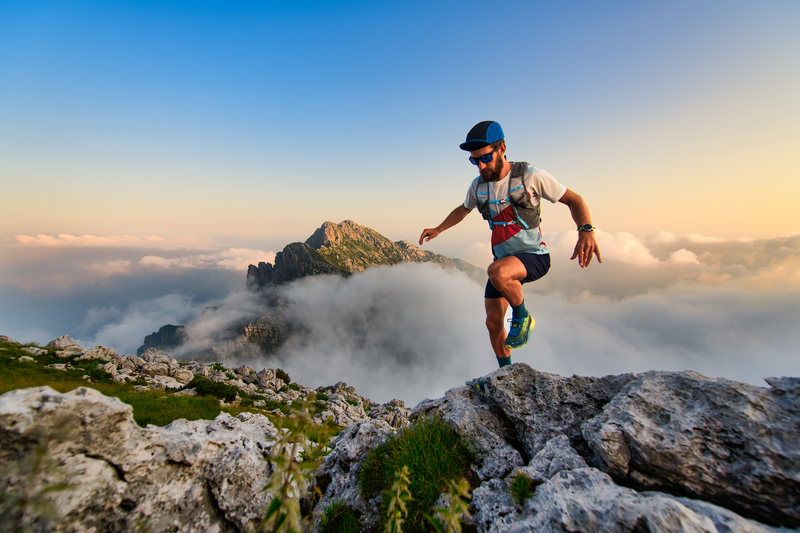
95% of researchers rate our articles as excellent or good
Learn more about the work of our research integrity team to safeguard the quality of each article we publish.
Find out more
ORIGINAL RESEARCH article
Front. Endocrinol. , 19 September 2013
Sec. Clinical Diabetes
Volume 4 - 2013 | https://doi.org/10.3389/fendo.2013.00121
This article is part of the Research Topic Lifestyles: The cornerstone of diabetes treatment View all 8 articles
This study tested the hypothesis that an 8-week exercise intervention supported by mobile health (mHealth) technology would improve metabolic syndrome (MetS) risk factors and heart rate variability (HRV) in a population with MetS risk factors. Participants (n = 12; three male; aged 56.9 ± 7.0 years) reported to the laboratory for assessment of MetS risk factors and fitness (VO2max) at baseline (V0) and after 8-weeks (V2) of intervention. Participants received an individualized exercise prescription and a mHealth technology kit for remote monitoring of blood pressure (BP), blood glucose, physical activity, and body weight via smartphone. Participants underwent 24-h ambulatory monitoring of R–R intervals following V0 and V2. Low and high frequency powers of HRV were assessed from the recording and the ratio of low-to-high frequency powers and low and high frequency powers in normalized units were calculated. One-way repeated measures analysis of variance showed that waist circumference (V0: 113.1 ± 11.0 cm, V2: 108.1 ± 14.7 cm; p = 0.004) and diastolic BP (V0: 81 ± 6 mmHg, V2: 76 ± 11 mmHg; p = 0.04) were reduced and VO2max increased (V0: 31.3 ml/kg/min, V2: 34.8 ml/kg/min; p = 0.02) with no changes in other MetS risk factors. Low and high frequency powers in normalized units were reduced (V0: 75.5 ± 12.0, V2: 72.0 ± 12.1; p = 0.03) and increased (V0: 24.5 ± 12.0, V2: 28.0 ± 12.1; p = 0.03), respectively, with no other changes in HRV. Over the intervention period, changes in systolic BP were correlated negatively with the changes in R–R interval (r = −0.600; p = 0.04) and positively with the changes in heart rate (r = 0.611; p = 0.03), with no other associations between MetS risk factors and HRV parameters. Thus, this 8-week mHealth supported exercise intervention improved MetS risk factors and HRV parameters, but only changes in systolic BP were associated with improved autonomic function.
Cardiovascular diseases are the leading cause of death world-wide accounting for 48% of mortality from non-communicable diseases (1). Type 2 diabetes mellitus is an independent risk factor for cardiovascular diseases and cardiovascular complications are common in this patient population. Metabolic syndrome (MetS) is a clustering of risk factors including abdominal obesity, hypertension, dysglycemia, and dyslipidemia, which doubles the 5-year risk of developing cardiovascular diseases and increases the life-time risk of developing type 2 diabetes mellitus fivefold (2). Lifestyle interventions are currently recommended as first-line therapy for MetS (2, 3).
Heart rate variability (HRV) is a simple, non-invasive measure that can be used to quantify autonomic nervous system modulation (4). Diminished HRV predicts all cause and cardiovascular mortality (5–8) and impaired autonomic function may be especially dangerous for those with an already increased risk of developing cardiovascular disease. Patients with type 2 diabetes mellitus with low HRV have double the risk of mortality compared to those with normal HRV (5). Recent reviews have suggested that autonomic dysfunction may be involved in the development of MetS and further dysfunction may be associated with progression along the continuum of cardiovascular disease (9, 10). Cross-sectional analyses have shown that 24 h HRV is reduced in MetS populations (11, 12), which may further increase cardiovascular risk. There is evidence that lifestyle changes may have positive autonomic effects (13, 14) but, it is unknown whether changes in MetS risk factors with lifestyle modifications are associated with concomitant changes in HRV.
We recently completed a pilot study examining the effects of a prescriptive exercise intervention supported by mobile health (mHealth) technology to improve MetS risk factors and clinical markers of cardiovascular disease (15, 16). This paper reports on a subset of participants with sufficient data for assessment of HRV in a population presenting with MetS risk factors. Thus, the purpose was to test the hypothesis that an 8-week lifestyle intervention supported by mHealth technology would improve HRV and that changes in MetS risk factors would be related to changes in HRV parameters.
Twenty-five participants volunteered and provided informed consent to participate in this study. Participants were included if they had at least two MetS risk factors according to ATPIII guidelines: waist circumference ≥88 cm (women) or 102 cm (men); resting systolic blood pressure (SBP) ≥135 mmHg and/or diastolic blood pressure (DBP) ≥85 mmHg; fasting plasma glucose ≥6.1 mmol/L; triglycerides ≥1.7 mmol/L; and high density lipoprotein cholesterol (HDL) ≤1.03 mmol/L (men) or 1.29 mmol/L (women) [National Cholesterol Education Program (NCEP) Expert Panel on Detection, Evaluation, and Treatment of High Blood Cholesterol in Adults (Adult Treatment Panel III) (17)]. Exclusion criteria were SBP > 180 mmHg and/or DBP > 110 mmHg; type 1 diabetes; history of myocardial infarction, angioplasty, coronary artery bypass, or cerebrovascular ischemia/stroke; symptomatic congestive heart failure; atrial flutter; unstable angina; unstable pulmonary disease; use of medications known to affect heart rate (HR) (such as beta blockers); second or third degree heart block; history of alcoholism, drug abuse, or other emotional cognitive or psychiatric problems; pacemaker; unstable metabolic disease; and orthopedic or rheumatologic problems that could impair the ability to exercise. One participant withdrew from the study shortly following baseline testing due to hospitalization for a respiratory illness unrelated to the study. Twenty-four participants (aged 56.6 ± 9.0 year; six male) reported to Gateway Rural Health Research Centre at baseline (V0) and after four (V1) and eight (V2) weeks of intervention. This study was approved by Institutional Review Board Services (Aurora, ON, Canada; #RP-2008).
At each visit, blood pressure (BP) was measured following a 5-min rest in the seated position with an automated BP cuff (BPTru™ VSM MedTech Ltd., Coquitlam, BC, Canada). Clinic BP was calculated as the average of the last two of three measures taken at 2-min intervals. Waist circumference was measured as the midpoint between the lower rib and iliac crest (cm) (18). Blood was drawn from the antecubital vein and samples were sent to a central processing lab (Gamma Dynacare, London, ON, Canada) for analysis of fasting plasma glucose, triglycerides, and HDL.
The Step Test Exercise Prescription (STEP™) was administered to estimate fitness (VO2max; ml/kg/min) and counsel participants regarding physical activity. The full protocol is reported elsewhere (19). Briefly, participants were instructed to step up and down a set of 2 steps 20 times at a comfortable pace. HR was measured immediately following the test by palpation of the radial artery and input into the equation for calculation of predicted VO2max. Exercise prescription followed American College of Sports Medicine guidelines (20), with a target exercise HR of 70–85% of age predicted maximum HR, depending on VO2max. Goals included increasing pedometer-monitored steps per day with the overall goal of achieving 10,000 steps per day (21). The exercise prescription and goals were updated at V1.
Details of mHealth biometric and activity monitoring and database security are reported elsewhere (15). Briefly, participants received a smartphone (Blackberry® Curve 8300, Research in Motion, Waterloo, ON, Canada) equipped with health monitoring software (Healthanywhere™, IgeaCare Inc., Markham, ON, Canada), a Bluetooth™ enabled BP monitor (A&D Medical, UA-767PBT, San Jose, CA, USA), a glucometer (LifeScan OneTouch Ultra2™, Milpitas, CA, USA, with wireless Bluetooth™ adapter Polymap, PWR-08-03, Tucson, AZ, USA), and a pedometer (Omron, HJ-150, Kyoto, Japan). Blood glucose measures were to be submitted twice daily (fasted upon waking and non-fasted before bed), BP measures were to be submitted three times per week upon waking, pedometer steps were to be input nightly, and body weight input weekly. Real-time measurements were sent to a secure central database that was monitored regularly by researchers. Readings that were outside of pre-set limits triggered alarms that automatically sent a message to the study physician’s smartphone to follow up with the participant.
The participants underwent ambulatory 24-h recording of R–R intervals on their least active day during the week immediately following V0 and V2 visits. R–R interval recording was conducted using a HR monitor with sampling accuracy of 1 ms (Suunto Memory Belt, Vantaa, Finland). Data were downloaded from the monitor to a personal computer for subsequent analysis (Heart Signal Co, Oulu, Finland). Frequency domain analysis for 24-h HRV was calculated as the average of 1 h epochs. An autoregressive model (order 20) was used to estimate power spectrum density for low (0.04–0.15 Hz) and high frequency powers (0.15–0.4 Hz) (4). Low and high frequency powers were also expressed in normalized units by dividing the low and high frequency power, respectively, by the sum of the low and high frequency power and multiplying by 100. Recordings with <80% qualifying beats were excluded from analysis.
SigmaPlot (Version 11.0, Systat Software, San Jose, CA, USA) was used for analysis. One-way repeated measures analysis of variance was used to examine changes in MetS risk factors and HRV parameters from V0 to V2. Normality was tested with the Shapiro–Wilks test. Low and high frequency powers of HRV were logarithmically transformed to achieve normal distribution. Spearman rank order correlation was used to examine correlation between change in MetS risk factors and change in HRV over the course of the intervention. Spearman rank order correlation was used since mean change was not normally distributed and since the sample size was small. Data are shown as mean ± SD and significance was set at p < 0.05.
Participant characteristics, clinic, and home-monitoring results for the full population have been reported elsewhere (15, 16). Twelve participants (aged 44–69 year) had acceptable 24 h HRV recordings at both V0 and V2. Baseline characteristics for this subgroup and changes in MetS risk factors are reported in Table 1. Similar to the full population, this subgroup showed reductions in waist circumference (V0: 113.1 ± 11.0 cm, V2: 108.1 ± 14.7 cm; p = 0.004) and DBP (V0: 81 ± 6 mmHg, V2: 76 ± 11 mmHg; p = 0.04) with no changes in SBP, fasting plasma glucose, triglycerides, or HDL. Additionally, VO2max increased from 31.3 ml/kg/min at V0 to 34.8 ml/kg/min at V2 (p = 0.02). The average of pedometer steps per day entered during week 1 and 8 were calculated to determine physical activity at V0 and V2, respectively. Over the intervention period, daily steps increased from 6472 ± 1997 steps per day at V0 to 8376 ± 2285 steps per day at V2.
High frequency power in normalized units increased from 24.5 ± 12.0 at V0 to 28.0 ± 12.1 at V2 (p = 0.03) and low frequency power in normalized units decreased from 75.5 ± 12.0 at V0 to 72.0 ± 12.1 at V2 (p = 0.03). There were no changes in other 24 h HRV variables (Table 2).
Correlation analysis showed that the change in SBP was inversely associated with the change in R–R interval (−0.600, p = 0.04) and positively associated with the change in HR (0.611, p = 0.03). There were no other relationships between changes in MetS risk factors and changes in HRV parameters (Table 3).
Table 3. Correlation coefficients for changes in heart rate variability parameters and metabolic syndrome risk factors over the intervention period.
The main finding of this study was that a prescriptive exercise intervention supported by mHealth technology improved HRV by increasing and decreasing high and low frequency powers in normalized units, respectively. Additionally, the change in SBP was correlated with the change in R–R interval and HR.
Frequency domain analysis of HRV is commonly used since the different frequency bands are believed to reflect physiological processes (4). Specifically, it is generally accepted that the high frequency band reflects parasympathetic modulation, though there is some debate over the physiological significance of the low frequency band (4). The low frequency band may represent a combination of sympathetic and parasympathetic activity (4) or may be reflective of baroreflex activity (22, 23). There is evidence to suggest that when low frequency power is expressed in normalized units, it is reflective of sympathetic activity (24). Thus, the increase in high frequency power and decrease in low frequency power expressed in normalized units may indicate positive modifications in autonomic activity with an increase in parasympathetic and decrease in sympathetic activity following the intervention.
Exercise training is recommended as first-line treatment for MetS (2, 3) and has proved to effectively improve waist circumference, BP, and HDL in MetS populations (25). Additionally, lifestyle interventions combining diet and exercise reduced incident type 2 diabetes mellitus in a population of adults with MetS risk factors (26, 27). In the DaTA pilot study, the 8-week mHealth supported exercise intervention reduced DBP and total cholesterol and increased predicted VO2max (16). There is some evidence to suggest that exercise may also have positive effects on HRV, but results are mixed. In an early study, young adults with mild hypertension completed 22 min of calisthenics followed by 20 min of jogging a minimum of five times per week (28). Upon completion of the intervention, resting R–R interval was increased and, similar to the present study, high and low frequency powers expressed in normalized units were increased and reduced, respectively, demonstrating improved autonomic balance (28). Exercise training interventions, of moderate-to-vigorous intensity in middle-aged to older men (13, 29, 30) and a lower intensity exercise intervention at 50% of VO2max in post-menopausal women also showed improvements in HRV (31). One study in patients with type 2 diabetes mellitus with and without cardiac autonomic neuropathy showed improvement in HRV parameters following 6 months of aerobic exercise three times per week at 70–85% of HR reserve (32). Conversely, one study showed no change in HRV parameters at rest or during exercise in older men and women after 8 weeks of aerobic exercise training three times per week for 60 min each session (33).
Other studies found that while resting HRV was not altered with endurance training, HRV was altered during or after exposure to stressors (34, 35). In obese women with and without type 2 diabetes mellitus, 16 weeks of moderate intensity endurance training (65% VO2max) 4 days per week increased post-exercise HR recovery and post-exercise high and low frequency powers with no changes in resting HRV (34). Six months of exercise training 2 days per week for 70 min at moderate intensity did not affect resting HRV in patients with type 2 diabetes mellitus, but high frequency power in normalized units was increased and the ratio of low-to-high frequency powers was reduced during an orthostatic challenge (35). Thus, inclusion of an acute stressor to the study protocol may have revealed additional alterations in autonomic activity following the intervention.
Frequency of exercise training may be an important factor, as those with sessions five to six times per week (13, 28, 30) showed improved HRV, while in those with training sessions four times per week or less, results were mixed. In four studies that did not show changes in resting autonomic function, exercise training frequency was only two or three times per week or only one session per week was supervised and the remaining were completed at home, so compliance to the exercise protocol may not be as high as reported. Since the exercise prescription in the present study included attainment of 10,000 steps daily along with other more specific aerobic exercises at moderate-to-vigorous intensity, the frequent exercise bouts may have been responsible for the improvements in high and low frequency powers in normalized units. Unfortunately, only pedometer steps were recorded and other activities were not logged, so frequency and intensity of exercise completed throughout the intervention cannot be quantified.
Age may play an important role in the response of HRV to exercise. Lee and colleagues (36) used low dose atropine (which augments parasympathetic activity, as opposed to higher doses, which are inhibitory) to provoke autonomic changes in younger and older fit and unfit individuals. Resting R–R interval was increased more in fit compared to unfit individuals in the younger age group. However, the responses to atropine in the older age group were not affected by fitness status (36). Thus the lack of change in HRV in response to exercise training in older adults may be due to reduced function or sensitivity of the sinus node with age, which may not be modified with intervention. One study in older women showed that exercise improved HRV when 50, 100, or 150% of national exercise guidelines was completed. However, in women aged >60 year, there were no improvements at lower exercise doses, suggesting that a higher dose of exercise may be necessary to evoke autonomic changes in older adults (37). Though our sample was on average older, it included a range of ages. Interestingly, age was not correlated with change in any HRV parameter in this study.
In the Diabetes Prevention Program, an intensive lifestyle intervention including physical activity and a low fat diet reduced HR and increased HRV parameters indicative of overall or beat-by-beat variability (reflecting parasympathetic activity) compared to metformin or placebo (38). Importantly, reductions in HR and increases in HRV over time were associated with lower risk of incident diabetes, independent of weight loss, and physical activity in the lifestyle modification group, supporting the use of HRV or perhaps the change in HRV in response to an intervention, as a risk indicator (38). HRV was measured from 10 s ECG segments, so frequency domain parameters could not be examined, which also have important prognostic power. Nevertheless, these results are promising and suggest that lifestyle modifications are important for risk reduction in a diabetes prevention strategy. Improvements in autonomic function may have positive effects in the long-term. Longitudinal follow-up studies are warranted to examine the effects of improved HRV on prognosis.
To our knowledge, this study was the first to examine correlation between the change in HRV parameters and the change in MetS risk factors over an intervention period. The change in SBP was strongly and inversely associated with changes in R–R interval and positively associated with changes in HR. These findings are in accordance with previous studies that have shown reduced BP and HR with endurance exercise training (39). The correlations between changes MetS risk factors and changes in HRV parameters over an intervention period are not the same as cross-sectional associations between 24 h HRV and MetS risk factors. Assoumou et al. (11) showed associations between hypertension and total power, but did not examine relationships with SBP and DBP individually. Their study showed a negative association between fasting plasma glucose and high frequency power of HRV (11), while the present study showed no associations between fasting plasma glucose and HRV parameters. When sex differences were examined triglycerides were only associated with HRV in men, but not women and fasting plasma glucose was negatively associated with high frequency power and positively associated with ratio of low-to-high frequency powers in women with no associations in men (11). In a cohort of men with MetS, all MetS risk factors except HDL were associated with HRV parameters (12). Notably, these authors showed that each one unit increment in the number of MetS components reduced low frequency power of HRV by 15% (12), highlighting the importance of treating and controlling all MetS components. Liao and colleagues (40) examined 2-min HRV in people with what they termed multiple MetS, which could be any combination of hypertension, type 2 diabetes mellitus, and dyslipidemia. Interestingly, they showed that the combination of risk factors presenting together determined the HRV outcome. When hypertension was present in combination with either type 2 diabetes mellitus or dyslipidemia, the reductions in HRV were additive, but when type 2 diabetes mellitus and dyslipidemia presented together, reductions in HRV were multiplicative (40). These findings may account for differences between the present study and others, as specific combinations of risk factors may be more important contributors to HRV than individual MetS components. The sample size for this pilot project was too small to include an analysis based on the change in MetS risk factors, but such analyses are important for future studies. Additionally, the present study was the first to examine associations between longitudinal changes in HRV and changes in MetS, which may be different than cross-sectional associations.
In this study, changes in waist circumference were not significantly correlated with changes in HRV. There was however, a strong trend (p = 0.06) for a negative association between the change in waist circumference and the change in high frequency power of HRV, suggesting that reductions in waist circumference may not be associated with improvements in HRV, but rather with declines in HRV. On the contrary, Hautala and colleagues (13) showed that an 8-week intervention with supervised exercise training sessions six times per week at 70–80% of maximum HR improved HRV. The change in body mass index was moderately correlated with the change in high frequency power following the subsequent 10-week home-based maintenance program, despite the fact that overall improvements in HRV were not maintained during this period (13). Furthermore, in a small sample of abdominally obese men and women, weight loss with a very low calorie diet increased total and low frequency power of HRV (41). However, despite weight maintenance over a 1-year period, improvements in HRV were not sustained (41). These studies suggest that improvements in HRV with lifestyle interventions may be transient. Long-term studies to determine effective strategies for maintenance of HRV improvements with exercise or weight loss interventions are needed.
This pilot study was conducted in a sample of highly motivated volunteers and may therefore lack external validation. Despite the fact that only 12 participants had acceptable HR data for 24 h HRV analysis, modifications were seen in high and low frequency powers of HRV when expressed in normalized units. However, this analysis was underpowered and a larger sample size would have been ideal and may have shown greater change. Although Suunto activity monitor has been validated, our participants had difficulties putting the monitor on properly to collect heart period. Additionally, some participants reported discomfort and removed the monitor during the nighttime period due to disturbed sleep. Traditional Holter monitors may be preferable to improve data quality and participant comfort. This exploratory pilot study used correlation analysis to examine relationships between MetS risk factors and HRV parameters. Unfortunately, correlation cannot show direction of association. Additionally, previous studies have shown sex differences in associations between MetS risk factors and HRV (11), but the sample for this pilot study was not sufficiently powered to examine sex differences. Nevertheless, potentially important relationships were identified in this sample. Future studies examining relationships and sex differences in a larger population are warranted.
In conclusion, an 8-week exercise prescription supported by mHealth technologies for biometric and activity monitoring improved 24 h HRV profile with increased high frequency power of HRV in normalized units and reduced low frequency power of HRV in normalized units, suggesting improved autonomic balance. Additionally, changes in SBP were associated with changes in R–R interval and HR.
The authors declare that the research was conducted in the absence of any commercial or financial relationships that could be construed as a potential conflict of interest.
This pilot project was supported by Sykes Assistance Services Corporation and funding from Canadian Institute of Health Research Cardiovascular Complications of Diabetes Team Grant CCT-83029. The authors gratefully acknowledge E. Russell-Minda for study coordination and E. Read for assistance with data collection.
1. Mendis S, Puska P, Norrving B, editors. Global Atlas on Cardiovascular Disease Prevention and Control. Geneva: World Health Organization (2011).
2. Alberti KGMM, Eckel RH, Grundy SM, Zimmet PZ, Cleeman JI, Donato KA, et al. Harmonizing the metabolic syndrome: a joint interim statement of the international diabetes federation task force on epidemiology and prevention; National Heart, Lung, and Blood Institute; American Heart Association; World Heart Federation; International Atherosclerosis Society; and International Association for the Study of Obesity. Circulation (2009) 120(16):1640–5.
3. Cardiometabolic Risk Working Group: Executive Committee, Leiter LA, Fitchett DH, Gilbert RE, Gupta M, Mancini GB, et al. Cardiometabolic risk in Canada: a detailed analysis and position paper by the cardiometabolic risk working group. Can J Cardiol (2011) 27(2):e1–33. doi:10.1016/j.cjca.2010.12.054
4. Heart rate variability: standards of measurement, physiological interpretation and clinical use. Task Force of the European Society of Cardiology and the North American Society of Pacing and Electrophysiology. Circulation (1996) 93(5):1043–65. doi:10.1161/01.CIR.93.5.1043
5. Gerritsen J, Dekker JM, Tenvoorde BJ, Kostense PJ, Heine RJ, Bouter LM, et al. Impaired autonomic function is associated with increased mortality, especially in subjects with diabetes, hypertension, or a history of cardiovascular disease: the Hoorn Study. Diabetes Care (2001) 24(10):1793–8. doi:10.2337/diacare.24.10.1793
6. Kleiger RE, Miller JP, Bigger JT Jr. Decreased heart rate variability and its association with increased mortality after acute myocardial infarction. Am J Cardiol (1987) 59(4):256–62. doi:10.1016/0002-9149(87)90795-8
7. La Rovere MT, Bigger JT Jr, Marcus FI, Mortara A, Schwartz PJ. Baroreflex sensitivity and heart-rate variability in prediction of total cardiac mortality after myocardial infarction. ATRAMI (Autonomic Tone and Reflexes After Myocardial Infarction) investigators. Lancet (1998) 351(9101):478–84. doi:10.1016/S0140-6736(97)11144-8
8. Tsuji H, Larson MG, Venditti FJ Jr, Manders ES, Evans JC, Feldman CL, et al. Impact of reduced heart rate variability on risk for cardiac events. The Framingham Heart Study. Circulation (1996) 94(11):2850–5. doi:10.1161/01.CIR.94.11.2850
9. Tentolouris N, Argyrakopoulou G, Katsilambros N. Perturbed autonomic nervous system function in metabolic syndrome. Neuromolecular Med (2008) 10(3):169–78. doi:10.1007/s12017-008-8022-5
10. Thayer JF, Yamamoto SS, Brosschot JF. The relationship of autonomic imbalance, heart rate variability and cardiovascular disease risk factors. Int J Cardiol (2010) 141(2):122–31. doi:10.1016/j.ijcard.2009.09.543
11. Assoumou HGN, Pichot V, Barthelemy JC, Dauphinot V, Celle S, Gosse P, et al. Metabolic syndrome and short-term and long-term heart rate variability in elderly free of clinical cardiovascular disease: the PROOF study. Rejuvenation Res (2010) 13(6):653–63. doi:10.1089/rej.2010.1019
12. Gehi AK, Lampert R, Veledar E, Lee F, Goldberg J, Jones L, et al. A twin study of metabolic syndrome and autonomic tone. J Cardiovasc Electrophysiol (2009) 20(4):422–8. doi:10.1111/j.1540-8167.2008.01363.x
13. Hautala AJ, Mäkikallio TH, Kiviniemi A, Laukkanen RT, Nissilä S, Huikuri HV, et al. Heart rate dynamics after controlled training followed by a home-based exercise program. Eur J Appl Physiol (2004) 92(3):289–97. doi:10.1007/s00421-004-1077-6
14. Madden KM, Levy WC, Stratton JR. Exercise training and heart rate variability in older adult female subjects. Clin Invest Med (2006) 29(1):20–8.
15. Stuckey M, Fulkerson R, Read E, Russell-Minda E, Munoz C, Kleinstiver P, et al. Remote monitoring technologies for the prevention of metabolic syndrome: the Diabetes and Technology for Increased Activity (DaTA) study. J Diabetes Sci Technol (2011) 5(4):936–44.
16. Stuckey M, Russell-Minda E, Read E, Munoz C, Shoemaker K, Kleinstiver P, et al. Diabetes and Technology for Increased Activity (DaTA) study: results of a remote monitoring intervention for prevention of metabolic syndrome. J Diabetes Sci Technol (2011) 5(4):928–35.
17. National Cholesterol Education Program (NCEP) Expert Panel on Detection, Evaluation, and Treatment of High Blood Cholesterol in Adults (Adult Treatment Panel III). Third Report of the National Cholesterol Education Program (NCEP) Expert Panel on Detection, Evaluation, and Treatment of High Blood Cholesterol in Adults (Adult Treatment Panel III) final report. Circulation (2002) 106(25):3143–421.
18. Alberti KG, Zimmet P, Shaw J, IDF Epidemiology Task Force Consensus Group. The metabolic syndrome – a new worldwide definition. Lancet (2005) 366(9491):1059–62. doi:10.1016/S0140-6736(05)67402-8
19. Stuckey MI, Knight E, Petrella RJ. The step test and exercise prescription tool in primary care: a critical review. Crit Rev Phys Rehabil Med (2012) 24(1–2):109–23. doi:10.1615/CritRevPhysRehabilMed.2013006823
20. Haskell WL, Lee I, Pate RR, Powell KE, Blair SN, Franklin BA, et al. Physical activity and public health: updated recommendation for adults from the American College of Sports Medicine and the American Heart Association. Circulation (2007) 116(9):1081–93. doi:10.1161/CIRCULATIONAHA.107.185649
21. Tudor-Locke C, Bassett DR Jr. How many steps/day are enough? Preliminary pedometer indices for public health. Sports Med (2004) 34(1):1–8. doi:10.2165/00007256-200434010-00001
22. Moak JP, Goldstein DS, Eldadah BA, Saleem A, Holmes C, Pechnik S, et al. Supine low-frequency power of heart rate variability reflects baroreflex function, not cardiac sympathetic innervation. Heart Rhythm (2007) 4(12):1523–9. doi:10.1016/j.hrthm.2007.07.019
23. Rahman F, Pechnik S, Gross D, Sewell L, Goldstein DS. Low frequency power of heart rate variability reflects baroreflex function, not cardiac sympathetic innervation. Clin Auton Res (2011) 21(3):133–41. doi:10.1007/s10286-010-0098-y
24. Pagani M, Montano N, Porta A, Malliani A, Abboud FM, Birkett C, et al. Relationship between spectral components of cardiovascular variabilities and direct measures of muscle sympathetic nerve activity in humans. Circulation (1997) 95(6):1441–8. doi:10.1161/01.CIR.95.6.1441
25. Pattyn N, Cornelissen VA, Eshghi SRT, Vanhees L. The effect of exercise on the cardiovascular risk factors constituting the metabolic syndrome: a meta-analysis of controlled trials. Sports Med (2013) 43(2):121–33. doi:10.1007/s40279-012-0003-z
26. Knowler WC, Barrett-Connor E, Fowler SE, Hamman RF, Lachin JM, Walker EA, et al. Reduction in the incidence of type 2 diabetes with lifestyle intervention or metformin. N Engl J Med (2002) 346(6):393–403. doi:10.1056/NEJMoa012512
27. Lindstrom J, Ilanne-Parikka P, Peltonen M, Aunola S, Eriksson JG, Hemio K, et al. Sustained reduction in the incidence of type 2 diabetes by lifestyle intervention: follow-up of the Finnish Diabetes Prevention Study. Lancet (2006) 368(9548):1673–9. doi:10.1016/S0140-6736(06)69701-8
28. Pagani M, Somers V, Furlan R, Dell’Orto S, Conway J, Baselli G, et al. Changes in autonomic regulation induced by physical training in mild hypertension. Hypertension (1988) 12(6):600–10. doi:10.1161/01.HYP.12.6.600
29. Seals DR, Chase PB. Influence of physical training on heart rate variability and baroreflex circulatory control. J Appl Physiol (1989) 66(4):1886–95.
30. Tulppo MP, Hautala AJ, Mäkikallio TH, Laukkanen RT, Nissilä S, Hughson RL, et al. Effects of aerobic training on heart rate dynamics in sedentary subjects. J Appl Physiol (2003) 95(1):364–72.
31. Jurca R, Church TS, Morss GM, Jordan AN, Earnest CP. Eight weeks of moderate-intensity exercise training increases heart rate variability in sedentary postmenopausal women. Am Heart J (2004) 147(5):G1–8. doi:10.1016/j.ahj.2003.10.024
32. Pagkalos M, Koutlianos N, Kouidi E, Pagkalos E, Mandroukas K, Deligiannis A. Heart rate variability modifications following exercise training in type 2 diabetic patients with definite cardiac autonomic neuropathy. Br J Sports Med (2008) 42(1):47–54. doi:10.1136/bjsm.2007.035303
33. Perini R, Fisher N, Veicsteinas A, Pendergast DR. Aerobic training and cardiovascular responses at rest and during exercise in older men and women. Med Sci Sports Exerc (2002) 34(4):700–8. doi:10.1097/00005768-200204000-00022
34. Figueroa A, Baynard T, Fernhall B, Carhart R, Kanaley JA. Endurance training improves post-exercise cardiac autonomic modulation in obese women with and without type 2 diabetes. Eur J Appl Physiol (2007) 100(4):437–44. doi:10.1007/s00421-007-0446-3
35. Zoppini G, Cacciatori V, Gemma ML, Moghetti P, Targher G, Zamboni C, et al. Effect of moderate aerobic exercise on sympatho-vagal balance in type 2 diabetic patients. Diabet Med (2007) 24(4):370–6. doi:10.1111/j.1464-5491.2007.02076.x
36. Lee K, Picard G, Beske SD, Hwang GS, Taylor JA. Effects of fitness and age on the response to vagotonic atropine. Auton Neurosci (2008) 139(1–2):60–7. doi:10.1016/j.autneu.2008.01.007
37. Earnest CP, Blair SN, Church TS. Heart rate variability and exercise in aging women. J Womens Health (2012) 21(3):334–9. doi:10.1089/jwh.2011.2932
38. Carnethon MR, Prineas RJ, Temprosa M, Zhang ZM, Uwaifo G, Molitch ME, et al. The association among autonomic nervous system function, incident diabetes, and intervention arm in the Diabetes Prevention Program. Diabetes Care (2006) 29(4):914–9. doi:10.2337/diacare.29.04.06.dc05-1729
39. Cornelissen VA, Verheyden B, Aubert AE, Fagard RH. Effects of aerobic training intensity on resting, exercise and post-exercise blood pressure, heart rate and heart-rate variability. J Hum Hypertens (2010) 24(3):175–82. doi:10.1038/jhh.2009.51
40. Liao D, Sloan RP, Cascio WE, Folsom AR, Liese AD, Evans GW, et al. Multiple metabolic syndrome is associated with lower heart rate variability: the Atherosclerosis Risk in Communities Study. Diabetes Care (1998) 21(12):2116–22. doi:10.2337/diacare.21.12.2116
Keywords: autonomic nervous system, exercise training, metabolic syndrome, mobile health, heart rate variability
Citation: Stuckey MI, Kiviniemi AM and Petrella RJ (2013) Diabetes and technology for increased activity study: the effects of exercise and technology on heart rate variability and metabolic syndrome risk factors. Front. Endocrinol. 4:121. doi: 10.3389/fendo.2013.00121
Received: 20 June 2013; Paper pending published: 06 August 2013;
Accepted: 28 August 2013; Published online: 19 September 2013.
Edited by:
Rhonda C. Bell, University of Alberta, CanadaReviewed by:
Julianne Toohey, University of California, USACopyright: © 2013 Stuckey, Kiviniemi and Petrella. This is an open-access article distributed under the terms of the Creative Commons Attribution License (CC BY). The use, distribution or reproduction in other forums is permitted, provided the original author(s) or licensor are credited and that the original publication in this journal is cited, in accordance with accepted academic practice. No use, distribution or reproduction is permitted which does not comply with these terms.
*Correspondence: Robert J. Petrella, Aging, Rehabilitation and Geriatric Care Research Centre, Lawson Health Research Institute, 801 Commissioners Road East, Suite B3002, London, ON N6C 5J1, Canada e-mail:cGV0cmVsbGFAdXdvLmNh
Disclaimer: All claims expressed in this article are solely those of the authors and do not necessarily represent those of their affiliated organizations, or those of the publisher, the editors and the reviewers. Any product that may be evaluated in this article or claim that may be made by its manufacturer is not guaranteed or endorsed by the publisher.
Research integrity at Frontiers
Learn more about the work of our research integrity team to safeguard the quality of each article we publish.