- 1Department of Geological Engineering, School of Geological Engineering and Geomatics of Chang’An University, Xi’an, China
- 2Department of Geological Engineering, Mine Geological Disasters Mechanism and Prevention Key Laboratory, Xi’an, China
- 3Department of Geotechnical Engineering, National University of Science and Technology, Islamabad, Pakistan
- 4Department of Engineering Geology and Hydrogeology, Kabul Polytechnic University, Kabul, Afghanistan
- 5Department of Sciences and Humanities, National University of Computer and Emerging Sciences (FAST-NUCES), Islamabad, Pakistan
The main difficulties in urban development, choosing a location, and creating preventative safety precautions are accurately characterizing and valuing subsurface soil information from a geotechnical and geological standpoint. This paper discusses how to define and build geotechnical subsoil soil zonation maps (SZMs) for the new Kabul city, Afghanistan, using traditional ArcGIS software assessing Kriging interpolation approaches. With the city’s expansion plans, including New Kabul City’s development, our research supports informed urban development strategies. Subsoil data from 2,13 locations across the city were collected from geotechnical studies, focusing on soil classification, Standard Penetration Test (SPT-N values), undrained shear strength, and consolidation characteristics up to 15 m depth. SPT-N and soil type were used to create SZMs, and other parameters were used to evaluate bearing capacity and settlement. The results revealed that SPT-N values divided the research region into three main sections: A (8–>50), B (13–>50), and C (14–>50). The subsurface strata consist of low-plasticity clay (CL) and clayey sand (SC) underlain by highly plastic clay (CH) and silt (MH). Linear regression predicted SPT-N values with depth, showing a strong R2 of 0.95. This speeds up sub-soil stiffness and strength assessments during building project planning and feasibility studies. The shallow Kabul foundation has an allowable bearing capacity of over 100 kPa, making it suitable for lightly loaded buildings. Predicting SPT-N levels has an 85% correlation coefficient, while soil type has 94%. Accurate geotechnical data on the soil’s underlying layers will help characterize the site and identify future project risks.
1 Introduction
Modern data management methods and technology make data preservation and use easier to optimize new construction planning in response to urban sprawl. To create digital geotechnical soil maps applicable to individuals working at various levels within the infrastructure construction industry, researchers have utilized techniques associated with geographical information systems (GIS). The soil maps generated can effectively depict various subsurface engineering attributes, including stratigraphy, groundwater table, shear strength, bearing capacity, and the identification of problematic soils. The soil maps offer engineers practical and valuable information to plan future projects, eliminating the need for extensive desk and feasibility studies (Akbarimehr and Aflaki, 2019). Geotechnical engineers can better handle uncertainty by dealing with limited data using interpolation (Dodagoudar, 2018); a spatial geotechnical database that is easily available may facilitate design processes and save time (Amini et al., 2024).
The theory of spatial autocorrelation, which is the foundation of interpolation according to Tobler’s First Law of Geography (Tobler, 1970), asserts that locations that are closer together are more similar than those that are farther apart (Arun, 2013). In other words, spatial interpolation estimates attribute values in unobserved locations within a study area that lack data (Waters, 1989; Mitas and Mitasova, 1999) use interpolation methods to calculate unknown values for geographic point data like chemical concentrations, rainfall, noise, and elevation. Interpolation methods create a surface from points and are used to estimate each cell in grids (Chang and Wang, 2008). They fall into local or global categories (Burrough et al., 2015).
The difference between the two clusters is how they approximate unspecified values with predetermined points. When using the global approach, all of the data that is accessible inside the initial dataset is utilized. Instead, the local technique uses only the points nearest the grid node where the value must be estimated (Vansarochana et al., 2009). Interpolation algorithms are exact or approximate depending on whether they preserve sample point values on the surface (Lam, 1983). In addition, there is a distinct distinction between the stochastic and deterministic approaches to approach research. Previous methods use numerical values directly. This idea applies when adequate geographical data is available to characterize its qualities mathematically. Quantifying the spatial autocorrelation between sample points and examining the spatial arrangement of observed points near the projected location (ESRI) uses the statistical features of the given data. Popular deterministic approaches include radial basis functions, inverse distance weighting (IDW), and local polynomial functions of various orders (Eberly et al., 2004). IDW weighs added points using the inverse distance from the exclamation point (Bhattacharjee et al., 2013). The mass of an object reduces at an exponential rate with increasing distance (Hassan et al., 2024). According to (Childs, 2004), interpolation with polynomials ensures that sample points are inserted into an even surface. This method holds a rubber membrane via sample points and lowers surface curvature like radial basis functions (Erdogan, 2009). An additional category of interpolation methods, called geostatistical interpolation approaches, was discovered by (Ouma et al., 2012). Statistical models that consider autocorrelation or statistical correlations between the locations that have been recorded are the foundation upon which these methods work. (Chiles and Delfiner, 2012). State that ordinary and universal kriging are two widely used stochastic methods.
A complete explanation of ordinary kriging, which was utilized in the trials that were carried out for our research, can be found in the “Methodology” section. There are many interpolation approaches for geotechnical site characterization and subsurface data modeling. Data-centric deep learning technique IC-XGBoost3D was used to build a 3D sub-surface geological model (Shi and Wang, 2022; Dell’Arciprete et al., 2012) Sequential indicators, transition probability, and multiple-point simulation replicate alluvial sediment hydrofacies. From sparse observations, (Wang et al., 2019), used Karhunen-Loève expansion and Bayesian compressive sampling to model linear or nonlinear spontaneous fields without detrending. Using data-driven iterative convolution XGBoost, (Shi and Wang, 2021a), generated 2D sub-surface geological cross-sections. The technique extracts stratigraphic relationships from a single image at various scales by fusing training images with the CNN framework. In (Shi and Wang, 2021b) study, a nonparametric, data-driven approach was proposed for geotechnical site characterization. Based on a few observations, this method estimates subsurface lithology using multiple-point statistics. One of the most recent studies, which was conducted by (Shi and Wang, 2021c), suggested a complex sampling strategy. Multiple-point statistics and information entropy accurately identify subsurface lithology and geotechnical drilling locations. Data-driven methods combined prior data into a training picture using point statistics. To measure soil and rock factor changes, (Wang and Zhao, 2016), created a Bayesian inverse assessment model. Used universal and conventional kriging and GIS interpolation to create 3D bathymetric models of Italy’s Giglio Island Seabed (Alcaras et al., 2022; Al-Mamoori et al., 2020) made use of kriging, which is a combination of indicator and ordinary. (Pham et al., 2019). used regression and ordinary kriging to map soil characteristics (total nitrogen, pH, and organic carbon) in hilly central Vietnam. Li et al. (Li et al., 2023) proposed an innovative deep learning-based loess landslide assessment and segmentation model. Li et al. (Li et al., 2022) present a two-phase, image-based, data-driven model for partitioning and discovering landslide areas employing Satellite photos.
For mapping soil attributes, regression kriging was less accurate than standard kriging. Chabala et al. (2017) mapped Zambian soil organic carbon using standard kriging. Spatial and uncertainty maps show soil organic carbon levels in the region. In the study that (Kieft et al., 2014) conducted, Kriging was utilized in order to generate scintillation maps of receiver-satellite connection precision maps. An investigation into the differences and similarities between regression kriging and conventional methods of analyzing soil characteristics was carried out by (Zhu and Lin, 2010). Regression kriging can estimate soil characteristics when values and auxiliary variables are strongly correlated. Unless this is the case, regular kriging is more accurate. Regression and back-propagation networks were used to compare (Huang et al., 2019) soil electrical conductivity maps used standard kriging and regression. They found that conventional kriging prevents extreme value overestimation and underestimation in interpolation surfaces. Utilizing standard kriging in combination with back-propagation data is a precise approach for mapping soil salinity. Safi et al. (2020) developed a GIS-based model to calculate soil erosion in the Chakwal region, Pakistan. This is due to the substantial levels of damaging dispersion clay present in Chakwal and the nearby district of Khushab, which presents serious obstacles to the infrastructure that is now in place (Fatima et al., 2023; Hassan et al., 2023c; Hassan et al., 2023a; Hassan et al., 2023b). Digital soil maps were created by (Taharin and Roslee, 2021) in Sabah, Malaysia. Maps showed clay percentage and cohesiveness. Researchers created these maps using kriging and semivariogram methods. Using geotechnical maps, (Orhan and Tosun, 2010), assessed the foundation’s residential suitability. The authors of the study (Mohammed et al., 2012) developed a geotechnical database beneath the surface in Baghdad using ArcGIS. Extensive investigations have utilized GIS methods to establish a comprehensive global database of sub-surface soil. It has been demonstrated that geotechnical modeling has been carried out in the following countries: Turkey (Kolat et al., 2006), Thailand (Suwanwiwattana et al., 2001), Palestine (Jardaneh, 2007), Australia (Al-Ani et al., 2013a), Portugal (Teves-Costa et al., 2014), Iraq (Al-Mamoori et al., 2020), Pakistan (Khalid et al., 2021; Hassan et al., 2022b; Hassan et al., 2023e; Hassan et al., 2024) and Canada (Chung and Rogers, 2010).
The domain of geotechnical studies in Afghanistan has followed a succinct historical trajectory, marked by the absence of a dedicated department exclusively focused on this discipline within the country’s academic institutions. Notwithstanding this institutional gap, numerous scholars have been instrumental in advancing the field through rigorous research endeavours. In order to carry out a comprehensive investigation into the soil carrying capacity inside the urban expanse of Kabul City, (Hussaini and Hozeh, 2021b), made use of zonation mapping. The scholarly work that they have done in the past demonstrates that this is a substantial contribution that they have made. Their scientific approach makes use of a geographical information system (GIS) environment, and it seamlessly combines the standard penetration test. This test has shown to be a vital component in achieving their study objectives. Research by (Woods et al., 2022) has improved our understanding of Salang highway geological and geotechnical difficulties. They discovered the complexity of the geological landscape and the engineering challenges of this vital infrastructure. On the basis of a distinct plan of action, Zaryab et al. (2019) investigated the intricate engineering features of the rock mass that was situated at the location of the Shah-was-Arus dam in Kabul, Afghanistan. The Chelsaton Sedimentary Basin near Kabul was the subject of research carried out by both (Rasouli, 2020; Rasouli and Safi, 2021), with a specific focus on geology, soil, and sediment within the basin. During the previous study, the utilization of soil physical and chemical parameters in Kabul sedimentary basins was explored and compared. (Rasouli and Vaseashta, 2023). investigated the physicochemical characteristics of the Qalay Abdul Ali Soil in Kabul. The pH, EC, CaCO3, and mechanical properties of Kabul’s Qala Wahid Soil were investigated in another study that was conducted by (Shamal and Rasouli, 2018).
Hussaini and Hozeh (Hussaini and Hozeh, 2021a) strategically utilized data that was derived from a particular subset of boreholes. These boreholes amounted to a significant but limited number, and they were dispersed across a variety of sectors within Kabul City respectively. Even though the study acknowledges the spatial constraints that were imposed by the selectivity of the borehole locations, it still manages to provide valuable insights into the soil properties of the larger urban expanse. It is important to note that utilizing a relatively smaller number of boreholes might present limitations in offering a comprehensive evaluation of the entire city’s bearing capacity. However, the acquired data serves as a foundational reference point, offering initial perspectives on the geotechnical nuances of the soil within Kabul. The strategic placement of these boreholes enables the researchers to capture certain key variations in soil properties, albeit within the confines of the sampled locations. Therefore, while the findings may not be exhaustive, they contribute significantly to the initial understanding of the soil characteristics across diverse sectors of Kabul city, guiding future endeavors for a more comprehensive assessment of its bearing capacity. However, research has not been conducted explicitly on the New Kabul City. Afghanistan’s enormous and daring construction project, the New Kabul City, is a big step toward modernization. Thanks to this project, the country’s capital, Kabul, will experience less population burden. The project also intends to give its people access to a sustainable, organized urban environment.
Moreover, the current work attempts to solve some of the major drawbacks of previous research that has applied various interpolated techniques to geotechnical assessments, such as utilizing the IDW methodology for soil zonation mapping (Ahmed et al., 2020; Hussaini and Hozeh, 2021a). However, the precision and geographic accuracy of the simulations weren't sufficient to capture local shifts in soil characteristics, which might compromise the reliability of their predictions. Studies by (Arshid and Kamal, 2020; Ijaz et al., 2023) created GIS models, although they did not thoroughly test the models’ geographical accuracy with actual data. The lack of comprehensive validation could result in disparities in regions with diverse geologic circumstances. Many studies (Pham et al., 2019; Sulyman et al., 2020; Hassan et al., 2022b; Ullah et al., 2022; Daniyal et al., 2023) only examined N-values and/or kinds of soil within a certain length. To construct the geological map for Erzincan, Cabalar et al. (2021) used GIS interpolation based on various index properties of soil. However, they neglected to consider the deeper thickness or additional variables, like evaluation of bearing capacity and settlement, which is crucial for designing the foundation of structures. Much research (Akhter et al., 2014; Pham et al., 2019; Khalid et al., 2021; Daniyal et al., 2022) was carried out using scant data, such as an approximate number of 75 boreholes, raising questions concerning the prediction’s dependability. The geotechnical variables were not correlated in most research (Al-Ani et al., 2013b; Cabalar et al., 2021; Hussaini and Hozeh, 2021b). To ensure the accuracy and reliability of geological subsurface depiction, it is crucial to evaluate subsurface information’s reliability and thoroughly verify processes. It needs to correct any prospective inconsistencies brought on by insufficient confirmation.
Thus, the purpose of this work is to create soil zonation maps (SZMs) for the new Kabul City, Afghanistan, utilizing kriging interpolation methods, SPT-N values, and soil types, and to develop bearing capacity and settlement charts for each zone, which will be accomplished by conducting soil property analysis at depths of up to 15 m and to examine how these results may affect Kabul’s future construction methods and geotechnical studies. To achieve this objective, extensive field and laboratory test data were carefully collected. A comprehensive selection of 130 boreholes and 83 test pits was made based on geotechnical investigation reports of the developing terrain in the new Kabul city. This systematic integration of diverse sources of empirical data underpins the reliability and robustness of the subsequent analyses and interpretations, ensuring a comprehensive and accurate characterization of the soil properties essential for the proposed SZM and foundation design considerations. SPT-N values, classification, undrained cohesion, density, compression index, and void ratio were obtained from the reports. Developing a comprehensive subsoil database has the potential to decrease construction expenses and timeframes greatly. Having access to dependable geotechnical data regarding the layers beneath the surface is essential for effectively planning future site investigations, conducting preliminary design work, performing desk studies, and identifying potential geotechnical risks.
The paper is organized into distinct sections: “Study Area” provides information about the geographical context of the study, “Methodology” explains the research procedures that were used, and “Results and Discussion” presents geotechnical maps, statistical analyses, and correlations in a comprehensive manner. The concluding section, “Conclusions,” synthesizes the study’s insights and discusses their broader implications in geotechnical engineering. This organized format aims to provide a clear and coherent presentation of the research.
2 Study area
The projected New Kabul City is strategically located between Kabul and Bagram airports in the northern region, having latitudes from 34°-33′to 34°-40′and longitudes from 69°-14′to 69°-16′. It includes essential territories such as Karabagh, Kohdaman, Kalkan, Barik Ab, portions of Shekardara, and the Dehsabz districts. The master plan for the future city delineates three separate sections: an economic-agricultural zone, South Dehsabz, North Dehsabz, and Paymanar. This research primarily examines the early stage of building Kabul New City (Dehsabz South), with a particular emphasis on analyzing the usage of the Kabul-Jalalabad route and the expected ring road in Kabul province. Figure 1 shows that the study area is large, covering 25,000 acres and over 80,000 housing units. Figures 2, 3 display the annual distribution of precipitation in the Kabul River Basin, as determined by (JICA, 2009) using WORLDCLIM data. The air temperature data in the Kabul River Basin is derived from the same study. Distribution Flat and productive plains primarily define the sub-surface. It has a significant deposit of sediment, which is more than 3 m thick. The sediment consists of clays, silts, and sands with poor sorting. Figure 2 illustrates the presence of scorching and arid summers and frigid and snowy winters in Kabul. The average temperatures in Kabul range from 4.4°C to 33.8°C, with occasional extremes of −10°C or 37.2°C, as shown in Figure 4. Kabul’s climate, assessed using the beach/pool score, is ideal for engaging in hot-weather activities from mid-June to late August. The city experiences intermittent rainfall, with the rainy season lasting for 9.8 months from January 19 to November 14. During this period, there are 31 days with precipitation over 0.5 inches. The most significant rainfall in March was 1.9 inches, while in December, the lowest precipitation was 0.3 inches. Kabul has fluctuating snowfall quantities, primarily throughout the winter, from December 8 to March 9. Among these months, February receives the most significant average snowfall, 2.9 inches. This is the first comprehensive study of Dehsabz soil geotechnology. This is because this region has never been systematically analyzed. For informed economic building planning, experts recognize the city’s growing industrial and infrastructure growth and the need for underground data. Consequently, this study signifies substantial advancement in fulfilling the demands of this essentiality.
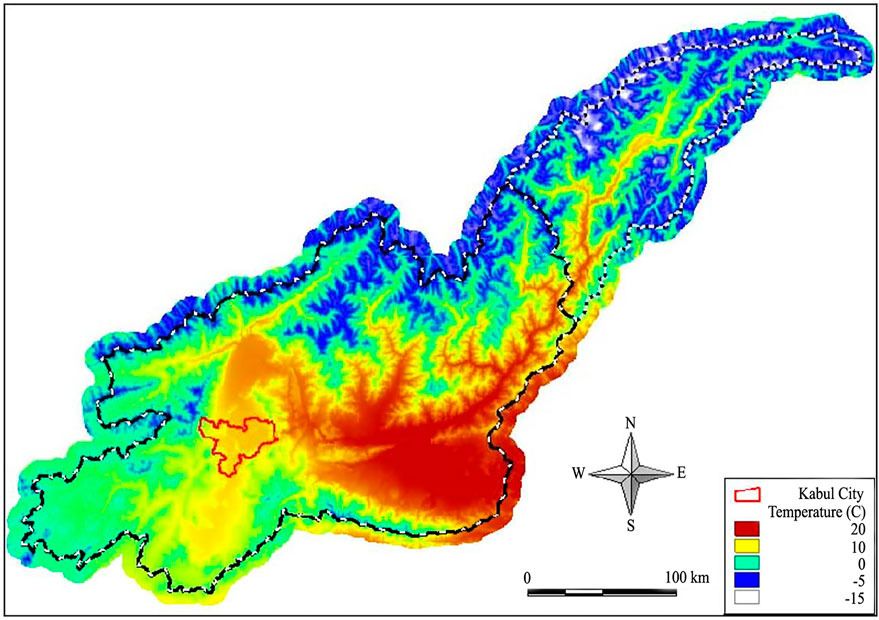
Figure 4. Kabul river basin average air temperature distribution. The average temperatures in Kabul range from 4.4°C to 33.8°C, with occasional extremes of −10°C or 37.2°C, as shown in Figure 4.
2.1 Geology setting
The Kabul Block presents a compelling geological and tectonic landscape characterized by its intricate structure. The Ghazni Fault zone delineates its border with the Katawaz Basin in the southeast (Collett et al., 2015). The Altimoor Fault divides the Nuristan Block from the Kabul Block, which is located to the east. In regional geology and tectonics, the Kabul Block is a subject of noteworthy significance because this geological configuration adds a layer of complexity and interest to the overall structure. The Herat-Panjshir Suture Zone and the Western Hindu Kush are located on the Northern Kabul Block border (Tapponnier et al., 1981). The Kabul Block is made even more complicated and significant in the region due to this suture zone, which serves as a major geological border between the Eurasian and Afghan Central Blocks. There are a few higher-grade granulite-facies rocks among the basement rocks of Kabul City, but the majority of the stones are migmatites, gneisses, and schists (Collett et al., 2015). Researchers discovered granulite-facies structures in carbonates that were felsic, mafic, and impure.
Unaltered to mildly altered Late Paleozoic to Cenozoic volcano-sedimentary phases distinguish the area (Fesefeldt, 1964; Abdullah and Chmyriov, 1977; Collett et al., 2015). The Kabul Basin is largely terrestrial or Neogenic lacustrine sediments, with loess layers being the youngest. Local sedimentation is indicated by substantial loess deposits on the basin’s periphery. Migmatites, gneisses, schists, and higher-grade granulite-facies basement rocks form Kabul City’s structure and geology. The study site is in the late Pleistocene Q3loe Loess, distinguished by a greater abundance of loess than sand and clay. The geological intricacy of this region has been heightened by the buildup of shingle and debris layers, including gravel, sand, and clay, throughout the late Pleistocene epoch, as seen in Figure 5, compiled by (Wahl and Bohannon, 2005).
3 Methodology
A flowchart (Figure 6) illustrates the current study methodology, providing a brief and explicit summary of the approach taken. Detailed explanations for each step are presented in the following subsections.
3.1 Data collection
Geotechnical field and laboratory data from 130 boreholes and 83 test pits from various sites were collected with public and private sector consent. Consultants, geotechnical contractors, and government agencies are examples. No conflict of interest. All private companies in Afghanistan were registered with the Afghanistan National Standards Authority (ANSA), the Ministry of Urban Development and Land, and the Ministry of Public Works. The study area borehole locations is marked on the New kabul city map and shown in Figure 7, which represents phase one of the new Kabul city map. From the reports of geotechnical soil investigations, the following categories of information are extracted: the coordinates of the sites, the SPT-N values, the soil classifications according to the unified soil classification system (USCS), the undrained shear strength, the Atterberg limits, the soil density, the compression index, the void ratio, the depth of the groundwater table, and the bearing capacity values at various depths. For light-load structures, the zoning strategy was carefully planned to include only the top 7.0 m of soil strata. In a more detailed analysis, depths of up to 16 m for Standard Penetration Test (SPT-N) values and 30 m for soil types were considered, as classified by the Unified Soil Classification System (USCS).
The geological parameters collected in this study include stratigraphy/soil classification, SPT-N values, and depth to bedrock (if encountered). Stratigraphy was determined by drilling boreholes at the study sites. Soil samples were then collected from these boreholes and classified based on laboratory testing per USCS classification system (ASTM D2487, 2017), which included grain size analysis (gradation) per (ASTM D422, 2007) and Atterberg’s limits tests per (ASTM D4318-17, 2010). SPT-N values were obtained by conducting Standard Penetration Tests (SPT) at various depth intervals within the boreholes per ASTM (ASTM D 1586, 2022). The bearing capacity and settlement analysis evaluation included additional parameters such as undrained shear strength, soil density, compression index, and void ratio. The undrained shear strength was measured in accordance with (ASTM D 2166-98a, 2000), while the soil density was determined from Proctor tests following (ASTM-D698, 2021). Soil samples were collected using a Shelby tube to obtain undisturbed samples was also used to determine the bulk density of soil. Additionally, the compression index and void ratio were determined from consolidation tests performed in the laboratory (ASTM D 2435-04, 2011). The hydrological parameter collected was the depth of the groundwater table, which was measured during borehole drilling at the study sites. After drilling to the required depth, the boreholes were left undisturbed for 24 h, allowing the groundwater to stabilize. The depth of the groundwater table was then measured. The meteorological data for the study area, including rainfall, snowfall, and temperature, were obtained from publicly available databases provided by national meteorological organizations.
3.2 Statistical analysis of SPT-N
The SPT-N data were analyzed statistically to validate the data, ascertain dispersion, and measure the variation of SPT-N at various depths. A detailed study of borehole data using descriptive and inferential statistical methods can reveal soil variability and geotechnical hazards. In the early stages of this significant urban development project, an accurate and customized study of the project’s needs is essential for informed planning and risk avoidance. For instance, we have thoroughly analyzed a wide range of descriptive statistical measures within the dataset to determine the level of variation and the average value. This was done to examine the data associated with the SPT-N value. The result of a comprehensive investigation was attained. In a numerical sequence, the mean is the average value, the mode is the most frequently appearing, the median is the middle value, and the standard error estimates the difference between the sample mean and the population mean. Quantifying the variation or dispersion of a set of values is another purpose for which the standard deviation has been computed. As a standardized method of measuring dispersion concerning the mean, the percentage coefficient of variation (%CV) is also evaluated with this method.
To compile average SPT-N values for all the suggested sections, one could calculate the comparative dispersion in the data for each section using the coefficient of variation (%CV). This would be possible if one used the methodology that found that a higher percentage of CV values increased data dispersion and decreased accuracy. Empirical correlations were built from linear regression analysis of all developed sections. In addition, kurtosis and skewness were investigated to understand the peakedness and asymmetry of the data distribution. Specific inferential statistical methods, specifically the one-way Analysis of Variance (ANOVA) test, have been utilized to validate the statistical significance of the mean and variance observed in the dataset. The one-way ANOVA is an ideal option for examining SPT-N values among different layers of soil and depth ranges. This technique is especially useful for detecting substantial discrepancies in averages between at least three distinct groups. Before performing the ANOVA analysis, the authors carefully evaluated the study’s assumptions, such as equality and normality of variability, to confirm the outcomes’ robustness. By corresponding conflict within and between groups, the one-way ANOVA delivers a trustworthy and reasonable framework for deciding whether the commemorated deviations in SPT-N values are statistically influential, keeping knowledgeable interpretations of variability in soil.
3.3 Development of soil zonation maps (SZMs)
The mapping process used ArcMap’s advanced features (ArcGIS version 10.5) and kriging interpolation. The utilization of this method was based on the inclusion of SPT-N values and USCS soil types in each geotechnical report for the study area. This ensured a thorough and consistent approach to zoning. In light of the specific geotechnical properties gleaned from the 130 boreholes drilled as part of the New Kabul City project, tailoring the approach to zonation and subsurface analysis is essential. With this extensive data set primarily focusing on Standard Penetration Test (SPT-N) values and soil types, we have established the foundation of our geotechnical understanding, which is essential for directing the initial stages of urban development in this region. The New Kabul City project does not contain large buildings or specialist linear constructions, but it is increasingly vital to examine and handle accessible and relevant geotechnical challenges. This is because the project seeks a sustainable future. Our zonation maps will precisely represent the distinct subsurface features of the New Kabul City project area. They will conform to the practical limitations and potential for expansion in the region. This approach is specifically tailored to meet the region’s requirements. In order to demonstrate the practical application of this work, generalized soil profiles were created using collected data. The profiles contained the settlement and bearing capacity curves for individual footings. The depths of the footings were 1 and 1.5 m.
3.4 Kriging interpolation technique
For the purpose of estimating the weight at a location that has not been tested, the Kriging interpolation method, which is described by (Krivoruchko, 2012), makes use of a geostatistical model. The model is modified in accordance with the spatial correlation that exists between the areas that have been tested. This allows for the achievement of the desired result. The essential principle that underpins the Kriging technique is that the spatial variation of any property is too irregular to be represented by a straightforward mathematical procedure. This thought is the foundation of the Kriging technique. Stochastic interpolation is able to provide this peculiar spatial fluctuation (Chiles and Delfiner, 2012), and the results are typically satisfactory overall. According to (Salekin et al., 2018), Kriging is a method of stochastic interpolation that is based on the idea that the connection between neighboring sites can be characterized by a statistical relationship that does not always represent the physical meaning of the proximity. Kriging is a method that was developed to solve this problem. One method that can be used for stochastic interpolation is called Kriging. Stochastic interpolation method kriging assumes neighboring places can be connected by a statistical relationship that does not necessarily indicate proximity. Many fields use the accurate and straightforward Kriging approach. It aids meteorological precipitation intensity investigations. It is used in soil property variability research, a significant geosciences field, and indicates the technique’s versatility (Robinson and Metternicht, 2003).
Furthermore, the Kriging approach has been successfully utilized in the modeling of spatial temperature, which is an essential component in the field of climatology and environmental research. Positive results have been achieved as a consequence of the use of the Kriging technique. The method’s ability to capture and analyze spatial temperature fluctuations was proved by (Wu and Li, 2013; Alcaras et al., 2020) Parente, It's relevant to topographical and geographical information systems because it's used to produce DEMs. Kriging is a versatile geographical data analysis and modeling tool that has been applied to meteorology, geosciences, and climatology. Kriging can be used in conventional, universal, probability, indicator, and disjunctive applications. Each method adapts the basic kriging approach. We have chosen to employ the standard kriging method to assess the precision of these approaches in generating zonation maps using different soil variables, such as SPT-N values and soil types (Wackernagel and Wackernagel, 2003). State that conventional kriging may estimate a value at a point in a variogram-known area using data from the nearby. According to (Taharin and Roslee, 2021), the premise upon which the ordinary kriging prediction is built is Equation 1.
Z(s) denotes the desired target value, µ denotes the anticipated average or mean value, and ε(s) represents the unforeseen error that will diminish the value discrepancy.
A semivariogram (as depicted in Figure 8) would be generated first to determine the most accurate model for the data. This semivariogram would then be used as a mapping tool to trace the development of SPT-N maps as well as soil-type maps.
The kriging method would be considered. The semivariogram is a graph developed by (Jian et al., 1996) that represents the semi-variance as a function of the distance between two ends. The semi-variance is determined using Equation 2 from the study conducted by (Rishikeshan et al., 2014).
The symbol
3.5 Validation method
Data validation methods are essential when conducting studies because they guarantee the precision, dependability, and universality of models and findings. The collected dataset was split into two parts: one for model development and the other for validation. 80% of the data (170 points) was used to develop zonation maps and empirical models for SPT-N value prediction, bearing capacity, and settlement charts. This more significant portion ensures that the model or map has sufficient data to accurately capture the underlying patterns and trends. The remaining 20% of the data (43 points) was reserved to validate the developed models, maps and charts. By keeping this data separate, the model’s performance on unseen data can be tested, indicating how well the model generalizes to new situations. This approach enables consider whether the model is overfitting or performing as expected. Since it offers a fair balance, the 20–80 data split is frequently suggested in the literature and used by numerous researchers for model validation and training (Hassan J. et al., 2022; 2022b; Nawaz et al., 2022b; 2024e; Amini et al., 2024). A sizeable chunk of the data is used to create the model, with enough kept aside to ensure its accuracy. Various sections used different performance metrics to validate the model’s performance, including the correlation coefficient, coefficient of determination, mean, root mean square error, mean standardized error, root mean square standardized error, and average standard error.
4 Results and discussion
4.1 SPT-N values statistical evaluation
An assortment of statistical parameters was incorporated into the analysis to evaluate the degree of uniformity of the test data obtained from the study area. Table 1 presents the statistical parameters for these parameters up to a depth of 16 m. These parameters include the mean, mode, median, standard error, standard deviation, and the maximum and minimum SPT-N values. The table displays these parameters. Statistical analyses of SPT N-Values in three sections of a study area show in Table 1 indicate unique soil resistance characteristics and significant differences in resistance levels. Among the three sections, Section A has the lowest average soil resistance with a mean N-value of 49.07, Section B has the highest soil resistance with 62.36, and Section C is in the middle with 55.99. The precision of these mean calculations is evidenced by their standard errors, with Section B showing the highest precision. The lowest standard deviation in Section B indicates a more uniform soil resistance. According to the coefficient of variation (%COV), Section B displayed the most minor relative variability, 30.68%. There are deviations from a normal distribution in every section, as shown by the shapes of the distributions, which are indicated by kurtosis and skewness variables (Nawaz et al., 2023b; 2024c; 2024a; 2024d). On the other hand, Section B demonstrates a significant negative skew, which suggests that there is a tail towards lower values. The inferential ANOVA analysis indicates that the means of soil resistance across the sections are statistically different, with a significant F-value of 5.5776 and a very low P-value of 9.08E-21. This detailed statistical analysis shows variability within and across sections and confirms their statistical significance, allowing informed conclusions about soil resistance in the research area (Hassan et al., 2017; Nawaz et al., 2024e).
4.2 SZM based on SPT-N values
To generate a soil map of the study area using SPT-N values, the SPT-N data from the top 30 m of soil are analyzed using statistical methods. There is a higher number of data accessible at depths up to 16 m, as indicated by the outcomes of this study. These findings are utilized in the process of developing the soil map. Consequently, the SPT-N value soil map is generated by using data extending as far as 16 m below the natural surface level by utilizing SPT-N data obtained from the uppermost 16 m, consisting of 2080 values, as an input in ArcMap10.5, a series of experiments were conducted to generate a comprehensive soil representation. Four distinct semivariogram models have been chosen for the SPT-N numerical values. Exponential, Gaussian, spherical, and circular models are all included in this category. These models are depicted in Figure 9 and Table 2 provides a list of significant indices that can be utilized to evaluate the degree of accuracy obtainable from these models. According to (Cambardella et al., 1994), autocorrelation or spatial dependence was high if the nugget-to-sill ratio was less than 0.25, medium if it was 0.25–0.75, and low if it was greater than 0.75. Following the data presented in Table 1, the autocorrelation is moderate, and the ratio of nuggets to sills is 0.59. According to the exponential model, this is true.
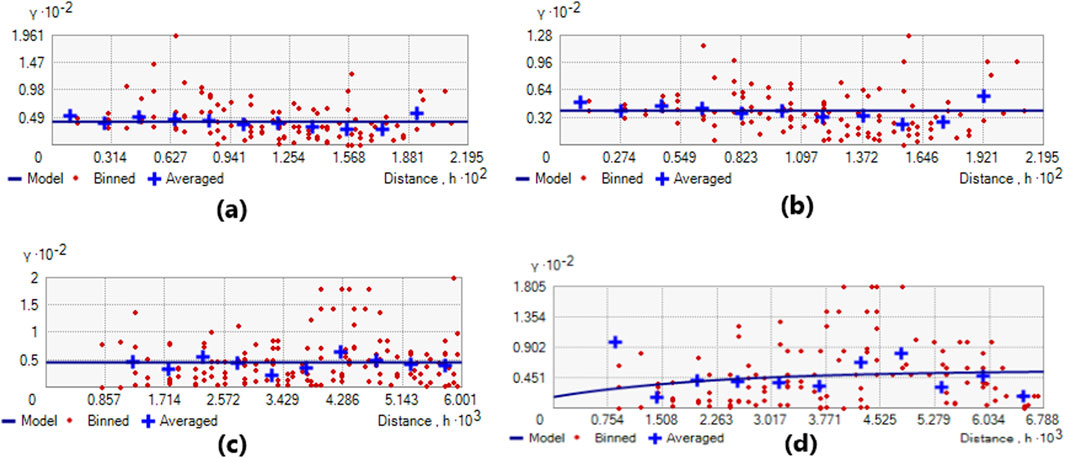
Figure 9. Displays the semivariogram model for SPN N-Values, including the exponential model (A), Gaussian model (B), spherical model (C), and circular model (D).
Table 2 presents statistical indices that summarize the cross-validation results of four predictive models, namely Exponential, Gaussian, Spherical, and Circular when applied to SPT-N values. The Exponential model demonstrates excellent performance based on its lowest Mean (0.091), Root Mean Square (6.798), Mean Standardized (0.012), Root Mean Square Standardized (1.0386), and Average Standard Error (6.556). These findings suggest that the exponential model makes the most accurate and consistent predictions. Gaussian, Spherical, and Circular models have similar RMS, MS, RMSS, and ASE values with a slightly higher Mean. These three models perform similarly but are less effective than the Exponential model in this context. The utilization of ArcMap10.5 and the Spatial Analyst tool, along with the ordinary kriging interpolation technique, has yielded a dependable and accurate SZM for the uppermost 7-m layer. Figure 10 Shows the study area’s soil zonation map based on SPT-N-values in the new Kabul city phase one development plan.
The top 7-m strata were the ones through which this analysis was carried out. Considering that the physical appearance of SZM is the same at different depths, the authors decided to present a single map. The SPT-N threshold was between 4 and 15 depending on the soil consistency (Peck) that was defined, and it was between 16 and 30 and higher than 30. The various ranges of SPT-N represented three distinct subsoil strata: firm to stiff (four to fifteen), very stiff (16 to thirty), and hard/very hard (more than thirty). Because of the SPT-N values, the region being investigated has been divided into three primary sections, and the variations between these sections are presented in Table 3. While the SPT-N value range for section A is between 7 and 17, section B is between 7 and 23, and section C is between 7 and 12. All these values are found within the top 7 m of strata. If we look at the 7–12 m deep strata, the SPT-N range for zones A, B, and C is 27–43, 21–44, and 17-42, respectively. Hussaini and Hozeh (Hussaini and Hozeh, 2021b) reported a similar range of results in the study area, as they developed bearing capacity maps utilizing SPT-N values. Amini et al. (Amini et al., 2024) also reported similar results of SPT-N values in the study area, and both studies validated current findings.
The attributes of each zone indicate the geological attributes correlated to high and inferior SPT-N values. Generally, high SPT-N denotes denser, better-compacted grounds, which possess moderately higher unit weight, strengthened load-bearing ability, and lessened compressibility. In contrast, more inferior values of SPT-N denote softer/compressible soils with moderately inferior unit weights that are prone to settlement under applied loads (Terzaghi and Peck, 1967). Section C demonstrates low average compressibility/settlement and bearing capacity, indicating that soil stabilization needs to be optimized for foundation use. Section A exhibits inferior compressibility/settlement compared to Section C, providing adequate load-bearing ability and needing minor stabilization for infrastructure construction. Section B shows minor to no issues and offers superior load-bearing capability. Recognizing these aspects is vital for advising decisions in geotechnical engineering tasks, particularly in site choice, foundation, and construction planning. Geosynthetic reinforcement can be effectively employed in areas where low bearing capacity, high settlement, and poor strength are concerns (Hassan et al., 2022c; 2023d).
Together with the percentage coefficient of variance (%CV), the SPT-N ranges of values are shown in Table 2. Measuring the dispersion in each zone is possible by separately calculating the percentage CV. (Hassan et al., 2022b), Suggest that the CV percentage in Table 3 can help prioritize projects in favorable soil conditions and reduce geotechnical hazards. This would lower the costs associated with building foundations. Data dispersion percentages in each area can be used to select the geotechnical investigation scope carefully. As an illustration, in circumstances where the rate of CV is more excellent than twenty percent, geotechnical investigations need to be more exhaustive, and the foundation design needs to incorporate a higher safety factor (Hassan et al., 2023e). Table 2 shows that in each of the three sections, the computed value exceeds 20%. This is due to the significant differences in soil compaction within the large city area, as indicated by the greater deviation in SPT-N values from their mean values. Sections A, B, and C each have their unique SPT-N profile, depicted in Figures 11A–C, respectively. Since the SPT-N test is typically not performed in areas where rock is present, the SPT-N profile for Section C does not include any additional SPT-N data. This is because the SPT-N test generally is not carried out in these areas. According to (Terzaghi et al., 1996), it should be mentioned that there is a correlation between the depth and an increase in the SPT-N values. This highlights the soil’s consistency change and the transition from firm to complex. Furthermore, the SPT-N values tend to rise as the depth of measurement increases and agreed with the findings of (Hussaini and Hozeh, 2021a; Amini et al., 2024).
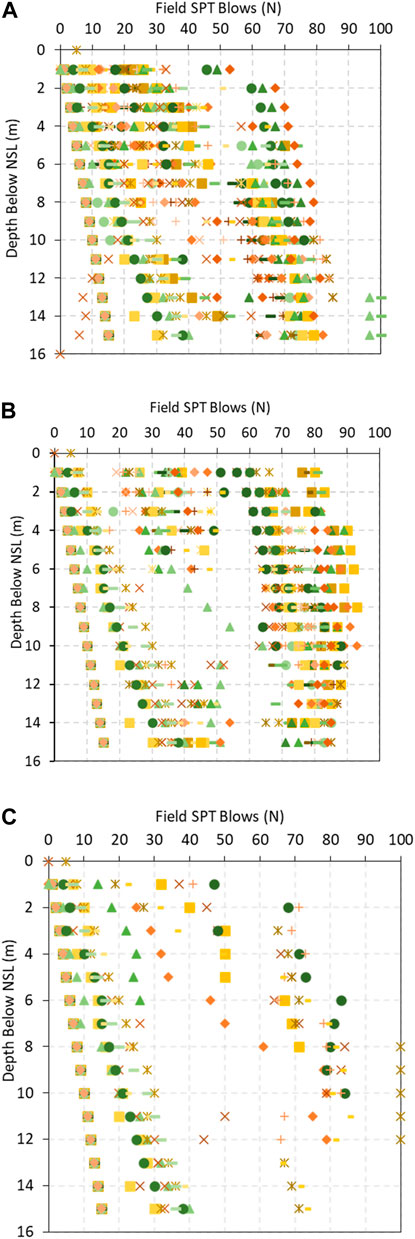
Figure 11. Displays Standard Penetration Test-N values for various sections. (A) Section A, (B) Section B, (C) Section C.
4.3 Correlations for predicting SPT-N values
Linear regression was utilized to develop soil map section correlations. The statistical variation that is displayed in Figure 12 was used to predict the SPT-N value with depth. For map and correlation validation, 42 borehole SPT-N values (1–15 m) were compared to predicted values at corresponding depths. (Nawaz et al., 2022c; 2023a; 2024b). extensively discuss GIS performance indicators like R2, RMSE, and correlation coefficient. Table 4 shows distinct linear relationships in SPT N-value three-section regression. Section A has moderate R-squares of 0.18132 and 0.12064, indicating a limited variance explanation. Section B has higher R-squares, Pearson’s r, and correlation coefficients. Section C has strong correlations with high R-squared values of 0.734 and 0.914, indicating that the independent variable can predict much of the dependent variable’s variability. Each section has distinct regression line levels, trends, intercept, and slope uncertainties. Section C has the most accurate fits, lowest residual sums of squares, and highest adjusted R-square values.
An examination of the differences between the SPT-N values that were predicted and those that were collected at different depths is depicted in Figure 13. A satisfactory correlation coefficient of 0.91 was obtained by comparing the two variables. A correlation coefficient that is greater than 0.8 indicates that there is a strong relationship between two different sets of variables, as stated by (Alshameri, 2020). Since 85 percent of the predicted values are contained within a confidence interval of ±10 percent, the SZM demonstrates a low range of error, as demonstrated by the fact that found that the SZM has a low range of error.
4.4 SZM depending on lithology and soil types
The research area is comprised of a variety of soil types, including lean clay (CL), silty clay (CL-ML), silt (ML), sandy clay (SC), fat clay (CH), and fill materials. Each of these soil types possesses unique characteristics that determine whether they are suitable for use in engineering and construction applications. The 2080 soil classifications were classified into five groups and entered ArcMap along with Northing/Easting coordinates. The soil map of the study area was generated by employing the kriging method, which involved analyzing the maximum depth of up to 30 m and was based on the USCS soil classification. The exponential model demonstrated superior performance after cross-validation on the soil type-based map. The research area utilizes data from 130 boreholes drilled to depths ranging from 1 to 30 m to examine the materials’ engineering and geotechnical characteristics. Figure 14 contains an accurate representation of the soil map. Vertical profiles were created for each section by conducting trials in ArcMap10.5 at 3-m depth intervals (1, 3, 5, 7, 15 m). For the upper 15 m of soil, including the natural surface, USCS soil type distribution geospatial representations have been carefully created. The study area has lean clay, silty clay, and silt soils (CL, CL-ML, and ML) according to the USCS framework. Figure 15 depicts the research region’s soil distribution zonation maps at various depths. The upper 9 m are loose, clayey soil. From 9 to 13 m, low to moderately flexible cohesive silty clay soil (SC) takes over. The layers from 13 to 15 m are silt and extremely pliable clay (CH), revealing the study area’s complex subsurface composition. After examining the stratigraphy of the three primary sections, a simplified average vertical profile was created using statistical analysis, as depicted in Figure 15. A similar kind of strata was also reported by (Rasouli, 2020); in their study, he investigated the soil chemical and physical properties of sedimentary basins of Kabul city. In other research, (Rasouli and Safi, 2021), examined the soil geology and sediment of the Chelsaton basin and agreed with the current findings. Numerous other studies have reported similar data at shallow depths in the study region (Shamal and Rasouli, 2018; Amin and Helmi, 2021; Rasouli and Vaseashta, 2023; Amini et al., 2024).
In addition, the results of this investigation provide a critical new understanding of the geological risks related to the different kinds of soil in the subject region. This study helps to evaluate potential hazards associated with soil settlement and integrity in construction endeavors through the identification of particular features like compressibility and bearing capacity. These observations are consistent with those made by (Rasouli, 2020) and other researchers, who have emphasized the significance of comprehending soil characteristics concerning geologic potential hazards. The recurring themes found in many studies highlight the need for in-depth geotechnical studies in comparable areas to reduce hazards and provide guidance for efficient construction methods.
4.5 Groundwater table
Many researchers have studied Kabul’s groundwater table (GWT), including (Saffi, 2011; Nasir et al., 2021; Zaryab et al., 2022) which found shallow water tables in Dehsabs district’s north and south. According to 130 boreholes in Phase One of Kabul’s new city, the groundwater table (GWT), often found at 30 m, significantly affects geotechnical characteristics and foundations. This level of discussion highlights foundation planning challenges and considerations. It affects soil load-bearing capacity, saturation, and earthquake liquefaction. Monitoring excavation and dewatering are essential when the groundwater table is high. Groundwater levels also affect soil expansion, contraction, and permeability, affecting foundation performance. This requires careful design. The environmental effects must also be assessed, including on groundwater-dependent plant life and ecosystems. Underground investigations necessitate a minimum of 30 m of groundwater table studies. Geotechnical investigations are essential for guaranteeing the stability and integrity of nearby construction projects, as they provide vital information.
4.6 Settlement and load-bearing capability assessment
Shear and settlement requirements were taken into consideration during the ABC examination of shallow foundations, specifically square and strip footings. According to (Li et al., 2017), the recommended safety factor was 3.0, and the maximum amount of settlement that could be tolerated was 25 mm. Both ultimate limit state (ULS) and serviceability limit state (SLS) design requirements will apply to foundation designs. ULS is the absolute maximum load the earth can absorb without the structure collapsing. Serviceability limit state (SLS) refers to the foundation’s ability to endure settlement without failure. Meyerhof’s method, which was first introduced by (Meyerhof, 1951), is used for the purpose of determining the load-bearing capacity of shallow foundations, with a particular emphasis on shear needs. This calculation is expressed by Equation 3.
where
This method is frequently utilized because it provides more dependable outcomes than others (Shafique and Qayyum, 2011; Shill and Hoque, 2015; Abdul-husain, 2016; Nawaz M. M. et al., 2022). Both (Meyerhof, 1970; 1985; Mohurd, 2012) suggest a factor of safety (FOS) between 2 and 3, whereas the US standard (Design, 1992) recommends an FOS between 2 and four for the foundation design of the building. As modified by (Joseph and Bowles, 1997), the Meyerhof method was utilized to meet settlement criteria, as described in Equations 4, 5.
The equation uses the following variables:
The equation includes the following variables:
Regarding the pressure in Figure 17A, the settlement values are 22 mm and 20 mm, respectively. Figure 17B shows the bearing capacity curves of Section B, indicating relatively higher load-bearing capacity compared to Section A. Figures 18A, B illustrates the ABC and settlement curves associated with section C, precisely the SPT-N values ranging from 14 to 50. The highest recorded values of ABC for square and strip footings are approximately 155 kPa and 120 kPa, respectively, at a depth of 1.5 m. The maximum consolidation settlement that can occur under this pressure is 24 mm, with a possibility of exceeding this value by 20 mm only in rare circumstances.
In every geotechnical section of the research area, the shallow foundation’s bearing capacity at 1.5 m depth is greater than 100 kPa and agreed with the findings of (Hussaini and Hozeh, 2021a).
This implies that the foundation can withstand a load of dense structures without experiencing any failures unless unforeseen exceptional circumstances arise. The examination of the soil profile indicates the presence of clearly defined layers, and a deeper groundwater table below 30 m reinforces the observation that the soil is less prone to settling. Buildings in Kabul are commonly constructed on foundations, which occasionally necessitate the use of soil improvement techniques to enhance the cost-effectiveness of the project. In order to achieve this, the foundations are usually built. Furthermore, foundation design engineers can adapt and refine foundation design in order to achieve optimal results. This is accomplished by decreasing the factor of safety (FOS) from 3 to 2.5 for buildings that do not have significant loads.
Based on the findings mentioned earlier, it is recommended that a shallow foundation be constructed with a depth of 1.5 m for a structure that undergoes minimal loading in all three sections. The study recommends using isolated footings with 1 m by 1 m or 1.5 m by 1.5 m for small-storey buildings. On the other hand, strip footings with a depth of 1 m are considered sufficient. With the given bearing capacities, the anticipated settlement of clay layers will not surpass 25 mm by the suggested dimensions for small structures. During foundation construction, if the base of the foundation comes across soft or weak soil and fill material, it is crucial to completely replace the foundation with coarse material with a maximum acceptable content of 10%. This is a limitation of the bearing capacity curves.
The coarse fill material will be compacted to achieve a minimum threshold of 95% of the modified Proctor maximum dry density. Engineers and geologists possess the ability to precisely ascertain the bearing capacity of lightly loaded structures, resulting in time and cost savings. To verify the curves, the bearing capacity of 15 distinct sites was assessed by comparing the actual bearing capacity with different dimensions of footings. Subsequently, the obtained results were juxtaposed with the anticipated load-bearing capacity of a footing with identical dimensions and depth. Figure 19 depicts a comparison between the estimated and actual bearing capacity (Alshameri, 2020). Approximately 85 percent of the predicted bearing capacity values exhibited a significant error, with the majority falling within a confidence interval of ±10 percent. These curves apply to structures with low-weight loads. Nevertheless, the extensive project and heavily burdened structure necessitate a comprehensive geotechnical investigation. The scope of this study was restricted to sensitive projects, resulting in limitations on including maps, soil parameters, and bearing capacity curves, which in turn constrained the extent of soil investigation.
5 Conclusion
The study thoroughly examines the extensive geotechnical data and spatial soil maps of the newly established city of Kabul. The analysis relies on the integration of SPT-N values and lithology data. According to the SPT-N assessment, the soil maps indicate that the study area was divided into three sections, while the lithology analysis identified six sub-sections. The SPT-N values for Section A, Section B, and Section C range from 8 to greater than 50, 13 to greater than 50, and 14 to greater than 50 for the top 15 m of soil.
The map displays lower SPT-N values to indicate the presence of alluvial deposits, whereas higher values indicate the presence of sandstone, shale, and gravel. The thickness of the fill material ranged from 1 to 2 m, with localized areas experiencing an increase of 2.5 to 3 m. After the fill material, a layer below shows compositional changes. This layer is characterized by a transition from a low to moderately flexible cohesive silty clay soil (SC) between 9 and 13 m in depth. The layers measuring 13–15 m are composed of silt and extremely pliable clay (CH). We have effectively established several linear relationships that can precisely forecast the SPT-N value. The R2 value of our models is 0.99, indicating a high level of accuracy.
Additionally, the RMSE is 5.8, which is considered low. The validity of empirical correlation equations was verified by conducting tests on 42 borehole SPT-N values extracted from geotechnical reports. Approximately 91% of the estimated SPT-N values fell within the confidence interval of ±10%. This indicates a minimal margin of error in the projected SPT-N values. The shallow foundation in New Kabul City was determined to have sufficient bearing capacity to support the structure’s foundation at a depth of 1.5 m. The square footing and strip footing exhibit a significantly high average bearing capacity, surpassing 145 and 110 kPa, respectively. This suggests that they are highly suitable for supporting the base of structures with minimal weight, ensuring exceptional levels of safety. The statistical analysis of the database shows that this study generates soil maps from a variety of uniformly distributed data ranges. This paper provides geotechnical maps for desk studies, initial designs, hazard recognition, feasibility studies, and extensive geotechnical soil investigations. Maps, soil parameters, and bearing capacity curves limit sensitive projects’ soil investigations.
6 Limitations and recommendations for future works
The reliability of the maps can be affected by data gaps, and their precision is contingent upon the availability and caliber of soil exploration data. SZMs authentication and reliability could be enhanced by conducting additional investigations in the subject region and gathering more subsoil data. The study’s findings are unique to the examined area; thus, they might not immediately apply to other places with different geological environments and circumstances. Other geotechnical variables which might influence the overall evaluation are excluded from the study, including underground water situations, long-term soil behavior, dynamic variables, soil physio-chemical and chemical features (i.e., pH, chloride, sulphate, EC, TDS, and organic content), and environmental effects.
To offer a more thorough geotechnical evaluation, future research may investigate incorporating other characteristics such as durability, dynamic behaviors, chemical properties, aquifer situations, and environmental consequences. It is advised to use sophisticated interpolation techniques, such as algorithms based on machine learning, which can improve the precision of spatial predictions and effectively record localized fluctuations. More reliable tools for planning might come through modeling the periodic shifts in geotechnical characteristics caused by buildings, catastrophes, or cyclic environmental variables. Enhancing soil data to create comprehensive risk assessment models for landslides, flooding, and earthquakes will increase awareness and disaster prevention.
Data availability statement
The original contributions presented in the study are included in the article/supplementary material, further inquiries can be directed to the corresponding author.
Author contributions
MA: Methodology, Conceptualization, Data curation, Formal Analysis, Investigation, Project administration, Resources, Software, Validation, Visualization, Writing–original draft. LD: Conceptualization, Methodology, Visualization, Funding acquisition, Supervision, Writing–review and editing. WH: Conceptualization, Formal Analysis, Methodology, Resources, Writing–review and editing, Data curation, Visualization. FZ: Data curation, Project administration, Software, Writing–review and editing, Resources. AZ: Data curation, Methodology, Resources, Writing–review and editing, Conceptualization, Visualization. AS: Formal Analysis, Validation, Visualization, Writing–review and editing.
Funding
The author(s) declare that financial support was received for the research, authorship, and/or publication of this article. This study was sponsored by the National Key and Program of China (Grant No. 2022YFC3003403) and the National Science Foundation of China (Grand numbers: 42220104005 and 41877245).
Acknowledgments
Thank you to the National Key Laboratory and Program of China and the National Science Foundation of China for their generous funding, which helped us complete our study.
Conflict of interest
The authors declare that the research was conducted in the absence of any commercial or financial relationships that could be construed as a potential conflict of interest.
Publisher’s note
All claims expressed in this article are solely those of the authors and do not necessarily represent those of their affiliated organizations, or those of the publisher, the editors and the reviewers. Any product that may be evaluated in this article, or claim that may be made by its manufacturer, is not guaranteed or endorsed by the publisher.
References
Abdul-husain, H. A. (2016). Comparison of theoretical ultimate bearing capacity of cohesionless soils with experimental and field data. J. Babylon. Univ. Sci. 24, 596–603.
Abdullah, S., and Chmyriov, V. M. (1977). Geologiya I poleznye iskopaemye afganistana, kniga 1. Geol. Moscow: Nedra.
Ahmed, C., Mohammed, A., and Tahir, A. (2020). Geostatistics of strength, modeling and GIS mapping of soil properties for residential purpose for Sulaimani City soils, Kurdistan Region, Iraq. Model. Earth Syst. Environ. 6, 879–893. doi:10.1007/s40808-020-00715-y
Akbarimehr, D., and Aflaki, E. (2019). Site investigation and use of artificial neural networks to predict rock permeability at the Siazakh Dam, Iran. Q. J. Eng. Geol. Hydrogeol. 52, 230–239. doi:10.1144/qjegh2017-048
Akhter, K., Khan, A. J., Khan, Q., and Asim, M. (2014). “Soil bearing capacity modelling A case study on islamabad and rawalpindi,” in Proceedings of second international conference on modern trends in science engineering and technology, 135–139.
Al-Ani, H., Eslami-Andargoli, L., Oh, E., and Chai, G. (2013a). Categorising geotechnical properties of surfers Paradise soil using geographic information system (GIS). Int. J. GEOMATE 5, 690–695. doi:10.21660/2013.10.3114a
Al-Ani, H., Oh, E., Eslami-Andargoli, L., and Chai, G. (2013b). Subsurface visualization of peat and soil by using GIS in surfers paradise, southeast queensland, Australia. Electron. J. Geotech. Eng. 18, 1761–1774.
Alcaras, E., Amoroso, P. P., and Parente, C. (2022). The influence of interpolated point location and density on 3D bathymetric models generated by kriging methods: an application on the Giglio Island seabed (Italy). Geosci 12, 62–23. doi:10.3390/geosciences12020062
Alcaras, E., Falchi, U., and Parente, C. (2020). Digital terrain model generalization for multiscale use. Int. Rev. Civ. Eng. 11, 52–59. doi:10.15866/irece.v11i2.17815
Al-Mamoori, S. K., Jasem Al-Maliki, L. A., Al-Sulttani, A. H., El-Tawil, K., Hussain, H. M., and Al-Ansari, N. (2020). Horizontal and vertical geotechnical variations of soils according to USCS classification for the city of an-najaf, Iraq using GIS. Geotech. Geol. Eng. 38, 1919–1938. doi:10.1007/s10706-019-01139-x
Alshameri, B. (2020). Maximum dry density of sand–kaolin mixtures predicted by using fine content and specific gravity. SN Appl. Sci. 2, 1693–1697. doi:10.1007/s42452-020-03481-9
Amin, H., and Helmi, M. (2021). Impacts of land-use transformation on agriculture land in Afghanistan, kabul city as case study. Int. J. Environ. Sci. Sustain. Dev. 6, 52–62. doi:10.21625/essd.v6i1.791
Amini, M., Deng, L., Hassan, W., Nawaz, M. N., Zidane, F. Z., and Fang, R. (2024). Integrative geospatial analysis: unveiling insights through GIS modelling and statistical evaluation of SPT-N and soil types data of new kabul city, Afghanistan. Adv. Civ. Eng. doi:10.3389/feart.2024.1460169
Arshid, M. U., and Kamal, M. A. (2020). Regional geotechnical mapping employing kriging on electronic geodatabase. Appl. Sci. 10, 7625. doi:10.3390/app10217625
Arun, P. V. (2013). A comparative analysis of different DEM interpolation methods. Egypt. J. Remote Sens. Sp. Sci. 16, 133–139. doi:10.1016/j.ejrs.2013.09.001
ASTM D 1586 (2022). Standard test method for standard penetration test (SPT) and split-barrel sampling of soils. Am. Soc. Test. Mater. doi:10.1520/D1586_D1586M-18E01
ASTM D 2166-98a (2000). Standard test method for unconfined compressive strength of cohesive soils. Am. Soc. Test. Mater. doi:10.1520/D2166-00
ASTM D 2435-04 (2011). Standard test methods for one-dimensional consolidation properties of soils using incremental loading. Am. Soc. Test. Mater. doi:10.1520/D2435-04
ASTM D2487 (2017). Standard practice for classification of soils for engineering purposes (unified soil classification system).
ASTM D422 (2007). Standard test method for particle-size analysis of soils. Astm D422-63, 1–8. doi:10.1520/D0422-63R07
ASTM D4318-17 (2010). Standard test methods for liquid limit, plastic limit, and plasticity index of soils. Available at: https://www.astm.org/Standards/D4318 (Accessed February 14, 2018).
ASTM-D698 (2021). “Standard test methods for laboratory compaction characteristics of soil using standard effort (12 400 ft-lbf/ft 3 (600 kN-m/m3)),” in ASTM international, 100 barr harbor drive, 700. West Conshohocken, PA, USA: PO Box C. PA19428-2959, United States. doi:10.1520/D0698-12R21
Bhattacharjee, S., Mitra, P., and Ghosh, S. K. (2013). Spatial interpolation to predict missing attributes in GIS using semantic kriging. IEEE Trans. Geosci. Remote Sens. 52, 4771–4780. doi:10.1109/tgrs.2013.2284489
Burrough, P. A., McDonnell, R. A., and Lloyd, C. D. (2015). Principles of geographical information systems. USA: Oxford University Press.
Cabalar, A. F., Karabas, B., Mahmutluoglu, B., and Yildiz, O. (2021). An IDW-based GIS application for assessment of geotechnical characterization in Erzincan, Turkey. Arab. J. Geosci. 14, 2129. doi:10.1007/s12517-021-08481-6
Cambardella, C. A., Moorman, T. B., Novak, J. M., Parkin, T. B., Karlen, D. L., Turco, R. F., et al. (1994). Field-scale variability of soil properties in central Iowa soils. Soil Sci. Soc. Am. J. 58, 1501–1511. doi:10.2136/sssaj1994.03615995005800050033x
Chabala, L. M., Mulolwa, A., and Lungu, O. (2017). Application of ordinary kriging in mapping soil organic carbon in Zambia. Pedosphere 27, 338–343. doi:10.1016/s1002-0160(17)60321-7
Chang, T. J., and Wang, W. L. (2008). “Spatial analysis using gis to study performance of highway culvers in Ohio,” in Pipelines 2008: pipeline asset management: maximizing performance of our pipeline infrastructure, 1–10.
Chiles, J.-P., and Delfiner, P. (2012). Geostatistics: modeling spatial uncertainty. John Wiley and Sons.
Chung, J.-W., and Rogers, J. D. (2010). GIS-based virtual geotechnical database for the St. Louis metro area. Environ. Eng. Geosci. 16, 143–162. doi:10.2113/gseegeosci.16.2.143
Collett, S., Faryad, S. W., and Mosazai, A. M. (2015). Polymetamorphic evolution of the granulite-facies paleoproterozoic basement of the kabul Block, Afghanistan. Mineral. Petrol. 109, 463–484. doi:10.1007/s00710-015-0371-9
Daniyal, M., Sohail, G. M., and Rashid, H. M. A. (2022). GIS-based mapping of geotechnical and geophysical properties of lahore soils.
Daniyal, M., Sohail, G. M., and Rashid, H. M. A. (2023). GIS-based mapping of geotechnical and geophysical properties of Lahore soils. Environ. Earth Sci. 82, 540. doi:10.1007/s12665-023-11201-w
Dell’Arciprete, D., Bersezio, R., Felletti, F., Giudici, M., Comunian, A. P. R., and Renard, P. (2012). Comparison of three geostatistical methods for hydrofacies simulation: a test on alluvial sediments. Hydrogeol. J. 20, 299–311. doi:10.1007/s10040-011-0808-0
Dodagoudar, G. R. (2018). An integrated geotechnical database and GIS for 3D subsurface modelling: application to Chennai City, India. Appl. Geomatics 10, 47–64. doi:10.1007/s12518-018-0202-x
Eberly, S., Swall, J., Holland, D., Cox, B., and Baldridge, E. (2004) Developing spatially interpolated surfaces and estimating uncertainty, NC 27711. Research Triangle Park: US Environmental Protection Agency, 1–160. Report No. EPA-454/R-04-004.
Erdogan, S. (2009). A comparision of interpolation methods for producing digital elevation models at the field scale. Earth Surf. Process. Landforms 34, 366–376. doi:10.1002/esp.1731
Fatima, B., Alshameri, B., Hassan, W., Maqsood, Z., Jamil, S. M., and Madun, A. (2023). Sustainable incorporation of Plaster of Paris kiln dust for stabilization of dispersive soil: a potential solution for construction industry. Constr. Build. Mater. 397, 132459. doi:10.1016/j.conbuildmat.2023.132459
Fesefeldt, K. (1964). Das Paläozoikum im Gebiet der oberen Logar und im östlichen Hazarajat südwestlich Kabul, Afghanistan. Beih. Geol. Jb. 70, 185–227.
Hassan, J., Alshameri, B., and Iqbal, F. (2022a). Prediction of California bearing ratio (CBR) using index soil properties and compaction parameters of low plastic fine-grained soil. Transp. Infrastruct. Geotechnol. 9, 764–776. doi:10.1007/s40515-021-00197-0
Hassan, W., Ahmad, M., Farooq, A., Ajwad, A., Ali, H. Q., and Ilyas, Y. (2017). Correlation of maximum laboratory dry density and optimum moisture content of soil with soil parameters. NFC-IEFR J. Eng. Sci. Res. 5. doi:10.24081/nijesr.2016.1.0003
Hassan, W., Alshameri, B., Haider, A., Maqsood, Z., Muhammad, S., and Shahzad, A. (2023a). A novel technique for the construction industry to mitigate dispersibility and internal erosion problems of sodium rich clays by using Water-Soluble potassium rich ions material. Constr. Build. Mater. 400, 132780. doi:10.1016/j.conbuildmat.2023.132780
Hassan, W., Alshameri, B., Maqsood, Z., Haider, A., Jamil, S. M., and Mujtaba, H. (2023b). An innovative application of fine marble dust for the construction industry to mitigate the piping, internal erosion and dispersion problems of sodium-rich clays. Constr. Build. Mater. 408, 133834. doi:10.1016/j.conbuildmat.2023.133834
Hassan, W., Alshameri, B., Muhammad, S., Maqsood, Z., Haider, A., and Shahzad, A. (2023c). Incorporating potassium-rich waste material in a sustainable way to stabilize dispersive clay: a novel practical approach for the construction industry. Constr. Build. Mater. 400, 132717. doi:10.1016/j.conbuildmat.2023.132717
Hassan, W., Alshameri, B., Nawaz, M. N., Ijaz, M. I., and Qasim, M. (2022b). Geospatial and statistical interpolation of geotechnical data for modeling zonation maps of Islamabad, Pakistan. Environ. Earth Sci. 81, 547. doi:10.1007/s12665-022-10669-2
Hassan, W., Alshameri, B., Nawaz, M. N., and Qamar, S. U. (2022c). Experimental study on shear strength behavior and numerical study on geosynthetic-reinforced cohesive soil slope. Innov. Infrastruct. Solut. 7, 349. doi:10.1007/s41062-022-00945-2
Hassan, W., Farooq, K., Mujtaba, H., Alshameri, B., Shahzad, A., Naqeeb, M., et al. (2023d). Experimental investigation of mechanical behavior of geosynthetics in different soil plasticity indexes. Transp. Geotech. 39, 100935. doi:10.1016/j.trgeo.2023.100935
Hassan, W., Qasim, M., Alshameri, B., Shahzad, A., Khalid, M. H., and Qamar, S. U. (2024). Geospatial intelligence in geotechnical engineering: a comprehensive investigation into SPT-N, soil types, and undrained shear strength for enhanced site characterization. Bull. Eng. Geol. Environ. 83, 380. doi:10.1007/s10064-024-03884-7
Hassan, W., Raza, M. F., Alshameri, B., Shahzad, A., Khalid, M. H., and Nawaz, M. N. (2023e). Statistical interpolation and spatial mapping of geotechnical soil parameters of District Sargodha, Pakistan. Bull. Eng. Geol. Environ. 82, 37–23. doi:10.1007/s10064-022-03059-2
Huang, Y., Li, Z., Ye, H., Zhang, S., Zhuo, Z., Xing, A., et al. (2019). Mapping soil electrical conductivity using Ordinary Kriging combined with Back-propagation network. Chin. Geogr. Sci. 29, 270–282. doi:10.1007/s11769-019-1027-1
Hussaini, M. S., and Hozeh, N. (2021a). “The zonation mapping of soil allowable bearing capacity for kabul city urban area using standard penetration test in GIS environment,” in Kabul polytech, 14–28. Afghanistan: Kabul Polytechnical university Available at: https://www.researchgate.net/publication/355772121.
Hussaini, M. S., and Hozeh, N. (2021b). The zonation mapping of soil allowable bearing capacity for kabul city urban area using standard penetration test in GIS environment. Kabul Polytech. 1.
Ijaz, Z., Zhao, C., Ijaz, N., Rehman, Z. ur, Ijaz, A., and Junaid, M. F. (2023). Geospatial modeling of heterogeneous geotechnical data using conventional and enhanced conception of modified Shepard method-based IDW algorithms: application and appraisal. Bull. Eng. Geol. Environ. 82, 428. doi:10.1007/s10064-023-03435-6
Jardaneh, I. (2007). Geotechnical map for the city of Nablus-Palestine. An-Najah Univ. J. Res. Nat. Sci. 21, 201–219. doi:10.35552/anujr.a.21.1.578
Jian, X., Olea, R. A., and Yu, Y.-S. (1996). Semivariogram modeling by weighted least squares. Comput. Geosci. 22, 387–397. doi:10.1016/0098-3004(95)00095-x
JICA (2009). The development of the master plan for kabul metropolitan area development in the islamic republic of Afghanistan.
Khalid, M. H., Alshameri, B., and Abid, U. (2021). Application of Kriging for development of SPT N value contour maps and USCS-based soil type qualitative contour maps for Islamabad, Pakistan. Environ. Earth Sci. 80, 413–13. doi:10.1007/s12665-021-09720-5
Kieft, P., Aquino, M., and Dodson, A. (2014). “Using ordinary kriging for the creation of scintillation maps,” in Mitigation of ionospheric threats to GNSS: an appraisal of the scientific and technological outputs of the TRANSMIT project. (IntechOpen).
Kolat, Ç., Doyuran, V., Ayday, C., and Lütfi Süzen, M. (2006). Preparation of a geotechnical microzonation model using geographical information systems based on multicriteria decision analysis. Eng. Geol. 87, 241–255. doi:10.1016/j.enggeo.2006.07.005
Lam, N. S.-N. (1983). Spatial interpolation methods: a review. Am. Cartogr. 10, 129–150. doi:10.1559/152304083783914958
Li, H., He, Y., Xu, Q., Deng, J., Li, W., and Wei, Y. (2022). Detection and segmentation of loess landslides via satellite images: a two-phase framework. Landslides 19, 673–686. doi:10.1007/s10346-021-01789-0
Li, H., He, Y., Xu, Q., Deng, J., Li, W., Wei, Y., et al. (2023). Sematic segmentation of loess landslides with STAPLE mask and fully connected conditional random field. Landslides 20, 367–380. doi:10.1007/s10346-022-01983-8
Li, Y., Fan, W., Chen, X., Liu, Y., and Chen, B. (2017). Safety criteria and standards for bearing capacity of foundation. Hindawi Math. Probl. Eng. 2017, 1–8. doi:10.1155/2017/3043571
Meyerhof, G. G. (1951). The ultimate bearing capacity of foudations. Geotechnique 2, 301–332. doi:10.1680/geot.1951.2.4.301
Meyerhof, G. G. (1970). Safety factors in soil mechanics. Can. Geotech. J. 7, 349–355. doi:10.1139/t70-047
Meyerhof, G. G. (1985). Safety factors and limit states analysis in geotechnical engineering: reply. Can. Geotech. J. 22, 145. doi:10.1139/t85-016
Mitas, L., and Mitasova, H. (1999). Spatial interpolation. Geogr. Inf. Syst. Princ. Tech. Manag. Appl. 1, 481–492.
Mohammed, A. H., Yahya, A. Y., and Ahmed, B. A. (2012). Database for Baghdad soil using GIS techniques. J. Eng. 18, 1307–1324. doi:10.31026/j.eng.2012.12.02
Mohurd, G. (2012). Code for design of building foundation, GB. Beijing, China: China Archit. Build. Press, 50007–52011.
Nasir, M. J., Khan, S., Ayaz, T., Khan, A. Z., Ahmad, W., and Lei, M. (2021). An integrated geospatial multi-influencing factor approach to delineate and identify groundwater potential zones in Kabul Province, Afghanistan. Environ. Earth Sci. 80, 453. doi:10.1007/s12665-021-09742-z
Nawaz, M. M., Khan, S. R., Farooq, R., Nawaz, M. N., Khan, J., Tariq, M. A. U. R., et al. (2022a). Development of a cost-based design model for spread footings in cohesive soils. Sustainability 14, 5699. doi:10.3390/su14095699
Nawaz, M. N., Akhtar, A. Y., Awan, T. A., Nawaz, M. M., Qamar, S. U., Shehzad, T., et al. (2024a). Multivariate formulation to predict the frictional strength of fiber reinforced soils using gene expression programming. Eng. Appl. Artif. Intell. 134, 108660. doi:10.1016/j.engappai.2024.108660
Nawaz, M. N., Alshameri, B., Maqsood, Z., and Hassan, W. (2024b). Predictive modelling of cohesion and friction angle of soil using gene expression programming: a step towards smart and sustainable construction. Neural comput. Appl. 36, 10545–10566. doi:10.1007/s00521-024-09626-w
Nawaz, M. N., Chong, S. H., Nawaz, M. M., Haider, S., Hassan, W., and Kim, J. S. (2023a). Estimating the unconfined compression strength of low plastic clayey soils using gene-expression programming. Geomech. Eng. 33, 1–9. doi:10.12989/gae.2023.33.1.001
Nawaz, M. N., Haseeb, M., Qamar, S. U., Hassan, W., and Shahzad, A. (2024c). Gene expression programming-based multivariate model for earth infrastructure: predicting ultimate bearing capacity of rock socketed shafts in layered soil-rock strata. Model. Earth Syst. Environ. 10, 5241–5256. doi:10.1007/s40808-024-02061-9
Nawaz, M. N., Khan, M. H. A., Hassan, W., Jaffar, S. T. A., and Jafri, T. H. (2024d). Utilizing undisturbed soil sampling approach to predict elastic modulus of cohesive soils: a Gaussian process regression model. Multiscale Multidiscip. Model. Exp. Des. 7, 4255–4270. doi:10.1007/s41939-024-00458-8
Nawaz, M. N., Nawaz, M. M., Awan, T. A., Jaffar, S. T. A., Jafri, T. H., Oh, T. M., et al. (2023b). A sustainable approach for estimating soft ground soil stiffness modulus using artificial intelligence. Environ. Earth Sci. 82, 579. doi:10.1007/s12665-023-11193-7
Nawaz, M. N., Qamar, S. U., Alshameri, B., Karam, S., Çodur, M. K., Nawaz, M. M., et al. (2022b). Study using machine learning approach for novel prediction model of liquid limit. Buildings 12, 1551. doi:10.3390/buildings12101551
Nawaz, M. N., Qamar, S. U., Alshameri, B., Nawaz, M. M., Hassan, W., and Awan, T. A. (2022c). A robust prediction model for evaluation of plastic limit based on sieve # 200 passing material using gene expression programming. PLoS One 17, 1–19. doi:10.1371/journal.pone.0275524
Nawaz, M. N., Yar Akhtar, A., Hassan, W., Hasnain Ayub Khan, M., and Muneeb Nawaz, M. (2024e). Artificial intelligence-based prediction models of bio-treated sand strength for sustainable and green infrastructure applications. Transp. Geotech. 46, 101262. doi:10.1016/j.trgeo.2024.101262
Orhan, A., and Tosun, H. (2010). Visualization of geotechnical data by means of geographic information system: a case study in Eskisehir city (NW Turkey). Environ. Earth Sci. 61, 455–465. doi:10.1007/s12665-009-0357-1
Ouma, Y. O., Owiti, T., Kipkorir, E., Kibiiy, J., and Tateishi, R. (2012). Multitemporal comparative analysis of TRMM-3B42 satellite-estimated rainfall with surface gauge data at basin scales: daily, decadal and monthly evaluations. Int. J. Remote Sens. 33, 7662–7684. doi:10.1080/01431161.2012.701347
Pham, T. G., Kappas, M., Huynh, C. V., and Nguyen, L. H. K. (2019). Application of ordinary kriging and regression kriging method for soil properties mapping in hilly region of central Vietnam. ISPRS Int. J. Geo-Information 8, 147. doi:10.3390/ijgi8030147
Rasouli, H. (2020). Application of soil physical and chemical parameters and its Comparing in Kabul Sedimentary basins, Kabul, Afghanistan. Int. J. Recent Sci. Res. 11, 37368–37380. doi:10.24327/ijrsr.2020.1102.5095
Rasouli, H., and Safi, A. G. (2021). Geological, soil and sediment studies in Chelsaton sedimentary basin, Kabul, Afghanistan. Int. J. Geosci. 12, 170–193. doi:10.4236/ijg.2021.122011
Rasouli, H., and Vaseashta, A. (2023). Investigation of physicochemical properties of Qalay Abdul Ali soil, kabul, Afghanistan. Adv. Geol. Geotech. Eng. Res. 5, 55–68. doi:10.30564/agger.v5i3.5773
Rishikeshan, C. A., Katiyar, S. K., and Vishnu Mahesh, V. N. (2014). “Detailed evaluation of dem interpolation methods in GIS using DGPS data,” in Proceedings - 2014 6th international conference on computational intelligence and communication networks, CICN 2014. Bhopal, India, 14-16 November 2014, (IEEE), 666–671. doi:10.1109/CICN.2014.148
Robinson, T. P., and Metternicht, G. (2003). A comparison of inverse distance weighting and ordinary kriging for characterising within-paddock spatial variability of soil properties in Western Australia. Cartography 32, 11–24. doi:10.1080/00690805.2003.9714231
Saffi, M. H. (2011). Groundwater natural resources and quality concern in Kabul Basin, Afghanistan. Kabul: DACAAR Kabul.
Safi, M. W. A., Siddiqui, S., Tariq, A., Rehman, N. U., and Haider, S. W. (2020). GIS based universal soil erosion estimation in district chakwal Punjab, Pakistan. Int. J. Econ. Environ. Geol. 11, 30–36. doi:10.46660/ijeeg.vol11.iss2.2020.443
Salekin, S., Burgess, J. H., Morgenroth, J., Mason, E. G., and Meason, D. F. (2018). A comparative study of three non-geostatistical methods for optimising digital elevation model interpolation. ISPRS Int. J. Geo-Information 7, 300–315. doi:10.3390/ijgi7080300
Shafique, M. A., and Qayyum, T. I. (2011). Comparison of bearing capacity equations for vertical central loading. First Int. Conf. Geotechnique, Constr. Mater. Environmen, 583–588.
Shamal, S., and Rasouli, H. (2018). Comparison between pH, EC, CaCO3 and mechanical analysis of Qala Wahid and company areas soil, kabul, Afghanistan. Int. J. Sci. Res. 8, 429–433.
Shi, C., and Wang, Y. (2021a). Development of subsurface geological cross-section from limited site-specific boreholes and prior geological knowledge using iterative convolution XGBoost. J. Geotech. Geoenvironmental Eng. 147, 4021082. doi:10.1061/(asce)gt.1943-5606.0002583
Shi, C., and Wang, Y. (2021b). Nonparametric and data-driven interpolation of subsurface soil stratigraphy from limited data using multiple point statistics. Can. Geotech. J. 58, 261–280. doi:10.1139/cgj-2019-0843
Shi, C., and Wang, Y. (2021c). Smart determination of borehole number and locations for stability analysis of multi-layered slopes using multiple point statistics and information entropy. Can. Geotech. J. 58, 1669–1689. doi:10.1139/cgj-2020-0327
Shi, C., and Wang, Y. (2022). Data-driven construction of Three-dimensional subsurface geological models from limited Site-specific boreholes and prior geological knowledge for underground digital twin. Tunn. Undergr. Sp. Technol. 126, 104493. doi:10.1016/j.tust.2022.104493
Shill, S. K., and Hoque, M. (2015). Comparison of bearing capacity calculation methods in designing shallow foundations. Gazipur, Bangladesh.
Skempton, A. W. (1986). Standard penetration test procedures and the effects in sands of overburden pressure, relative density, particle size, ageing and overconsolidation. Geotechnique 36, 425–447. doi:10.1680/geot.1986.36.3.425
Sulyman, M., Noori, A., and Al-Attar, A. (2020). “Study and GIS-based mapping of soil chemical properties in kirkuk city, Iraq,” in Proceedings of the 1st international multi-disciplinary conference theme: sustainable development and smart planning. IMDC-SDSP 2020, Cyperspace, 28-30 June. doi:10.4108/eai.28-6-2020.2298163
Suwanwiwattana, P., Chantawarangul, K., Mairaing, W., and Apaphant, P. (2001). “The development of geotechnical database of bangkok subsoil using GRASS-GIS,” in 22nd asian conference on remote sensing (Singapore), 5–9. Available at: http://citeseerx.ist.psu.edu/viewdoc/summary?doi=10.1.1.20.6119.
Taharin, M. R., and Roslee, R. (2021). The application of semi variogram and ordinary Kriging in determining the cohesion and clay percentage distribution in hilly area of Sabah, Malaysia. Int. J. Des. Nat. Ecodynamics 16, 525–530. doi:10.18280/ijdne.160506
Tapponnier, P., Mattauer, M., Proust, F., and Cassaigneau, C. (1981). Mesozoic ophiolites, sutures, and arge-scale tectonic movements in Afghanistan. Earth Planet. Sci. Lett. 52, 355–371. doi:10.1016/0012-821x(81)90189-8
Terzaghi, K., and Peck, R. B. (1967). Soil mechanics in engineering practice. New York: New york, USA: John Wiley and Sons, Inc.
Terzaghi, K., Peck, R. B., and Mesri, G. (1996). Soil mechanics in engineering practice. New york, USA: John Wiley & Sons.
Teves-Costa, P., Almeida, I. M., Rodrigues, I., Matildes, R., and Pinto, C. (2014). Geotechnical characterization and seismic response of shallow geological formations in downtown Lisbon. Ann. Geophys. 57. doi:10.4401/ag-6390
Tobler, W. R. (1970). A computer movie simulating urban growth in the Detroit region. Econ. Geogr. 46, 234–240. doi:10.2307/143141
Ullah, H., Imtiaz, K., Jahanzaib, R., and Zhang, G. (2022). Geotechnical characterization and statistical evaluation of alluvial soils of Lahore. Arab. J. Geosci. 15, 1–12. doi:10.1007/s12517-022-10154-x
Vansarochana, A., Tripathi, N. K., and Clemente, R. (2009). Finding appropriate interpolation techniques for topographic surface generation for mudslide risk zonation. Geocarto Int. 24, 313–332. doi:10.1080/10106040802547735
Wackernagel, H., and Wackernagel, H. (2003). Ordinary kriging. Multivar. geostatistics Introd. Appl, 79–88. doi:10.1007/978-3-662-05294-5_11
Wahl, R. R., and Bohannon, R. G. (2005). “The digital geologic map of Afghanistan,” in Digital mapping techniques ‘05—workshop proceedings, 131.
Wang, Y., and Zhao, T. (2016). Interpretation of soil property profile from limited measurement data: a compressive sampling perspective. Can. Geotech. J. 53, 1547–1559. doi:10.1139/cgj-2015-0545
Wang, Y., Zhao, T., Hu, Y., and Phoon, K.-K. (2019). Simulation of random fields with trend from sparse measurements without detrending. J. Eng. Mech. 145, 4018130. doi:10.1061/(asce)em.1943-7889.0001560
Waters, M. N. (1989). “Spatial interpolation I, lecture 40,” in NCGIA core curriculum, tech. Issues GIS. St. Barbar: Univ. California, 40–43.
Woods, N., Karimi, M., Jafari, I., and Hinchliffe, R. (2022). Geological and geotechnical investigations for the Salang highway, Afghanistan. Q. J. Eng. Geol. Hydrogeol. 55, qjegh2021–189. doi:10.1144/qjegh2021-189
Wu, T., and Li, Y. (2013). Spatial interpolation of temperature in the United States using residual kriging. Appl. Geogr. 44, 112–120. doi:10.1016/j.apgeog.2013.07.012
Zaryab, A., Najaf, M. I., and Jamal, M. Z. (2019). Analysis of engineering properties of rock mass of Shah-wa-Arus dam site, Kabul, Afghanistan. Cent. Asian J. Water Res. Центральноазиатский журнал исследований водных ресурсов 5, 18–28. doi:10.29258/cajwr/2019-r1.v5-1/18-28.eng
Zaryab, A., Nassery, H. R., and Alijani, F. (2022). The effects of urbanization on the groundwater system of the Kabul shallow aquifers, Afghanistan. Hydrogeol. J. 30, 429–443. doi:10.1007/s10040-021-02445-6
Keywords: statistical interpolation, soil type, geotechnical investigation reports, SPT-N value, bearing capacity, and regional database
Citation: Amini M, Deng L, Hassan W, Zidane FZ, Zaryab A and Shahzad A (2024) Empowering urban development: geospatial modeling and zonation mapping in New Kabul City, Afghanistan. Front. Earth Sci. 12:1460169. doi: 10.3389/feart.2024.1460169
Received: 05 July 2024; Accepted: 14 October 2024;
Published: 30 October 2024.
Edited by:
Hans-Balder Havenith, University of Liège, BelgiumReviewed by:
Ming Zhang, China University of Geosciences Wuhan, ChinaHuajin Li, Chengdu University, China
Copyright © 2024 Amini, Deng, Hassan, Zidane, Zaryab and Shahzad. This is an open-access article distributed under the terms of the Creative Commons Attribution License (CC BY). The use, distribution or reproduction in other forums is permitted, provided the original author(s) and the copyright owner(s) are credited and that the original publication in this journal is cited, in accordance with accepted academic practice. No use, distribution or reproduction is permitted which does not comply with these terms.
*Correspondence: Longsheng Deng, ZGxzaEBjaGQuZWR1LmNu