- 1Business College, Qingdao University, Qingdao, Shandong, China
- 2School of European Language and Culture Studies, Dalian University of Foreign Languages, Dalian, China
Introduction: The coupling and coordination development level (CCDL) of science and technology innovation (STI) between universities and enterprises is a key determinant of the effectiveness of the national STI system.
Methods: This study utilized data from universities and industrial enterprises spanning from 2012 to 2021 to establish an indicator system for measuring university STI ability and enterprise STI ability. The study then applied the entropy TOPSIS method to calculate the STI scores and further analyzed them using a coupling coordination model. Various research methods were employed to quantify the spatiotemporal characteristics and elucidate the patterns of evolution, with the grey relational analysis model being utilized to examine the mutual influence at the micro-index level.
Results and discussion: The study found a significant transformation in the collaboration between universities and enterprises in China, shifting from extreme incoherence to highly coordinated cooperation. Secondly, the reason for the differences is the uneven development in the four regions. Thirdly, the CCDL of STI between universities and enterprises in each region is notably different, but all show σ convergence trend. Finally, the university STI ability and enterprise STI ability are identified as key factors in the CCDL of STI between universities and enterprises.
1 Introduction
The spatial structure of China’s economic development is currently undergoing a period of significant transformation, with the overall situation of coordinated development across regions showing signs of improvement. However, it is becoming increasingly evident that new situations and problems are emerging, such as a noticeable differentiation in regional economic development, an increasingly prominent polarization of development momentum, and some regions facing considerable challenges in their development (Chinese Academy of Science and Technology for Development, 2023). In the critical period of economic development transformation, STI has become the basic idea guiding China to promote high-quality development of productivity. Taking collaborative innovation of science and technology as the foundation of regional coordinated development, relying on major regional strategies, and improving the spatial layout of regional technological innovation, it is an important guarantee for narrowing the regional development gap, breaking the urban-rural dual structure, and ensuring that developed and underdeveloped regions achieve common prosperity together in China (Chen, 2024).
Given the above considerations, this study selects university and enterprise STI ability as the research subjects, to conduct a comprehensive investigation into the regional differences, spatial-temporal characteristics, and dynamic progression of the CCDL of STI between universities and enterprises at national and regional levels. Next, this study analyzes the influencing factors of the CCDL of STI between universities and enterprises. The ultimate goal of this study is to provide a scientific basis for improving the CCDL of STI between universities and enterprises in the four regions and at the national level, which is essential to promote the coupling and coordination development of nations and regions, optimize China’s spatial configuration, and deepen the integration of universities and enterprises.
2 Literature review
Universities and enterprises, as the two main bodies of the national STI system, can directly enhance the effectiveness of the STI system of China and the regions. This can facilitate the achievement of STI self-reliance and self-strengthening at higher levels, the domestic and foreign transfer of advanced technology, the transformation and industrialization of technology, and the optimization of China’s regional comparative advantages (Jia et al., 2024). Due to the CCDL of STI between universities and enterprises play a pivotal role in boosting regional STI. Numerous scholars have conducted extensive research on this subject. Yang (2021) proposed that the CCDL of STI between universities and enterprises can facilitate the allocation of productive forces, thereby addressing the challenges of regional development and promoting coordinated development across regions. In a recent study, Jiang et al. (2024) used a technological correlation lens to examine the progress of CCDL of STI between universities and enterprises in China’s four regions, to gain insight into the development and upgrading of new productive forces, to identify a practical way forward. In light of the tenets of evolutionary economic geography, He and Zhibin (2023) posited that the CCDL of STI between universities and enterprises can assist regions in leveraging their intrinsic regional advantages to cultivate an innovation-driven emerging industry system, thereby promoting coordinated development across regions.
In the study targeting the CCDL of STI between universities and enterprises, Wang and Chen (2019) discovered that the level of CCDL of STI between universities and enterprises in most provinces is on the rise, but there are significant regional differences, through empirical analysis of the coupling and coordination relationship between university STI ability and industrial enterprise STI ability across 27 provinces in China. These problems primarily stem from China’s vast size, different regions have certain differences in terms of resource endowment, industrial structure, and social and cultural background, which leads to a significant imbalance in the distribution of science and technology innovation resource endowments across universities and enterprises, and a low level of connectivity (Song G et al., 2024). Secondly, STI in universities is primarily conducted by individual teachers or teams engaged in free-form exploration. The majority of innovations can be classified as basic theoretical research. In contrast, enterprises are required to undertake STI and development based on market demand. This often involves a conflict between the pursuit of short-term solutions by enterprises and the long-term goals of STI (Zheng S. et al., 2024). At the same time, there is a lack of problem-oriented awareness of the coupled and coordinated development of STI between universities and enterprises, which hinders the ability to focus scientific research efforts on key aspects of key tasks and unable to align with the requirements of significant national developmental strategies (Guo, 2023). Furthermore, an issue of misaligned functions and positioning between universities and enterprises, and there is a discernible upward trend (Yun et al., 2023). In addition to the lack of adequate intellectual property protection and sharing mechanisms, there is a lack of willingness to collaborate on STI research between universities and enterprises. This is particularly evident in the sharing, mobility and exchange of various types of large-scale scientific research instruments and equipment, scientific equipment, scientific data, and talents (Han and Zhe, 2023). The existence of these problems has failed to establish the requisite ideological consensus in the practical operation of the CCDL of STI between universities and enterprises. Furthermore, there is a certain degree of confusion in practice, which has made it challenging to form the necessary strategic synergy, thereby impeding the CCDL of STI between universities and enterprises and reducing the efficiency of technological innovation (Li, 2022).
In conclusion, the extant literature has primarily investigated the coupling and coordination relationship and micro-coupling mechanism of university STI ability and enterprise STI ability at the national or regional levels through empirical research or theoretical analysis. However, there is a lack of quantitative analysis on the regional differences, spatial-temporal characteristics, dynamic evolution, and coupling factors of the CCDL of STI between universities and enterprises. In light of the above considerations, this study focuses on the CCDL of STI between universities and enterprises in China. Firstly, the study uses the Dagum Gini coefficient and its decomposition method, the kernel density estimation method and the coefficient of variation to examine the regional differences, spatial-temporal characteristics, and dynamic development in China and the four regions. Second, this study explores the role of micro indicators in understanding the dynamics of the CCDL of STI between universities and enterprises. As a result, the following hypotheses are clarified.
Hypothesis 1. The CCDL of STI between universities and enterprises in China and the four regions shows an upward trend.
Hypothesis 2. The university STI ability and enterprise STI ability are key factors affecting the CCDL of STI between universities and enterprises.
3 Materials and methods
This study refers to the research method and statistical indicator selection scheme of Yu et al. (2024) and presents a version of the measurement index of university STI ability and enterprise STI ability (Tables 1, 2).
Table 1 measures the university STI ability. The technology workforce represents the human capital input of universities, the technology funding represents the capital factor input of universities, and the technological achievements and technology transfer represent the STI output of high-level research universities.
Table 2 measures the enterprise STI ability. The technology workforce assessment of the human capital input of enterprises, the technology funding evaluation of the capital factor input of enterprises. The technological achievements and technology transfer measure the enterprises’ new product development and production as well as technological innovation such as technology acquisition and technological transformation.
In this study, the university STI ability and enterprise STI ability are taken as the subjects of study. Drawing on the research results of Ma and Ma (2024) and Cheng et al. (2024), the study selects universities published by the Ministry of Education, which have perfect information disclosure, and industrial enterprises above the designated size of China Science and Technology Statistical Yearbook as statistical objects. EPS China data are used to compile data on STI indicators for universities and enterprises from 2012 to 2021. The data required in Table 1 are selected from the China Education Database-Annual on the EPS C. data; and the data required in Table 2 are selected from the China High-Tech Industry Database-Annual on the EPS C. data. In the case of incomplete data for individual provinces, the data have been processed using the average growth rate or the average value. In addition, the raw data are processed in a dimensionless manner in order to eliminate the effects of differences in size and shape between the indicators.
In this study, the entropy method can combine the uncertainty between the indicators to calculate the weight of each indicator, which makes the evaluation results more reliable and accurate; and the TOPSIS method can consider the scores of each indicator, which makes the results more comprehensive and objective (Li H et al., 2024). Therefore, this study uses the entropy TOPSIS method to measure the STI ability of universities and enterprises. Drawing on the approach of Yu et al. (2024), the coupling coordination model used calculates the CCDL of STI between universities and enterprises and applies the grey relational analysis model to examine the influence of university STI ability and enterprise STI ability on the CCDL of STI between universities and enterprises. The division of regions according to the national economic zones published by the National Bureau of Statistics (Table 3 for the specific division). The Dagum Gini coefficient and its decomposition were used to analyze intra-regional differences, inter-regional differences, and the contribution Rate of regional differences in each region (Hu C and Ma X, 2023). This study further uses the kernel density estimation method to describe the overall shape and dynamic evolution of the CCDL of STI between universities and enterprises, including the distribution location, peak distribution, distribution ductility, and polarization trend (Zhang X. et al., 2023). To more accurately examine the evolution trajectory and trend characteristics of the differences in CCDL of STI between universities and enterprises, a convergence model is adopted to test the convergence of CCDL of STI between universities and enterprises (Zhang W. et al., 2023).
4 Empirical analysis
The entropy TOPSIS method is applied to measure the CCDL of STI between universities and enterprises from 2012 to 2021. Based on the calculation results, the time sequence variation chart in the level of university STI ability and enterprise STI ability was plotted (Figure 1), to provide a more intuitive response to the trend of changes in the two.
Figure 1 illustrates that the level of university STI ability and enterprise STI ability in China are undergoing a continuous and analogous process of improvement. This indicates that there is a coupling and coordination relationship between the university STI and enterprise STI.
Using the coupling coordination model, the coupling and coordination time sequence of university STI ability and enterprise STI ability was calculated (Table 4).
According to Table 4, the C-value of coupling degree decreases and then increases during the period 2012-2021, and the T-value of coordination degree and the D-value of coupling coordination degree show an increasing trend. In 2012, although the C-value of the coupling degree was 1, the T-value of the coordination degree was only 0.01, the D-value of the coupling coordination degree was only 0.1, and the coupling coordination state was Extreme non-coordination. This phenomenon shows that although there was a strong interaction between university STI ability and enterprise STI ability in 2012, the university STI ability and enterprise STI ability were relatively independent, with very low consistency and effectiveness, and means that the universities and enterprises were in a “fight the enemy separately” in STI activities. There was a lack of effective communication and cooperation between them, forming a low-level strong coupling state.
In 2014, the C-value of coupling degree reached its lowest point. However, both the T-value of coordination degree and the D-value of coupling coordination degree showed an increase. This suggests that the CCDL of STI between universities and enterprises had entered a period of adjustment. Although the intensity of the interaction in STI activities had decreased, but the communication and cooperation abilities had shown signs of improvement. The data suggest that the CCDL of STI between universities and enterprises is gradually converging towards a state of cooperation, marking a significant shift from non-coordination to coordination in 2016. In 2021, the C-value of coupling degree was 1, and the T-value of coordination degree and the D-value of coupling coordination degree were both close to the maximum value of 1, reaching 0.99 and 0.995 respectively. This suggests that after a decade of development, the level of coupling and coordination of STI between universities and enterprises in China has reached a high level of integration. The relationship between universities and enterprises in STI has not only demonstrated robust interaction but also reached a state of high-quality coordinated development. The positive interaction and unified development of universities and enterprises in STI have been gradually realized.
5 Spatial-temporal characteristics and source of CCDL of STI between universities and enterprises in China
5.1 Intra-regional differences of the CCDL of STI between universities and enterprises
The Dagum Gini coefficient was used to calculate the degree of intra-regional differences in the CCDL of STI between universities and enterprises across the nation and in the four major regions (Figure 2).
Nationwide, it can be seen that the overall Gini coefficient of the CCDL of STI between universities and enterprises in China shows a decreasing trend in the sample observation period, which indicates that the uncoordinated and unbalanced phenomenon of the CCDL of STI between universities and enterprises in China and four regions is continuously weakening. It proves that since the 18th National Congress of the CPC, under the guidance of the new development philosophy, China has improved the overall CCDL of STI between universities and enterprises across China and reducing the differences between regions (Ning et al., 2023).
In terms of regional comparison, the Gini coefficients of the four regions all show a decreasing trend, and the average values of the Gini coefficients within the four regions during the sample observation period (0.154, 0.199, 0.1616, and 0.1963 for the Northeast, East, Central, and West regions, respectively) are all lower than the overall Gini coefficient for the whole country (0.210), indicating that the intra-regional differences are not the main source of the differences. The overall differences in the CCDL of STI between universities and enterprises are mainly due to inter-regional differences.
Specifically, the Gini coefficient in the Northeast region was the lowest in the country in 2012, at 0.279, with a clear downward trend between 2012 and 2014, after which it became a fluctuating upward trend, reaching a maximum of 0.261 in 2018 and then falling sharply in 2019, with an average of 0.154 over the sample observation period, the lowest in the country. Since the Strategy of Revitalizing Northeast China achieved phased results from 2003 to 2013, the Gini coefficient showed a downward trend in the early stage. However, in 2014, due to the deep-rooted structural problems formed over a long period of time, the demographic dividend of the northeastern region gradually declined (Sun Y et al., 2023), and with the gradual depletion of resources and the decline of the power of state-owned enterprises (Wang and Chen, 2024), resulting in obvious fluctuations in the Gini coefficient.
The intra-regional difference of the Eastern region shows a clear three-stage development trend: a high Gini coefficient of 0.334 in 2012, which is only lower than that of the central region (0.373), followed by a clear downward trend after 2014, a stable period from 2015 to 2019, and a further decline after 2019. In 2012, the intra-regional difference in the eastern region was only lower than that in the central region, which was 0.373. The reason for this is that although there are more top universities and enterprises in the eastern region, they tend to be spatially concentrated in core cities such as Beijing, Shanghai, and Guangzhou, and the feature of regional aggregation effect is more obvious (Xie and Shang, 2024).
The Central region’s Gini coefficient was the highest in the country in 2012 and then showed a clear downward trend, with only a small increase between 2016 and 2017, falling to 0.036 in 2021, the lowest in the country. This indicates that the CCDL of STI is more effective between provinces within the central region. The main reason for this is the uneven distribution of resources between universities and enterprises in the central region in 2012, with top universities and enterprises concentrated in Anhui, Hubei and Hunan provinces. However, after 2016, based on Shanxi’s energy advantages, Henan’s agricultural advantages, and Jiangxi’s industrial and location advantages, local universities have received more resource inputs and have fostered a number of advanced manufacturing industries and strategic emerging industries, contributing to a rapid decline in the differences of the CCDL of STI within the central region.
In 2012, the intra-regional difference was small (0.303) and only slightly higher than in the Northeast region (0.279), due to the small number of high-level universities and enterprises in the Western region. Although there was a significant downward trend after 2015, the Gini coefficient of 0.196 in 2021 was the highest among the four regions and even slightly higher than the national level, indicating that the intra-regional difference was still large. The reasons for this are: on the one hand, the full implementation of the National Basic Ability Construction Project of Western and Central after 2013, which have comprehensively upgraded the capacity of education and scientific and technological innovation in the western regions. On the other hand, the construction of the Belt and Road and the Development of The Western Region have promoted the transformation of the western region into an outward-oriented economy and fostered a large number of strategic emerging industries with regional characteristics. However, as the development of the western region is mainly concentrated in a few provinces such as Chongqing, Sichuan, and Shaanxi, the problem of uncoordinated and unbalanced development in the western region still exists.
5.2 Inter-regional differences of the CCDL of STI between universities and enterprises
The Dagum Gini coefficient was used to measure the inter-regional differences of in the CCDL of STI between universities and enterprises among the four regions (Figure 3).
From Figure 3 it can be seen that the inter-regional differences in the CCDL of STI between universities and enterprises among the four regions generally show a decreasing trend over the sample period, but they are manifested in two decreasing patterns.
Among these, the inter-regional differences between the northeast and the other three regions exhibit significant volatility characteristics, while the eastern and western, eastern and central, and central and western regions are mainly characterized by a slow downward trend over the sample period. In terms of the numerical level of inter-regional differences, the average of Gini coefficient in the central and eastern region is the lowest (0.203) in the whole sample, the average Gini coefficient in the central and western region is 0.211, and the eastern and western region is larger at 0.227, which is on a par with the average of the Northeast and central region, and is higher than that in the northeast and western region, which has the highest average of 0.225, and that in the northeast and eastern region, which has the highest average of 0.248. The largest value of the difference between the four regions comes from the comparison between the eastern region and the northeast region, followed by the western and eastern region and the northeast and central region. The results show that the CCDL of STI between universities and enterprises is comparatively low in the northeast and western regions. This is generally consistent with the spatial distribution of China’s top universities and strategic technology enterprises. This alignment suggests that the balanced allocation of regional technological resources significantly influences the CCDL of STI between universities and enterprises (Wu, 2024). From the time-varying trend of inter-regional differences, the degree of differences between the northeast and eastern, northeast and central, and northeast and western regions decreased by 67.85%, 72.77%, and 69.11%, respectively, which was lower than that among the other three regions. In contrast, the difference between the central and eastern regions decreased by 84.97%, the largest decrease in the country. This once again shows that the CCDL of STI between universities and enterprises in the Northeast and Western regions temporarily lags behind the Eastern and Central regions.
5.3 The contribution rate of regional differences in CCDL of STI between universities and enterprises
Figure 4 describes the source and contribution rate of the gap in the CCDL of STI between universities and enterprises in China. As can be seen from Figure 4, the characteristics in the change curve of the inter-regional variation and the intensity of transvariation were roughly symmetrically distributed and had significant fluctuation during the sample period, while the intra-regional variation shows relative stability.
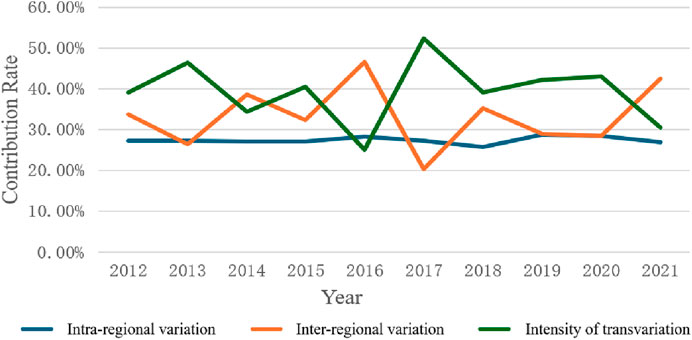
Figure 4. The intra-regional differences, Inter-regional differences and intensity of transvariation to the contribution rate of overall differences of CCDL of STI between universities and enterprises.
In terms of the specific evolution process, the contribution of the intra-regional Gini coefficient of the CCDL of STI between universities and enterprises to the overall difference is 27.25% in 2012 and 26.90% in 2021, which was almost unchanged over the sample period, and the average contribution over the sample period is 27.43%. It further showed that the overall difference in the CCDL of STI between universities and enterprises mainly comes from the inter-regional differences, which is consistent with the previous conclusion. The contribution rate of the inter-regional differences to the total difference shows obvious fluctuation characteristics, from 33.70% at the beginning of the sample period to 46.51% in 2016, then rapidly decreasing to 20.35% in 2017 and then fluctuating to 42.56% in 2021. The average contribution rate over the sample period is 33.30%. The contribution rate of intensity of transvariation to the overall difference was the highest in most years, which is only less than that among regions in 2014 and 2016. The average contribution rate during the sample period is 39.27%.
But comprehensively, the differences between the three are not overwhelming, showing there was still a more obvious unbalanced and insufficient development among the four regions and within each region. The pairing support from the eastern region to other regions has significantly improved the CCDL of STI between universities and enterprises in other regions. However, the pairing support was mainly concentrated in a few key provinces in other regions, which instead increases the intra-regional Gini coefficient and further exacerbates inequality in the overlapping parts of the region, making the overall Gini coefficient rise rather than fall. Therefore, in addition to reducing the unbalanced and insufficient development between regions, the key work to reduce the differences in the CCDL of STI between universities and enterprises in the future must also focus on the intra-regional differences.
5.4 Time dynamic evolution of the CCDL of STI between universities and enterprises in four regions
This study used the kernel density estimation method to portray the time dynamic evolution characteristics of the differentiation of the CCDL of STI between universities and enterprises in the four regions. To better represent the change characteristics in the four regions, 2012, 2015, 2018, and 2021 were selected as key nodes for display, as shown in Figure 5.
According to Figure 5, from the direction of change of the main peak of the kernel density curve, the center position of the main peaks of the four regions shows a trend of gradual shifts to the right, indicating that the performance of China’s CCDL of STI between universities and enterprises shows a positive development trend.
The analysis of the kernel density curve’s main peaks shows a significant increase in peak value and a narrowing width. This indicates a decreasing difference in the CCDL of STI between universities and enterprises in the four regions. The main peak in the northeast region exhibits a “left-right” shift trend, suggesting a decrease in the CCDL of STI between universities and enterprises followed by a rapid increase after 2018. In the eastern region, the kernel density curve has transitioned from single peaks to multi-modal peaks, indicating a multi-polarization phenomenon. Moreover, there was a significant right-tailing phenomenon in the eastern and central regions in 2012, which disappeared in 2021, suggesting improved development in provinces with lower levels of CCDL of STI between universities and enterprises. Conversely, the western region showed a left-tailing phenomenon in 2021, indicating widening gaps with the average level in the region and implying a “the best are better” effect.
5.5 Convergence analysis of CCDL of STI between universities and enterprises
To more accurately examine the development path and trend characteristics of the CCDL of STI between universities and enterprises, a convergence model is adopted to test the convergence of CCDL of STI between universities and enterprises in China and four regions. Specifically, σ convergence to further comprehensively reveal the convergence feature. The results are shown in Figure 6.
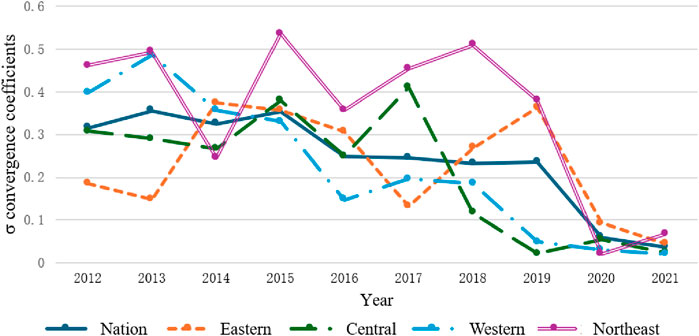
Figure 6. The σ coefficient evolution trend of CCDL of STI between universities and enterprises in China and four regions.
Figure 6 shows the σ convergence results of the CCDL of STI between universities and enterprises in China and four regions. From a national perspective, the convergence coefficient of the CCDL of STI between universities and enterprises showed a slow downward trend, indicating the presence of a σ convergence feature. It can be observed that the convergence characteristics of the CCDL of STI between universities and enterprises in China, as well as in the four regions, have obvious similarities with the evolution pattern of Dagum Gini coefficient. This indicated that the convergence coefficient of both China and the four regions has significant convergence characteristics, suggesting that the CCDL of STI between universities and enterprises in China and the four regions gradually converges to synergistic development.
6 Grey relational analysis
Grey relational analysis model is a system science theory and is one of the important achievements in the field of uncertainty system research. In the grey relational analysis model, levels of relations between main factors and sub-factors are analyzed to determine primary and secondary factors resulting in the development of the system. This study adopts a grey relational analysis model to analyze more deeply the mechanism of the influencing factors of STI of universities and the influencing factors of STI of enterprises on the CCDL of STI between universities and enterprises from the micro level.
Using the national CCDL of STI between universities and enterprises as the reference sequence and the university STI ability indicators and enterprise STI ability indicators as the comparability sequences, the data were processed with homogenized dimensionless and grey relational analysis. Table 5 shows the grey relation coefficients between different indicators of STI in universities and enterprises and the national CCDL of STI between universities and enterprises from 2012 to 2021. The classification of grey correlation coefficients is as follows: 0.8-1 indicates that there is a strong correlation, 0.6-0.8 indicates there is a general correlation, 0-0.6 indicates there is a weak correlation.
Table 5 shows that from the perspective of universities, R&D achievements and the number of R&D personnel have the greatest impact on the national CCDL of STI between universities and enterprises. R&D books and academic papers, as important manifestations of university research results, have an important impact on the CCDL of STI between universities and enterprises. At the same time, the number of teaching and research, R&D personnel have a direct relationship with the quantity and quality of university R&D achievements, so it also has a strong correlation with the CCDL of STI between universities and enterprises. In addition, the National Natural Science Award, National Technology Invention Award, and National Science and Technology Progress Award can be strong proof of the scientific research ability and technology application and transformation ability of universities. This shows that R&D achievements and research talents, as important indicators to measure the STI ability of universities, have a significant impact on the CCDL of STI between universities and enterprises. Higher STI ability can help universities attract more STI resource support, and help universities cooperate with enterprises.
For enterprises, the R&D capability as well as the ability to import, digest, and absorb technology are key factors. The STI talents of enterprises represent the R&D strength of enterprises and the degree of emphasis on R&D. On the other hand, through the introduction and digestion of technology, it can help enterprises to establish good cooperation with universities, to reduce the R&D investment of enterprises in basic theories, and allocate more resources to specialized and sophisticated technology research and development, transformation of scientific and technological achievements and industrialization. At the same time, more enterprises through the establishment of R&D institutions and STI activities, can better attract university R&D researchers to join the enterprise, which is conducive to the exchange of STI achievements between the university and enterprises, thus improving the CCDL of STI between universities and enterprises.
In conclusion, the key factor influencing the CCDL of STI between universities and enterprises is the STI ability of universities and enterprises and the degree of attention paid to R&D innovation. By improving STI ability and conducting basic research, universities can produce more high-quality STI results, which can not only provide enterprises with STI talents but also attract more R&D resources. By introducing STI talents and increasing capital investment in technology introduction and digestion, enterprises can not only improve the STI ability, but also ensure that the achievements of STI in universities can be transformed into stable market income, and provide stable capital investment for universities.
Taking the STI indicators of universities as the reference sequence, the index data of STI enterprises as the comparability sequences, and the STI indicators of enterprises as the reference sequence and the index data of STI universities as the comparability sequences, the grey relational analysis model is used to analyze the influence factors between the universities and enterprises on each other’s active participation in the coupling and coordination development of STI. The results are shown in Table 6.
From Table 6, it can be seen that, from the perspective of universities, among the indicators of enterprises that influence the CCDL of STI between universities and enterprises, the inputs and scales of enterprise STI are ranked high, and most of the inputs for purchasing or introducing technologies are ranked low. Therefore, it showed that for universities, the willingness of enterprises to invest in STI and the R&D environment were the key factors that attract universities to engage in STI coupling and coordination with enterprises. To attract universities to engage in STI coupling and coordination, enterprises need to demonstrate a strong willingness and ability to engage in STI. This is essential to provide university researchers with a favorable environment for STI and to ensure a consistent market demand for university scientific research results. In this way, university R&D achievements can be successfully industrialized and commercialized.
From the perspective of enterprises, universities disclose their patents and take the initiative to seek cooperation to better promote the participation of enterprises in STI coupling and coordination, followed by the university STI ability. The STI of enterprises largely benefits from the application of R&D achievements and scientific and technological services of universities and other research institutions and the transformation of their R&D achievements (Yin et al., 2024). Therefore, universities are willing to disclose and authorize their patents and R&D achievements to enterprises, showing their willingness to cooperate, which has an important impact on the CCDL of STI between universities and enterprises. Universities should take the initiative to carry out the coupling and coordinated development with enterprises and take the initiative to industrialize the R&D achievements.
7 Conclusion and policy recommendations
7.1 Discussion
The present study used a coupling coordination degree model to evaluate the CCDL of STI between universities and enterprises, used the Dagum Gini coefficient to calculate the spatial-temporal characteristics, used the kernel density estimation method to calculate dynamic evolution characteristics, and used the grey relational analysis model to explore deeply the influencing factors.
From the results of the study, the national CCDL of STI between universities and enterprises is gradually improving, reflecting the obvious results of the coordinated regional development strategy (Chen et al., 2025), the CCDL of STI between universities and enterprises in the four regions has been improved, Hypothesis 1 has been proved. Meanwhile, from the spatial distribution situation, it can be found that the CCDL of STI between universities and enterprises is higher in the regions with better economic development, and the regions with weaker economic development show a more obvious polarization phenomenon, which is consistent with the results of related studies (Tan and Yuxuan, 2024). This study reveals that the Northeast and Western regions play a crucial role in the national CCDL of STI between universities and enterprises. Specifically, the development of the Northeast region is heavily influenced by policy, whereas the Western region faces greater constraints due to its environment and geographical location. The government needs to implement proactive macroeconomic policies to narrow the gap between the four regions, while the core provinces in the region should use spillover effects and other means to establish cooperation mechanisms to jointly address development challenges, share experiences, and resources, and prevent excessive polarization that may hinder coordinated regional development (Wang et al., 2023; Luo X et al., 2023).
Regarding the various types of factors affecting the CCDL of STI between universities and enterprises in China, a large amount of literature has explored the influencing factors from multiple perspectives, with most of the results focusing on the fact that universities and enterprises should collaborate to improve the quality of human capital, promote integration of industry and education (Liu et al., 2024). On the university side, it is imperative to reinforce the development of advantageous disciplines and enhance independent STI ability to establish a solid foundation for the CCDL of STI between universities and enterprises (Shen et al., 2023). From the enterprise’s perspective, possessing independent STI ability has a positive moderating effect on the CCDL of STI between universities and enterprises (Liang et al., 2023). These findings align with the conclusions of this study, thereby substantiating Hypothesis 2. Simultaneously, this study further discovered that the willingness of universities to publicize their STI accomplishments and the willingness of enterprises to invest in STI are the crucial elements that additionally impact the CCDL of STI collaboration between universities and enterprises. Therefore, both parties must establish a strong communication mechanism.
7.2 Conclusions of the study
The study results showed that, first, the CCDL of STI between universities and enterprises has gradually overcome the low-level mutual influence and achieved the consistency and effectiveness of STI between universities and enterprises, from extreme non-coordination development to an excellent coordination state. Second, from the point of view of regional differences, the difference in the CCDL of STI between universities and enterprises is mainly due to the unbalanced and uncoordinated problem of inter-regional differences, but the intra-regional difference still exists. Third, from the perspective of dynamic development, the CCDL of STI between universities and enterprises in China and all regions shows an upward trend, but there are large differences in the specific performance of each region. Fourth, the CCDL of STI between universities and enterprises in China and four regions demonstrates σ convergence, and its convergence characteristics bear obvious similarities to the evolution of Dagum Gini coefficient. Fifth, the university STI ability and enterprise STI ability are key factors to enhancing the CCDL of STI between universities and enterprises.
7.3 Policy recommendations
Based on the research findings of this study, the following policy implications are drawn: Firstly, at the national level, the primary objective is to minimize inter-regional differences. In the Northeast and West regions, there is a pressing need to enhance the overall STI capacity of the area. Additionally, in the eastern region, the emphasis is on mitigating intra-regional differences and fostering a more balanced development. Second, for universities, in addition to continuously strengthening their own STI capacity, they also need to focus on the transformation of R&D achievements. Through the establishment of a platform for sharing R&D achievements between universities and enterprises, it has promoted the initiative of R&D teams to stay in enterprises to carry out the application of R&D achievements joint research and development activities, and so on. Third, for enterprises, their own scientific and technological innovation willingness and ability to attract universities to take the initiative to carry out the STI coupling and coordination development key factors. Enterprises must continue to strengthen the intensity of scientific and technological funding, strengthen the sharing of benefits with universities, and be market-driven by the improvement of the STI ability of universities.
7.4 Research limitations and recommendations for future research
The limitation of this study lies in ignoring the heterogeneity of provinces within each region, and the division of the four regions may be insufficient. In subsequent studies, it is necessary to fully consider the characteristics of intra-regional heterogeneity to make a more scientific regional division. In addition, the emergence of digital technology and artificial intelligence will be key factors affecting the CCDL of STI between universities and enterprises, which may influence and cross-talk with each other (Lai S et al., 2024). Therefore, it is necessary to incorporate the impact of new technologies into the follow-up research to analyze the coupled and coordinated development mechanism of both sides more deeply.
Data availability statement
The original contributions presented in the study are included in the article/supplementary material, further inquiries can be directed to the corresponding authors.
Author contributions
XL: Conceptualization, Data curation, Funding acquisition, Investigation, Software, Writing–original draft, Writing–review and editing. QW: Conceptualization, Formal Analysis, Funding acquisition, Writing–review and editing. HG: Data curation, Validation, Writing–review and editing. QC: Methodology, Writing–review and editing.
Funding
The author(s) declare that financial support was received for the research, authorship, and/or publication of this article. Funded by Qingdao Postdoctoral Science Foundation (QDBSH20240102069) and Shandong Postdoctoral Science Foundation (SDCX-RS-202400007).
Conflict of interest
The authors declare that the research was conducted in the absence of any commercial or financial relationships that could be construed as a potential conflict of interest.
Generative AI statement
The author(s) declare that no Generative AI was used in the creation of this manuscript.
Publisher’s note
All claims expressed in this article are solely those of the authors and do not necessarily represent those of their affiliated organizations, or those of the publisher, the editors and the reviewers. Any product that may be evaluated in this article, or claim that may be made by its manufacturer, is not guaranteed or endorsed by the publisher.
References
Chen, M., Nan, S., and Qian, L. (2025). The Road to high-quality development of major strategic regions in China in the new era: logic, effectiveness, and prospects. Rev. Econ. Manag. (01), 28–41. doi:10.13962/j.cnki.37-1486/f.2025.01.003
Cheng, Q., Jiang, S., and Wang, H. (2024). The impact of policy instruments on enterprises' original innovation capacity: empirical evidence based on the panel data of science and technology activities of provincial industrial enterprises. Manag. Rev. 36 (05), 75–88. doi:10.14120/j.cnki.cn11-5057/f.2024.05.006
Chinese Academy of Science and Technology for Development (2023). China regional science and technology innovation evaluation report 2023. Beijing: Scientific And Technical Documentation Press, 1–8.
Guo, D. (2023). Thoughts on building a national strategic scientific and technological force in manufacturing. Res. Dev. Manag. 35 (4), 8–10. doi:10.13581/j.cnki.rdm.20230781
Han, J., and Zhe, L. (2023). Strengthening national strategic scientific and technological power: awareness, problems and suggestions. China Sci. Technol. Forum (03), 11–17. doi:10.13580/j.cnki.fstc.2023.03.011
He, C., and Zhibin, L. (2023). On regional high-quality development in the process of Chinese-style modernisation. Soc. Sci. J. (2), 112–119.
Hu, C., and Ma, X. (2023). Regional differences, dynamic evolution and convergence of carbon emissions from rural residents’ living consumption: evidence from China. Energies 16, 5951. doi:10.3390/en16165951
Jia, R., Wang, J., and Dou, H. (2024). Promoting regional high-quality development with new quality productivity. Reform (03), 38–47.
Jiang, S., He, C., and Zhibin, L. (2024). Theoretical logic and realisation of regional coordinated development by accelerating the formation of new productivity. J. Lanzhou Univ. Soc. Sci. Ed. 52 (2), 5–14. doi:10.13885/j.issn.1000-2804.2024.02.001
Lai, S., Chen, H., and Zhao, Y. (2024). Measurement and prediction of the development level of China’s digital economy. Humanit. Soc. Sci. Commun. 11 (1), 1756. doi:10.1057/s41599-024-04352-z
Li, H., Wang, Y., Zhang, H., Yin, R., Liu, C., Wang, Z., et al. (2024). The spatial-temporal evolution and driving mechanism of Urban resilience in the Yellow River Basin cities. J. Clean. Prod. 447, 141614. doi:10.1016/j.jclepro.2024.141614
Li, Z. (2022). How to accurately understand national strategic scientific and technological power. China Sci. Technol. Forum (4), 1–8. doi:10.13580/j.cnki.fstc.2022.04.011
Liang, Z., Zhang, Y., and Shi, D. (2023). Strategic scientific and technological cooperation between universities and enterprises, industrial dual innovation and high-quality development of the industry—analysis based on China’s IT industry. J. Henan Univ. Soc. Sci. 63 (05), 40–48+153. doi:10.15991/j.cnki.411028.2023.05.009
Liu, H., Niu, J., and Gu, L. (2024). Multiplying high-quality development: synergy and integration of education, science and technology, and talents. Tsinghua J. Educ. 45 (03), 31–36. doi:10.14138/j.1001-4519.2024.03.003106
Luo, X., Liu, Q., and Song, X. (2023). China's strategies for promoting differentiated urban resilience measurement from the social ecosystem perspective. Syst. Res. Behav. Sci. 40 (1), 235–249. doi:10.1002/sres.2832
Ma, Y., and Ma, C. (2024). Coupling coordination and path analysis of university scientific and technological innovation and urban innovation. J. Tianjin Univ. Soc. Sci. 26 (03), 250–258.
Ning, J., Lin, Y., and Wang, S. (2023). Collaborative integration of industry-university-research and performance of science and technology achievement transformation: the moderating effect of cooperative relationship cognition. Sci. Technol. Manag. Res. 43 (01), 92–101.
Shen, J., Jun, Z., and Chen, J. (2023). The integrated innovation model and motivation mechanism of school-enterprise in application-oriented universities: a multiple case study from the perspective of regional innovation ecosystem. J. High. Educ. Manag. 17 (03), 100–110+124. doi:10.13316/j.cnki.jhem.20230504.010
Song, G., Tang, C., Zhong, S., and Song, L. (2024). Multiscale study on differences in regional economic resilience in China. Environ. Dev. Sustain 26 (26), 29021–29055. doi:10.1007/s10668-023-03853-2
Sun, Y., Wang, Y., Zhou, X., and Chen, W. (2023). Are shrinking populations stifling urban resilience? Evidence from 111 resource-based cities in China. Cities 141, 104458. doi:10.1016/j.cities.2023.104458
Tan, F., and Yuxuan, Z. (2024). Coupling coordination between digital economy and green innovation in China: major regional development strategy. Inq. into Econ. Issues (12), 17–34.
Wang, H., and Chen, M. (2019). The coupled and coordinated development of science and technology innovation of universities and innovation of industrial enterprises-an empirical analysis based on 27 provinces in China. Mod. Univ. Educ. (04), 105–111.
Wang, H., Liu, Z., and Zhou, Y. (2023). Assessing urban resilience in China from the perspective of socioeconomic and ecological sustainability. Environ. Impact Assess. Rev. 102, 107163. doi:10.1016/j.eiar.2023.107163
Wu, H. (2024). Developing new quality productive forces according to local conditions: the dimension of regional coordinated development. Explor. And Free Views (08), 119–128+179.
Xie, Y., and Shang, H. (2024). Study on the spatio-temporal pattern of coupling and coordination between higher education scale and regional economy in Guangdong, Hong Kong and Macao Greater Bay Area — a case study of Guangdong region. High. Educ. Explor. (04), 14–23.
Yang, C. (2021). Coordinated allocation of productivity and regional economic layout under the new development pattern. Economy (2), 23–28. doi:10.16528/j.cnki.22-1054/f.202102023
Yin, X., Sun, B., Yuan, L., and Chen, L. (2024). Research on the mechanism of innovation consortium Co-constructed by enterprise from S&T self-reliance and self-improvement perspective. Sci. Sci. Manag. S.&T. 45 (11), 74–89. doi:10.20201/j.cnki.ssstm.2024.11.003
Yu, Z., Jing, Z., Jin, Z., and Dongmei, S. (2024). Analysis on the coupling and coordination of innovation between strategic scientific and technological forces of universities and enterprises. Stud. Sci. Sci. 42 (04), 885–896. doi:10.16192/j.cnki.1003-2053.20230504.001
Yun, Z., Yue, C., Chaoyang, H., and Luyi, C. (2023). The logic and evolution of national strategic science and technology power from the perspective of historical institutionalism. Laboratory Res. Explor. 42 (10), 140–147. doi:10.19927/j.cnki.syyt.2023.10.029
Zhang, W., Hangyu, L., and Zhang, T. (2023). Measurement of the resilience of China's manufacturing industry chain and its spatial and temporal differentiation. Econ. Geogr. 43 (4), 134–143. doi:10.15957/j.cnki.jjdl.2023.04.014
Zhang, X., Zhou, X., and Liao, K. (2023). Regional differences and dynamic evolution of China’s agricultural carbon emission efficiency. Int. J. Environ. Sci. Technol. 20 (4), 4307–4324. doi:10.1007/s13762-022-04196-7
Zheng, S., Han, X., Guo, X., and Zhang, Z. (2024). Construction of national strategic scientific and technological power and enterprises’ key core technology breakthroughs: evidence from national and provincial key laboratories. China's Ind. Econ. 36 (09), 62–80. doi:10.19581/j.cnki.ciejournal.2024.09.004
Keywords: science and technology innovation, coupling and coordination development level, between universities and enterprises, regional differences, spatialtemporal characteristics, influencing factors
Citation: Liu X, Wang Q, Gao H and Cui Q (2025) Analysis of regional differences, spatial-temporal characteristics, and influencing factors of the coupling and coordination development of science and technology innovation between universities and enterprises in China. Front. Earth Sci. 13:1540117. doi: 10.3389/feart.2025.1540117
Received: 05 December 2024; Accepted: 10 January 2025;
Published: 30 January 2025.
Edited by:
Karoly Nemeth, Institute of Earth Physics and Space Science (EPSS), HungaryReviewed by:
Qiang Jason Li, Lancaster University, United KingdomMilos Dimitrijevic, University of Kragujevac, Serbia
Copyright © 2025 Liu, Wang, Gao and Cui. This is an open-access article distributed under the terms of the Creative Commons Attribution License (CC BY). The use, distribution or reproduction in other forums is permitted, provided the original author(s) and the copyright owner(s) are credited and that the original publication in this journal is cited, in accordance with accepted academic practice. No use, distribution or reproduction is permitted which does not comply with these terms.
*Correspondence: Liu Xiao, MTE5MjYzNTE2N0BxcS5jb20=; Wang Qingjin, d2FuZ3FpbmdqaW4yMDA1QDEyNi5jb20=