Seabed classification of multibeam echosounder data into bedrock/non-bedrock using deep learning
- 1NTNU, Department of Circulation and Medical Imaging, Trondheim, Norway
- 2Kongsberg Discovery, Horten, Norway
- 3The Geological Survey of Norway (NGU), Trondheim, Norway
A Corrigendum on
Seabed classification of multibeam echosounder data into bedrock/non-bedrock using deep learning
by Garone RV, Birkenes Lønmo TI, Schimel ACG, Diesing M, Thorsnes T and Løvstakken L (2023). Front. Earth Sci. 11:1285368. doi: 10.3389/feart.2023.1285368
In the published article, there were minor inaccuracies, specifically concerning the metrics values for multiple-input models (Table 2) and misclassification values in Table 3. The corrections also extend to the corresponding confusion matrices in the Supplementary Material.
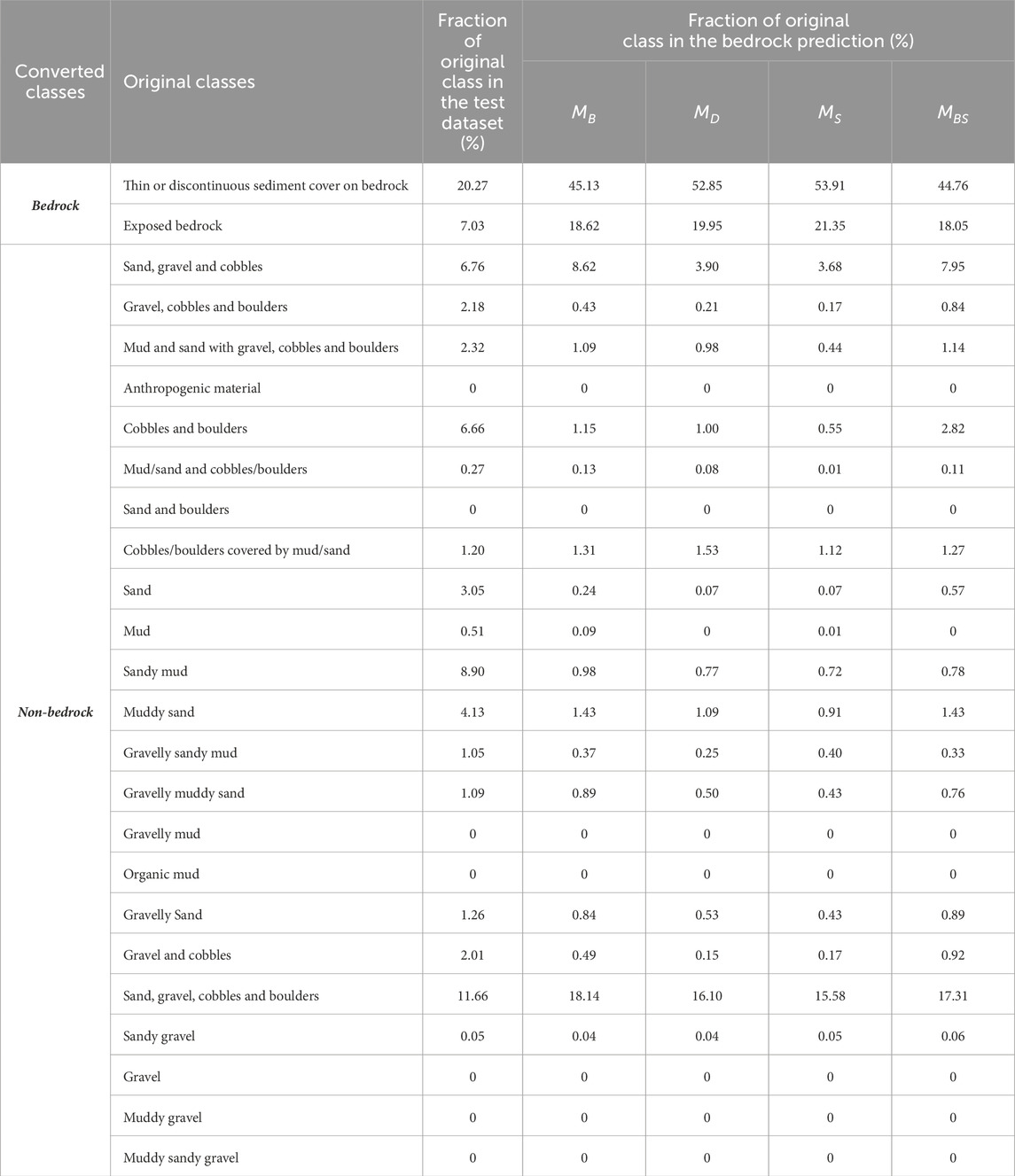
Table 3. The table analyzes the over-prediction of the bedrock class resulting in pixels predicted as bedrock even if belonging to a different original sediment class. The over-prediction of the bedrock was quantified by dividing the number of pixels of each original class predicted as bedrock, by the total number of pixels predicted as bedrock. These results are displayed respectively for the backscatter, depth, slope and the backscatter and depth models in the column “Fraction of original class in the bedrock prediction (%)”. A column showing the fraction of original sediment classes in the test dataset (%) has also been added. To be noted that the sum of percentages in this column adds up to 80.37%, the remaining 19.63% belongs the background class, not included in the calculation.
In Table 2, the sub-headers PAcc and UAcc were interchanged. In addition, the metrics for the multiple-input models have been re-evaluated to reflect minor miscalculations in the code. The corrected Table 2 and its caption appear below.
In Table 3, there was a slight miscalculation of some of the statistical values in the columns under “Fraction of original class in the bedrock prediction (%)”, and a transcription error in the value for the original class “Sand, gravel and cobbles” for the model MB. The corrected Table 3 and its caption appear below.
In 3 Results, paragraph 1, it was stated: “The results for the multiple-input models confirmed the higher predictive power of the depth and slope over backscatter, as all the models incorporating backscatter data (MBD, MBH and MBS) consistently showed lower performance metrics. Noticeably, while MDS displayed the highest metrics among the multiple-input models, it did not outperform the single-input models MD and MS.” The corrected paragraph is as follows:
“The results for the multiple-input models confirmed the higher predictive power of the depth and slope over backscatter, as all the multiple-input models incorporating backscatter data (MBD, MBH and MBS) consistently showed lower performance metrics than the corresponding single-input models without backscatter data (respectively, MD, MH, and MS). Noticeably, no multiple-input models outperformed the best single-input models.”
In 3 Results, paragraph 3, it was stated: “This observation is confirmed by the results listed in Table 2 where the UAcc values for the bedrock class for all the models are higher than the corresponding PAcc ones”. To address the mislabeling of “PAcc” and “UAcc”, the sentence has been corrected as follows:
“This observation is confirmed by the results listed in Table 2 where the PAcc values for the bedrock class for all the models are higher than the corresponding UAcc ones.”
In 3 Results, paragraph 4, it was stated: “While the models generally over-predict the bedrock class, as seen from the higher UAcc values compared to the corresponding PAcc values (Table 2) and from Figure 9, instances of under-prediction are also evident.” To address the mislabeling of “PAcc” and “UAcc”, the sentence has been corrected as follows:
“While the models generally over-predict the bedrock class, as seen from the higher PAcc values compared to the corresponding UAcc values (Table 2) and from Figure 9, instances of under-prediction are also evident.”
In 3 Results, paragraph 5, it was stated: ‘As an example of the table interpretation, for the original class “exposed bedrock” and for the model
‘As an example of the table interpretation, for the original class “exposed bedrock” and for the model
In 4 Discussion, paragraph 3, it was stated: “MBD, MBH and MBS showed comparable performance to one another but a lower performance compared to the single-layer depth models (Table 2).” The sentence has been corrected as follows:
“MBD, MBH and MBS showed a varied range of performance, but it was in each case lower compared to the corresponding single-layer model without the backscatter layer (Table 2).”
To reflect the updated metric values for the multiple-input models, Supplementary Figures S5–S10 have been updated.
The authors apologize for these errors and state that this does not change the scientific conclusions of the article in any way. The original article has been updated.
Publisher’s note
All claims expressed in this article are solely those of the authors and do not necessarily represent those of their affiliated organizations, or those of the publisher, the editors and the reviewers. Any product that may be evaluated in this article, or claim that may be made by its manufacturer, is not guaranteed or endorsed by the publisher.
Keywords: deep-learning, seabed, segmentation, multibeam, backscatter, bathymetry, classification
Citation: Garone RV, Birkenes Lønmo TI, Gregory Schimel AC, Diesing M, Thorsnes T and Løvstakken L (2024) Corrigendum: Seabed classification of multibeam echosounder data into bedrock/non-bedrock using deep learning. Front. Earth Sci. 12:1415557. doi: 10.3389/feart.2024.1415557
Received: 10 April 2024; Accepted: 23 April 2024;
Published: 16 May 2024.
Edited and reviewed by:
Davide Oppo, University of Louisiana at Lafayette, United StatesCopyright © 2024 Garone, Birkenes Lønmo, Gregory Schimel, Diesing, Thorsnes and Løvstakken. This is an open-access article distributed under the terms of the Creative Commons Attribution License (CC BY). The use, distribution or reproduction in other forums is permitted, provided the original author(s) and the copyright owner(s) are credited and that the original publication in this journal is cited, in accordance with accepted academic practice. No use, distribution or reproduction is permitted which does not comply with these terms.
*Correspondence: Rosa Virginia Garone, rosa.v.garone@ntnu.no