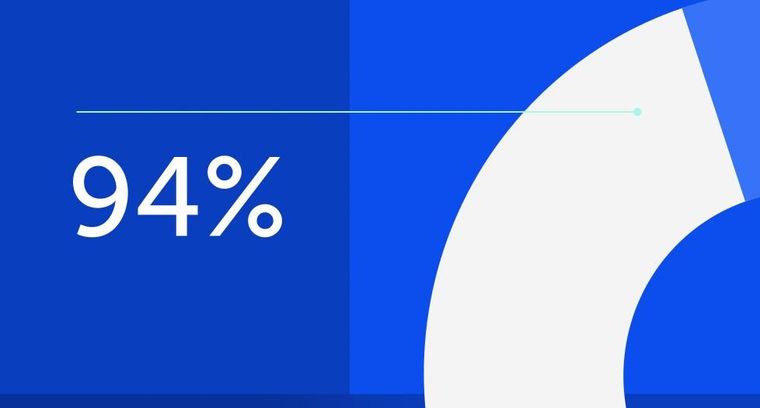
94% of researchers rate our articles as excellent or good
Learn more about the work of our research integrity team to safeguard the quality of each article we publish.
Find out more
SYSTEMATIC REVIEW article
Front. Endocrinol., 14 August 2020
Sec. Obesity
Volume 11 - 2020 | https://doi.org/10.3389/fendo.2020.00562
This article is part of the Research TopicCovid-19 and ObesityView all 16 articles
A better understanding of the SARS-CoV-2 virus behavior and possible risk factors implicated in poor outcome has become an urgent need. We performed a systematic review in order to investigate a possible association between body weight and prognosis among patients diagnosed with COVID-19. We searched in Cochrane Library, EMBASE, MEDLINE, WHO-Global Literature on Coronavirus Disease, OpenGrey, and Medrxiv. We used the ROBINS-I tool or Cross-Sectional/Prevalence Study Quality tool from AHRQ, to evaluate the methodological quality of included studies. Nine studies (two prospective cohorts, four retrospective cohorts and three cross-sectional) were included and assessed the relationship between obesity and COVID-19 prognosis. Risk of bias of the included studies ranged from moderate to critical. Clinical and methodological heterogeneity among them precluded meta-analyses. Most of the included studies showed some degree of association to: (a) higher BMI and worse clinical presentation and (b) obesity and need of hospitalization. The results were inconsistent about the impact of obesity on mortality. Based on limited methodological quality studies, obesity seems to predict poor clinical evolution in patients with COVID-19. Further studies with appropriate prospective design are needed to reduce the uncertainty on this evidence.
A better understanding of the SARS-CoV-2 virus behavior has become an urgent need as the pandemic caused continues to plague the world adding more and more victims. A series of reports have looked for risk factors in order to provide means of prevention and treatment to the population. The first Chinese publications made clear that age may impact prognosis (1–3), but with the advance of COVID-19 to western European and North American countries, some novel factors have emerged as determinants of risk and poor outcome. In contrast to China, there is a high prevalence of obesity in these countries (4) that may help explain, at least in part, the reason why obesity has just emerged as a marker of unfavorable clinical evolution.
The prevalence of obesity has rapidly increased over the years (5), especially among elderly (6). Obesity is a multifactorial disorder characterized by excessive fat accumulation and an increment of proinflammatory cytokines, which entails a constant state of immune deregulation (7). This chronic deregulation may interfere with immune homeostasis and impair the effectiveness of the immune response. It is not without reason that obesity has been implicated in poor outcomes among many clinical conditions and high all-cause mortality (8).
However, although it accumulates rapidly, data on the role of obesity on COVID-19 risk and prognosis are still confusing and hard to interpret. Scrambling to learn more about the virus, doctors and scientists try to rapidly share their findings generating a large flood of publications that has put new strain on a scientific process accustomed to vetting and publishing new results much more slowly.
Herein, we perform a systematic review in order to evaluate if overweight and obesity may predict poor outcome in patients with COVID-19.
To investigate a possible association between body weight and prognosis among patients diagnosed with COVID-19.
The clinical question is, as structured through the PECO acronym: (P, population): individuals with COVID-19; (E, exposure): overweight or obesity); (C, comparator): normal body weight; (O, outcomes): clinical, laboratory and image outcomes on COVID-19.
This was a systematic review carried out in the Universidade Federal de São Paulo (Unifesp) through a collaboration with the University of Campinas (Unicamp), Brazil. The study was conducted in accordance with the AMSTAR-2 (Assessing the Methodological quality of Systematic Reviews) (9). The protocol was prospectively registered at the PROSPERO database (registration number CDR42020182189, https://www.crd.york.ac.uk/prospero/display_record.php?RecordID=182189). This reporting was written following the PRISMA statement (10).
We considered any study design using a comparative group as follows: controlled trials (randomized, quasi-randomized, or non-randomized) that conducted subgroup analyses according to body weight, cohort and case-control studies, and analytic cross-sectional studies with a control group.
Adults or children with confirmed diagnosis of COVID-19, in accordance with World Health Organization criteria (11).
We considered any definition of overweight or obesity, as assumed by the authors of primary studies. However, only similar definitions were evaluated together into quantitative or qualitative synthesis.
We considered all clinical, laboratory and image outcomes as presented by the authors of primary studies. However, we prioritized the outcomes below:
Primary outcomes:
• All-cause mortality;
• Serious adverse events: assessed by the rate of participants who experienced at least one serious adverse event, as per defined as those that are life-threatening; which may lead to death, requirement of a treatment in an emergency room, hospitalization (initial or prolonged), disability or permanent damage, or congenital anomaly/birth defect (12).
• SARS-CoV-2 acute respiratory syndrome: assessed by the rate of participants who progressed to acute respiratory syndrome.
• Clinical status, assessed by the Ordinal Scale for Clinical Improvement—World Health Organization (scale from 0 to 7, the higher the score, the worse the clinical condition), as defined by the World Health Organization (WHO) (13).
Secondary outcomes:
• Mortality related to SARS-CoV-2 infection (COVID-19);
• Any adverse event: assessed by the rate of participants who experienced at least one adverse event.
• Time to clinical improvement, defined as a reduction of at least two points in the score of the Ordinal Scale for Clinical Improvement—World Health Organization (scale from 0 to 7, the higher the score, the worse the clinical condition), as defined by the World Health Organization (WHO) (13).
• Hospitalization in an intensive care unit;
• Need for invasive mechanical ventilation;
• Length of hospitalization.
• Length of hospitalization in intensive care unit;
• Length of invasive mechanical ventilation;
• Rate of negative PCR viral load (any specimen).
We assessed all dichotomous outcomes listed above at any time point. However, we only pooled similar time points together: short term (up to 1 month, inclusive) or long term (more than 1 month). When a study reported an outcome more than once in the same period, we considered the last measurement.
A comprehensive search of the literature was carried out using an electronic search with no restriction regarding date, language or status of publication. Sensitive search strategies (Supplementary File 1) were developed for the following databases:
• Cochrane Library (via Wiley);
• EMBASE (via Elsevier);
• MEDLINE (via PubMed);
• World Health Organization—Global Literature on Coronavirus Disease (https://search.bvsalud.org/global-literature-on-novel-coronavirus-2019-ncov/).
A search for gray literature was conducted in the Opengrey database (https://opengrey.eu) and for preprint studies in the Medrxiv (https://www.medrxiv.org/). Manual search was performed in the reference lists of the relevant studies.
The selection process was conducted in a two-stage process aided by the Rayyan platform (14). In the first phase, two review authors independently assessed all titles and abstracts retrieved by the search strategies. Studies marked as “potentially eligible” were then screened at the second phase, which consisted in the reading of the full text to confirm its eligibility. Any divergence was solved by a third reviewer. Studies excluded in the second phase were presented in the “excluded studies table” and the reasons for exclusion as well.
The procedures for data extraction were performed by two independent reviewers and a pre-established data extraction form was used. Disagreements in this process were solved by a third reviewer.
The methodological quality of the included studies was evaluated by two independent reviewers by the use of validated tools for each study design, as following:
• Randomized controlled trial: Cochrane Risk of Bias Table (15);
• Non-randomized, quasi-randomized trial: ROBINS-I (16);
• Cohort or case-control: ROBINS-I (16). ROBINS-I was used as there are, as yet, no draft versions of ROBINS-E available. The domains “classification of interventions” and “deviations from intended interventions” were adapted to consider “exposures” instead of “interventions.”
• Cross-sectional: Cross-Sectional/Prevalence Study Quality, Agency for Healthcare Research and Quality (17).
The unit of analysis was the individual. Considering the context requiring a rapid answer, the authors from primary studies were not contacted for missing data.
Depending on data availability and homogeneity of studies, we planned to pool results from similar studies by random-effects meta-analyses (software Review Manager 5.3). Risk ratios (or odds ratios) and mean differences would be calculated for dichotomous and continuous data, respectively. A 95% confidence interval would be considered for the analyses. When meta-analysis was not possible the results were presented as qualitative synthesis (descriptive presentation).
Methodological and clinical diversity of included studies would be considered for conducting or not meta-analyses. The existence of statistical heterogeneity would be evaluated by Chi2 test and its extension by the I2 test (I2 ≥ 50% indicates high heterogeneity among studies).
We planned to conduct the following subgroup analyses: (a) presence of diabetes and/or hypertension and (b) age of participants (< 65 vs. ≥65).
We planned to conduct the following subgroup analyses: (a) fixed effects vs. random effects model meta-analysis. When the results of fixed effect meta-analysis provide a different result, both would be reported; (b) excluding from analysis studies at high risk of bias; and (c) excluding from analysis unpublished studies or those available exclusively in a pre-print version and not peer reviewed.
Investigation of publication bias assessment was planned by visual inspection of funnel plots for meta-analysis with at least 10 studies.
However, due to heterogeneity between included studies it was not possible to conduct meta- analyses nor additional analyses.
The search retrieved 937 records. After excluding 88 duplicates, we screened the titles and abstracts of 849 references, excluded 836 that did not comprise inclusion criteria, and selected 13 for full text reading. We excluded four studies (detailed below). Therefore, the review included nine observational studies. The flow diagram of the process of study identification and selection is presented in Figure 1.
This systematic review included nine studies: two prospective cohort studies (18, 19), four retrospective cohort studies (20–23), and three cross-sectional studies (24–26). Table 1 presents the main characteristics of the included studies. Table 2 summarizes the studies excluded after selection.
BMI was the only measure used as a criteria for classifying body weight, considered as a continuous or ordinal scale variable. The included studies had different study designs and considered different outcomes of interest.
Due to these clinical and methodological heterogeneity among included studies it was not appropriate to conduct meta-analyses.
Risk of bias assessment of the included studies and reasons for judgement are presented in Tables 3, 4. Overall, cohort studies were classified as critical to moderate risk of bias, and cross-sectional studies varied between 36 and 63% of agreement with bias domains.
Table 3. Risk of bias of cohort studies: ROBINS-I (16).
Table 4. Risk of bias of cross-sectional studies: Cross-Sectional/Prevalence Study Quality, Agency for Healthcare Research and Quality (AHRQ) (17).
We reviewed data from 17,568 patients with SARS-CoV-2 infection, included in nine studies. Most of these studies highlighted some level of association between obesity and disease severity, encompassing hospitalization rate, admission to ICU, invasive ventilation need and mortality. According to validated tools, these studies presented moderate to critical risk of bias, which limits the reliability in the results.
According to COVIDView database of Centers for Disease Control and Prevention (CDC) in the USA, until May 2, 2020 the overall rate for COVID-19-associated hospitalization were 162.2 per 100,00 in individuals 65 years and older, decreasing to 79.0–26.2 for individuals < 65 years. Furthermore, preliminary data showed that about 91.5% of hospitalized patients present at least one underlying medical condition. Besides obesity, the most common critical comorbidities observed in the hospitalized COVID-19 patients were hypertension, metabolic disease, cardiovascular and pulmonary diseases (31).
Three of 4 North American studies showed increased BMI among patients who required hospitalization. Argenziano et al. (24) also described that patients who require in-hospital admission had more chronic diseases, such as hypertension, diabetes, and obesity. To date, ICU patients presented significantly higher BMI compared to those admitted in the emergency or inpatient floors. Lighter and colleagues (20) analyzed retrospectively a cohort of 3,615 patients positive for COVID-19 stratified by age. Thirty-eight percent (38%) of these patients presented BMI >30 kg/m2. An increased risk of hospitalization in acute care or ICU was demonstrated for patients < 60 years older with obesity (BMI 30–34 kg/m2) and severe obesity (BMI ≥35 kg/m2) compared to patients BMI < 30 kg/m2. Once younger patients generally do not represent higher risk for a severe presentation of COVID-19, authors suggest that obesity may be an unrecognized risk factor for hospital care. In a cross-sectional study, Petrilli et al. (26) showed that hospitalized patients were more likely to be male and present cardiovascular diseases, diabetes and obesity. In fact, as confirmed by a multivariate analysis, obesity (BMI > 40 kg/m2), older age (≥65 years) and history of heart failure were independent predictors of unfavorable outcome. Cummings et al. (18) observed similar prevalence of obesity among hospitalized patients. However, authors failed to demonstrate that obesity is a predictor of mortality.
Two cohort studies evaluate the severity of COVID-19 disease in Chinese patients. The retrospective study by Liu et al. (21) evaluated 30 medical staff infected with novel coronavirus in January, 2020. Most of them presented a common type of the disease (n = 26) and four patients a more severe condition defined as pulmonary insufficiency. Until the end of the study, 80% of the patients were discharged, none of them needed critical hospital care or died. In relation to obesity the authors reported higher BMI in patients with severe compared to the mild presentation. Zheng et al. (19) prospectively evaluated 66 patients with metabolic associated fatty liver disease (MAFLD) stratified by obesity status dividing patients according to severe and non-severe COVID-19 based on the National Health Commission & State Administration of Traditional Chinese Medicine. Frequency of obesity was higher between severe disease patients compared to non-severe, furthermore MAFLD patients with concurrent obesity had more severe presentation of the disease. Indeed, obesity in patients with MAFLD increased the risk of severe illness in almost 6-fold (unadjusted OR 5.77, 95% CI 1.19–27.91, p = 0.029). After adjustment for age, sex, smoking, diabetes, hypertension, and dyslipidemia, association with obesity and COVID-19 remained significant and confirmed obesity as an independent marker of critical illness. However, as commented by Hussain et al. (32), MAFLD and obesity are rarely considered as independent conditions, in the cases of concurrent diseases they coexist due to obesity. A third Chinese study (22) demonstrated that mean BMI of the 16 patients who needed ICU care (25.5 kg/m2) were higher than the general group (22.0 kg/m2). Between the 17 deaths reported, 88% had BMI >25 kg/m2. Most of the deceased patients also presented hypertension, coronary heart disease and heart insufficiency.
In a Mexican study (25), obese patients, as expected, had higher proportions of other comorbidities as hypertension, diabetes, cardiovascular disease, asthma, and chronic obstructive pulmonary disease (COPD). Increased lethality of COVID-19 was reported specially in patients with diabetes, early onset diabetes (< 40 years), concurrent obesity or several concurrent comorbidities (p < 0.001). As presented in Table 1, obese patients had higher risks of hospitalization, pneumonia, ICU admission, invasive ventilation and 7-fold increased risk of mortality.
Simonnet et al. (23) described that the distribution of BMI categories in COVID-19 patients admitted to ICU care in France differed from the control patients with non-SARS-Cov-2 respiratory disease. The frequency of obesity (BMI > 30 kg/m2, 47.6%) and severe obesity (BMI > 35 kg/m2, 28.2%) were higher among patients with COVID-19 infection compared to control patients (25.2 and 10.8%, respectively). Besides, the median of BMI (31.1 kg/m2) of the 85 patients who required invasive ventilation was higher than the patients who did not (27.0 kg/m2, n = 39). An univariate logistic regression analysis showed that BMI ≥35 kg/m2 (vs. BMI < 25 kg/m2) was a risk for need of invasive ventilation (OR 6.75, 95%CI 1.76–25.85, p = 0.015), remaining significant after adjustment for age, diabetes and hypertension in a multivariate analysis (OR 7.36 95% CI 1.63–33.14, p = 0.021).
This systematic review presents some strengths including the use of stringent methods of Cochrane reviews, reproduced in a short term due to the need of rapid responses to guide clinical decisions during the pandemic. The search for studies was highly sensitive and it was conducted in formal databases, preprint, and gray literature repositories and specific sources for COVID-19 as well.
The present study has some limitations. The included studies adopted different methods to assess obesity as a predictor of poor outcome precluding a meta-analysis. Once COVID-19 is a public health emergency, a considerable amount of research is being published every week and it is possible that recent articles may not be included in the final version of our review. For assessing the risk of bias of cohort studies, we slightly adapted the ROBINS-I tool, since there are, as yet, no draft versions of ROBINS-E available. This adaptation was inconspicuous and sought to preserve the definition of domains.
In summary, our systematic review suggests that obesity is likely to be a predictor of poor outcome in patients with COVID-19, in all continents. Obesity is associated with several clinical conditions (e.g., diabetes and hypertension). It is associated with restrictive lung ventilatory defect, which may worsen the severe respiratory failure syndrome. In obesity, the dysfunctional adipocytes produce massive amounts of pro-inflammatory cytokines, which entails a chronic inflammation, harming innate and adaptative immune responses (33–35). The increase in pro-inflammatory cytokines observed among obese patients may add to the inflammatory response triggered by the SARS-CoV-2, and both contribute to poor outcome and high all-cause mortality (7, 8). Likewise, obesity is a well-established risk factor for cardiovascular disease, which triggers diverse physiologic alterations that include activation of renin-angiotensin-aldosterone system, reduction of vasculo-protective effects, upregulation of procoagulant factors, downregulation of anticoagulant factors and chronic oxidative stress and inflammation (36–38). Hence, obese patients with COVID-19 may benefit from an aggressive approach, including eager evaluation and early hospitalization. In addition, health politics may assure obese patients prompt access to the health care system. The investigation of the mechanisms that may be underlying the association between obesity and poor outcome in patients with COVID-19 will certainly help the understanding of this subject. Therefore, further studies with appropriate prospective design are needed to reduce the uncertainty on this evidence.
All datasets presented in this study are included in the article/Supplementary Material.
KP contributed to the design, critical review of the literature and data, composition of the manuscript, and final approval. RR and AM contributed to the design, critical review of the literature and data, risk of bias, composition of the manuscript, and final approval. LW contributed to the design, selection of the notable articles for review, critical review of the literature and data, composition of the manuscript, clinical and translational orientation, and final approval. LC contributed to the conception and design, selection of the notable articles for review, critical review of the literature and data, composition of the manuscript, and final approval. All authors contributed to the article and approved the submitted version.
The authors declare that the research was conducted in the absence of any commercial or financial relationships that could be construed as a potential conflict of interest.
The Supplementary Material for this article can be found online at: https://www.frontiersin.org/articles/10.3389/fendo.2020.00562/full#supplementary-material
1. Zhang JJ, Dong X, Cao YY, Yuan YD, Yang YB, Yan YQ, et al. Clinical characteristics of 140 patients infected with SARS-CoV-2 in Wuhan, China. Allergy. (2020) 75:1730–41. doi: 10.1111/all.14238
2. Yang X, Yu Y, Xu J, Shu H, Xia J, Liu H, et al. Clinical course and outcomes of critically ill patients with SARS-CoV-2 pneumonia in Wuhan, China: a single-centered, retrospective, observational study. Lancet Respir Med. (2020) 8:475–81. doi: 10.1016/S2213-2600(20)30079-5
3. Huang C, Wang Y, Li X, Ren L, Zhao J, Hu Y, et al. Clinical features of patients infected with 2019 novel coronavirus in Wuhan, China. Lancet. (2020) 395:497–506. doi: 10.1016/S0140-6736(20)30183-5
4. World Health Organization (WHO). Global Health Observatory (GHO) Data. Overweight and Obesity. Prevalence of Obesity Among Adults. (2016). Available online at: https://www.who.int/gho/ncd/risk_factors/overweight_obesity/obesity_adults/en/ (accessed April 25, 2020).
5. Ford ES, Mokdad AH. Epidemiology of obesity in the Western Hemisphere. J Clin Endocrinol Metab. (2008) 93:S1–8. doi: 10.1210/jc.2008-1356
6. Peralta M, Ramos M, Lipert A, Martins J, Marques A. Prevalence and trends of overweight and obesity in older adults from 10 European countries from 2005 to 2013. Scand J Public Health. (2018) 46:522–9. doi: 10.1177/1403494818764810
7. Tilg H, Moschen AR. Adipocytokines: mediators linking adipose tissue, inflammation and immunity. Nat Rev Immunol. (2006) 6:772–83. doi: 10.1038/nri1937
8. Lavie CJ, Laddu D, Arena R, Ortega FB, Alpert MA, Kushner RF. Healthy weight and obesity prevention: JACC health promotion series. J Am Coll Cardiol. (2018) 72:1506–31. doi: 10.1016/j.jacc.2018.08.1037
9. Shea BJ, Reeves BC, Wells G, Thuku M, Hamel C, Moran J, et al. AMSTAR 2: a critical appraisal tool for systematic reviews that include randomised or non-randomised studies of healthcare interventions, or both. BMJ. (2017) 358:j4008. doi: 10.1136/bmj.j4008
10. Moher D, Liberati A, Tetzlaff J, Altman DG, Altman D, Antes G, et al. Preferred reporting items for systematic reviews and meta-analyses: the PRISMA statement. PLoS Med. (2009) 6:e1000097. doi: 10.1371/journal.pmed.1000097
11. World Health Organization (WHO). Global Surveillance for COVID-19 Caused by Human Infection With COVID-19 Virus. Interim Guid 20 March 2020 (2020). Available online at: https://www.who.int/docs/default-source/coronaviruse/global-surveillance-for-COVID-v-19-final200321-rev.pdf (accessed April 22, 2020).
12. U.S. Food and Drug Administration (FDA). U.S Food and Drug Administration. What Is a Serious Advers Event? Content Curr as 02/01/2016 (2016). Available online at: https://www.fda.gov/safety/reporting-serious-problems-fda/what-serious-adverse-event (accessed April 22, 2020).
13. WHO R&D Blueprint. Novel Coronavirus. COVID-19 Therapeutic Trial Synopsis. (2020). Available online at: https://www.who.int/blueprint/priority-diseases/key-action/COVID-19_Treatment_Trial_Design_Master_Protocol_synopsis_Final_18022020.pdf (accessed April 22, 2020).
14. Ouzzani M, Hammady H, Fedorowicz Z, Elmagarmid A. Rayyan—a web and mobile app for systematic reviews. Syst Rev. (2016) 5:210. doi: 10.1186/s13643-016-0384-4
15. Higgins JPT, Thomas J, Chandler J, Cumpston M, Li T, Page MJ, et al. Cochrane Handbook for Systematic Reviews of Interventions Version 6.0 (Updated July 2019). Cochrane (2019). Available online at: https://www.training.cochrane.org/handbook (accessed April 22, 2020).
16. Sterne JA, Hernán MA, Reeves BC, Savović J, Berkman ND, Viswanathan M, et al. ROBINS-I: a tool for assessing risk of bias in non-randomised studies of interventions. BMJ. (2016) 355:i4919. doi: 10.1136/bmj.i4919
17. Rostom A, Dubé C, Cranney A, Saloojee N, Sy R, Garritty C, et al. Celiac Disease. Evidence Reports/Technology Assessments, No. 104. Appendix D: Quality Assessment Forms. (2004). Available online at: https://www.ncbi.nlm.nih.gov/books/NBK35156/ (accessed April 22, 2020).
18. Cummings MJ, Baldwin MR, Abrams D, Jacobson SD, Meyer BJ, Balough EM, et al. Epidemiology, clinical course, and outcomes of critically ill adults with COVID-19 in New York City: a prospective cohort study. medRxiv. (2020). doi: 10.1016/S0140-6736(20)31189-2
19. Zheng KI, Gao F, Wang X-B, Sun Q-F, Pan K-H, Wang T-Y, et al. Obesity as a risk factor for greater severity of COVID-19 in patients with metabolic associated fatty liver disease. Metabolism. (2020) 108:154244. doi: 10.1016/j.metabol.2020.154244
20. Lighter J, Phillips M, Hochman S, Sterling S, Johnson D, Francois F, et al. Obesity in patients younger than 60 years is a risk factor for Covid-19 hospital admission. Clin Infect Dis. (2020) 71:896–7. doi: 10.1093/cid/ciaa415
21. Liu M, He P, Liu HG, Wang XJ, Li FJ, Chen S, et al. Clinical characteristics of 30 medical workers infected with new coronavirus pneumonia. Zhonghua Jie He He Hu Xi Za Zhi. (2020) 43:209–214. doi: 10.3760/cma.j.issn.1001-0939.2020.0016
22. Peng YD, Meng K, Guan HQ, Leng L, Zhu RR, Wang BY, et al. Clinical characteristics and outcomes of 112 cardiovascular disease patients infected by 2019-nCoV. Zhonghua Xin Xue Guan Bing Za Zhi. (2020) 48:E004. doi: 10.3760/cma.j.cn112148-20200220-00105
23. Simonnet A, Chetboun M, Poissy J, Raverdy V, Noulette J, Duhamel A, et al. High prevalence of obesity in severe acute respiratory syndrome coronavirus-2 (SARS-CoV-2) requiring invasive mechanical ventilation. Obesity (Silver Spring). (2020) 28:1195–9. doi: 10.1002/oby.22831
24. Argenziano MG, Bruce SL, Slater CL, Tiao JR, Baldwin MR, Barr RG, et al. Characterization and clinical course of 1000 Patients with COVID-19 in New York: retrospective case series. medRxiv. (2020). doi: 10.1101/2020.04.20.20072116
25. Bello-Chavolla OY, Bahena-Lopez JP, Antonio-Villa NE, Vargas-Vázquez A, González-Díaz A, Márquez-Salinas A, et al. Predicting mortality due to SARS-CoV-2: A mechanistic score relating obesity and diabetes to COVID-19 outcomes in Mexico. medRxiv. (2020). doi: 10.1101/2020.04.20.20072223
26. Petrilli CM, Jones SA, Yang J, Rajagopalan H, O'Donnell LF, Chernyak Y, et al. Factors associated with hospitalization and critical illness among 4,103 patients with COVID-19 disease in New York City. medRxiv. (2020). doi: 10.1101/2020.04.08.20057794
27. Malavazos AE, Corsi Romanelli MM, Bandera F, Iacobellis G. Targeting the adipose tissue in COVID-19. Obesity. (2020) 28:1178–9. doi: 10.1002/oby.22844
28. Ryan PMD, Caplice NM. Is adipose tissue a reservoir for viral spread, immune activation, and cytokine amplification in coronavirus disease 2019? Obesity (Silver Spring). (2020) 28:1191–4. doi: 10.1002/oby.22843
29. Garg S, Kim L, Whitaker M, O'Halloran A, Cummings C, Holstein R, et al. Hospitalization rates and characteristics of patients hospitalized with laboratory-confirmed coronavirus disease 2019—Covid-net, 14 states, March 1–30, 2020. Morb Mortal Wkly Rep. (2020) 69:458–64. doi: 10.15585/mmwr.mm6915e3
30. Richardson S, Hirsch JS, Narasimhan M, Crawford JM, McGinn T, Davidson KW, et al. Presenting characteristics, comorbidities, and outcomes among 5700 patients hospitalized with COVID-19 in the New York City area. JAMA. (2020) 323:2052–9. doi: 10.1001/jama.2020.6775
31. Centers for Disease Control and Prevention. COVIDView. A Wkly Surveill Summ US COVID-19 Act. (2020). Available online at: https://www.cdc.gov/coronavirus/2019-ncov/covid-data/covidview/index.html (accessed May 9, 2020).
32. Hussain A, Vasas P, El-Hasani S. Obesity as a risk factor for greater severity of COVID-19 in patients with metabolic associated fatty liver disease. Metabolism. (2020) 108:154256. doi: 10.1016/j.metabol.2020.154256
33. Frydrych LM, Bian G, O'Lone DE, Ward PA, Delano MJ. Obesity and type 2 diabetes mellitus drive immune dysfunction, infection development, and sepsis mortality. J Leukoc Biol. (2018) 104:525–34. doi: 10.1002/JLB.5VMR0118-021RR
34. Giamarellos-Bourboulis EJ, Netea MG, Rovina N, Akinosoglou K, Antoniadou A, Antonakos N, et al. Complex immune dysregulation in COVID-19 patients with severe respiratory failure. Cell Host Microbe. (2020) 27:992–1000.e3. doi: 10.1016/j.chom.2020.04.009
35. McLaughlin T, Ackerman SE, Shen L, Engleman E. Role of innate and adaptive immunity in obesity-associated metabolic disease. J Clin Invest. (2017) 127:5–13. doi: 10.1172/JCI88876
36. Wong C, Marwick TH. Obesity cardiomyopathy: pathogenesis and pathophysiology. Nat Clin Pract Cardiovasc Med. (2007) 4:436–43. doi: 10.1038/ncpcardio0943
37. Avogaro A, de Kreutzenberg SV, Fadini GP. Oxidative stress and vascular disease in diabetes: is the dichotomization of insulin signaling still valid? Free Radic Biol Med. (2008) 44:1209–15. doi: 10.1016/j.freeradbiomed.2007.12.021
Keywords: obesity, body mass index, SARS-CoV-2, COVID-19, risk factor
Citation: Peres KC, Riera R, Martimbianco ALC, Ward LS and Cunha LL (2020) Body Mass Index and Prognosis of COVID-19 Infection. A Systematic Review. Front. Endocrinol. 11:562. doi: 10.3389/fendo.2020.00562
Received: 13 May 2020; Accepted: 09 July 2020;
Published: 14 August 2020.
Edited by:
Alexandra Kautzky-Willer, Medical University of Vienna, AustriaReviewed by:
Themistoklis Tzotzas, St. Luke's Hospital, GreeceCopyright © 2020 Peres, Riera, Martimbianco, Ward and Cunha. This is an open-access article distributed under the terms of the Creative Commons Attribution License (CC BY). The use, distribution or reproduction in other forums is permitted, provided the original author(s) and the copyright owner(s) are credited and that the original publication in this journal is cited, in accordance with accepted academic practice. No use, distribution or reproduction is permitted which does not comply with these terms.
*Correspondence: Lucas Leite Cunha, bHVjYXNsZWl0ZWN1bmhhQGdtYWlsLmNvbQ==
Disclaimer: All claims expressed in this article are solely those of the authors and do not necessarily represent those of their affiliated organizations, or those of the publisher, the editors and the reviewers. Any product that may be evaluated in this article or claim that may be made by its manufacturer is not guaranteed or endorsed by the publisher.
Research integrity at Frontiers
Learn more about the work of our research integrity team to safeguard the quality of each article we publish.