- 1Institute of Environmental Management, Faculty of Earth Science, University of Miskolc, Miskolc, Hungary
- 2Geology Department, Faculty of Science, Beni-Suef University, Beni-Suef, Egypt
- 3Desert Research Center, Division of Water Resources and Arid Land, Hydrogeochemistry Department, Cairo, Egypt
- 4Faculty of Earth science, Beni-Suef University, Cairo, Egypt
- 5Industria Engineering Department, College of Engineering, King Saud University, Riyadh, Saudi Arabia
- 6INFN, Laboratori Nazionali di Frascati, Frascati, Italy
Introduction: The groundwater in arid countries such as Egypt represent the main water resources in the desert regions due to the long distance between these regions (oasis) and Nile River. Contamination of these limited water resources with toxic metals threaten the health of individuals in these regions.
Methods: The current study integrates isotopic tracers, hydrogeochemistry, geophysical logs, positive matrix factorization (PMF model), and Monte Carlo (MCS) simulation for pollution source apportionment and health risks associated with heavy metals in the Nubian Sandstone aquifer (NSSA).
Results and Discussion: The water resource used for drinking purposes (NSSA) is pale meteoric water (non-rechargeable aquifer). Silicate weathering, old trapped sea water, reverse ion exchange evaporation, and dissolution are the dominant mechanisms controlling water chemistry. PMF model showed that the major ions and heavy metals in groundwater of the NSSA originated from four significant sources (anthropogenic activities, dissolution of minerals, iron-bearing minerals, mixing with old trapped seawater, and hydrothermal water). The total risk (HI) oral values highlighted significant non-carcinogenic dangers for adults and children through the oral exposure. At the same time, dermal contact posed a no risk for adults and a high risk for children. Most samples had carcinogenic risk (CR) values higher than the allowed limits (1.0E-4) for metals like Cadmium, chromium, and lead, suggesting carcinogenic effects across all age groups. The Monte Carlo approach-based health concern evaluation model assessed the 5th % CR oral values (child) as 0.00012, 0.0036, and 0.0088 for Cd, Cr, and Pb, respectively, indicating more significant potential dangers to children. Urgent and comprehensive water treatment measures are imperative to mitigate the identified carcinogenic and non-carcinogenic health risks in the study area.
Introduction
Groundwater is a critical resource for people’s survival and growth, and its purity has a significant influence on human wellness, food availability, and environmental stability (Liu R. et al., 2021; Abanyie et al., 2023; Al-Mashreki et al., 2023; Eid et al., 2023; 2024a). Global population growth, urbanization, economic development, and long-term agricultural activities contributed to groundwater quality degradation, raising widespread concern (Gaagai et al., 2023; Gad et al., 2023; Ibrahim et al., 2023; Salem et al., 2023; Eid et al., 2024b). Contaminating groundwater exposes humans to harmful chemicals through direct consumption and skin contact, posing a significant health risk (Jaydhar et al., 2023). According to WHO (WHO, 2017), contaminated drinking water affects about 500,000 people worldwide yearly. As a result, assessing the state of groundwater is critical to ensuring public safety and appropriate water management (Eid et al., 2024c; Klimach and Zębek, 2024).
Finding and monitoring contamination sources helps formulate effective environmental policies (Liu W. et al., 2021). Nonetheless, groundwater quality is regularly changing due to natural sources, such as water-rock interaction, and human interference, such as home sewage, agricultural, and chemical plant discharges (Guo et al., 2018; Ligate et al., 2021). The combination of naturally occurring and anthropogenic effects creates significant challenges for source identification (Sheng et al., 2022).
Researchers have lately adopted multivariate statistical evaluation approaches such as Absolute Principal Component Scores-Multiple Linear Regression (APCS-MLR), Positive Matrix Factorization (PMF), and Unmix to solve these issues (Li et al., 2020; Zhang et al., 2020a; 2020b). While the APCS-MLR approach is practical, it requires a substantial amount of samples, which raises the expense of investigation (Cheng et al., 2020; Hu et al., 2024). The Unmix approach is vulnerable to outliers and missing values in the dataset, limiting its utility (Kwak and Kim, 2017; Li et al., 2020). In contrast, the PMF technique allows for uncertainty in changing concentrations, reducing the influence of analytical error. The PMF model was originally developed for analyzing the sources of particulate matter in the atmosphere and has been endorsed and refined by the Environmental Protection Agency. Over time, it has seen extensive use in the study of groundwater, soil, and sediment (Chen et al., 2022; Ren et al., 2023; Zhang et al., 2023). Furthermore, the PMF technique enables the rotation of the initially collected data, resulting in more precise attributions of source factors. Previous research has used these models to identify the principal sources of organophosphate (Qi et al., 2021). The results showed that the PMF approach performed better than the other two types of models. Furthermore, Zhang (Zhang et al., 2020a) employed the PMF and APCS-MLR approaches to identify groundwater pollutant sources in China’s Chengdu Plain. Researchers concluded that the PMF technique produced more reliable source apportionment estimates. Salim and Li (Salim et al., 2019; Li et al., 2021) found similar findings.
Based on several results applied globally to detect the source of contamination using PMF, Zanotti made integration between PMF and isotopes for samples collected from surface water and groundwater around Oglio River basin in Italy and confirmed that PMF could be effective tool for contamination source determination (natural and anthropogenic) (Zanotti et al., 2019). Zanotti could detect five contamination sources of major ions and traces (Fe, NO3−, Mn, and As) based on PMF and isotopic data analysis including surface water used for agriculture or irrigation, groundwater undergoing advanced-stage reduction processes, groundwater experiencing early-stage reduction processes, groundwater residence time, and the impact of agricultural land use on both groundwater and surface water (Zanotti et al., 2019). In China, Zhang used Random Forest with PMF for major ions and toxic elements (F−, As, and Cr6+) and revealed that the primary sources of pollution in the Minjiang River, in descending order, were agricultural activities (30.26%), domestic sewage (29.07%), and industrial wastewater (26.25%), along with minor contributions from seasonal factors, and soil erosion (Zhang et al., 2024). The PMF and PCA-MLR analysis identified five key sources of groundwater contamination in Mardan, Pakistan (Rashid et al., 2023): geochemical processes, industrial effluents, dissolution of sulfide and fluoride-bearing minerals, Fe and Mn dissolution, and agricultural pollution. This approach revealed that fluoride and arsenic contamination in groundwater is widespread and influenced by both natural and anthropogenic factors, with significant implications for public health and water management strategies. The global previous studies proved the efficiency to apply this technique in the current study.
The public health risk approach created by the United States EPA is widely used to assess environmental quality (EPA, 2004). It assesses the health hazards of various populations by calculating average daily exposure to pollutants (Zheng et al., 2020; Ruidas et al., 2023). However, the standard risk assessment calculates human health risk values using predefined parameters, which may not adequately reflect a given group’s exposure risk (Kumar and Singh, 2020). For example, different adults have diverse body weights and water intake rates. Toxic Metals to humans, such as cadmium, zinc, lead, chromium, iron, manganese, nickel, and copper, cause particular risks to people of all ages, primarily through ingestion and skin exposure. These toxic elements can infiltrate the human system via direct consumption or skin contact (EPA, 2004). Moreover, they may be absorbed by flora and fauna, subsequently making their way into the human body through dietary pathways. As these elements build up and intensify within the body, they pose risks to human health, potentially leading to a range of both non-cancerous and cancerous health conditions (Chai et al., 2021; Li et al., 2023). Oral contact with toxic metals such as cadmium, lead, and copper, for example, can cause kidney dysfunction, neurotoxicity, and gastrointestinal problems. Skin exposure to specific metals can cause skin irritation and, in some circumstances, allergic reactions (Loredana Ungureanu and Mustatea, 2022). The researchers can develop effective techniques to reduce contamination and protect water resources by investigating toxic metal levels, sources, and behavior within groundwater systems.
Estimating their exposure hazard based on daily water consumption and average body weight can result in substantial uncertainty and ambiguity. To solve this limitation, Monte Carlo simulation can provide a more precise estimate of contaminants’ health risks (Yu et al., 2022; Li et al., 2024). Monte Carlo simulation evaluates the likelihood of risk occurrence using several random iterations, resulting in a more exact estimation of the range and distribution of human health risks and this probabilistic method was used globally in several studies (Chang et al., 2022; Saeed et al., 2023a; 2023b; Eid et al., 2024b; 2024d; Vesković et al., 2024). Although a Monte Carlo model is frequently used to evaluate health risks from hazardous components in soil, it is rarely employed in groundwater quality studies (Huang et al., 2021). As a result, researchers combined the PMF and Monte Carlo models to increase the accuracy and efficiency of determining acceptable contaminant concentration limits (Ren et al., 2023; Eid et al., 2024e; 2024b; Li et al., 2024). This strategy offers a novel solution to the limitations of current risk assessment methodologies, resulting in understanding of the possible health risks linked to pollutants. Recent study applied Monte Carlo Simulation to detect the health risk of PTEs in Siwa Oasis in different aquifer system with different geological and flow system (TCA). This study was performed by Eid that confirmed that the shallow aquifer that mainly used for irrigation purposes and contain brackish water was contaminated with several PTEs that originate from both natural and anthropogenic source. Eid proved with probabilistic method (MCS) that there is high carcinogenic risk for adult and children through ingestion and dermal contact with estimated CR>1*10−4 and high non-carcinogenic risk for child through oral contact (Eid et al., 2024b). Eid couldn’t investigate the risk of PTEs in the main deep fresh water aquifer (Nubian Sandstone) in Siwa Oasis which is used mainly for drinking and could have direct risk to individuals of different age group.
The Siwa Oasis, situated in Egypt’s western desert, depends heavily on groundwater for potable and irrigation. Because of the scarcity of freshwater streams, the oasis is sustained by a combination of surface water (lakes and drains) and groundwater, which is vital to its survival (Aly, 2020; Hamdy Eid et al., 2022). Groundwater is sourced from hot springs and boreholes in aquifers such as the Nubian sandstone (NSSA) and shallow aquifers (Tertiary carbonate aquifer, TCA). This underground water provides a variety of uses, including agriculture, drinking, and domestic use. Four salt lakes in the lowlands or depressions of the Siwa Oasis region get their water from cultivated land drainage, hot springs, and boreholes that penetrate the aquifer systems (Abdulaziz and Faid, 2015a; Eid et al., 2024a). Groundwater demand in the study area (Siwa Oasis) has increased over time due to agricultural activities, tourist attractions, and the economy’s expansion. Consequently, multiple wells have been constructed. However, the haphazard installation of these boreholes, along with an excessive amount of groundwater extraction, endangers water pressure and quality (Abdel-Mogheeth, 1996; Aly, 2001; Abdallah, 2007; Abou El-Magd and Faid, 2007; Aly and Benaabidate, 2010; El Hossary, 2013; Aly et al., 2016). Illegal groundwater drilling worsens these challenges, emphasizing the significance of researching to monitor the amount and quality of limited water resources. Studying hydrochemistry, stable isotopes, and heavy metals in groundwater is critical for sustainable water resource management, safe and reliable water supplies, and protecting human and environmental health, especially in Siwa Oasis.
As a result, the objectives of this research were to: 1) determine the recharge source and mechanisms that drive the enrichment of different elements and contaminants in groundwater; 2) detect potential factors influencing groundwater quality and determine their contributions; 3) application of positive matrix factorization model (PMF) for pollution source apportionment of heavy metals and its contribution percentage; and 4) assess the possible adverse health effects of metals exposure to various people of different age groups (child and adults). This study is the first source-oriented health concern evaluation of the Nubian aquifer (NSSA), and it provides critical insights and reference information for the optimal mitigation and management of groundwater pollution.
Materials and methods
Study area
Location and climate
Siwa Oasis is a topographical depression (−18 m BSL) in Egypt’s western desert (the northern part of the western desert). It is Located at longitude 25°43′E and latitude 29°12′N (Figure 1). The Mediterranean Sea is 320 kmKm from Siwa, the Great Sand Sea in the south, and Cairo is 560 Kmkm away (Abdulaziz and Faid, 2015a). The total area of the Oasis is approximately 1100 kkm2 (Aly et al., 2016), and it has an estimated population of 23,000 (Moez and Younan, 2016). Agriculture is the primary economic activity at Siwa Oasis, which includes palm tree cultivation, olive oil production, and a variety of vegetables and fruits. Industrial activities like mineral water bottling and olive oil extraction significantly contribute to the local economy (Abou El-Magd and Faid, 2007; Aggour and Faid, 2007; Elnaggar et al., 2016). Siwa Oasis has an arid climate with a substantial evaporation rate of 16.8 mm/d, which decreases to around 5.4 mm per day during the winter. Insufficient precipitation (around 10 mm) contributes to the parched conditions. This distinct climatic environment, the oasis’s seclusion, and limited supply of water presents obstacles for people and businesses alike (Abdulaziz and Faid, 2015a).
Hydrogeology
Siwa Oasis’ geology consists of different hydrostratigraphic layers (Figure 2A), including deposits like dunes and salt flats (quaternary) and older Middle Eocene layers comprised of limestone and shale. Layers from other geological ages, including the Palaeozoic, Mesozoic, and Cainozoic, are also present (Abdallah, 2007; Elnaggar et al., 2016). Siwa’s water resources are extracted mainly from two principal aquifers (deep NSSA and shallow TCA). The Miocene aquifer (Tertiary carbonate aquifer) serves agricultural and domestic requirements, whereas the Nubian sandstone aquifer (NSSA) is mainly used for drinking water and irrigation (Afifi, 2005; Aggour and Faid, 2007; El Hossary, 2013; Abdulaziz and Faid, 2015a). Soil salinization and waterlogging around salt lakes such as Zeitoun (eastern Siwa lake), Aghormi, Siwa (central Siwa lakes) and Maraqi Lake (western lake) highlight the significance of efficient water resource management in ensuring the sustainability of oasis water sources while addressing environmental challenges (Misak et al., 1997; Abdallah, 2007; El Hossary, 2013). The groundwater flow direction based on the hydraulic head from 27 wells penetrating NSSA (Figure 2B) is South east- northwest (SW-NE) and South west-north east. There is a cone of depression in the central part of Siwa Oasis due to intensive agricultural activities requiring the extraction of groundwater for irrigation and drinking.
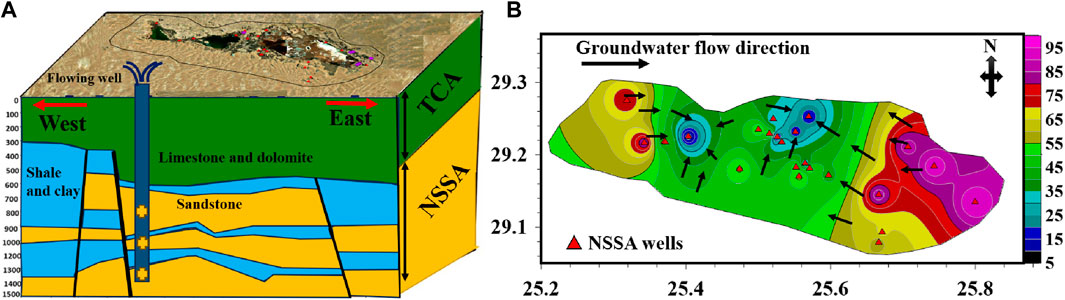
Figure 2. Hydrostratigraphic units of the aquifer systems (A), and groundwater flow direction showing the cone of depression in the central part of Siwa Oasis (B) modified after (Eid et al., 2024b).
Sampling and analysis
In 2022, an intensive trip was conducted to collect 27 samples from springs and NSSA. These samples were carefully preserved in polyethylene bottles for further investigation. The chemical analysis including major ions and trace elements was conducted at desert research center in Egypt and stable isotopes were performed in University of Miskolc in Hungary.
During the investigation, portable meters were used to measure essential physicochemical characteristics such as pH and electrical conductivity. Flame photometry was used to quantify alkaline metal ions, specifically Na+ and K+. The hardness (TH) was determined using EDTA methods, while CO32− and HCO3− were examined volumetrically. Ion chromatography was used to measure NO3− and SO42−enrichment. The Mg2+ concentration was determined using the TH and Ca2+ concentrations. Accurate chloride levels were determined using AgNO3 titration. The Inductively Coupled Plasma (ICP) method was used to investigate heavy metal concentrations. This analytical technique gives precise assessments of heavy metal content in water samples.
The stable tracers’ measures were expressed as δ 2H and δ 18O in per mil (‰) deviation of the 18O/16O and 2H/1H ratio in the standard formula termed as Vienna Standard Mean Ocean Water (VSMOW), with δ 18O and δ 2H values of 0‰ (Gat and Gonfiantini, 1981). The Institute for Geological and Geochemical Research used a Los Gatos Research Liquid-Water Isotope Analyser-24d to conduct isotope analysis on water samples. The instrument utilizes off-axis integrated cavity ring-down spectroscopy to estimate the absolute levels of 2H1H16O, 1H1H16O, and 1H1H18O by laser absorption. We used laboratory standards calibrated with international standard (δ2H = −147.7‰; −74.9‰; and −9.0‰; δ 18O = −19.95‰; −10.41‰ and −0.53‰; for BWS1, BWS2, and BWS3) (Czuppon et al., 2018). Precisions were greater than 0.15‰ and 1.0‰ for δ 18O and δ 2H, respectively.
Quality assurance and control
The water quality analysis followed the standard methodology specified by the American Public Health Association (APHA) in 2012 [38]. To ensure the accuracy of on-site testing equipment, we carefully standardized all instruments with deionized water and buffer solutions before starting sample analysis. Various quality assurance procedures were applied during the water sample examination. The analytical processes were validated by instrument calibration, accuracy, and predictability evaluations. Charging balance errors (CBE) were evaluated following field observations and then validated in the laboratory. The samples were examined in triplicate, and the average values were also given. Equation 1 was used to analyze anion-cation balance errors based on the principle of neutrality, which states that the sum of the number of cations equals the sum of the number of anions in meq/L. The CBE for all examined samples was within the permissible range of ±5%.
Furthermore, the quality assurance of the analytical procedure was double-checked through a meticulous examination blank technique analysis. The different metal contents in the sample solutions were obtained using a curve for calibration. To calibrate the device, a 50-mL (mL) intermediate standard was employed as an operational standard for toxic metals. When the correlation coefficient could be more than 0.999, it indicated that the relationship was strong. The measured amount of every metal in the collected sample was determined using interpolation of calibration curves. Every examination was conducted in triplicate.
The methods employed were validated by performing limit of detection and quantification (LOD and LOQ), accuracy, precision, and recovery testing based on the following equations below;
The variance and % of relative standard deviation (RSD) were used to determine the results’ accuracy and precision. The RSD of triplicate readings for every single sample was applied to calculate precision as described in the equation below;
Positive matrix factorization model (PMF)
The PMF model determines the contributions of several factors in a mixture. The basic bilinear factorization divides a multivariate database into two matrix data, G and F. This provides a linear combination of the data set’s variability as a series of constant factor profiles, as well as the influence of each component on each sample (Haghnazar et al., 2022). The model’s computational methodology uses weighted least squares to minimize the objective function Q for various factors (p). Q is defined as follows (Equation 2):
Q is the total of the squares of the difference between actual (X) and modeled (GF), weighted by the uncertainty of measurement (σij). σij represents the degree of uncertainty of the species j concentration data in the ith sample; xij represents the observed concentration for the jth chemical species in the ith sample; and gik represents the kth factor contribution to the ith sample. Fik represents the jth factor profile of factor kth. The EPA PMF User Guide (Brown et al., 2015) provides additional information on PMF principles.
PMF requires two input files (Lee et al., 2002; Arruti et al., 2011): the measured sample concentration and the related uncertainty file.
For concentrations below the detection limit (Equations 3, 4):
For concentrations higher than the detection limit (Equations 5–7):
Regarding missing values (Equation 8):
Here, xij is the concentration value of the variable measured in the sample; dij is the minimal detection limit (Supplementary Table S1); σij is the uncertainty of the related concentration. cij is the observed concentration of the water samples, while cij is the geometric mean of the observed concentration.
Hydrogeochemical and geophysical methods
The hydrogeochemical evaluation and recharge source were conducted using graphs and ionic ratios, including δ 18O Vs. δ 2H, Mg2+/Na+ vs. Ca2+/Na+(Gaillardet et al., 1999; Mukherjee and Fryar, 2008), a Sulin graph (Sulin, 1946), spearman correlation matrix (Hauke and Kossowski, 2011), and the chlor-alkaline index CAI-I (Schoeller, 1977) (Equation 9). An Excel sheet was used for the calculation and visualization.
Geophysical methods, including gamma ray (GR), resistivity (R), spontaneous potential (SP), and lithological logs (Supplementary Figure S1), were used to delineate the aquifer system and detect reliable geological composition and geogenic source of the dissolved ions or contaminants based on the practical investigation (Figure 3). Interpolation using the IDW method and Surfer software were used for spatial variation of the heavy metal’s concentration in the study area.
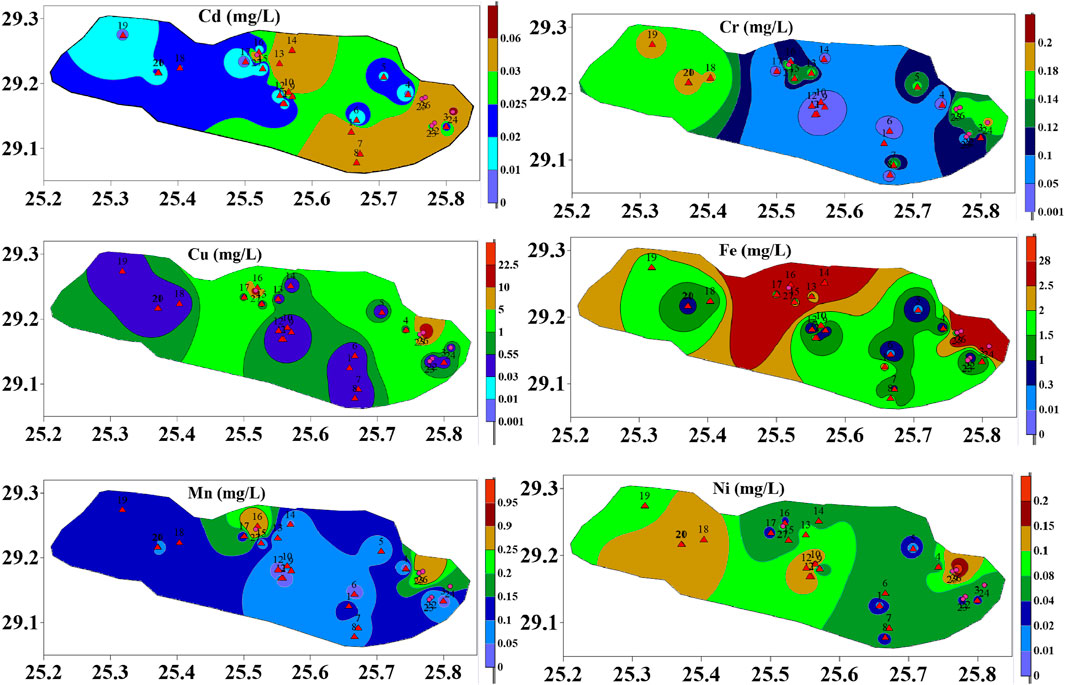
Figure 3. Distribution map and interpolation of metals in the groundwater samples from NSSA and springs.
Human health risks (HHR)
The human health hazards in this investigation are evaluated utilizing an approach approved by the US Environmental Protection Agency (EPA, 2004). This risk analysis extensively explores how environmental pollutants (heavy metals) affect human health. The identified hazards are classed as carcinogenic risk (CR) or non-carcinogenic risk (NCR) (Habib et al., 2020; Eid et al., 2024e; Gad et al., 2024; Saeed et al., 2024). Carcinogenic hazards relate to the risk of developing cancer as a result of prolonged exposure to a pollutant or a combination of contaminants. Non-carcinogenic risk, on the other hand, focus on exposure issues such as genetic and teratogenic effects. In this study, heavy metals (HMs) identified in drinking water sources (NSSA) are predominantly absorbed by the human body through food and skin contact (Gade et al., 2021). The mathematical calculation of the risk indices was performed using Equations 10 and 11. By considering different exposure pathways, the study provides a thorough knowledge of the potential health concerns associated with contaminants such as heavy metals in water supplies for drinking, providing significant insights for risk management and public health protection.
Here, C, IR, ED, BW, CF, SA, Kp, EF, ET, AT parameters or reference numbers represent element concentration in the water sample (mg/L), daily ingestion rate (L/day), exposure duration (year), body weight (kg), conversion factor (L/cm3), exposed skin area (cm2), skin permeability coefficient (cm/h), exposure frequency (day/year), contact duration (h/day), Average exposure time (day), The value of IR, ED, BW, CF, SA, Kp, EF, ET, AT are reported with references (Stein, 1975; Wu et al., 2009; Liang et al., 2011; Phillips and Moya, 2013; Giri and Singh, 2015; Saleem et al., 2019; Adimalla, 2020; Meng et al., 2023; Alam et al., 2024) in Supplementary Table S1.
Carcinogenic the health risk was categorized as non-carcinogenic or carcinogenic after calculating CDI oral and CDI dermal for chosen elements. The hazard quotient value (HQ) for, each component and the total health hazard for all metals combined together (non-carcinogenic risk) were determined using these equations (Equations 12–14):
The Reference Dose (RfD) is a crucial metric that represents the quantity of a chemical that an individual can be exposed to daily without significantly increasing the risk of undesirable health effects. ABS is used to calculate absorption efficiency in the digestive tract (Table 1).
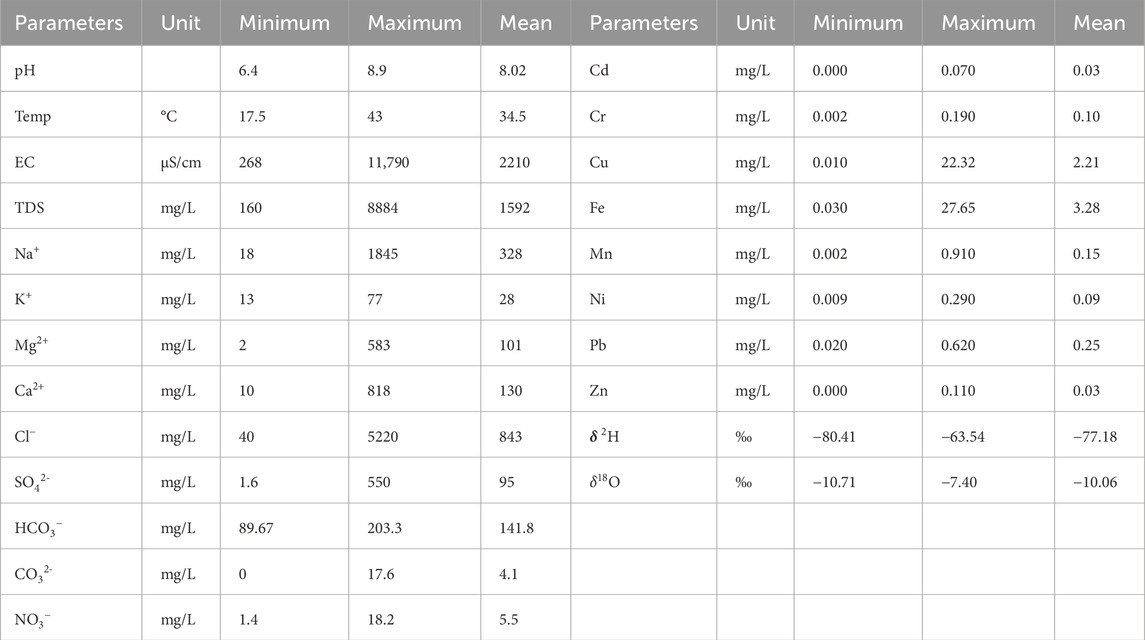
Table 1. The investigated physical parameters, major ions, heavy metals, and isotopic tracers in the water samples.
To calculate the potential for cancer by using the carcinogenic risk (CR) index from inhalation directly or skin exposure, the following equation (Equation 15) was used:
CSF is the cancer slope factor for the individual heavy metal (refer to Table 1).
Monte Carlo simulation (MCS) model
The Monte Carlo simulation approach is a probabilistic technique often used when selecting variables for risk analysis of uncertainty and risk assessment. The method formulates assumptions based on variable definitions, creates a probability model of the chosen parameters, defines the cells chosen for prediction, and uses the results of various random simulation experiments as an approximate solution to the problem to calculate the risk value (Hsu, 2010; Mohammadi et al., 2022; Mohammadpour et al., 2023). The uncertainty in health risk assessment arises mainly from exposure characteristics (such as body weight, consumption, and skin surface area), differences in pollutant levels between research areas, and the choice of health risk assessment model. These factors influence the calculation of the actual risk value, resulting in uncertainty in the risk evaluation (Chen et al., 2019; 2021). Supplementary Table S1 provides the selected parameters. This study employs the Monte Carlo method to model eight heavy metals’ carcinogenic and non-carcinogenic health hazards and conduct an uncertainty risk analysis. The Python code was developed for conducting Monte Carlo simulations, utilizing the language’s ability to run 10,000 iterations. This extensive iteration approach covers a wide range of potential scenarios, resulting in a more complete understanding of the possible risks of the investigated metals.
Results and discussion
Statistics and chemical analysis
Hydrochemistry was assessed by analyzing physical parameters, major ions, heavy metals, and isotopic tracers, as detailed in Table 1. The groundwater salinity regarding TDS value in the examined samples varied greatly, ranging from 160 mg/L in the Nubian Sandstone aquifer (NSSA) to 8,884 mg/L in the springs, with a mean of 1,592 mg/L pH values ranged between 6.4 and 8.9, denoting neutral and alkaline water conditions. Calcium (Ca2+) concentrations varied between 9.8 mg/L and 818 mg/L, with magnesium (Mg2+) values ranging from 2 mg/L in investigated samples to 583 mg/L in springs. Potassium ion (K+) levels ranged from 13 mg/L to 77 mg/L, whereas sodium ion (Na+) concentrations ranged between 18 mg/L and 1,845 mg/L. Chloride ion (Cl−) levels ranged between 40 mg/L and 5,220 mg/L, whereas sulfates (SO42-) concentrations varied from 1 mg/L to 550 mg/L. Bicarbonate ions (HCO3−) values were between 89 mg/L and 203 mg/L. The available water resources in the study area were categorized based on TDS values, with springs classified as brackish water and NSSA as freshwater. According to WHO standards (WHO, 2017), most physicochemical parameters in NSSA water samples were within drinking-safe limits, except for elevated K+ levels. Conversely, spring samples exceeded drinking limits for all major ions except for SO42- and HCO3−. The mean concentration values of metals were listed in the following sequence: Cu (2.21 mg/L), Cr (0.1 mg/L), Cd (0.03 mg/L), Pb (0.25 mg/L), Fe (3.28 mg/L), Ni (0.09 mg/L), Mn (0.15 mg/L), and Zn (0.03 mg/L) scored in descending sequence: Fe > Cu > Pb > Mn > Cr > Ni > Cd > Zn. In NSSA, average Fe, Pb, Cd, Mn, and Cr concentrations exceeded WHO (WHO, 2017) guidelines, while Cu, Ni, and Zn remained within limits. Conversely, all spring samples showed contamination with all heavy metals except Zn. The IDW method and Surfer 16 interpolation revealed concentration variations across Siwa Oasis. Cd, Fe, Mn, Zn, Ni, and Cu increased in the eastern and central part, while Cr and Pb showed maximum concentrations in the western part (Figure 3). Identifying the most affected locations is crucial for effective water treatment and management, especially considering the predominant use of NSSA and springs for drinking, irrigation, and domestic purposes in Siwa Oasis.
Groundwater’s origin and recharge source
Figure 4A uses the Sulin graph (Sulin, 1946; Eid et al., 2024b) to visually show the origin of water collected Nubian aquifer. Several studies used Sulin graph to determine the origin of the groundwater where the graph classify the groundwater into four types including recent marine, old marine water if the concentration (epm %) of Cl− > Na+ + K+ and deep meteoric and shallow meteoric water if the concentration Cl− > Na+ + K+ (Aref and Taj, 2017; Awadh et al., 2019; Zaghlool, 2020). The dominant water type or facies based on Sulin graph are CaCl2, MgCl2, Na2SO4, NaHCO3 for old marine, recent marine, old meteoric, and recent meteoric, respectively. Compared to piper diagram (Piper, 1944) that give more than two ions in the water type based on their order, Sulin graph indicate the most anion and cation or salt has significant signature in the water due to water rock interaction (Aref and Taj, 2017; Awadh et al., 2019; Zaghlool, 2020). This method has some limitations (Eid et al., 2024e) which can be solved or ignored by integration of stable isotopes with geological composition of the aquifer system and this is the case in the current study. The research showed that most (96.3%) of water samples in the Nubian Sandstone Aquifers (NSSA) are of old marine genesis, with a high CaCl2 content. In contrast, one sample from NSSA and one from springs are of recent marine water provenance, as evidenced by their MgCl2 compositions. The increased Na+ and K+ levels in these samples show that the water from the NSSA and springs is meteoric in origin. However, it is essential to note that all samples have a common marine origin, implying the presence of old trapped seawater in the aquifer system. The shallow aquifer above the NSSA, known as the Tertiary carbonate aquifers (TCA), is predominantly composed of deposits such as limestone and dolomite, indicating a marine origin. The springs’ water’s high salinity concentration reflects its source in the TCA, which is impacted by marine activity and mineral dissolution from rock formations. Considering this marine influence, the TCA appears to rely mostly on upward flow of deep NSSA, with limited contributions from rainfall in the Siwa Oasis region.
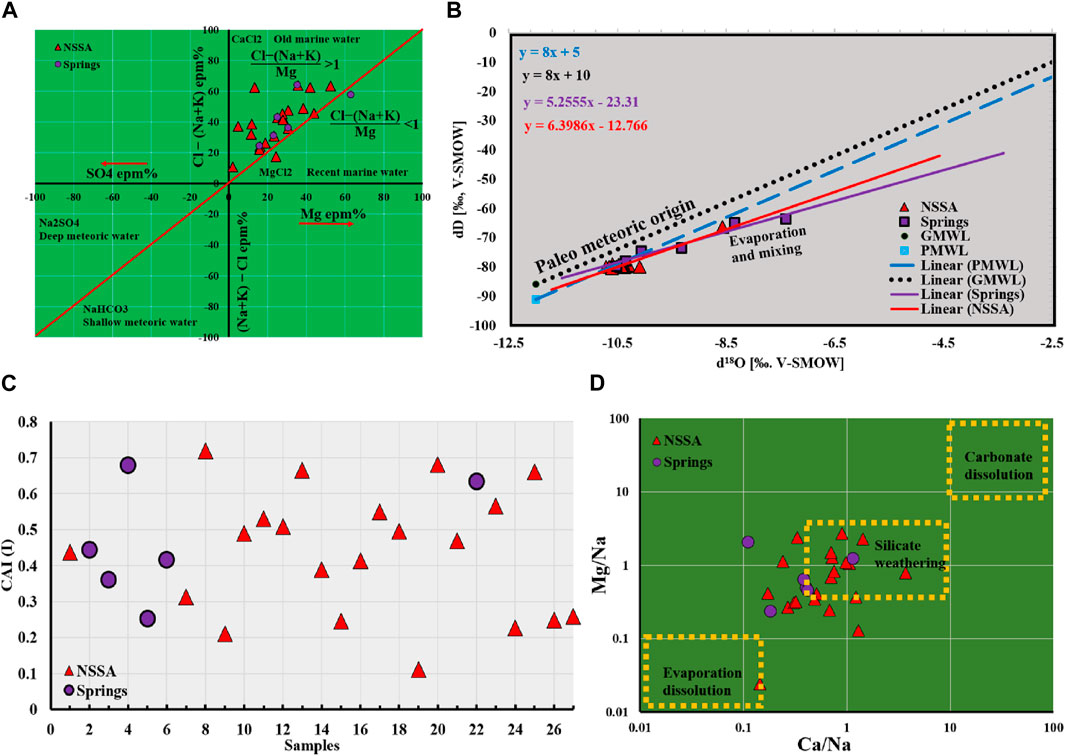
Figure 4. Sulin graph (A), dD vs d18O (B) showing the origin and recharge source in Siwa Oasis, chloro-alkaline index (CAI-I) (C) and a bivariate plotting of Mg2+/Na+ versus Ca2+/Na+ (D).
While the Sulin graph effectively detected the influence of marine deposits on groundwater origin, stable isotopes, specifically δ 2H, and δ 18O, were employed in the current study for more reliable confirmation of the recharge source. The water samples’ isotopic composition varied between −10.71 and −7.40‰ (average: −10.06‰) for δ 18O and −80.41 to −63.54‰ (average: −77.18‰) for δ 2H (Table 1). Springs had an enriched water isotopic composition compared to NSSA, which was attributed to a greater evaporation rate. Figure 4B depicts the relationship between δ 2H and δ 18O, paired with the Global Meteoric Water Line (GMWL) and Paleo Meteoric Water Line (PMWL), which were used to identify groundwater recharge sources and antecedent evaporation. The isotopic readings of groundwater samples collected in the lower right side of the GMWL show that atmospheric precipitation is their principal source.
Nevertheless, evaporation and water-rock interaction contribute to increasing heavy isotopes. Depletion of δ 2H and δ 18O indicates that NSSA and springs contain non-rechargeable paleowater (meteoric freshwater), with NSSA constituting the only source capable of replenishing TCA. Springs’ water is supplemented by evaporation and water-rock interaction and mixed with other water sources trapped in TCA, stressing the minimal importance of rainfall in recharging, with leached sediments of marine origin dominating the recharge zone. The opposite origin, as shown by the Sulin graph and stable isotopes, could be attributed to water-rock contact and ion exchange.
Geochemical processes controlling water chemistry
The challenging origin identified by the Sulin graph (old marine) and stable isotopes (old meteoric water) could be due to freshwater-rock interaction and ion exchanges/reverse ion exchange. Clay minerals can affect groundwater mineralization by facilitating ion exchange reactions. Clay minerals balance their charge by adsorbing monovalent cations such as Na+ and K+ and releasing Ca2+ and Mg2+, or vice versa. The chloro-alkaline index (Schoeller, 1967; Gaagai et al., 2023; Gad et al., 2023; Ibrahim et al., 2023; Salem et al., 2023) (CAI-I= Cl-(Na+K)/Cl) is a technique for determining ion exchange pathways among minerals in aquifers and groundwater. A positive CAI-I number suggests a reverse ion exchange process, while a negative value shows that ion exchange mechanisms govern water’s chemical composition. The Nubian aquifer consists of sandstone layers separated from each other by shale and clay layers. In this investigation, all samples had positive CAI-I readings (Figure 4C), showing that K+ and Na+ ions in water exchanged with Mg2+ and Ca2+ ions in the surrounding rock (silicate minerals).
Bivariate plots (Figure 4D) were employed to acquire insight into the mechanism and weathering type regulating water chemistry, focusing on the Ca2+/Na+ against Mg2+/Na+ ratio (Gaillardet et al., 1999; Mukherjee and Fryar, 2008). These plots (Figure 4D) demonstrated that silicate weathering influences the chemical composition of freshwater in NSSA. However, certain samples (springs) were found in zones related to evaporite dissolution caused by marine deposits in the TCA.
The water chemistry in the study area is influenced by several key processes. Silicate minerals in the aquifer contribute to the water’s ionic composition through weathering, as evidenced by the specific ratios of certain ions. The presence of high salinity and particular ions like sodium and chloride and the marine deposits of limestone and dolomite in the TCA suggests that ancient seawater is still affecting the aquifer which could be trapped in the fracture system, confirmed by isotopic analyses and the chemical profiles observed. The positive values in the chloro-alkaline index indicate that a process known as reverse ion exchange is occurring, where sodium and potassium in the water replace calcium and magnesium in the aquifer rocks, which is facilitated by the presence of clay layers. Evaporation plays a significant role, particularly in the springs, where it leads to the enrichment of heavy isotopes and higher concentrations of dissolved salts. Additionally, the dissolution of minerals, such as gypsum and anhydrite, further alters the water’s composition, contributing to elevated levels of calcium and sulfate. These findings demonstrate the combined impact of natural geological processes and the aquifer’s ancient origins on the region’s water quality.
Source apportionment and PMF model
Twelve chemical parameters (Na+, Cd, Fe, Cr, Ni, Mn, Ca2+, SO42-, Pb, NO3−, Mg2+, and Cl−) were implemented in the positive matrix factorization (PMF) model to identify anthropogenic and natural variables influencing groundwater chemical properties, as variables that are not expressed in concentration can’t be directly inputted (Zanotti et al., 2019; Li et al., 2021). After one hundred iterative computations, four components were identified by obtaining the smallest Q values. The robust Q-to-ideal Q value ratio was 1.4, demonstrating an appropriate number of components in this investigation (Huston et al., 2012; Li et al., 2021). We verified our findings by fitting observed and predicted results to typical contaminated water quality indicators (Figure 5). In Figure 5, two distinct lines are displayed in this manner: The one-to-one line represents theoretical fitting and R2 = 1, while the regression line represents actual fitting. A perfect fit would result in an overlap of the regression line with the one-to-one line. Results in Figure 4 show strong fits for the investigated parameters Mn (R2= 0.96), SO42‒ (R2= 0.95), Mg2+ (R2= 0.94), Ca2+ (R2=0.81), Cl− (R2=0.80), and Cd (R2=0.70).
To detect the primary sources based on a realistic approach, essential details about the study area, including field surveys and literature, were collected. Previous studies used 3D inversion of aerogravity and magnetic geophysical data for preliminary geothermal exploration and delineating depths to basement rocks in Siwa Oasis. The results indicate depths to Precambrian basement rocks commonly exceeding 2 km, ranging from 2 km to 5 km, with Curie Point Depth ranging from 21 kmkm to 28 km, alongside geothermal gradients ranging from 21°C to 27 °C/km and heat-flow values ranging from 49 to 64 mW/m2 (Abdel Zaher et al., 2018). The tertiary carbonate aquifer above the NSSA consists of marine sediments (limestone and dolomite intercalated with shale and clay) with brackish to saline water due to old trapped sea water (Eid et al., 2024a). The geophysical logs (GR, SP, R, and lithology) showed that the Nubian aquifer was mainly composed of sandstone layers separated by shale, silt, and clay with some iron oxides (Supplementary Figure S1). The previous study in Siwa Oasis showed that the water resources in the TCA (shallow aquifer) are supersaturated with hematite (oxidizing environment), and surface lakes are mixed with this aquifer (Eid et al., 2024a).
In contrast, NSSA was supersaturated with siderite (reducing environment) (Gad et al., 2018). The stable isotopes showed that NSSA is non-rechargeable, which indicates the only circulation in groundwater and enrichment of elements could be between NSSA and TCA due to the over-pumping for irrigation, domestic, and drinking purposes. As mentioned above, the main mechanisms controlling water chemistry are water-rock interaction (silicate weathering, evaporation, and dissolution). Most of the wells in Siwa Oasis were closed for water quality protection. Some wells penetrated some TCA layers with high salinity exceeding the WHO and FAO standards (Ayers and Westcott, 1994; WHO, 2017), and some wells made of lead material penetrating the NSSA were damaged because of corrosion. The old wells in Siwa Oasis constructed from lead pipes could be a potential source of Pb.
Regarding factors extracted from the PMF model, field survey, isotopes, hydrochemistry, geophysical logs, and literature (Mohamed et al., 2020; Hamdy Eid et al., 2022; Elnazer et al., 2023; Eid et al., 2024a; 2024b; 2024c), there are four primary sources of ions and heavy metals: geothermal source and old trapped sea water mixing source (factor 1), heavy metals bearing minerals (factor 2), rock weathering and dissolution or geogenic source (factor 3), and anthropogenic source from agricultural activity (factor 4). Figure 6 and Table 2 demonstrate The loading factors or factor profiles in water samples (Figures 6A–D) and factor’s fingerprint (Figure 6E) to show how the water quality indicator contributes to each factor based on the PMF model results.
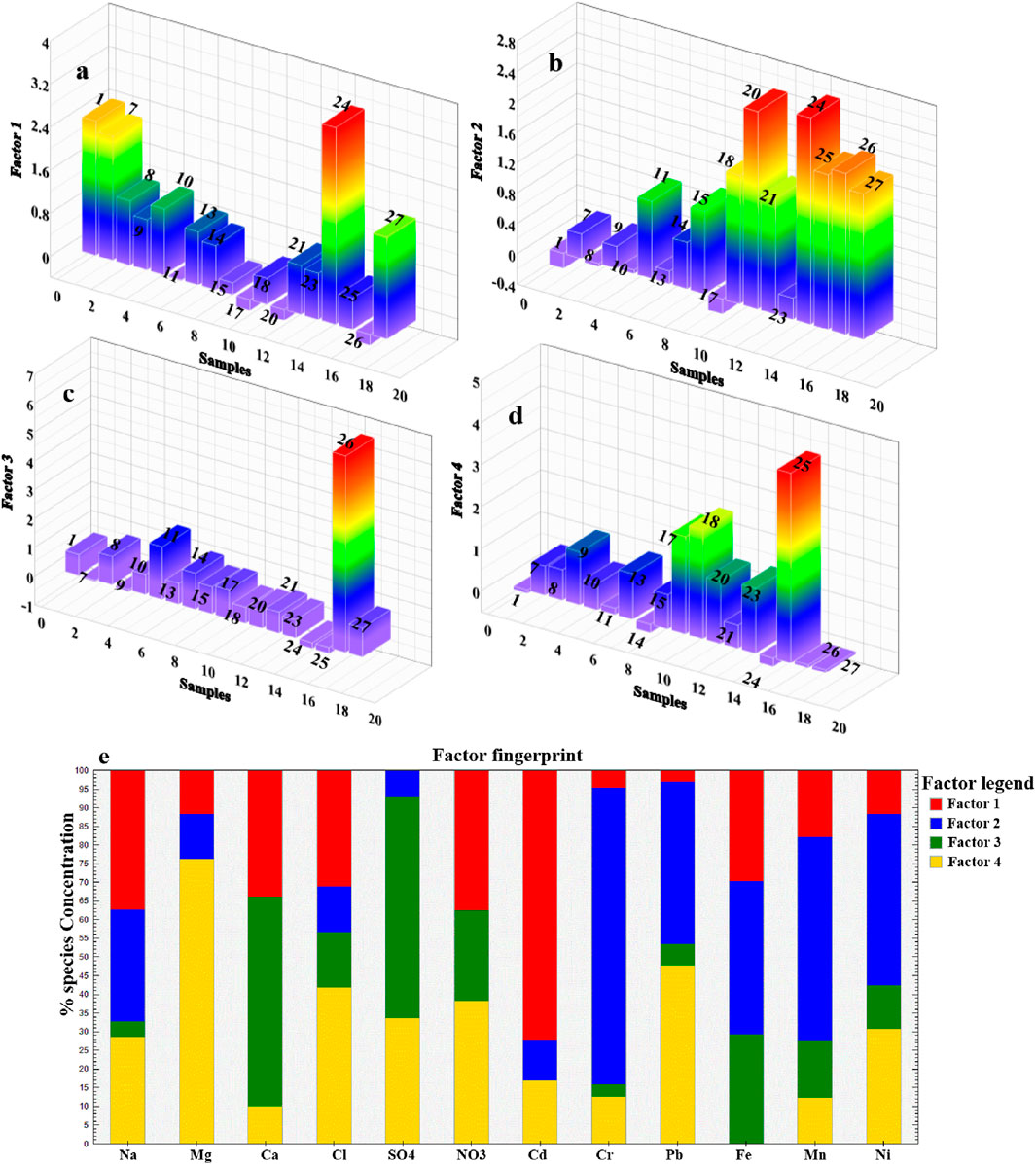
Figure 6. The loading factors or factor profiles in water samples (A–D) and factor’s fingerprint (E) to show how the water quality indicator contributes to each factor based on the PMF model results.
Na+ and Cd define the first factor (F1) with 37.3% and 72.2% contribution percentages, respectively. The upward flow of geothermal water from the deep layer through the fault plane and mixing with groundwater in the NSSA could be the source of Cd and Na+ and mixing with old trapped sea water in the aquifer system. The silicate weathering in the aquifer system could also increase Na+ concentration through ion exchange where the contribution percentage from factor 3 is close to factor 2 (29.9%). The Pearson correlation matrix (Table 3) between Na+ and Cd showed a negative coefficient value (−0.22) which suggest some other sources of Na+ including halite dissolution as it showed by strong positive coefficient value with Cl−.
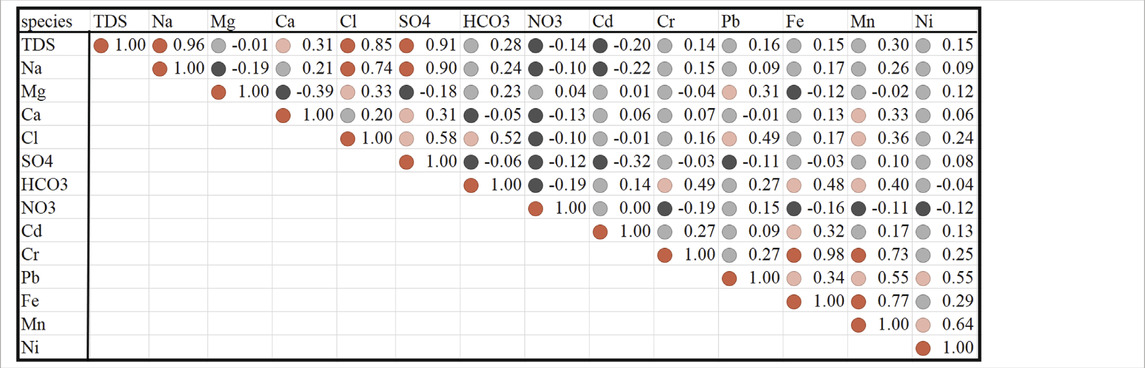
Table 3. The Pearson correlation matrix and the coefficient value between physical and chemical parameters.
The Second Factor included Fe, Mn, Cr, and Ni. The contribution percentage of species for factor 2 was 41%, 54.4%, 79.5%, and 46%, respectively. In sedimentary rocks, Ni and Cr is primarily associated with detrital ferromagnesian silicate minerals, detrital primary iron oxides, hydrous iron and manganese oxides, and clay minerals. Its concentration varies significantly across different types of sedimentary rocks: Ni is most abundant in shale, quarzitic and limestone (Loring, 1976; Yamamoto, 1988). Based on the geophysical logs, the geological composition of NSSA contains sandstone, shale, silt, and clay with traces of iron oxides. The source of these elements is heavy metals bearing minerals intercalated with shale and clay minerals such as siderite mineral as a source of Fe. In a reduced environment, the reduction of Fe and Mn could increase the mobility of Cr in water. The contribution percentage of Ni in factor 2 was close to that of factor 4, suggesting also anthropogenic activities from the use of nickel-containing fertilizers and pesticides in the agricultural land. The correlation matrix showed strong positive correlation between Mn and Fe, Cr and Fe, Mn and Ni with coefficient value 0.77, 0.98, and 0.64, respectively confirming the same results from the PMF model where the heavy metals or iron and manganese bearing minerals are the main source of Fe, Mn, Cr, and Ni in groundwater.
The third factor included SO42- and Ca2+, and the contribution percentage of species for factor 3 was 59.1% and 56.1%, respectively. The source of Calcium and sulfates in the groundwater could be rock weathering and mineral dissolution. The silicate weathering and dissolution of calcite and dolomite could increase the concentration of Ca ions. The dissolution of gypsum could add SO42- and Ca2+ in water, which was confirmed before through previous studies in the study area by using geochemical modeling, ionic ratio and subsurface geology where there are some evaporite minerals in the aquifer system increased the saturation index of gypsum and anhydrite in water (Abdulaziz and Faid, 2015b; Eid et al., 2024a; 2024c). Additionally, the current study showed that the water samples are mainly affected by evaporite dissolution and silicate weathering (Figure 4D) and the intermediate correlation between Ca2+ and SO42- indicates there are several sources of Ca2+ such as gypsum, anhydrite, and calcite dissolution. Dissolution of these minerals contribute to increasing and variation of the groundwater salinity in the study area which could be proved by strong correlation of TDS and SO42- (Table 3). Based on the correlation coefficient from the spearman correlation matrix, the Ca2+ has good correlation only with SO42- confirming the main source of Ca2+ could be gypsum and anhydrite dissolution.
The Fourth factor represents the anthropogenic source of pollution in the groundwater. Factor 4 included Pb, NO3−, Cl−, and Mg2+. The contribution percentage of species for factor 4 was 47.8%, 38.1%, 41.9%, and 76.3%, respectively. Although, the NSSA is non rechargeable aquifer due to the neglected precipitation in the study area, there is connection between NSSA and the TCA which could increase the anthropogenic activity in the deep aquifer. The over pumping from the NSSA decreased the pressure and water level (cone of depression) in the central part of Siwa Oasis which suggest downward flow from the TCA to NSSA and distribute in the aquifer based on the groundwater flow path. Anthropogenic activities can introduce lead (Pb), nitrate (NO3−), chloride (Cl−), and magnesium (Mg2+) into groundwater through various pathways. Agricultural activities, including the use of fertilizers, pesticides, and animal manure, can contribute to nitrate and chloride contamination in groundwater. Excessive application of nitrogen-based fertilizers can result in nitrate leaching into groundwater, while chloride from fertilizers and animal waste can also infiltrate groundwater. Additionally, magnesium-containing fertilizers and soil amendments can contribute to magnesium levels in groundwater. In Siwa Oasis, there is no drainage system where the local people use septic tanks and the agricultural drainage flow naturally under gravity. There are no protected pipes take the drainage water which increase the infiltration of contaminants such as Pb, NO3−, Cl−, and Mg2+ to the groundwater through the fracture system of TCA and hence to NSSA. The drilling of artificial canals and drains with permeable material in the floor increase the infiltration rate of these elements to the groundwater. The destruction of some old wells penetrating the NSSA due to corrosion pipes made of Pb could be a source of lead in the aquifer system. Improper disposal of domestic sewage and wastewater can result in the contamination of groundwater with lead, nitrate, chloride, and magnesium. Leakage from septic tanks, sanitary sewers, and wastewater treatment facilities can introduce these contaminants into the subsurface, where they may infiltrate groundwater. Previous studies confirmed that there is no lead forming minerals in the geological composition which indicate the only way for increasing Pb in water is the anthropogenic sources. Lead contamination is particularly concerning and can be attributed to historical practices and infrastructure issues (Tawfik et al., 2018; Eid et al., 2024b). The corrosion of old wells, especially those with lead pipes, is a notable source. As these pipes degrade, lead can leach into the water. Furthermore, improper disposal of domestic sewage and wastewater, potentially containing lead from household products, can contribute to groundwater contamination. These activities are ongoing, as the region lacks modern waste management systems, and agricultural practices continue without sufficient regulation or oversight. The combination of over-extraction of water from the NSSA, which creates a cone of depression, and the connection between the TCA and NSSA aquifers, allows these contaminants to migrate and affect the deeper water resources. The construction of artificial canals and drains with permeable materials further facilitates the infiltration of these pollutants, highlighting the need for improved management and infrastructure to protect groundwater quality. Some studies, such as Subba Rao (Subba Rao, 2021), have also indicated that anthropogenic activities contribute to the presence of Mg2⁺, and Cl− ions in groundwater. These ions can originate from the use of certain fertilizers and soil amendments, as well as from the leaching of these substances through agricultural runoff. Additionally, industrial processes, improper disposal of industrial waste can further elevate the levels of these ions in groundwater sources. The farmers in the study area still using different type of fertilizers (chemical and biofertilizers) and the most common fertilizers in the study area is NPK (Ibrahim et al., 2013) which could contribute significantly in the elevated concentration of NO3− in groundwater.
Based on the contribution and loading factors extracted from the positive matrix factorization model for all samples (Figures 6A–D), it was concluded that the samples such as S1, S7, S10, S13, S14, and S24 have more contribution from factor 1. The results of factor 2 showed high loading values for S26 and S27 only, which reveal that the aquifer system is rich with iron and manganese-bearing minerals in the North-central part and eastern part of Siwa Oasis. Factor 3 (gypsum dissolution) showed high loading regarding samples S8, S11, S15, and S17, which are located mainly in the central part of the study area, suggesting enrichment of gypsum minerals in the NSSA in this location as a source of Ca2+ and SO42-. The remaining samples (S9, S18, S20, S21, S23, and 25) are distributed in the study area close to intensive vegetation cover from agricultural land. These samples were mainly affected by anthropogenic activities where the samples had high loading value with factor 4, especially in the central part of Siwa Oasis where the over-extraction of water for drinking and irrigation decreased the hydraulic head and could accelerate the infiltration of NO3−, Cl−, and Pb to the NSSA.
Health risk assessment
Examining the health risks tied to heavy metal presence in Siwa Oasis, especially within the Nubian Sandstone Aquifer (NSSA), remains critical for the wellbeing of the local population. Employing methods such as hazard indices and quotients offers valuable insights into potential health hazards for both adults and children. Decision-makers can leverage this information to craft effective water management strategies that prioritize the safety and quality of the primary drinking water source. Pinpointing specific pollutants and exposure pathways facilitates tailored approaches to mitigating health risks, thereby ensuring continued access to clean water for the community. Promoting community awareness encourages active involvement in efforts to maintain water quality standards. Emphasizing continuous monitoring and adaptive strategies contributes to the sustainable and resilient management of Siwa Oasis’ water resources. The statistics (min, max, and mean), range, class, and contribution percentage of samples in each risk indices were stated in Table 4.
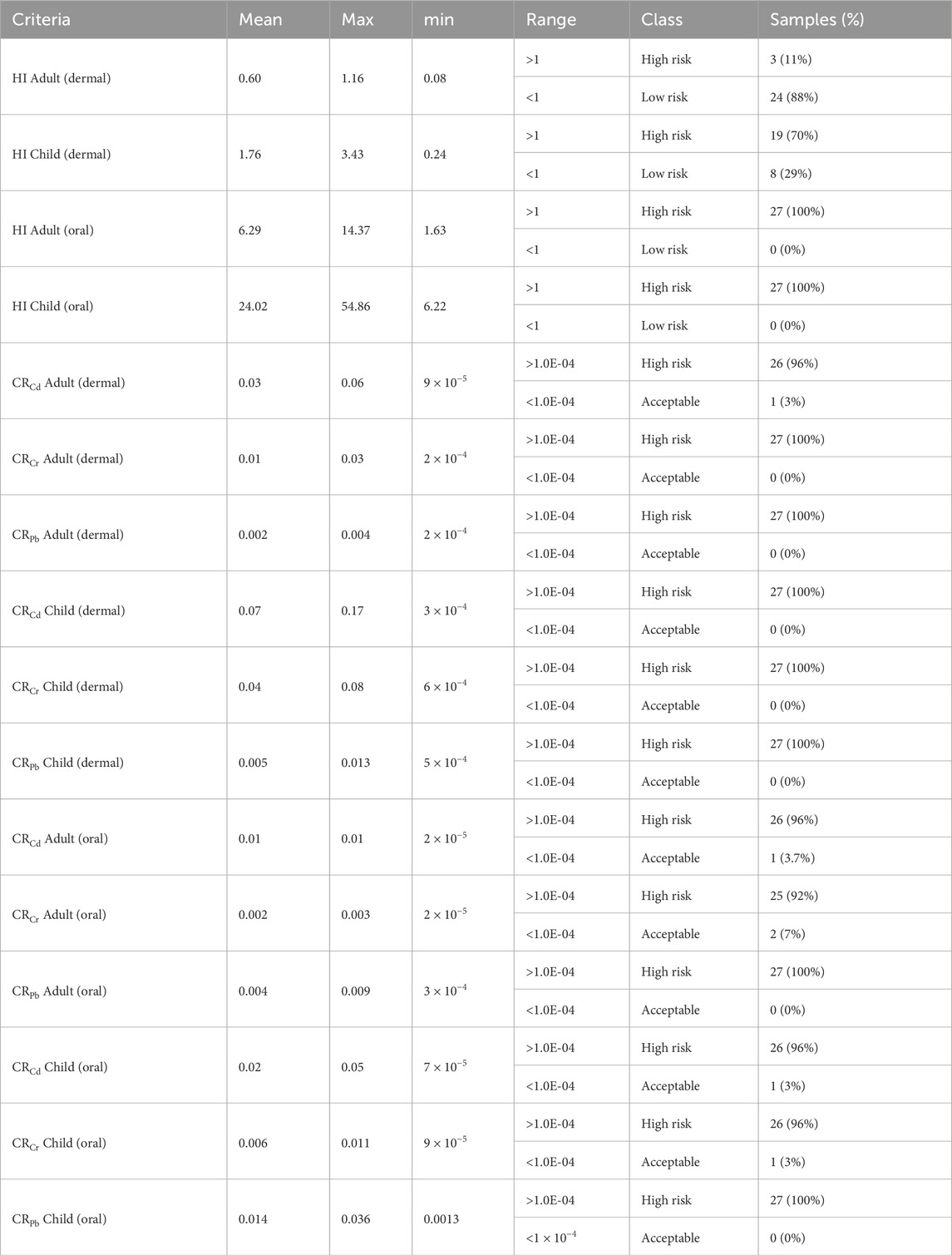
Table 4. Statistics (min, max, and mean), range, class, and contribution percentage of samples in each risk indices.
Noncarcinogenic health risk
The evaluation of toxic elements including Cd, Cr, Fe, Mn, Cu, Ni, Pb, and Zn in the NSSA and springs water aimed to assess the non-carcinogenic risk for both adults and children in Siwa Oasis through the oral and dermal contact (Figure 7). Adults’ hazard quotients (HQ) for ingestion varied across metals, ranging from 0.006 to 3.99 for Cd, 0.015 to 1.95 for Cr, 0.01 to 0.18 for Cu, 0.001 to 1.2 for Fe, 0.002 to 1.14 for Mn, 0.013 to 0.44 for Ni, 0.28 to 7.8 for Pb, and 5.02E-5 to 0.011 for Zn. In contrast, for children, the HQ ingestion ranged from 0.23 to 15.2 for Cd, 0.058 to 7.4 for Cr, 0.037 to 0.68 for Cu, 0.005 to 4.5 for Fe, 0.008 to 4.4 for Mn, 0.049 to 1.7 for Ni, 1.05 to 29.9 for Pb, and 1.9E-4 to 0.043 for Zn. Notably, HQ ingestion values demonstrate that children face more considerable health hazards from cadmium, chromium, copper, iron, magnesium, nickel, lead, and Zinc intake than adults. Most HQ oral values for all ages in water samples collected from NSSA and springs fall within acceptable levels (HQ<1), except lead, chromium, and cadmium, which surpassed the permitted levels (HQ>1).
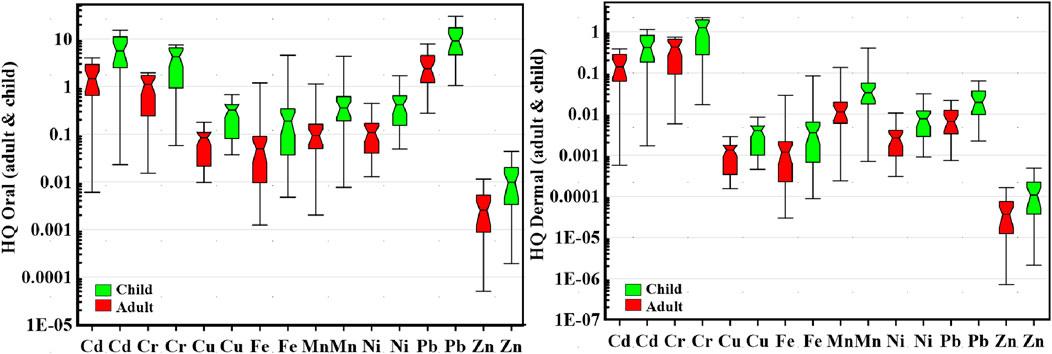
Figure 7. Notched box plot of the ingestion hazard quotient (HQ oral) (A), and skin hazard quotient (HQ dermal) (B) for all age groups (child and adult).
In the context of lead, chromium, and cadmium, if the HQ oral values are greater than 1, it indicates that there may be non-carcinogenic health risks associated with oral exposure to these heavy metals. Such risks could include gastrointestinal issues, kidney damage (for Cd), and neurological and developmental effects (for Pb). Meanwhile, HQ dermal values of Cu, Fe, Mn, Ni, Pb, and Zn for adults remained within acceptable limits (HQ<1), while for children, risks associated with Cd and Cr through dermal exposure were higher than the acceptable limits in 14.8% and 51.8% of samples. In summary, Pb, Cr, and Cd contribute significantly to human health risks through oral contact, and children face higher risks through dermal exposure with Cd and Cr in Siwa Oasis. These effects could include skin irritation, rashes, or other dermatological issues. Prolonged or repeated exposure to water containing elevated levels of Cd and Cr through activities such as bathing or swimming may increase the risk of absorbing these metals through the skin. Regular monitoring of water quality, implementing water treatment measures, and adopting safety measures to reduce exposure can be important strategies to manage and mitigate non-carcinogenic health risks associated with the oral ingestion of water polluted with Pb, Cr, and Cd.
The Hazard Index (HI) serves as a crucial indicator for evaluating the overall health risks associated with heavy metals in groundwater for adult and child (Mohammadi et al., 2022; Mohammadpour et al., 2023; Eid et al., 2024b). This comprehensive assessment considers a variety of exposure routes, including oral and skin mechanisms. The HI is calculated by summing or adding HQs for all heavy metals and interaction pathways, and it gives a comprehensive view of the cumulative health concerns associated with metal contamination in Siwa Oasis' drinking water supplies. The total risk (HI) values in, both oral and dermal, reveal potential health impacts for childeren and high non-carcinogenic risk for adult through oral contact only (Figure 8), with oral values ranging from 1.63 to 14.37 for adults and 6.22 to 54.86 for children, while dermal values vary between 0.08 and 1.16 in adults and 0.24 to 3.43 in children.
Unfortunately, HI oral levels for people of all ages are above permissible (HI > 1) in all water samples, indicating a substantial risk of non-carcinogenic effect. Notably, 88.88% of adult water samples fell into the low-risk category for cutaneous contact, whereas 11.11% were high-risk. In comparison, 29.6% of children fell into the low-risk category, while 70.4% had a high risk of skin contact. This implies that children are more susceptible to oral and skin exposure to toxic metals than adults., emphasizing the need for continuous monitoring of metal levels in Siwa Oasis' water resources, particularly considering the non-rechargeable nature of the groundwater, which serves as the main water source for various purposes.
Oral and dermal carcinogenic risk (CR)
This section targets the assessment of carcinogenic risk, which involve evaluating the chance of acquiring cancer due to prolonged exposure to certain metals found in Siwa Oasis’ water sources (NSSA and springs). The study used both standard calculations and advanced Monte Carlo simulations to determine carcinogenic risk (CR) levels for chromium (Cr), cadmium(Cd), and lead (Pb) in adults and children. The vast range of CR values highlights the various cancer risks of oral contact with these metals (Figure 9). For adults (dermal contact), CR values varied from 9E-5 to 0.06 for cadmium (Cd), 2E-4 to 0.03 for chromium (Cr), and 2E-4 to 0.004 for lead (Pb) (Figure 9). Similarly, in children, CR dermal values ranged between 3E-4 and 0.17 for Cd, 6E-4 and 0.08 for Cr, and 5E-4 and 0.018 for Pb (Figure 9). For adults (oral), CR values varied from 2E-5 to 0.01 for cadmium (Cd), 2E-5 to 0.003 for chromium (Cr), and 3E-4 to 0.009 for lead (Pb) (Figure 9). Similarly, in children, CR oral values ranged between 7E-5 and 0.05 for Cd, 9E-5 and 0.011 for Cr, and 0.0013 to 0.036 for Pb (Figure 9). The results showed that most of the water samples (96%–100%) collected from NSSA and springs in Siwa Oasis are contaminated with carcinogenic elements (Cd, Cr, and Pb). All scenarios analyzed in the table, which include different exposure routes (dermal and oral) and age groups (adults, children), have CR values greater than 1 × 10−4, which indicates a high potential for cancer risk according to the CR classification scheme. Cadmium (Cd) can damage kidneys, bones, and the nervous system. Chromium (Cr) can damage the lungs and kidneys. Lead (Pb) is Neurotoxic and can damage the developing brain in children.
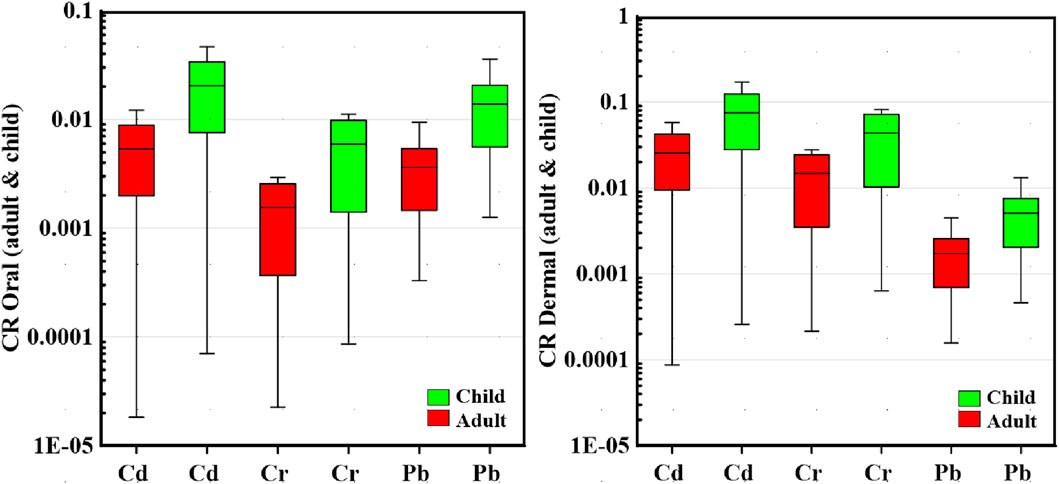
Figure 9. Box plot demonstrates the oral carcinogenic risk (CR oral) and dermal carcinogenic risk (CR dermal) for all age groups (adult and child).
It’s crucial to remember that the CR is just an estimate and does not guarantee that someone will develop cancer. Many factors, such as individual susceptibility, duration of exposure, and other environmental conditions, can influence the actual risk. This comprehensive analysis is crucial for understanding the health implications of using contaminated water in Siwa Oasis and emphasizes the significance of managing and mitigating these risks. The findings suggest an urgent need for improved treatment techniques for Siwa Oasis’ water resources. Heavy metals’ increased carcinogenic risk endangers the health of children and adults alike. Urgent action is necessary to implement effective treatment processes that can mitigate the impact of these contaminants, ensuring the safety and wellbeing of the local population.
Monte Carlo simulation (MCS) approach
This study employs MCS to thoroughly assess health risks associated with eight metals in groundwater, examining both oral and dermal exposure routes for adults and child. The Monte Carlo method, implemented through Python coding, considered the uncertainty and variability of input data, such as body weight, contaminant level, and ingestion rates. This method enabled a full assessment of the risks of metal exposure in various age groups.
Noncarcinogenic risk
The MCS model’s findings shed light on the health risks of heavy metal exposure via several pathways in Siwa Oasis’s water resources (Figure 10). Notably, the computed dermal hazard quotient (HQ dermal) reassures by showing that none of the metals surpass the stated limits (Figure 10). This indicates that exposure to the skin with water resources has no potential to cause health problems, which bodes well for humans of all ages. When oral exposure routes are taken into consideration, the scenario changes. Although certain metals, such as Mn, Fe, Cu, Ni, and Zn, have predicted HQ levels within acceptable boundaries, indicating that the risk is low for adults, lead, chromium, and cadmium have estimated HQ values greater than one (HQ >1), indicating a high risk of health issues for adults who consume water polluted with these elements through ingestion. A parallel pattern is observed for children as well (Figures 11C,D). This underlines the importance of addressing specific metals in water resources to mitigate potential health risks, particularly through oral exposure pathways.
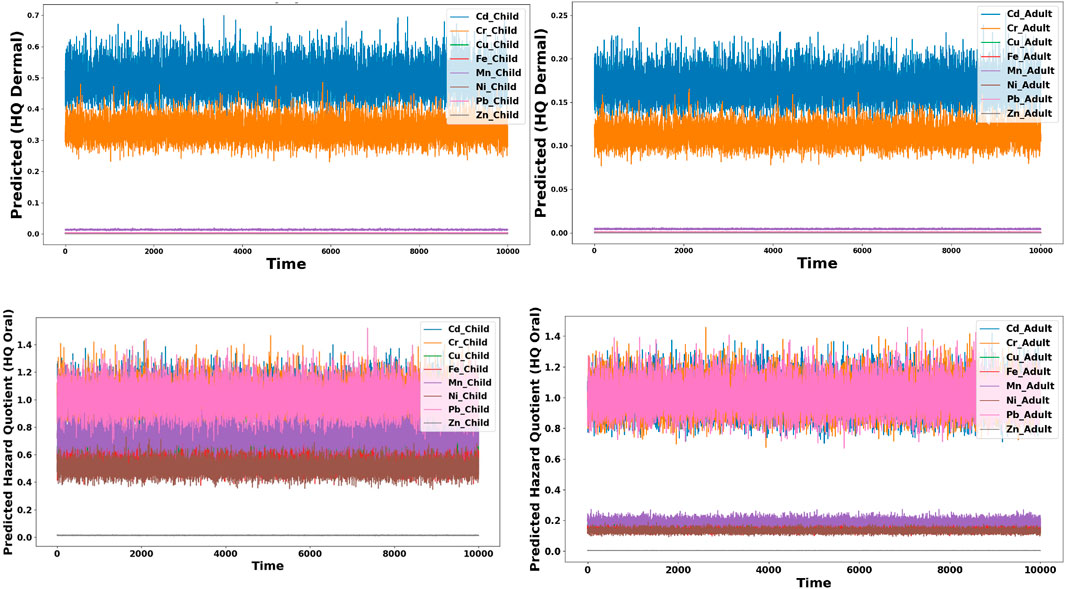
Figure 10. The simulated dermal hazard quotient (HQ adult) (A), (HQ child) (B), simulated oral hazard quotient (HQ adult) (C), and (HQ child).
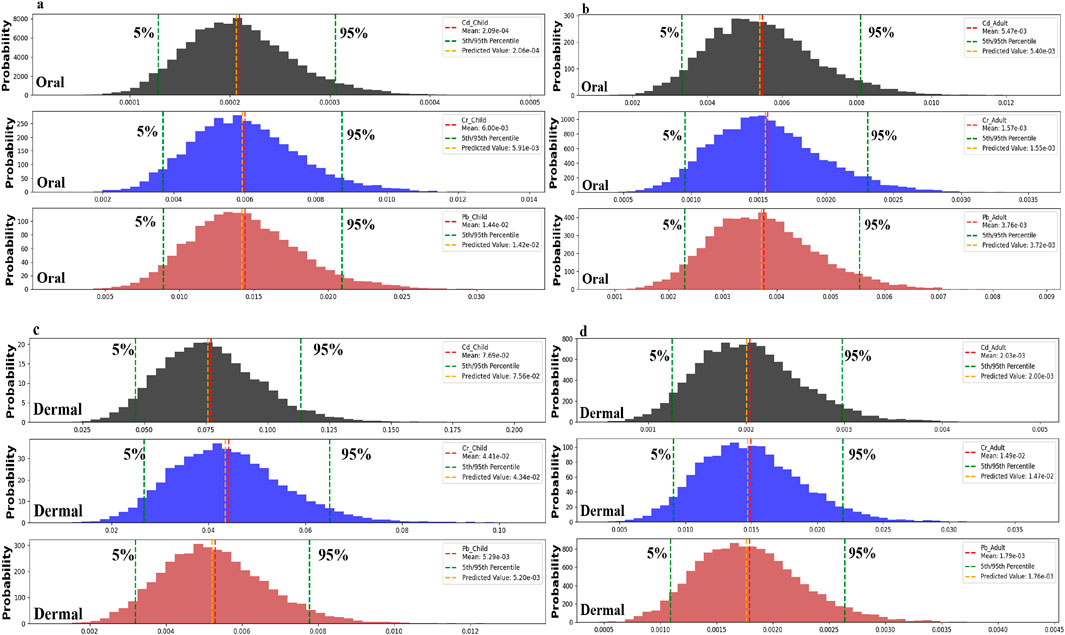
Figure 11. Simulated oral cancer risk (CR) of Cadmium, chromium, and Lead in child (A), adult (B), and dermal exposure CR in child (C), adult (D).
While specific metals such as iron, copper, nickel, Manganese, and Zinc are considered safe in children through ingestion pathways, lead, chromium, and cadmium have predicted hazard quotient (HQ) levels of more than one (HQ >1), indicating potential health hazards linked with the drinking of water containing these metals. Recognizing that these evaluations comprise hypotheses and uncertainties related to data sources is critical. As a result, continuous monitoring of exposure levels and regular risk assessment updates are required to protect the research area’s water resources and human health. The comparison of calculated HQ (Figure 7) and simulated HQ (Figure 10) from ingestion and skin exposure to metals identifies cadmium, lead, and Chromium as the primary variables responsible for a large non-carcinogenic influence on both children and adults in Siwa Oasis. The Monte Carlo model emerges as a reliable tool for predicting HQ values, giving valuable insights for continuing monitoring and management strategies.
Carcinogenic risk (CR)
Oral exposure
Examining CR probability in children and adults using oral measures reveals significant trends. CR test results consistently indicate greater values in adults than children for three carcinogenic metals (Cd, Pb, and Cr). The higher carcinogenic risk (CR) values observed in adults compared to children could be influenced by several factors related to exposure, physiology, and susceptibility. Adults generally have a longer history of exposure to contaminants than children. If adults have been exposed to heavy metals over an extended period, it can contribute to higher CR values. The parameters used to calculate CR include body weight and ingestion rates. Since adults typically have higher body weight and water ingestion rates than children, these factors can contribute to elevated CR values in adults. For children, the estimated 5th percentile CR ingestion value (lower limits of cancer risk) was 0.00012, 0.0036, and 0.0088 for Cadmium, chromium, and lead, respectively (Figure 11A). In contrast, at the 95th percentile level (upper boundaries of cancer risk), the estimated CR oral levels of Cadmium, chromium, and lead were 0.0003, 0.0087, and 0.021, respectively (Figure 11A), indicating that children face more significant risks. Adults, on the other hand, had predicted cancer risk levels that were significantly greater based on their percentile. Adults’ 5th percentile CR oral values (lower bounds of anticipated cancer risk) were 0.0033, 0.00096, and 0.0022 for Cadmium, chromium, and lead, respectively (Figure 11B). Furthermore, at the 95th percentile range, the computed CR for Cadmium, chromium, and Lead were 0.0081, 0.0023, and 0.0055, respectively (Figure 11B), indicating higher risks than those identified in children. The computed CR through ingestion suggests that most of the waters collected from NSSA and springs pose a considerable danger for people of all ages (CR > 1 × 10−4).
The comparison of calculated cancer Risk index (CR) values (Figure 9) with simulated CR through oral exposure to toxic metals (Figures 11A,B) reveals consistent and concerned results. Cadmium, chromium, and lead are three metals that have a substantial carcinogenic influence on people of all ages across all water samples in the Siwa Oasis. This alignment of measured and forecasted risks demonstrates the Monte Carlo method’s reliability and efficacy in predicting the potential cancer risks linked to heavy metal oral exposure. These findings highlight the urgent need for mitigating measures, intense monitoring, and regulatory initiatives to address the severe health hazards caused by these contaminants in Siwa Oasis’s water sources.
Dermal exposure
Children and adults reveal consistent patterns, with child consistently exhibiting higher CR values than adult across all metals (Cr, Cd, and Pb). Children’s estimated fifth % CR values for probable cancer development by exposure to the skin were 0.046, 0.026, and 0.0031 for Cadmium, chromium, and lead, respectively (Figure 11C). Children’s estimated 95th % CR risk values were 0.113, 0.065, and 0.0077 for Cadmium, chromium, and lead, respectively (Figure 11C). These values represent the top limits of possible cancer risks from skin exposure in children. Conversely, adults had lower predicted CR values for acquiring cancer by exposure to skin, with values of 0.0012, 0.0091, and 0.0011 at the lowest percentile (5th%) for Cadmium, chromium, and Lead (Figure 11D). Adults 95th percentile CR dermal levels were 0.0029, 0.0218, and 0.0026 for Cd, Cr, and Pb (Figure 11D). The lower CR dermal values for adults compared to children could be attributed to differences in body weight, skin surface area, and metabolic rates between the two age groups. Generally, children have higher metabolic rates and body surface area per unit of body weight than adults, leading to increased absorption rates of contaminants through the skin. These findings emphasize the substantial cancer risk associated with the intake of chromium, Cadmium, and Lead in Siwa Oasis' water resources, necessitating immediate action to reduce metal contamination and limit potential health risks for children and adults. The comparison of predicted CR (Figure 9) and calculated CR (Figures 11C,D) from dermal exposure with heavy metals demonstrates the consistency in identifying Cadmium, chromium, and Lead as contributors to a significant carcinogenic impact in a significant number of water samples from Nubian sandstone aquifer. Monte Carlo modeling proved a valuable and practical approach for predicting CR dermal value. This stresses the importance of predictive modeling in determining the potential health effects of heavy metal exposure via dermal pathways.
Comparison with several studies around the world
The application of probabilistic techniques such as Monte Carlo simulation were utilized globally by several researchers to decrease the uncertainty of the datasets and provide realistic risks linked with PTEs in different water resources. The current study was compared to different case studies (Table 5).
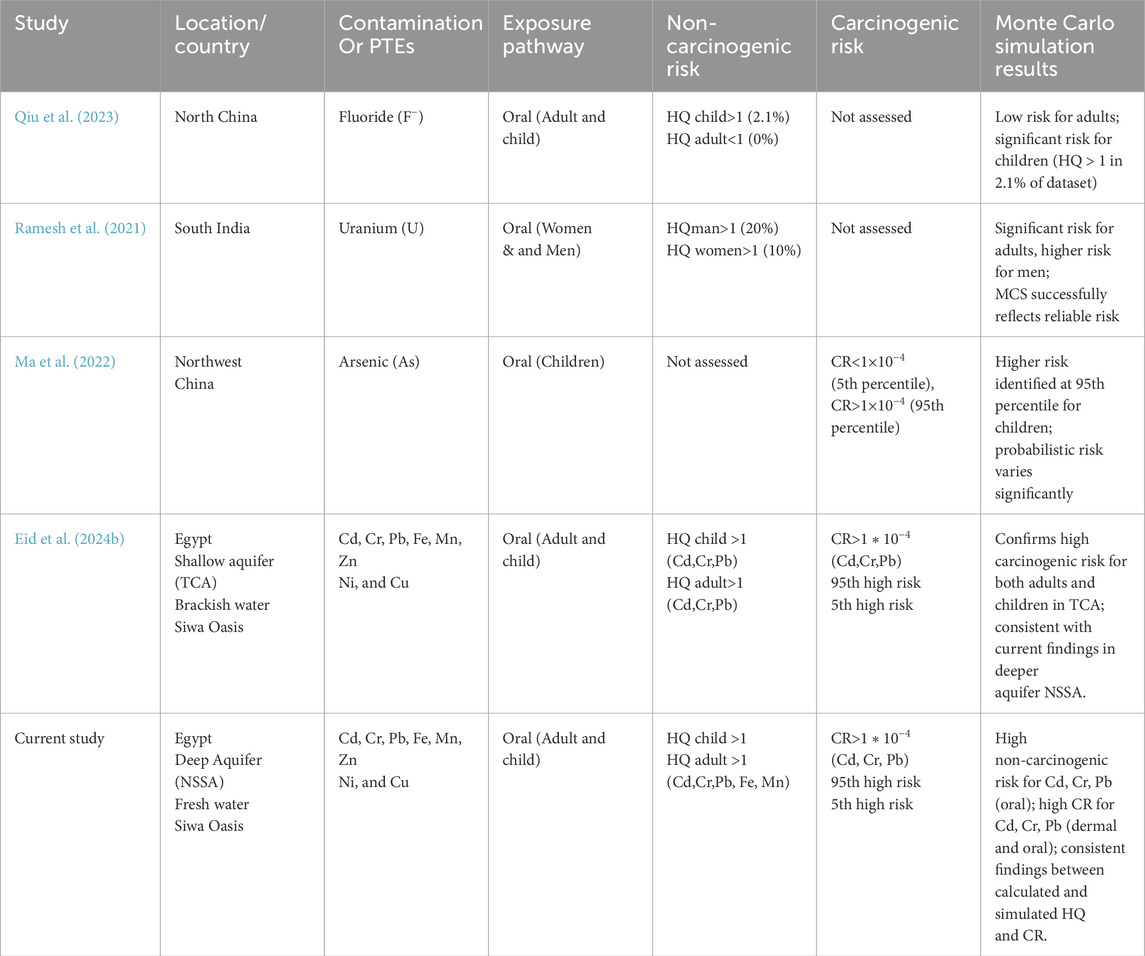
Table 5. Comparison of the Monte Carlo results in Siwa Oasis with global results in different water resources.
Qiu applied simulation method (MCS) to determine the risk of fluoride in the groundwater in the north China region and reported that the probabilistic risk for child through oral contact with F− metal for adult was low compared with children that were vulnerable to fluoride in 2.1% of the datasets (estimated HQ>1). Ramesh found that the probabilistic techniques (MCS) was successful to reflect the reliable risk of Uranium in groundwater in south India for adults (women and man) where the results showed that the estimated HQoral for both female and males was greater than threshold limit (HQ>1) in 10% and 20%, respectively. Ma investigated the probabilistic risk of As in the groundwater of Northwest China through estimated CR values obtained from MCS and demonstrated that the fifth percentile (5th) of the datasets had CR less than threshold value (1*10−4), while 95th showed high cancer risk for children through ingestion. Although the previous work in global study regions showed the effectiveness of the application of Monte Carlo as probabilistic method, it was important to calibrate the current study with previous work in the same study area such as the karst aquifer or shallow aquifer in Siwa Oasis which is called Tertiary carbonate aquifer (TCA). Recent study applied Monte Carlo Simulation to detect the health risk of PTEs in Siwa Oasis in different aquifer system with different geological and flow system (TCA). This study was performed by Eid that confirmed that the shallow aquifer that mainly used for irrigation purposes and contain brackish water was contaminated with several PTEs that originate from both natural and anthropogenic source. Eid proved with probabilistic method (MCS) that there is high carcinogenic risk for adult and children through ingestion and dermal contact with estimated CR>1*10−4 and high non-carcinogenic risk for child through oral contact. Eid couldn’t investigate the risk of PTEs in the main deep fresh water aquifer (Nubian Sandstone) in Siwa Oasis which is used mainly for drinking and could have direct risk to individuals of different age group. The current study could fill this gap of the previous investigations in the study area. Through the comparison between the deep fresh water aquifer (NSSA) and shallow brackish water aquifer (TCA), it was found that the NSSA is more contaminated with Fe and Mn and could cause non-carcinogenic risk for individuals through oral exposure with estimated HQ greater than one while no risk in the shallow aquifer regarding Fe and Mn. The shallow and deep aquifer were similar in safety of water through dermal contact with non-carcinogenic risk elements and high risk with cancer risk elements. However, the NSSA require further attention from the decision maker through effective treatment of the drinking water to protect the individuals from several health risks.
Strengths, limitations and recommended future research work
The current study could detect the hydrogeological framework in Siwa Oasis, where the correlation of geophysical logs (GR, SP, and resistivity) could determine the number of subsurface geological layers and structural connection between shallow and deep aquifer through the fault plain. The geological logs based on core samples could confirm the presence of iron oxides, limestone, dolomite, clay and shale minerals in the geological composition which refers to the geogenic source of Ca2+, Mg2+, Na+, Fe and Mn in the aquifer system. The elements that were not available in the geological composition such as NO3−, Pb, and Cl− could indicate the anthropogenic source. The correlation between these parameters from cluster analysis, PMF, and correlation matrix could agree with the geological composition and give more reliability of the interpretation of ion source detection. Application of stable isotopes could prove that there is no enough recharge of the aquifer system and the water rock interaction has more significant contribution to release the major and trace elements in the groundwater than anthropogenic activities.
The description of the NSSA as “pale meteoric water (non-rechargeable aquifer)” refers to groundwater that originated from ancient atmospheric precipitation and is not replenished by current rainfall. This characterization is significant because it implies that the NSSA is a finite water resource, which has critical implications for groundwater pollution and health risks in the Siwa Oasis. Since the NSSA does not receive new water inputs, any contamination from heavy metals or other pollutants can accumulate over time, leading to increased concentrations that pose a serious risk to human health. The lack of natural recharge means that pollutants are not naturally diluted or flushed out, making the water quality highly susceptible to deterioration from anthropogenic activities such as agriculture, waste disposal, and industrial processes. In terms of health risks, the accumulation of heavy metals like lead, cadmium, and chromium can lead to significant non-carcinogenic and carcinogenic health outcomes. Given that the NSSA is the sole source of drinking water for the local population, the potential for chronic exposure to these pollutants is high, increasing the urgency for monitoring and remediation efforts. The identification of the NSSA as pale meteoric water underscores the need for careful management to protect this crucial water source from pollution, as its non-rechargeable nature limits the potential for natural recovery or replenishment.
Although the current research integrated a compressive approach through physicochemical analysis, heavy metals, stable isotopes, geophysical logs, GIS, and applying different methods such as the PMF model, MCS model, and IDW interpolation to detect the recharge source, pollution source, and health risk concern, there are still some limitations regarding the number and type of samples which can be covered in further research in the future. This study recommends further investigation to collect more samples from different resources, including soil samples, surface lake samples, drains samples, and TCA water samples, to determine all the expected sources of contaminants and their health risks. Monitoring the water level and pressure of the aquifer will be groundwater modeling to detect the hydrodynamic condition between the TCA and NSSA and the possibility of anthropogenic activity to contaminate the NSSA and TCA. Application of nitrogen and sulfur isotopes will be strong indicators for the source of nitrates, and sulfates in the future work.
Conclusion
This study thoroughly examines heavy metal pollution, stable isotopes, and physicochemical parameters in various water resources (springs and NSSA) of the Egyptian Siwa Oasis, evaluating its sources and health implications. Including eight heavy metals (Fe, Ni, Cr, Mn, Zn, Cu, Pb, and Cd) adds complexity to the assessment.
The integration between the PMF model, geophysical logs, geochemical indexing approach (chloro-alkaline index, ionic ratio), and stable isotopes was successful in estimating the source of contamination of heavy metals and major ions and the primary mechanism controlling groundwater chemistry in deep aquifers (NSSA). The current research represents the first investigation of NSSA regarding source apportionment and health risks with these advanced methods.
The Sulin diagram, and Chloro-alkaline index (CAI), ionic ratio, stable isotopes revealed that the NSSA is a non-rechargeable (pale meteoric water) aquifer and shows the importance of monitoring its water quality regarding heavy metals where NSSA is the only source of drinking water in the Siwa Oasis. Silicate weathering, evaporation, dissolution, and reverse ion exchange play essential roles in groundwater evolution. The water type or composition of the water samples collected from NSSA and springs was dominated by CaCl2 due to the old marine sediments and water-rock interaction in the aquifer system. The geophysical logs (GR, SP, R, lithology) showed that the NSSA consists of several Sandstone layers separated by shale, silt, and clay layers intercalated with traces of iron oxides.
PMF model showed that the major ions and heavy metals in groundwater of the NSSA originated from 4 significant sources based on four factors extracted from the model and the contribution percentage of different species in each factor. The source of NO3−, Pb, Cl−, and Mg2+ is anthropogenic activities (agriculture, fertilizers, well corrosion, and wastewater) due to the over-extraction of water for irrigation, domestic, and drinking purposes. The elevated Ca2+ and SO42- ions concentration comes from the dissolution of gypsum and anhydrite. Fe, Mn, Ni, and Cr sources are iron and manganese-bearing minerals associated with clay minerals in the aquifer system. At the same time, Cd and Na are affected by mixing different water sources, including old trapped sea water and upward flow from hydrothermal water.
Elevated HI oral values, especially in children, underscore potential non-carcinogenic health risks associated with heavy metal exposure through oral and dermal contact and for adults through ingestion only. Metals like Cr, Cd, and Pb pose significant carcinogenic risk, as CR values exceed 1x10−4 in nearly all samples. MCS model affirm this carcinogenic impact, emphasizing elevated risks for both children and adults.
Actionable steps are imperative to mitigate metal pollution in Siwa Oasis, emphasizing treatment techniques for every source of drinking water to protect the ecosystem and individuals. Attention to both cancer-causing and non-carcinogenic health effects, as well as a reduction in exposure time, are critical. The practical application of the Monte Carlo method, particularly with Python code, underscores its utility in improving result reliability by reducing uncertainty in health risk assessments.
Data availability statement
The raw data supporting the conclusions of this article will be made available by the authors, without undue reservation.
Author contributions
ME: Conceptualization, Data curation, Formal Analysis, Investigation, Methodology, Software, Validation, Visualization, Writing–original draft, Writing–review and editing. VM: Conceptualization, Methodology, Writing–original draft. ME: Resources, Supervision, Validation, Writing–original draft. HR: Formal Analysis, Investigation, Writing–original draft. EM: Conceptualization, Formal Analysis, Resources, Writing–original draft. MA: Data curation, Validation, Writing–original draft. AE-S: Conceptualization, Funding acquisition, Writing–original draft. SB: Conceptualization, Data curation, Funding acquisition, Writing–original draft. AK: Resources, Supervision, Writing–original draft. PS: Formal Analysis, Project administration, Supervision, Writing–review and editing.
Funding
The author(s) declare that financial support was received for the research, authorship, and/or publication of this article. This work was funded by sustainable development and technologies national program of the Hungarian Academy of Sciences (FFT NP FTA). Also, the authors extend their appreciation to King Saud University for funding this work through Researchers Supporting Project number (RSP2024R133), King Saud University, Riyadh, Saudi Arabia.
Acknowledgments
We would like to thank the sustainable development and technologies national program of the Hungarian Academy of Sciences (FFT NP FTA) for funding this research work. Also, the authors extend their appreciation to King Saud University for funding this work through Researchers Supporting Project number (RSP2024R133), King Saud University, Riyadh, Saudi Arabia.
Conflict of interest
The authors declare that the research was conducted in the absence of any commercial or financial relationships that could be construed as a potential conflict of interest.
Publisher’s note
All claims expressed in this article are solely those of the authors and do not necessarily represent those of their affiliated organizations, or those of the publisher, the editors and the reviewers. Any product that may be evaluated in this article, or claim that may be made by its manufacturer, is not guaranteed or endorsed by the publisher.
Supplementary material
The Supplementary Material for this article can be found online at: https://www.frontiersin.org/articles/10.3389/feart.2024.1431635/full#supplementary-material
References
Abanyie, S. K., Apea, O. B., Abagale, S. A., Amuah, E. E. Y., and Sunkari, E. D. (2023). Sources and factors influencing groundwater quality and associated health implications: a review. Emerg. Contam. 9, 100207. doi:10.1016/j.emcon.2023.100207
Abdallah, A. (2007). Assessment of salt weathering in Siwa oasis (the western desert of Egypt). Bull. De. la Soc. De. Geog. De. Egypt 80, 66–83.
Abdel Zaher, M., Saibi, H., Mansour, K., Khalil, A., and Soliman, M. (2018). Geothermal exploration using airborne gravity and magnetic data at Siwa Oasis, Western Desert, Egypt. Renew. Sustain. Energy Rev. 82, 3824–3832. doi:10.1016/j.rser.2017.10.088
Abdulaziz, A. M., and Faid, A. M. (2015a). Evaluation of the groundwater resources potential of Siwa Oasis using three-dimensional multilayer groundwater flow model, Mersa Matruh Governorate, Egypt. Arab. J. Geosci. 8, 659–675. doi:10.1007/s12517-013-1199-4
Abdulaziz, A. M., and Faid, A. M. (2015b). Evaluation of the groundwater resources potential of Siwa Oasis using three-dimensional multilayer groundwater flow model, Mersa Matruh Governorate, Egypt. Arab. J. Geosci. 8, 659–675. doi:10.1007/s12517-013-1199-4
Abou El-Magd, I., and Faid, A. (2007). Hydrogeological and environmental studies on Siwa oasis, Egypt Using GIS technique. Egypt. J. Soil Sci. 47, 435–450.
Adimalla, N. (2020). Spatial distribution, exposure, and potential health risk assessment from nitrate in drinking water from semi-arid region of South India. Hum. Ecol. Risk Assess. An Int. J. 26, 310–334. doi:10.1080/10807039.2018.1508329
Afifi, A. H. (2005) Assessment of the hydrogeological conditions of groundwater in Siwa oasis, north western desert, 98p. Egypt: Minufiya University. Ph. D. Thesis.
Aggour, T. A., and Faid, A. M. (2007). Hydrogeology of Siwa oasis and landuse map, Egypt. J. Remote Sens. Space Sci. 9, 135–156.
Alam, S. M. K., Li, P., and Fida, M. (2024). Groundwater nitrate pollution due to excessive Use of N-fertilizers in rural areas of Bangladesh: pollution Status, health risk, source contribution, and future impacts. Expo. Health 16, 159–182. doi:10.1007/s12403-023-00545-0
Al-Mashreki, M. H., Eid, M. H., Saeed, O., Székács, A., Szűcs, P., Gad, M., et al. (2023). Integration of geochemical modeling, multivariate analysis, and irrigation indices for assessing groundwater quality in the Al-Jawf basin, Yemen. Water 15, 1496. doi:10.3390/w15081496
Aly, A. A. (2001). Spatiotemporal monitoring and assessment of water resources in Siwa Oasis. Egypt: Faculty of Agriculture Alexandria University. MSc Thesis.
Aly, A. A. (2020). Soil and groundwater salinization in Siwa Oasis and management opportunities: twenty year change detection and assessment. Arid. Land Res. Manag. 34, 117–135. doi:10.1080/15324982.2019.1635662
Aly, A. A., and Benaabidate, L. (2010). “Salinity of water resources in the Siwa oasis: monitoring and diagnosis,” in Water–rock interaction. London: Taylor & Francis Group.
Aly, A. A., Kishk, F. M., Gaber, H. M., and Al-Omran, A. M. (2016). Long-term detection and hydrochemistry of groundwater resources in Egypt: case study of Siwa Oasis. J. Saudi Soc. Agric. Sci. 15, 67–74. doi:10.1016/j.jssas.2014.04.003
Aref, M. A., and Taj, R. J. (2017). Hydrochemical characteristics of sabkha brines, evaporite crystallization and microbial activity in Al-Kharrar sabkha and their implication on future infrastructures in Rabigh area, Red Sea coastal plain of Saudi Arabia. Environ. Earth Sci. 76, 360. doi:10.1007/s12665-017-6686-6
Arruti, A., Fernández-Olmo, I., and Irabien, A. (2011). Impact of the global economic crisis on metal levels in particulate matter (PM) at an urban area in the Cantabria Region (Northern Spain). Environ. Pollut. 159, 1129–1135. doi:10.1016/j.envpol.2011.02.008
Awadh, S. M., Al-Auweidy, M. R., and Al-Yaseri, A. A. (2019). Hydrochemistry as a tool for interpreting brine origin and chemical equilibrium in oilfields: Zubair reservoir southern Iraq case study. Appl. Water Sci. 9, 65. doi:10.1007/s13201-019-0944-6
Ayers, R., and Westcott, D. (1994). “Water quality for agriculture,” in FAO irrigation and drainage paper 29 Rev. 1. Food and Agricultural Organisation of the United Nations.
Brown, S. G., Eberly, S., Paatero, P., and Norris, G. A. (2015). Methods for estimating uncertainty in PMF solutions: Examples with ambient air and water quality data and guidance on reporting PMF results. Sci. Total Environ. 518–519, 626–635. doi:10.1016/j.scitotenv.2015.01.022
Chai, N., Yi, X., Xiao, J., Liu, T., Liu, Y., Deng, L., et al. (2021). Spatiotemporal variations, sources, water quality and health risk assessment of trace elements in the Fen River. Sci. Total Environ. 757, 143882. doi:10.1016/j.scitotenv.2020.143882
Chang, C.-W., Zhou, S., Gao, Y., Lin, L., Liu, T., Bradley, J. D., et al. (2022). Validation of a deep learning-based material estimation model for Monte Carlo dose calculation in proton therapy. Phys. Med. Biol. 67, 215004. doi:10.1088/1361-6560/ac9663
Chen, L., Liang, S., Liu, M., Yi, Y., Mi, Z., Zhang, Y., et al. (2019). Trans-provincial health impacts of atmospheric mercury emissions in China. Nat. Commun. 10, 1484. doi:10.1038/s41467-019-09080-6
Chen, X., Li, F., Zhang, J., Liu, S., Ou, C., Yan, J., et al. (2021). Status, fuzzy integrated risk assessment, and hierarchical risk management of soil heavy metals across China: a systematic review. Sci. Total Environ. 785, 147180. doi:10.1016/j.scitotenv.2021.147180
Chen, Y., Rich, D. Q., and Hopke, P. K. (2022). Long-term PM2.5 source analyses in New York City from the perspective of dispersion normalized PMF. Atmos. Environ. 272, 118949. doi:10.1016/j.atmosenv.2022.118949
Cheng, G., Wang, M., Chen, Y., and Gao, W. (2020). Source apportionment of water pollutants in the upstream of Yangtze River using APCS–MLR. Environ. Geochem Health 42, 3795–3810. doi:10.1007/s10653-020-00641-z
Czuppon, G., Demény, A., Leél-Őssy, S., Óvari, M., Molnár, M., Stieber, J., et al. (2018). Cave monitoring in the Béke and Baradla caves (Northeastern Hungary): implications for the conditions for the formation cave carbonates. IJS 47, 13–28. doi:10.5038/1827-806X.47.1.2110
Eid, M. H., Eissa, M., Mohamed, E. A., Ramadan, H. S., Czuppon, G., Kovács, A., et al. (2024a). Application of stable isotopes, mixing models, and K-means cluster analysis to detect recharge and salinity origins in Siwa Oasis, Egypt. Groundw. Sustain. Dev. 25, 101124. doi:10.1016/j.gsd.2024.101124
Eid, M. H., Eissa, M., Mohamed, E. A., Ramadan, H. S., Tamás, M., Kovács, A., et al. (2024b). New approach into human health risk assessment associated with heavy metals in surface water and groundwater using Monte Carlo Method. Sci. Rep. 14, 1008. doi:10.1038/s41598-023-50000-y
Eid, M. H., Elbagory, M., Tamma, A. A., Gad, M., Elsayed, S., Hussein, H., et al. (2023). Evaluation of groundwater quality for irrigation in deep aquifers using multiple Graphical and indexing approaches supported with machine learning models and GIS techniques, Souf valley, Algeria. Water 15, 182. doi:10.3390/w15010182
Eid, M. H., Shebl, A., Eissa, M., Mohamed, E. A., Fahil, A. S., Ramadan, H. S., et al. (2024c). Comprehensive approach integrating remote sensing, machine learning, and physicochemical parameters to detect hydrodynamic conditions and groundwater quality deterioration in non-rechargeable aquifer systems. Heliyon 10, e32992. doi:10.1016/j.heliyon.2024.e32992
Eid, M. H., Tamma, A. A., Saeed, O., Székács, A., Abukhadra, M. R., El-Sherbeeny, A. M., et al. (2024d). Advanced approach combines integrated weight water quality index and potential toxic elements for environmental and health risk assessment supported by simulation technique in Oued Souf, Algeria. Sci. Rep. 14, 17805. doi:10.1038/s41598-024-68854-1
Eid, M. H., Tamma, A. A., Saeed, O., Székács, A., Abukhadra, M. R., El-Sherbeeny, A. M., et al. (2024e). Advanced approach combines integrated weight water quality index and potential toxic elements for environmental and health risk assessment supported by simulation technique in Oued Souf, Algeria. Sci. Rep. 14, 17805. doi:10.1038/s41598-024-68854-1
El Hossary, M. F. M. (2013). Investigating the development challenges to Siwa Oasis, northwestern desert, Egypt. N. Y. Sci. J. 6, 55–61.
Elnaggar, A., Mosa, A., El-Seedy, M., and Biki, F. (2016). Evaluation of soil agricultural productive Capability by using remote sensing and GIS techniques in Siwa oasis, Egypt. J. Soil Sci. Agric. Eng. 7, 547–555. doi:10.21608/jssae.2016.39785
Elnazer, A. A., Salman, S. A., Mohamed, Y. M. A., Stafford, J., Davies, P., and El Nazer, H. A. (2023). Siwa Oasis groundwater quality: factors controlling spatial and temporal changes. Environ. Monit. Assess. 195, 61. doi:10.1007/s10661-022-10646-z
Epa, A. (2004). “Risk assessment guidance for superfund,” in Volume I: human health evaluation manual (Part E, supplemental guidance for dermal risk assessment). EPA/540/R/99.
Gaagai, A., Aouissi, H., Bencedira, S., Hinge, G., Athamena, A., Heddam, S., et al. (2023). Application of water quality indices, machine learning approaches, and GIS to identify groundwater quality for irrigation purposes: a case study of Sahara aquifer, Doucen plain, Algeria. Water 15, 289. doi:10.3390/w15020289
Gad, M., Dahab, K., and Ibrahim, H. (2018). Applying of a geochemical model on the Nubian sandstone aquifer in Siwa oasis, western desert, Egypt. Environ. Earth Sci. 77, 401. doi:10.1007/s12665-018-7580-6
Gad, M., Gaagai, A., Agrama, A. A., El-Fiqy, W. F. M., Eid, M. H., Szűcs, P., et al. (2024). Comprehensive evaluation and prediction of groundwater quality and risk indices using quantitative approaches, multivariate analysis, and machine learning models: an exploratory study. Heliyon 10, e36606. doi:10.1016/j.heliyon.2024.e36606
Gad, M., Gaagai, A., Eid, M. H., Szűcs, P., Hussein, H., Elsherbiny, O., et al. (2023). Groundwater quality and health risk assessment using indexing approaches, multivariate statistical analysis, artificial neural Networks, and GIS techniques in El Kharga oasis, Egypt. Water 15, 1216. doi:10.3390/w15061216
Gade, M., Comfort, N., and Re, D. B. (2021). Sex-specific neurotoxic effects of heavy metal pollutants: Epidemiological, experimental evidence and candidate mechanisms. Environ. Res. 201, 111558. doi:10.1016/j.envres.2021.111558
Gaillardet, J., Dupré, B., Louvat, P., and Allègre, C. J. (1999). Global silicate weathering and CO2 consumption rates deduced from the chemistry of large rivers. Chem. Geol. 159, 3–30. doi:10.1016/S0009-2541(99)00031-5
Gat, J. R., and Gonfiantini, R. (1981). “Stable isotope hydrology. Deuterium and oxygen-18 in the water cycle,” in A monograph prepared under the aegis of the IAEA/UNESCO working group on nuclear techniques in hydrology of the international hydrological programme.
Giri, S., and Singh, A. K. (2015). Human health risk assessment via drinking water pathway due to metal contamination in the groundwater of Subarnarekha River Basin, India. Environ. Monit. Assess. 187, 63. doi:10.1007/s10661-015-4265-4
Guo, X., Zuo, R., Meng, L., Wang, J., Teng, Y., Liu, X., et al. (2018). Seasonal and spatial variability of anthropogenic and natural factors influencing groundwater quality based on source apportionment. IJERPH 15, 279. doi:10.3390/ijerph15020279
Habib, M. A., Islam, A. R. M. T., Bodrud-Doza, M., Mukta, F. A., Khan, R., Bakar Siddique, M. A., et al. (2020). Simultaneous appraisals of pathway and probable health risk associated with trace metals contamination in groundwater from Barapukuria coal basin, Bangladesh. Chemosphere 242, 125183. doi:10.1016/j.chemosphere.2019.125183
Haghnazar, H., Johannesson, K. H., González-Pinzón, R., Pourakbar, M., Aghayani, E., Rajabi, A., et al. (2022). Groundwater geochemistry, quality, and pollution of the largest lake basin in the Middle East: comparison of PMF and PCA-MLR receptor models and application of the source-oriented HHRA approach. Chemosphere 288, 132489. doi:10.1016/j.chemosphere.2021.132489
Hamdy Eid, M., Szűcs, P., and Kovács, A. (2022). Problems Threatening sustainability in Siwa oasis and Recommendations for understanding the sources of water quality deterioration. Geosci. Eng. 10, 138–153. doi:10.33030/geosciences.2022.15.138
Hauke, J., and Kossowski, T. (2011). Comparison of values of Pearson’s and Spearman’s correlation coefficients on the same sets of data. Quaest. Geogr. 30, 87–93. doi:10.2478/v10117-011-0021-1
Hsu, D. (2010). Algorithmic foundations of robotics. 9: selected contributions of the Ninth international Workshop on the Algorithmic Foundations of Robotics: the ninth WAFR was held on December 13 -15, 2010 at the national University of Singapore. Editor D. Hsu (Berlin Heidelberg: Springer).
Hu, Y., Chen, M., Pu, J., Chen, S., Li, Y., and Zhang, H. (2024). Enhancing phosphorus source apportionment in watersheds through species-specific analysis. Water Res. 253, 121262. doi:10.1016/j.watres.2024.121262
Huang, J., Wu, Y., Sun, J., Li, X., Geng, X., Zhao, M., et al. (2021). Health risk assessment of heavy metal(loid)s in park soils of the largest megacity in China by using Monte Carlo simulation coupled with Positive matrix factorization model. J. Hazard. Mater. 415, 125629. doi:10.1016/j.jhazmat.2021.125629
Huston, R., Chan, Y. C., Chapman, H., Gardner, T., and Shaw, G. (2012). Source apportionment of heavy metals and ionic contaminants in rainwater tanks in a subtropical urban area in Australia. Water Res. 46, 1121–1132. doi:10.1016/j.watres.2011.12.008
Ibrahim, H., Yaseen, Z. M., Scholz, M., Ali, M., Gad, M., Elsayed, S., et al. (2023). Evaluation and prediction of groundwater quality for irrigation using an integrated water quality indices, machine learning models and GIS approaches: a Representative case study. Water 15, 694. doi:10.3390/w15040694
Ibrahim, M. M., El-Beshbeshy, R. T., Kamh, N. R., and Abou-Amer, A. I. (2013). Effect of NPK and biofertilizer on date palm trees grown in S iwa O asis, E gypt. Soil Use Manag. 29, 315–321. doi:10.1111/sum.12042
Jaydhar, A. K., Pal, S. C., Saha, A., Islam, A. R. Md. T., Ruidas, D., and Costache, R. (2023). Hydrogeochemical properties of groundwater and associated human health hazards in coastal multiaquifers of India. Environ. Sci. Pollut. Res. 31, 18054–18073. doi:10.1007/s11356-023-27765-w
Klimach, A., and Zębek, E. (2024). Utility of water-based databases for underground water management: Legal and system perspective. Sustainability 16, 4608. doi:10.3390/su16114608
Kumar, A., and Singh, C. K. (2020). Arsenic enrichment in groundwater and associated health risk in Bari doab region of Indus basin, Punjab, India. Environ. Pollut. 256, 113324. doi:10.1016/j.envpol.2019.113324
Kwak, S. K., and Kim, J. H. (2017). Statistical data preparation: management of missing values and outliers. Korean J. Anesthesiol. 70, 407. doi:10.4097/kjae.2017.70.4.407
Lee, J. H., Yoshida, Y., Turpin, B. J., Hopke, P. K., Poirot, R. L., Lioy, P. J., et al. (2002). Identification of sources contributing to mid-Atlantic regional Aerosol. J. Air & Waste Manag. Assoc. 52, 1186–1205. doi:10.1080/10473289.2002.10470850
Li, T., Bian, J., Ruan, D., Xu, L., and Zhang, S. (2024). Groundwater health risk assessment and its temporal and spatial evolution based on trapezoidal fuzzy number-Monte Carlo stochastic simulation: a case study in western Jilin province. Ecotoxicol. Environ. Saf. 282, 116736. doi:10.1016/j.ecoenv.2024.116736
Li, W., Wu, J., Zhou, C., and Nsabimana, A. (2021). Groundwater pollution source identification and apportionment using PMF and PCA-APCS-MLR receptor models in Tongchuan city, China. Arch. Environ. Contam. Toxicol. 81, 397–413. doi:10.1007/s00244-021-00877-5
Li, X., Xiao, J., Chai, N., and Jin, Z. (2023). Controlling mechanism and water quality assessment of arsenic in China’s Yellow River Basin. J. Clean. Prod. 418, 137953. doi:10.1016/j.jclepro.2023.137953
Li, Y., Feng, X., Zhou, J., and Zhu, L. (2020). Occurrence and source apportionment of novel and legacy poly/perfluoroalkyl substances in Hai River basin in China using receptor models and isomeric fingerprints. Water Res. 168, 115145. doi:10.1016/j.watres.2019.115145
Liang, F., Yang, S., and Sun, C. (2011). Primary health risk analysis of metals in surface water of Taihu lake, China. Bull. Environ. Contam. Toxicol. 87, 404–408. doi:10.1007/s00128-011-0379-8
Ligate, F., Ijumulana, J., Ahmad, A., Kimambo, V., Irunde, R., Mtamba, J. O., et al. (2021). Groundwater resources in the East African Rift Valley: understanding the geogenic contamination and water quality challenges in Tanzania. Sci. Afr. 13, e00831. doi:10.1016/j.sciaf.2021.e00831
Liu, R., Dong, X., Wang, X., Zhang, P., Liu, M., and Zhang, Y. (2021a). Study on the relationship among the urbanization process, ecosystem services and human well-being in an arid region in the context of carbon flow: Taking the Manas river basin as an example. Ecol. Indic. 132, 108248. doi:10.1016/j.ecolind.2021.108248
Liu, W., Xu, Y., Fan, D., Li, Y., Shao, X.-F., and Zheng, J. (2021b). Alleviating corporate environmental pollution threats toward public health and safety: the role of smart city and artificial intelligence. Saf. Sci. 143, 105433. doi:10.1016/j.ssci.2021.105433
Loredana Ungureanu, E., and Mustatea, G. (2022). “Toxicity of heavy metals,” in Environmental impact and remediation of heavy metals. Editors H. M. Saleh, and A. I. Hassan (IntechOpen). doi:10.5772/intechopen.102441
Loring, D. H. (1976). Distribution and partition of cobalt, nickel, chromium, and vanadium in the sediments of the Saguenay fjord. Can. J. Earth Sci. 13, 1706–1718. doi:10.1139/e76-180
Ma, Z., Li, J., Zhang, M., You, D., Zhou, Y., and Gong, Z. (2022). Groundwater health risk assessment based on Monte Carlo model Sensitivity analysis of Cr and as—a case study of Yinchuan city. Groundw. health risk Assess. based Monte Carlo Model. Sensit. Anal. Cr As—a case study Yinchuan City. Water 14, 2419. doi:10.3390/w14152419
Meng, Y., Wu, J., Li, P., and Wang, Y. (2023). Distribution characteristics, source identification and health risk assessment of trace metals in the coastal groundwater of Taizhou City, China. Environ. Res. 238, 117085. doi:10.1016/j.envres.2023.117085
Misak, R. F., Abdel Baki, A. A., and El-Hakim, M. S. (1997). On the causes and control of the waterlogging phenomenon, Siwa Oasis, northern Western Desert, Egypt. J. Arid. Environ. 37, 23–32. doi:10.1006/jare.1997.0252
Moez, P., and Younan, D. N. A. (2016). High prevalence of haemoglobin S in the closed Egyptian community of Siwa Oasis. J. Clin. Pathol. 69, 632–636. doi:10.1136/jclinpath-2015-203199
Mohamed, M., Abou-Kota, M., Ganzou, S., and Abdellatif, A. (2020). MATHEMATICAL MODELING OF WATER QUALITY WITH A DIFFERENT CHEMICAL STATE; NUTRIENTS AND ARID ENVIRONMENTAL CONDITIONS, SIWA OASIS, EGYPT. EJPS 3, 15–36. doi:10.47672/ejps.619
Mohammadi, A., Mansour, S. N., Najafi, M. L., Toolabi, A., Abdolahnejad, A., Faraji, M., et al. (2022). Probabilistic risk assessment of soil contamination related to agricultural and industrial activities. Environ. Res. 203, 111837. doi:10.1016/j.envres.2021.111837
Mohammadpour, A., Motamed-Jahromi, M., Soleimani, H., Dehbandi, R., Doost, Z. E., Samaei, M. R., et al. (2023). Trace elements human health risk assessment by Monte Carlo probabilistic method in drinking water of Shiraz, Iran. Int. J. Environ. Sci. Technol. 20, 3775–3788. doi:10.1007/s13762-023-04815-x
Mukherjee, A., and Fryar, A. E. (2008). Deeper groundwater chemistry and geochemical modeling of the arsenic affected western Bengal basin, West Bengal, India. Appl. Geochem. 23, 863–894. doi:10.1016/j.apgeochem.2007.07.011
Phillips, L., and Moya, J. (2013). The evolution of EPA’s Exposure Factors Handbook and its future as an exposure assessment resource. J. Expo. Sci. Environ. Epidemiol. 23, 13–21. doi:10.1038/jes.2012.77
Piper, A. M. (1944). A graphic procedure in the geochemical interpretation of water-analyses. Trans. AGU 25, 914. doi:10.1029/TR025i006p00914
Qi, Z., Kang, G., Hu, C., Xu, Y., and Chen, H. (2021). Application of GWLF model in the Baohe River Watershed: analysis of the source composition of nitrogen and phosphorus in the region. IOP Conf. Ser. Earth Environ. Sci. 621, 012079. doi:10.1088/1755-1315/621/1/012079
Qiu, H., Gui, H., Xu, H., Cui, L., and Yu, H. (2023). Occurrence, controlling factors and noncarcinogenic risk assessment based on Monte Carlo simulation of fluoride in mid-layer groundwater of Huaibei mining area, North China. Sci. Total Environ. 856, 159112. doi:10.1016/j.scitotenv.2022.159112
Ramesh, R., Subramanian, M., Lakshmanan, E., Subramaniyan, A., and Ganesan, G. (2021). Human health risk assessment using Monte Carlo simulations for groundwater with uranium in southern India. Ecotoxicol. Environ. Saf. 226, 112781. doi:10.1016/j.ecoenv.2021.112781
Rashid, A., Ayub, M., Bundschuh, J., Gao, X., Ullah, Z., Ali, L., et al. (2023). Geochemical control, water quality indexing, source distribution, and potential health risk of fluoride and arsenic in groundwater: occurrence, sources apportionment, and positive matrix factorization model. J. Hazard. Mater. 460, 132443. doi:10.1016/j.jhazmat.2023.132443
Ren, X., Yang, C., Zhao, B., Xiao, J., Gao, D., and Zhang, H. (2023). Water quality assessment and pollution source apportionment using multivariate statistical and PMF receptor modeling techniques in a sub-watershed of the upper Yangtze River, Southwest China. Environ. Geochem Health 45, 6869–6887. doi:10.1007/s10653-023-01477-z
Ruidas, D., Pal, S. C., Chowdhuri, I., Saha, A., Biswas, T., Islam, A. R. Md. T., et al. (2023). Hydrogeochemical evaluation for human health risk assessment from contamination of coastal groundwater aquifers of Indo-Bangladesh Ramsar site. J. Clean. Prod. 399, 136647. doi:10.1016/j.jclepro.2023.136647
Saeed, O., Székács, A., Jordán, G., Mörtl, M., Abukhadra, M. R., and Eid, M. H. (2023a). Correction: investigating the impacts of heavy metal(loid)s on ecology and human health in the lower basin of Hungary’s Danube River: a Python and Monte Carlo simulation-based study. Environ. Geochem Health 45, 9785. doi:10.1007/s10653-023-01777-4
Saeed, O., Székács, A., Jordán, G., Mörtl, M., Abukhadra, M. R., and Eid, M. H. (2023b). Investigating the impacts of heavy metal(loid)s on ecology and human health in the lower basin of Hungary’s Danube River: a Python and Monte Carlo simulation-based study. Environ. Geochem Health 45, 9757–9784. doi:10.1007/s10653-023-01769-4
Saeed, O., Székács, A., Jordán, G., Mörtl, M., Abukhadra, M. R., El-Sherbeeny, A. M., et al. (2024). Assessing surface water quality in Hungary’s Danube basin using geochemical modeling, multivariate analysis, irrigation indices, and Monte Carlo simulation. Sci. Rep. 14, 18639. doi:10.1038/s41598-024-69312-8
Saleem, M., Iqbal, J., and Shah, M. H. (2019). Seasonal variations, risk assessment and multivariate analysis of trace metals in the freshwater reservoirs of Pakistan. Chemosphere 216, 715–724. doi:10.1016/j.chemosphere.2018.10.173
Salem, S., Gaagai, A., Ben Slimene, I., Moussa, A., Zouari, K., Yadav, K., et al. (2023). Applying multivariate analysis and machine learning approaches to evaluating groundwater quality on the Kairouan plain, Tunisia. Water 15, 3495. doi:10.3390/w15193495
Salim, I., Sajjad, R. U., Paule-Mercado, C., Memon, S. A., Lee, B.-Y., Sukhbaatar, C., et al. (2019). Comparison of two receptor models PCA-MLR and PMF for source identification and apportionment of pollution carried by runoff from catchment and sub-watershed areas with mixed land cover in South Korea. Sci. Total Environ. 663, 764–775. doi:10.1016/j.scitotenv.2019.01.377
Schoeller, H. (1967). Methods and techniques of groundwater investigation and development. Water Resour. Ser. 33.
Schoeller, H. (1977). Geochemistry of groundwater. Groundwater studies, an international guide for research and practice. Paris: UNESCO, 1–18.
Sheng, D., Meng, X., Wen, X., Wu, J., Yu, H., and Wu, M. (2022). Contamination characteristics, source identification, and source-specific health risks of heavy metal(loid)s in groundwater of an arid oasis region in Northwest China. Sci. Total Environ. 841, 156733. doi:10.1016/j.scitotenv.2022.156733
Stein, J. M. (1975). The effect of adrenaline and of α-and β-adrenergic blocking agents on ATP concentration and on incorporation of 32Pi into ATP in rat fat cells. Biochem. Pharmacol. 24, 1659–1662. doi:10.1016/0006-2952(75)90002-7
Subba Rao, N. (2021). Spatial distribution of quality of groundwater and probabilistic non-carcinogenic risk from a rural dry climatic region of South India. Environ. Geochem Health 43, 971–993. doi:10.1007/s10653-020-00621-3
Sulin, V. A. (1946). Waters of petroleum formations in the system of natural water. Moscow: Gostoptekhiz-dat. (in Russian) 3596.
Tawfik, H. A., Salah, M. K., Maejima, W., Armstrong-Altrin, J. S., Abdel-Hameed, A. T., and El Ghandour, M. M. (2018). Petrography and geochemistry of the lower Miocene Moghra sandstones, Qattara depression, north western desert, Egypt. Geol. J. 53, 1938–1953. doi:10.1002/gj.3025
Vesković, J., Deršek-Timotić, I., Lučić, M., Miletić, A., Đolić, M., Ražić, S., et al. (2024). Entropy-weighted water quality index, hydrogeochemistry, and Monte Carlo simulation of source-specific health risks of groundwater in the Morava River plain (Serbia). Mar. Pollut. Bull. 201, 116277. doi:10.1016/j.marpolbul.2024.116277
Wu, B., Zhao, D. Y., Jia, H. Y., Zhang, Y., Zhang, X. X., and Cheng, S. P. (2009). Preliminary risk assessment of trace metal pollution in surface water from Yangtze river in Nanjing section, China. Bull. Environ. Contam. Toxicol. 82, 405–409. doi:10.1007/s00128-008-9497-3
Yamamoto, S. (1988). “Chapter 2 ferromagnesian and Metalliferous Pelagic clay minerals in Oceanic sediments,” in Developments in Sedimentology (Elsevier), 115–146. doi:10.1016/S0070-4571(08)70007-4
Yu, H., Lin, M., Peng, W., and He, C. (2022). Seasonal changes of heavy metals and health risk assessment based on Monte Carlo simulation in alternate water sources of the Xinbian River in Suzhou City, Huaibei Plain, China. Ecotoxicol. Environ. Saf. 236, 113445. doi:10.1016/j.ecoenv.2022.113445
Zaghlool, E. (2020). Geochemical modeling and statistical analysis for groundwater evolution assessment in Wadi Qasab, Sohag, eastern desert, Egypt. GEP 08 08, 33–61. doi:10.4236/gep.2020.89003
Zanotti, C., Rotiroti, M., Fumagalli, L., Stefania, G. A., Canonaco, F., Stefenelli, G., et al. (2019). Groundwater and surface water quality characterization through positive matrix factorization combined with GIS approach. Water Res. 159, 122–134. doi:10.1016/j.watres.2019.04.058
Zhang, H., Cheng, S., Li, H., Fu, K., and Xu, Y. (2020a). Groundwater pollution source identification and apportionment using PMF and PCA-APCA-MLR receptor models in a typical mixed land-use area in Southwestern China. Sci. Total Environ. 741, 140383. doi:10.1016/j.scitotenv.2020.140383
Zhang, H., Li, H., Yu, H., and Cheng, S. (2020b). Water quality assessment and pollution source apportionment using multi-statistic and APCS-MLR modeling techniques in Min River Basin, China. Environ. Sci. Pollut. Res. 27, 41987–42000. doi:10.1007/s11356-020-10219-y
Zhang, H., Ren, X., Chen, S., Xie, G., Hu, Y., Gao, D., et al. (2024). Deep optimization of water quality index and positive matrix factorization models for water quality evaluation and pollution source apportionment using a random forest model. Environ. Pollut. 347, 123771. doi:10.1016/j.envpol.2024.123771
Zhang, Q., Zhang, J., Wang, H., Zhai, T., Liu, L., Li, G., et al. (2023). Spatial patterns in water quality and source apportionment in a typical cascade development river southwestern China using PMF modeling and multivariate statistical techniques. Chemosphere 311, 137139. doi:10.1016/j.chemosphere.2022.137139
Keywords: groundwater, Siwa oasis, source appointement, health risk, PTES, Nubian Aquifer system
Citation: Eid MH, Mikita V, Eissa M, Ramadan HS, Mohamed EA, Abukhadra MR, El-Sherbeeny AM, Bellucci S, Kovács A and Szűcs P (2024) Monte Carlo simulation and PMF model for assessing human health risks associated with heavy metals in groundwater: a case study of the Nubian aquifer, Siwa depression, Egypt. Front. Earth Sci. 12:1431635. doi: 10.3389/feart.2024.1431635
Received: 12 May 2024; Accepted: 04 September 2024;
Published: 27 September 2024.
Edited by:
Guochang Wang, Saint Francis University, United StatesReviewed by:
Jun Xiao, Chinese Academy of Sciences (CAS), ChinaAntonije Onjia, University of Belgrade, Serbia
Copyright © 2024 Eid, Mikita, Eissa, Ramadan, Mohamed, Abukhadra, El-Sherbeeny, Bellucci, Kovács and Szűcs. This is an open-access article distributed under the terms of the Creative Commons Attribution License (CC BY). The use, distribution or reproduction in other forums is permitted, provided the original author(s) and the copyright owner(s) are credited and that the original publication in this journal is cited, in accordance with accepted academic practice. No use, distribution or reproduction is permitted which does not comply with these terms.
*Correspondence: Mohamed Hamdy Eid, bW9oYW1lZGhhbWR5QHNjaWVuY2UuYnN1LmVkdS5lZw==; Stefano Bellucci, c3RlZmFuby5iZWxsdWNjaUBsbmYuaW5mbi5pdA==