rich repeat kinase 2 (LRRK2) is required for insulin-stimulated translocation of GLUT4. Sci Rep. (2019) 9:4515. doi: 10.1038/s41598-019-40808-y
87. Riachy R, Vandewalle B, Belaich S, Kerr-Conte J, Gmyr V, Zerimech F, et al. Beneficial effect of 1,25 dihydroxyvitamin D3 on cytokine-treated human pancreatic islets. J Endocrinol. (2001) 169:161–8. doi: 10.1677/joe.0.1690161
88. Riachy R, Vandewalle B, Kerr Conte J, Moerman E, Sacchetti P, Lukowiak B, et al. 1,25-dihydroxyvitamin D3 protects RINm5F and human islet cells against cytokine-induced apoptosis: implication of the antiapoptotic protein A20. Endocrinology. (2002). 143:4809–19. doi: 10.1210/en.2002-220449
89. Gysemans CA, Cardozo AK, Callewaert H, Giulietti A, Hulshagen L, Bouillon R, et al. 1,25-Dihydroxyvitamin D3 modulates expression of chemokines and cytokines in pancreatic islets: implications for prevention of diabetes in nonobese diabetic mice. Endocrinology. (2005) 146:1956–64. doi: 10.1210/en.2004-1322
90. Miettinen ME, Niinisto S, Erlund I, Cuthbertson D, Nucci AM, Honkanen J, et al. Serum 25-hydroxyvitamin D concentration in childhood and risk of islet autoimmunity and type 1 diabetes: the TRIGR nested case-control ancillary study. Diabetologia. (2020) 63:780–7. doi: 10.1007/s00125-019-05077-4
91. Norris JM, Lee HS, Frederiksen B, Erlund I, Uusitalo U, Yang J, et al. Plasma 25-Hydroxyvitamin D concentration and risk of islet autoimmunity. Diabetes. (2018) 67:146–54. doi: 10.2337/db17-0802
92. Herold KC, Bundy BN, Long SA, Bluestone JA, DiMeglio LA, Dufort MJ, et al. An anti-CD3 antibody, teplizumab, in relatives at risk for type 1 diabetes. N Engl J Med. (2019) 381:603–13. doi: 10.1056/NEJMoa1902226
93. Keymeulen B, Vandemeulebroucke E, Ziegler AG, Mathieu C, Kaufman L, Hale G, et al. Insulin needs after CD3-antibody therapy in new-onset type 1 diabetes. N Engl J Med. (2005) 352:2598–608. doi: 10.1056/NEJMoa043980
94. Pescovitz MD, Greenbaum CJ, Bundy B, Becker DJ, Gitelman SE, Goland R, et al. B-lymphocyte depletion with rituximab and β-cell function: two-year results. Diabetes Care. (2014) 37:453–9. doi: 10.2337/dc13-0626
95. Ramos-Rodriguez M, Raurell-Vila H, Colli ML, Alvelos MI, Subirana-Granes M, Juan-Mateu J, et al. The impact of proinflammatory cytokines on the β-cell regulatory landscape provides insights into the genetics of type 1 diabetes. Nat Genet. (2019) 51:1588–95. doi: 10.1101/560193
96. Nakayasu ES, Syed F, Tersey SA, Gritsenko MA, Mitchell HD, Chan CY, et al. Comprehensive proteomics analysis of stressed human islets identifies GDF15 as a target for type 1 diabetes intervention. Cell Metab. (2020) 31:363–74 e6. doi: 10.1016/j.cmet.2019.12.005
97. Thompson PJ, Shah A, Ntranos V, van Gool F, Atkinson M, Bhushan A. Targeted elimination of senescent β cells prevents type 1 diabetes. Cell Metab. (2019) 29:1045–60.e10. doi: 10.1016/j.cmet.2019.01.021
98. Aguayo-Mazzucato C, Andle J, Lee TB Jr, Midha A, Talemal L, Chipashvili V, et al. Acceleration of β cell aging determines diabetes and senolysis improves disease outcomes. Cell Metab. (2019) 30:129–42.e4. doi: 10.1016/j.cmet.2019.05.006
99. Kaestner KH, Powers AC, Naji A, Consortium H, Atkinson MA. NIH initiative to improve understanding of the pancreas, islet, and autoimmunity in type 1 diabetes: the human pancreas analysis program (HPAP). Diabetes. (2019) 68:1394–402. doi: 10.2337/db19-0058
100. Brissova M, Haliyur R, Saunders D, Shrestha S, Dai C, Blodgett DM, et al. α cell function and gene expression are compromised in type 1 diabetes. Cell Rep. (2018) 22:2667–76. doi: 10.1016/j.celrep.2018.02.032
101. Der E, Suryawanshi H, Morozov P, Kustagi M, Goilav B, Ranabathou S, et al. Tubular cell and keratinocyte single-cell transcriptomics applied to lupus nephritis reveal type I IFN and fibrosis relevant pathways. Nat Immunol. (2019) 20:915–27. doi: 10.1038/s41590-019-0386-1
Keywords: type 1 diabetes, beta cells, pancreatic islets, insulitis, inflammation, therapeutics, interferon, RNA-sequencing
Citation: Colli ML, Szymczak F and Eizirik DL (2020) Molecular Footprints of the Immune Assault on Pancreatic Beta Cells in Type 1 Diabetes. Front. Endocrinol. 11:568446. doi: 10.3389/fendo.2020.568446
Received: 01 June 2020; Accepted: 17 August 2020;
Published: 15 September 2020.
Edited by:
Todd M. Brusko, University of Florida, United StatesReviewed by:
Guoqiang Gu, Vanderbilt University, United StatesScott Soleimanpour, University of Michigan, United States
Copyright © 2020 Colli, Szymczak and Eizirik. This is an open-access article distributed under the terms of the Creative Commons Attribution License (CC BY). The use, distribution or reproduction in other forums is permitted, provided the original author(s) and the copyright owner(s) are credited and that the original publication in this journal is cited, in accordance with accepted academic practice. No use, distribution or reproduction is permitted which does not comply with these terms.
*Correspondence: Maikel L. Colli, bWNvbGxpJiN4MDAwNDA7dWxiLmFjLmJl