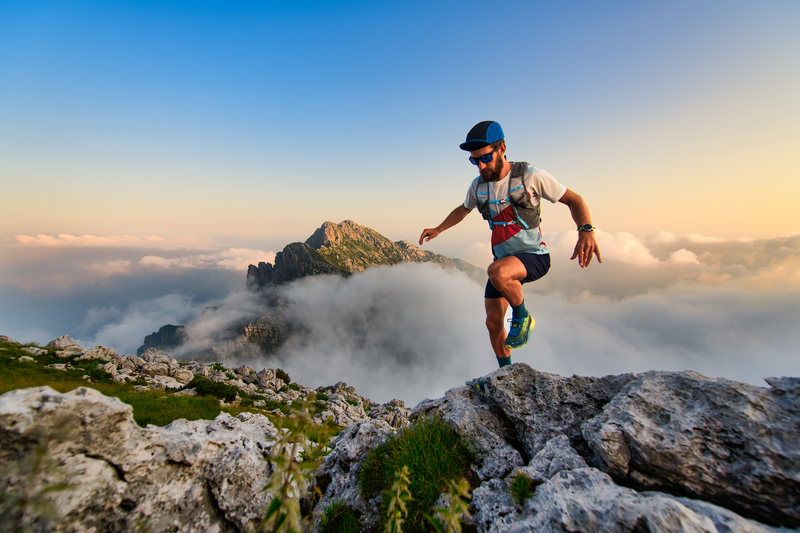
95% of researchers rate our articles as excellent or good
Learn more about the work of our research integrity team to safeguard the quality of each article we publish.
Find out more
METHODS article
Front. Earth Sci. , 09 June 2023
Sec. Cryospheric Sciences
Volume 11 - 2023 | https://doi.org/10.3389/feart.2023.960363
The Greenland Ice Sheet is a leading source of global sea level rise, due to surface meltwater runoff and glacier calving. However, given a scarcity of proglacial river gauge measurements, ice sheet runoff remains poorly quantified. This lack of in situ observations is particularly acute in Northwest Greenland, a remote area releasing significant runoff and where traditional river gauging is exceptionally challenging. Here, we demonstrate that georectified time-lapse camera images accurately retrieve stage fluctuations of the proglacial Minturn River, Inglefield Land, over a 3 year study period. Camera images discern the river’s wetted shoreline position, and a terrestrial LiDAR scanner (TLS) scan of riverbank microtopography enables georectification of these positions to vertical estimates of river stage. This non-contact approach captures seasonal, diurnal, and episodic runoff draining a large (∼2,800 km2) lobe of grounded ice at Inglefield Land with good accuracy relative to traditional in situ bubble-gauge measurements (r2 = 0.81, Root Mean Square Error (RMSE) ±0.185 m for image collection at 3-h frequency; r2 = 0.92, RMSE ±0.109 m for resampled average daily frequency). Furthermore, camera images effectively supplement other instrument data gaps during icy and/or low flow conditions, which challenge bubble-gauges and other contact-based instruments. This benefit alone extends the effective seasonal hydrological monitoring period by ∼2–4 weeks each year for the Minturn River. We conclude that low-cost, non-contact time-lapse camera methods offer good promise for monitoring proglacial meltwater runoff from the Greenland Ice Sheet and other harsh polar environments.
The Greenland Ice Sheet is a leading contributor to global sea-level rise due to surface meltwater runoff and marine glacier calving (King et al., 2018; Shepherd et al., 2020; van den Broeke et al., 2016). Surface mass balance (SMB) models estimate that surface meltwater runoff is becoming the primary contributor (50.3%) of all Greenland meltwater compared to dynamic losses (49.7%) from glacial calving and ice discharge from 1992–2018 (Shepherd et al., 2020). Calving mass losses are relatively well-constrained by satellite and airborne remote sensing of outlet glacier ice surface velocities and elevations (King et al., 2020). Surface meltwater runoff, however, is poorly constrained by observations, with in situ records of proglacial river discharge obtained almost exclusively from Southwest Greenland (e.g., Bartholomew et al., 2011, Bartholomew et al., 2012; Hasholt et al., 2013; Mankoff et al., 2020; Mikkelsen et al., 2016; Rennermalm et al., 2013; Russell, et al., 1995; Smith et al., 2015, 2021; Tedstone et al., 2013; Tedstone, 2017; van As et al., 2014; van As et al., 2017; van As et al., 2018; van As et al., 2020).
In principle, satellite remote sensing could be used to monitor proglacial river discharge elsewhere around the ice sheet (e.g., Durand et al., 2014; Gleason and Smith, 2014; Feng et al., 2021; Li et al., 2022), but such methods are less accurate and limited by the size of the river, satellite observational frequency, and lack of ground measurements for calibration and validation (Smith et al., 1995; Smith et al., 1996; Smith, 1997; Bjerklie et al., 2018; Li et al., 2022). Our knowledge of current and future ice sheet runoff is therefore predominantly derived from SMB models which do not account for flow routing and contain biases (e.g., Overeem et al., 2015; Smith et al., 2015; Smith et al., 2017; van As et al., 2018; Yang et al., 2019a; Mouginot et al., 2019). Such models benefit from in situ runoff observations for calibration and validation, yet such observations are challenging to obtain in remote, harsh Arctic environments (Gleason et al., 2015).
In general, comparative studies suggest most climate models tend to overestimate ice sheet runoff relative to SMB models (Overeem et al., 2015), the physical reasons for which are not well understood but may be related to water retention processes on the bare ice surface (Smith et al., 2017; Cooper et al., 2018; Yang et al., 2018; Cooper and Smith, 2019; Muthyala et al., 2022). However, this conclusion is based largely on proglacial discharge studies in SW Greenland, where virtually all supraglacial runoff enters moulins and the subglacial system, introducing substantial storages and/or delays between surface runoff generation and its delivery to the proglacial zone, which make direct comparisons of proglacial discharge and surface runoff models challenging (Smith et al., 2015; Smith et al., 2017). In contrast, the proglacial Minturn River in Inglefield Land, Northwest Greenland is well suited for validating climate/SMB models, as this area of the ice sheet generates runoff flowing entirely across the ice surface to the proglacial zone without interference from en- or sub-glacial flow pathways and/or storage (Yang et al., 2019b; Li et al., 2022).
Traditional river gauging requires installation of a bubble-gauge, pressure transducer, stilling well/float, or comparable sensor into the water to measure river stage (Rantz, 1982; Sauer, 2002; Sauer and Turnipseed, 2010). Such in-channel sensors are difficult to maintain in remote, icy environments due to cost, power, safety, and reliability constraints. In addition, in situ instruments often fail to capture important melt season features such as ice break-up and freeze-up (Beltaos and Prowse, 2009). To overcome such challenges, the use of time-lapse camera images to monitor changing proglacial river water levels and/or inundation area has been explored (Ashmore and Sauks, 2006; Gleason et al., 2015; Overeem et al., 2015; Smith et al., 2015). Some studies have also used short 15 s videos to calculate streamflow velocity. These studies show promising results for future camera-based discharge measurements; however, they are limited by significant power consumption that is poorly suited for remote polar environments (Stumpf et al., 2016; Eltner et al., 2020). Other studies have used time-lapse cameras to estimate river stage in settings outside of Greenland (Young et al., 2015; Leduc et al., 2018; Lin et al., 2018). Such studies show promising results of the reliability of time-lapse images to estimate river stage.
To estimate stage, time-lapse camera images should be georectified to provide absolute changes in water levels in meters rather than pixels. While river discharge is an important measurement, in this study we focus on river stage only. To convert stage to discharge, a rating curve using in situ gauges and streamflow rate measurement is required (Sauer, 2002). Subsequent discharge measurements acquired in a follow-up study are available from Esenther et al. (in review, 2023). Gleason et al. (2015) used photogrammetry and satellite data to correct their images. Alternatively, a Terrestrial LiDAR Scanner (TLS) survey could obtain a high precision, georeferenced digital elevation model (DEM) of the imaged river bank (e.g., Kociuba et al., 2014; Longoni et al., 2016). TLS surveys have previously been combined with time-lapse images for various purposes, such as for quantifying ice flow velocities and subsidence (e.g., James et al., 2016) and streamflow monitoring (e.g., Stumpf et al., 2016; Peña-Haro et al., 2021). However, to our knowledge TLS surveying has not previously been combined with time-lapse camera images to monitor river stage variations in remote polar environments. Such environments present numerous data collection challenges, and the high quality of TLS data presents exciting possibilities for future work.
Here, we demonstrate the utility of ground-based oblique time-lapse camera images, georectified using a TLS survey, to derive a 3-year (2019–2021) record of river stage at 3-h intervals at a single location on the Minturn River in Inglefield Land, Northwest Greenland (78.591°, −68.988°). First, we visually inspect the time-lapse image records to determine ice break-up and freeze-up dates for each melt season. Next, we use a semi-automated method to derive a unique wetted shoreline position in each of the 1,618 usable time-lapse camera images. We then use PyTrx, an open source, object-oriented toolset (How et al., 2020), for extracting physical measurements from monoscopic time-lapse images, to georectify the images to a high-resolution TLS survey DEM. Shoreline positions are then converted to georeferenced vertical estimates of river stage. Finally, we validate the derived stage time-series using independent stage measurements from a standard, in situ bubble-gauge. Using the bubble-gauge as ground-truth, we estimate errors in our camera-based approach, and conclude with a discussion of its opportunities and limitations for low-cost, non-contact river gauging in harsh polar environments.
Time-lapse camera images were collected on the main stem of the Minturn River, a major proglacial river draining a ∼2,800 km2 supraglacial catchment draining a large grounded lobe of the Greenland Ice Sheet in Inglefield Land, Northwest Greenland (Figure 1). The Minturn River evacuates supraglacial runoff from cold-bedded, slow-moving ice that experiences extensive surface melting during summer (Yang et al., 2019b; Li et al., 2022). Absence of en- and subglacial flow processes ensures that virtually all ice-sheet runoff measured at our gauging station originates from surface mass balance processes operating on the ice surface. Selection of a gauging site on the Minturn River ∼15 km downstream of the ice edge enables collection of runoff from the proglacial zone’s largest river, fed by multiple coalescing proglacial streams, and an ice catchment sufficiently large for comparison with regional climate models. Selection of this downstream location on the Minturn River also ensured higher discharges (and thus larger stage variations) necessary for camera-based estimation of river stage changes. This site is uniquely well-suited for monitoring ice sheet surface meltwater runoff because the upstream supraglacial catchment contains virtually no moulins or crevasse fields due to regional compression and a gentle surface slope (Yang et al., 2019b; Li et al., 2022). This enables the survival of long, fully supraglacial streams that traverse entirely over the ice sheet surface to its edge without delays in proglacial discharge (in direct contrast to SW Greenland, where nearly all supraglacial streams enter moulins, Pitcher and Smith, 2019; Smith et al., 2015). From there, a well-defined system of incised bedrock-channel proglacial streams collect the ice sheet runoff and convey it overland through the Minturn River into the Nares Strait.
FIGURE 1. The Minturn River catchment drains ∼2,800 km2 of the NW Greenland Ice Sheet ablation zone. Red lines delineate the boundary of the Minturn River watershed derived from 10 m ArcticDEM, using the gauging location as the watershed outlet. Red dot indicates the location of our field site. Blue lines indicate river and tributaries of the Minturn River derived from 10 m Arctic DEM (see Li et al., 2022 for further details about the approach). Inset shows overall location of the study area in Greenland. Basemap: Google, Landsat, Copernicus.
From July 8–11, 2019, we installed an instrument monitoring network on the Minturn River. The instruments used in this study include an in situ bubble-gauge (see Section 3.4) and two ruggedized time-lapse camera systems (Figure 2). The camera system was designed and built by the U.S. Army Corps of Engineers Cold Regions Research and Engineering Laboratory (CRREL) to routinely collect and telemeter images from this remote location using an Iridium satellite dial-up modem. The camera is a StarDot SD500B SC NetCam with a StarDot Technologies Megapixel Lens with 5-megapixel resolution (LEN-MV4510CS), mounted on a 1.5-m mast that is bolted and guy-wired into underlying rock. The lens has an adjustable focal length, which was adjusted in the field for optimal viewer geometry and focus. The camera installed on the west bank was used in this study and acquires one digital image every 3 h when there is sufficient ambient light for good picture exposure (controlled through the use of an integrated light sensor) and power. Power to the system is supplied by a battery and 90 W photovoltaic solar panel. The power consumption of the camera was not the only limiting factor in the frequency of images captured and transmitted. Image upload time, battery power, and ambient lighting all contribute to the frequency of images that can be captured and telemetered. The upload time to send the captured images to the server via Iridium satellite modem was the limiting factor. Upload of a single image could take over 1-h depending on satellite connection quality. The satellite modem is the largest power consumer at the study site. The camera was set to “Automatic” mode, meaning that the shutter speed, ISO and aperture were optimized for each image based on the lighting conditions. The images are transmitted to a file transfer protocol via the Iridium modem and time-stamped in Coordinated universal Time. The camera used in this study has an oblique field-of-view aimed directly across the river to image the right (east) bank of the river channel, from which the wetted shoreline position is clearly evident as a semi-horizontal line (Figure 3).
FIGURE 2. On-site photo of our Minturn River gauging station, including a bubble-gauge and both ruggedized StarDot SD500B SC NetCam time-lapse cameras. Photo taken 2022-08-20 by Cuyler Onclin.
FIGURE 3. Example time-lapse camera images of a fixed field-of-view across the river. The extent of the river at high flow is ∼46 m. The water edge (i.e., wetted shoreline position) is clearly evident despite varying illumination and flow conditions, including (A) typical illumination; (B) sun glint; (C) low illumination; (D) low flow; (E) ice breakup/flow onset; and (F) freeze-up/flow cessation. Images were acquired (A) 2021-07-22 03:00:00; (B) 2021-07-22 21:00:00; (C) 2021-07-29 00:00:00; (D) 2021-09-03 00:00:00; (E) 2021-06-08 00:00:00; (F) 2019-09-08 09:00:00 UTC 0.
We conducted a Terrestrial LiDAR Scanner (TLS) survey July 10–11, 2019 to generate a high-precision DEM of the Minturn River field site and surrounding riverbanks. The TLS instrument was a Riegl VZ-400i, which has a reported instrument accuracy of 2 cm (RIEGL - Produktdetail, 2022). The TLS survey included 27 scan positions acquired over the 2 day period. To enable global registration, external 10 cm cylinder reflectors were placed within each scan scene and surveyed using a Septentrio Altus NR3 GNSS receiver and rapid-static survey techniques. All scan positions were registered together via reflector registration and multi-station adjustment techniques as per the Riegl RiSCAN Pro User Manual. The maximum scanner range at the pulse rate used was reported at 250 m. The raw point cloud density is variable across the area of interest. An “octree” filter was applied in order to grid the full resolution point cloud and generate a sub-sampled DEM with 2 cm resolution (point cloud density of 2,500 points/m2).
We derive river stage from time-lapse camera images using a two-step approach: 1) develop a time series of wetted shoreline positions from oblique time-lapse camera images; and 2) georectify the oblique camera images to convert the derived shoreline positions into vertical units of river stage. These steps were implemented as follows:
A total of 3,544 time-lapse camera images were acquired at 3-h intervals between July 12 and December 17 in 2019, between June 21 and December 31 in 2020, and between January 1 and September 17 in 2021. The 12 July 2019 start date commenced with camera installation. The 21 June 2020 and 1 January 2021 start dates occurred when the camera’s battery became sufficiently charged. The end dates occurred when the camera’s battery lost sufficient charge. Ice break-up and freeze-up processes were determined by visual inspection (except spring 2019). The ice break-up and freeze-up processes were actually extremely rapid and occurred in the 3-h interval between camera images. Out of the 3,544 images acquired, 1,783 (50.3%) were removed because they were acquired before/after the meltwater runoff season in the river channel. After filtering, 1,761 images collected from July 12 to 7 September 2019, from June 21 to 1 September 2020, and from June 8 to 5 September 2021 remained. Further visual inspection revealed that eleven (0.006%) images were dark and/or obscured by dust, rain or snow. These images were also removed from our image collection prior to analysis. An additional 41 images in 2020 and 91 in 2021 were missing due to telemetry receiving errors. In total, 1,618 high-quality images (455 in 2019, 533 in 2020, and 630 in 2021) remained for final analysis.
We used a semi-automated edge detection approach to delineate the wetted shoreline position in each camera image using open-source software packages (SciPy, SciKit-Image, Pandas, Numpy, MatPlotLib, and PyTrx) in a Python programming environment. In the time-lapse images, the water typically appears dark and land bright but, depending on illumination conditions, the reverse also occurs. Under most light conditions, the contrasting edge between land and water is readily apparent. A Canny edge detection method (Canny, 1986) was used to delineate the shoreline edge as part of a semi-automated procedure as follows: First, we applied a 3x3 Gaussian filter to smooth an RGB composite of each image. The Canny Edge Python package automatically generates a greyscale composite from an imported RGB image by averaging the three imported image bands (Figures 4A, D, G). Then we used a Canny edge detector (σ = 3) to generate an array of edge and non-edge pixels (Figures 4B, E, H). The row in each image containing the most edge values was then visually reviewed to confirm that the detected edge correctly located the wetted shoreline position on the side of the image where the edge detection was clearest (Figures 4C, F, I). Next, we applied a correction for the prevailing water edge slope across the field of view (a consistent ∼40 pixel height difference amounting to 1.9 m between the left and right sides of the image). We had to generate a line because the algorithm detected a number of edges that are not part of the water-land boundary. This semi-automated method retrieved shoreline positions for 72.3%, 63.0%, and 47.9% of images in 2019, 2020, and 2021, respectively. Shoreline positions from the remaining images were manually estimated by inspecting each image and identifying the row of the wetted shoreline position approximately in the middle columns of the image. Our semi-automated method yielded a time series of 1,618 shoreline positions over the study period.
FIGURE 4. Canny edge detection results under different lighting and flow conditions. The left column presents three greyscale images acquired 2019-07-12 (A), 2019-08-10 (D), 2019-09-04 (G). The middle column (B,E,H) presents the results of applying Canny edge detection to each image, the x-axis presenting the number of edge pixels identified for each row and the y-axis corresponding to the row (y-axis) of each camera image. The right column (C,F,I) presents the results of the Canny filtering, with a red horizontal line indicating the row containing the most Canny edge pixels to estimate wetted shoreline position.
We georectified the 1,618 derived shoreline positions using PyTrx (How et al., 2020). The toolset was originally developed for measuring glacier velocities and feature geometries in Svalbard, but is here used to georectify our time-lapse image collection of the Minturn River (How et al., 2017, 2019). The CamEnv class in PyTrx is used to perform the georectification by inverse-projecting the images onto the TLS survey derived DEM. This requires detailed knowledge of the camera environment, namely, camera location and orientation, an intrinsic camera model (i.e., focal length, principal point, and skew), lens distortion coefficients, and ground control points (GCPs). PyTrx uses one image (i.e., a master) to estimate camera orientation (yaw, pitch, roll). All images are then co-registered to this master image, thereby accounting for shifts in the camera orientation. There was no evidence for camera shake in the images. The Applied Imagery Quick Terrain Reader (https://appliedimagery.com) was used to manually gather three GCPs and camera location with 2 cm position accuracy from our TLS DEM of the study site (Figure 5). PyTrx does not use GCPs in the calibration step itself, rather they are used in the georectification process. The camera angle makes it challenging to add more GCPs, and for the purpose of PyTrx’s georectification process, three GCPs are sufficient. PyTrx optimization routines were used to refine the projection model, based on the difference between the positions of the physical GCPs and the projected GCPs (for more details on PyTrx see How et al., 2020).
FIGURE 5. Georectifications of camera images were performed using a Terrestrial Lidar Scanner (TLS) DEM and PyTrx software. Corresponding ground control points (GCPs) from PyTrx georectification are marked on (A) a 2019-07-12 camera image; and (B) 2019-07-11 TLS DEM. White areas signify no data due to low laser returns, e.g., from the river surface and topographic shadowing. Successful GCP projection (excepting areas of no data), indicates accurate georectification with a resulting residual error of 11.63 pixels and vertical accuracy of ±0.37 m.
Projecting the camera image onto the DEM was challenging because the far bank is irregular. It is evenly sloped just above the waterline, then levels out, before sloping up again (Figure 5A). Despite that, we were able to project the camera image with a vertical accuracy of ±0.21 m. Even though the residuals between the actual and projected GCP positions were non-negligible, we were still able to derive accurate river stage from the camera images because the projection of the camera image in the vertical direction is most important for converting pixels to units of length (Section 4).
That the TLS survey was not conducted during minimum flow presented a challenge, in that we could not derive the topography of the lowest ∼75% of the riverbank. We therefore extrapolated the submerged topography using a linear regression model based on the exposed, georectified bank slope. Comparison of the resultant stage estimates in this extrapolated zone with our bubble-gauge validation data yields reasonably good correlation (r2 = 0.61), lending confidence to this procedure (Section 5). To further improve our confidence in the sensitivity of our results, we extended the extrapolation plane to the exposed, georectified bank slope. For the above water points, we compared the DEM-derived stage to the estimated planar stage with the following results by year: 2019: RMSE = 0.01 m, r2 = 0.99; 2020: RMSE = 0.01 m, r2 = 0.99; 2021: RMSE = 0.02 m, r2 = 0.99.
The end result is 1,618 estimates of water surface elevation (in meters), referenced to WGS84/UTM Zone 19N (horizontal), WGS84 Ellipsoid Height (vertical), EPSG code 32619 (https://epsg.io/32619).
We evaluated our Minturn River stage estimates derived from TLS georectified time-lapse camera images against simultaneously-collected stage measurements collected from an in situ bubble-gauge located near the time-lapse camera. The installed bubble-gauge is a Sutron® Compact Constant Flow Bubbler, a standard, high-quality instrument for measuring stage in rivers, streams, and lakes. The Compact CF Bubbler is housed within a vented galvanized pipe bolted to boulders on the left (west) bank of the Minturn River channel and programmed to record stage (in centimeters above the submerged sensor) every 15 min. It has a nominal accuracy of 0.05% and minimum error of 0.003 m, and its measurements are here treated as absolute ground-truth for the purpose of validating our camera-derived stage estimates. However, it is possible that the bubble-gauge orifice shifts during and/or between meltwater runoff seasons. At our Minturn River field site, the Compact CF Bubbler becomes exposed at low flow when there is still observable flow in the river channel. The compact bubbler only was able to measure water stage when submerged. Due to access to the site and the level of the river at the time of installation, the team was unable to get the exit orifice of the bubbler (where the stage measurement is made) to the bottom of the river channel. Therefore, when the river level was below the bubbler, the bubbler was simply measuring atmospheric pressure and not water level. The bubbler failed, likely on 29 July 2021, when the protective conduit was struck, shearing off the exit orifice. This was likely caused by a large upstream boulder impacting the conduit.
To compare the two datasets, we selected observations from the bubble-gauge (every 0.25 h) with the observation time nearest to the time-lapse camera image collection (every 3 h). Additionally, both bubble-gauge and time-lapse image data were resampled to derive a daily mean stage estimate to facilitate comparison with other runoff models. Daily data resampling also allows us to focus on seasonal changes, which are commonly used in climate/SMB models. We then compared the two river stage datasets using coefficient of determination (r2) and Root Mean Square Error (RMSE).
We find that georectified time-lapse camera imagery can accurately quantify river stage fluctuations in a harsh polar environment. Strong correlations exist between camera-derived and in situ Minturn River stage measurements, with r2 values of 0.82 (RMSE ±0.059 m), 0.73 (±0.065 m), and 0.76 (±0.079 m) for 2019, 2020, and 2021, respectively (Figure 6). Resampling both datasets to daily average stage values further improves correlations and reduces the RMSE to r2=0.95 (RMSE: ±0.100 m), 0.96 (±0.076 m), and 0.86 (±0.152 m), respectively. With yearly fluctuations of 6–10 m, our RMSEs are 0.02%–0.03% of the total range in observed water level. While camera-derived estimates do effectively capture diurnal fluctuations in river stage, they overestimate them relative to in situ bubble-gauge measurements (Figure 7). The bubble-gauge orifice may likely have shifted slightly on 29 July 2021, which partially accounts for less accurate measurements that year. However, due to safety considerations, the shift could not be accounted for in the field. Averaged over the 3-year study period, our approach yields river stage estimates with r2 = 0.81 (RMSE ±0.185 m) at 3-h frequency; and r2 = 0.92 (RMSE ±0.109 m) at daily frequency, relative to in situ validation measurements.
FIGURE 6. Correlations between Minturn River stage estimates as measured by an in situ bubble-gauge and TLS-georectified camera images acquired from 2019-2021. The top row (A–C) presents comparisons of the two datasets at 3-h intervals (A: r2 =0.82, RMSE ±0.059 m; B: r2 =0.73 ±0.065 m; C: r2 =0.76 ±0.079 m), and the bottom row (D–F) presents comparisons of the two datasets averaged to daily intervals (D: r2 =0.95, RMSE: ±0.100 m; E: r2 = 0.96 ±0.076 m; F: r2 =0.86 ±0.152 m). The worsening estimations (i.e., in 2021), can be partially explained by the shift in the bubble gauge orifice described in Section 4 and the abnormal 2021 hydrograph is more thoroughly discussed in Esenther et al. (in review, 2023).
FIGURE 7. Time series of Minturn River stage for 2019, 2020, and 2021. Each plot shows river stage as derived from TLS orthorectified camera imagery (in black), and a standard in situ bubble-gauge (in blue). The 2019 observations extend from July 12 (date of installation) to September 2 (freeze-up). The 2020 stage observations extend from June 21 (ice breakup) to September 1 (freeze-up). 2021 camera observations extend from June 8 (ice breakup) to September 5 (freeze-up). In all 3 years the non-contact camera installation acquired longer records with fewer data gaps than the standard in situ instrument. Points w and x in (A) and (B) indicate late season flood events. Points y and z in (C) indicate the peak (y) and trough (z) pattern. Red dashed lines show where the waterline was at time of TLS scan. Camera-based stage estimates below this line were extrapolated based on bank topography.
Camera-derived river stage retrievals successfully capture important characteristics of the Minturn River hydrological cycle (Figure 7). While the date of ice breakup/flow onset was missed in 2019 (due to July installation of the instrument cluster), it was successfully detected in 2020 (on June 21) and 2021 (on June 8). In all 3 years the camera retained sufficient power through the summer to observe flow cessation and ice freeze-up, which occurred on 2 September 2019, 1 September 2020, and 5 September 2021. The camera record extends stage measurements by ∼2–4 weeks for each year relative to the bubble-gauge, especially late in the season when flows were too low to be detected by the in situ sensor.
Overall, Minturn River stages were notably higher in 2019 and 2020 than 2021, indicating greater meltwater runoff from the ice sheet (Figures 7A, B vs. Figure 7C). In 2019 and 2020, high stages persisted throughout July, followed by an overall decline interrupted by a large late-season flood in August (w and x in Figures 7A, B). In 2020, this late season event yielded the highest stage of the year. In contrast, July flows in 2021 displayed a pronounced peak and trough pattern through early August (y and z in Figure 7C), followed by the customary decline but no late-season flood (Figure 7C).
Time-lapse camera imagery georectified with high precision Terrestrial LiDAR Scanner (TLS) DEM offers a reliable, accurate, non-contact approach for monitoring river stage in remote, harsh polar environments. While the camera-based approach is less precise than traditional in situ methods, the combined TLS-camera method is sufficiently accurate, and provides a number of practical advantages, especially considering the challenges of maintaining traditional in situ instruments (e.g., bubble-gauge or pressure transducer). High-quality georectification afforded by a TLS survey, in particular, enables conversion of imaged wetted shoreline positions to absolute vertical river stage. This approach captures the timing of flow onset and cessation, and overall flow variations at interannual, seasonal, and diurnal scales. Advantages of this approach include installation ease and relatively low cost and maintenance requirements. Since the camera is not submerged, it is far simpler to service and avoids destruction from ice, which is particularly problematic for high-latitude river gauging.
Another advantage of camera-based imaging is its ability to capture water levels at lower flows than a traditional bubble-gauge. Remote proglacial rivers such as the Minturn River are typically fast-flowing and difficult to access, often preventing installation of a bubble-gauge in the very deepest part of the channel. At our Minturn River field site, the Compact CF Bubbler was exposed at low flow (i.e., yielding a value of 0 in Figure 7) when there is still significant flow in the river channel, leading to significant data gaps of ∼2–4 weeks per year. Time-lapse cameras, in contrast, can observe water levels at any stage and can also record the dates of annual ice break-up and freeze-up timing, an observation of scientific value for characterizing climatic trends (Smith, 2000; Beltaos and Prowse, 2009; Eltner et al., 2020; Magnusson et al., 2000; Yang et al., 2020).
Two notable scientific observations from this study are 1) significantly lower (∼-47%) overall Minturn River water levels in 2021, relative to 2019 and 2020; and 2) occurrence of episodic late-season floods in both 2019 and 2020, which peaked on August 30 and 21, respectively (Figure 7A-w, Figure 7B-x). Because raindrops were present on the camera lens during the latter, we suggest that at least one of these late-season runoff peaks may have been triggered by rainfall rather than glacial melt. However, interpretation of the meteorological and glaciological drivers of the observed stage hydrographs is beyond the scope of this paper and appears in Esenther et al., 2023.
Disadvantages of the combined TLS-camera approach presented here include occasional image obscuration due to darkness, dust, or precipitation, a finding consistent with previous studies reporting problems of dust, precipitation, shadowing, and/or animal interference (Ashmore and Sauks, 2006; Gleason et al., 2015; Leduc et al., 2018). Camera-derived stages are less accurate than in situ bubble-gauge measurements, especially at a shorter 3-h interval. While a TLS survey works well for georectification, it provides no data below the waterline, thus necessitating downward extrapolation of the bank slope to examine the channel during low flow conditions if conducted when the river channel is occupied by water. While this yielded reasonably good results in our particular study, its success is likely due to the near-vertical sheerness of the imaged bank (a relatively uncommon bank morphology). Future studies should thus target TLS surveys during winter (when the channel is dry) or lowest flows possible, in order to maximize coverage of the channel bathymetry. Additionally, while the semi-automated edge detection yielded promising results in our study, other approaches to correct for the prevailing water edge slope, such as locating scan lines parallel to the slope, projecting edge pixels directly onto the DEM, or projecting height contours from the DEM onto the image could improve the accuracy of the method.
These limitations aside, we conclude that time-lapse camera imaging and subsequent georectification using a TLS DEM offers a simple, non-contact method for effective river stage monitoring in remote polar environments, with r2 values of 0.81 (RMSE ±0.185 m) and r2 = 0.92 (RMSE ±0.109 m) for 3-hourly and daily observations, respectively. It is challenging to compare the accuracy of our study with others because previous studies were carried out in very different fluvial settings (e.g., Lin et al., 2018), did not evaluate river stage specifically (e.g., Stumpf et al., 2016), or did not compare to an external source of stage data (e.g., Young et al., 2015). Leduc et al. (2018) compared their camera-derived water levels with those recorded by a pressure transducer and found a r2 = 0.82 (R = 0.91), which is comparable to our results, although the small, boulder stream that they measured is much smaller than the Minturn River. Despite that, the accuracy of our results and other studies indicate that camera-based river stage has great potential for monitoring rivers in remote environments, especially when georectified using a high-quality TLS DEM.
While the focus of this study was to obtain measurements of river stage, this metric only provides relative changes in river flow. To convert these stage measurements to discharge, a rating curve would need to be produced. Traditional approaches to producing stage-discharge rating curves also require streamflow rate measurements (Sauer, 2002). But the oblique-looking terrestrial cameras may also be useful for this purpose (Stumpf et al., 2016). For the Greenland Ice Sheet, camera-based approaches may therefore be particularly valuable for testing and validation of climate/SMB models used to predict future ice sheet contributions to sea level rise, including from the Minturn River in Inglefield Land, a remote, little-studied, region of Northwest Greenland.
The datasets and code analyzed and generated in this study can be found in the code repository: DOI: 10.5281/zenodo.7951337.
SG conducted image and statistical analysis and drafted the manuscript. JR, PH, EK provided computational assistance. JR, PH, EK, JF, and LS assisted with conceptualizing the camera-TLS method. SE, LP, AL, and BO provided technical assistance with instrumentation. LP, AL, and BO conducted field work to install the instrument cluster. All authors contributed to the article and approved the submitted version.
This research was funded by the NASA Cryospheric Science Program (grant 80NSSC19K0942) managed by Dr. Thorsten Markus. Supplemental undergraduate research support was provided by a Voss Undergraduate Research Fellowship at Brown University.
Polar Field Services, Inc. and Thule Air Force Base provided logistical field support. TLS equipment, ruggedized camera design, in situ bubble-gauge design, and data telemetry were provided by the U.S. Army Corps of Engineers Cold Regions Research and Engineering Laboratory (CRREL). Cuyler Onclin provided invaluable logistics, safety, and technical support in the field. Galen Winsor provided computational assistance.
The authors declare that the research was conducted in the absence of any commercial or financial relationships that could be construed as a potential conflict of interest.
All claims expressed in this article are solely those of the authors and do not necessarily represent those of their affiliated organizations, or those of the publisher, the editors and the reviewers. Any product that may be evaluated in this article, or claim that may be made by its manufacturer, is not guaranteed or endorsed by the publisher.
Ashmore, P., and Sauks, E. (2006). Prediction of discharge from water surface width in a braided river with implications for at-a-station hydraulic geometry. Water Resour. Res. 42. doi:10.1029/2005WR003993
Bartholomew, I., Nienow, P., Sole, A., Mair, D., Cowton, T., Palmer, S., et al. (2011). Supraglacial forcing of subglacial drainage in the ablation zone of the Greenland ice sheet. Geophys. Res. Lett. 38. doi:10.1029/2011GL047063
Bartholomew, I., Nienow, P., Sole, A., Mair, D., Cowton, T., and King, M. A. (2012). Short-term variability in Greenland Ice Sheet motion forced by time-varying meltwater drainage: Implications for the relationship between subglacial drainage system behavior and ice velocity. J. Geophys. Res. 117, F03002. doi:10.1029/2011JF002220
Beltaos, S., and Prowse, T. (2009). River-ice hydrology in a shrinking cryosphere. Hydrol. Process. 23, 122–144. doi:10.1002/hyp.7165
Bjerklie, D. M., Birkett, C. M., Jones, J. W., Carabajal, C., Rover, J. A., Fulton, J. W., et al. (2018). Satellite remote sensing estimation of river discharge: Application to the Yukon River Alaska. J. Hydrology 561, 1000–1018. doi:10.1016/j.jhydrol.2018.04.005
Canny, J. (1986). A computational approach to edge detection. IEEE Trans. Pattern Analysis Mach. Intell. PAMI- 8, 679–698. doi:10.1109/TPAMI.1986.4767851
Cooper, M. G., Smith, L. C., Rennermalm, A. K., Miege, C., Pitcher, L. H., Ryan, J. C., et al. (2018). Meltwater storage in low-density near-surface bare ice in the Greenland ice sheet ablation zone. Cryosphere 12 (3), 955–970. doi:10.5194/tc-12-955-2018
Cooper, M. G., and Smith, L. C. (2019). Satellite remote sensing of the Greenland ice sheet ablation zone: A review. Remote Sens. 11 (20), 2405. doi:10.3390/rs11202405
Durand, M., Neal, J., Rodríguez, E., Andreadis, K. M., Smith, L. C., and Yoon, Y. (2014). Estimating reach-averaged discharge for the River Severn from measurements of river water surface elevation and slope. J. Hydrology 511, 92–104. doi:10.1016/j.jhydrol.2013.12.050
Eltner, A., Sardemann, H., and Grundmann, J. (2020). Technical Note: Flow velocity and discharge measurement in rivers using terrestrial and unmanned-aerial-vehicle imagery. Hydrology Earth Syst. Sci. 24, 1429–1445. doi:10.5194/hess-24-1429-2020
Esenther, S. E., Smith, L. C., LeWinter, A., Pitcher, L. H., Overstreet, B. T., Kehl, A., et al. (2023). New hydrometeorological observations from Inglefield land and Thule, NW Greenland. Geoscientific Instrum. Methods Data Syst. Discuss., 1–30. doi:10.5194/gi-2023-3
Feng, D., Gleason, C. J., Lin, P., Yang, X., Pan, M., and Ishitsuka, Y. (2021). Recent changes to Arctic river discharge. Nat. Commun. 12, 6917. doi:10.1038/s41467-021-27228-1
Gleason, C. J., Smith, L. C., Finnegan, D. C., LeWinter, A. L., Pitcher, L. H., and Chu, V. W. (2015). Technical Note: Semi-automated effective width extraction from time-lapse RGB imagery of a remote, braided Greenlandic river. Hydrol. Earth Syst. Sci. 19, 2963–2969. doi:10.5194/hess-19-2963-2015
Gleason, C. J., and Smith, L. C. (2014). Toward global mapping of river discharge using satellite images and at-many-stations hydraulic geometry. PNAS 111, 4788–4791. doi:10.1073/pnas.1317606111
Hasholt, B., Bech Mikkelsen, A., Holtegaard Nielsen, M., and Andreas Dahl Larsen, M. (2013). Observations of runoff and sediment and dissolved loads from the Greenland ice sheet at kangerlussuaq, west Greenland, 2007 to 2010. Stuttgart, Germany: Zeitschrift für Geomorphologie. Supplementary Issues 3–27. doi:10.1127/0372-8854/2012/S-00121
How, P., Benn, D. I., Hulton, N. R. J., Hubbard, B., Luckman, A., Sevestre, H., et al. (2017). Rapidly changing subglacial hydrological pathways at a tidewater glacier revealed through simultaneous observations of water pressure, supraglacial lakes, meltwater plumes and surface velocities. Cryosphere 11, 2691–2710. doi:10.5194/tc-11-2691-2017
How, P., Hulton, N. R. J., Buie, L., and Benn, D. I. (2020). PyTrx: A python-based monoscopic terrestrial photogrammetry toolset for glaciology. Front. Earth Sci. 8, 21. doi:10.3389/feart.2020.00021
How, P., Schild, K. M., Benn, D. I., Noormets, R., Kirchner, N., Luckman, A., et al. (2019). Calving controlled by melt-under-cutting: Detailed calving styles revealed through time-lapse observations. Ann. Glaciol. 60, 20–31. doi:10.1017/aog.2018.28
James, M. R., How, P., and Wynn, P. M. (2016). Pointcatcher software: Analysis of glacial time-lapse photography and integration with multitemporal digital elevation models. J. Glaciol. 62, 159–169. doi:10.1017/jog.2016.27
King, M. D., Howat, I. M., Candela, S. G., Noh, M. J., Jeong, S., Noël, B. P. Y., et al. (2020). Dynamic ice loss from the Greenland Ice Sheet driven by sustained glacier retreat. Commun. Earth Environ. 1, 1–7. doi:10.1038/s43247-020-0001-2
King, M. D., Howat, I. M., Jeong, S., Noh, M. J., Wouters, B., Noël, B., et al. (2018). Seasonal to decadal variability in ice discharge from the Greenland Ice Sheet. Cryosphere 12, 3813–3825. doi:10.5194/tc-12-3813-2018
Kociuba, W., Kubisz, W., and Zagórski, P. (2014). Use of terrestrial laser scanning (TLS) for monitoring and modelling of geomorphic processes and phenomena at a small and medium spatial scale in Polar environment (Scott River — spitsbergen). Geomorphol. Spec. Issue Stream Catchment Dyn. 212, 84–96. doi:10.1016/j.geomorph.2013.02.003
Leduc, P., Ashmore, P., and Sjogren, D. (2018). Technical note: Stage and water width measurement of a mountain stream using a simple time-lapse camera. Hydrol. Earth Syst. Sci. 22, 1–11. doi:10.5194/hess-22-1-2018
Li, Y., Yang, K., Gao, S., Smith, L. C., Fettweis, X., and Li, M. (2022). Surface meltwater runoff routing through a coupled supraglacial-proglacial drainage system, Inglefield Land, northwest Greenland. Int. J. Appl. Earth Observation Geoinformation 106, 102647. doi:10.1016/j.jag.2021.102647
Lin, Y.-T., Lin, Y.-C., and Han, J.-Y. (2018). Automatic water-level detection using single-camera images with varied poses. Measurement 127, 167–174. doi:10.1016/j.measurement.2018.05.100
Longoni, L., Papini, M., Brambilla, D., Barazzetti, L., Roncoroni, F., Scaioni, M., et al. (2016). Monitoring riverbank erosion in mountain catchments using terrestrial laser scanning. Remote Sens. 8, 241. doi:10.3390/rs8030241
Mankoff, K. D., Noël, B., Fettweis, X., Ahlstrøm, A. P., Colgan, W., Kondo, K., et al. (2020). Greenland liquid water discharge from 1958 through 2019. Earth Syst. Sci. Data 12, 2811–2841. doi:10.5194/essd-12-2811-2020
Mikkelsen, A. B., Hubbard, A., MacFerrin, M., Box, J. E., Doyle, S. H., Fitzpatrick, A., et al. (2016). Extraordinary runoff from the Greenland ice sheet in 2012 amplified by hypsometry and depleted firn retention. Cryosphere 10, 1147–1159. doi:10.5194/tc-10-1147-2016
Mouginot, J., Rignot, E., Bjørk, A. A., Broeke, M., Millan, R., Morlighem, M., et al. (2019). Forty-six years of Greenland Ice Sheet mass balance from 1972 to 2018. PNAS 116, 9239–9244. doi:10.1073/pnas.1904242116
Muthyala, R., Rennermalm, A., Leidman, S., Cooper, M., Cooley, S., Smith, L., et al. (2022). Supraglacial streamflow and meteorological drivers from southwest Greenland. Cryosphere 16 (6), 2245–2263. doi:10.5194/tc-16-2245-2022
Overeem, I., Hudson, B., Welty, E., Mikkelsen, A., Bamber, J., Petersen, D., et al. (2015). River inundation suggests ice-sheet runoff retention. J. Glaciol. 61, 776–788. doi:10.3189/2015JoG15J012
Peña-Haro, S., Carrel, M., Lüthi, B., Hansen, I., and Lukes, R. (2021). Robust image-based streamflow measurements for real-time continuous monitoring. Front. Water 3. doi:10.3389/frwa.2021.766918
Rantz, S. E. (1982). USGS numbered series 2175. U.S. GPO doi:10.3133/wsp2175Measurement and computation of streamflow, Measurement and computation of streamflow
Rennermalm, A. K., Smith, L. C., Chu, V. W., Box, J. E., Forster, R. R., Van den Broeke, M. R., et al. (2013). Evidence of meltwater retention within the Greenland ice sheet. Cryosphere 7, 1433–1445. doi:10.5194/tc-7-1433-2013
RIEGL - Produktdetail [WWW Document], 2022. Available at: http://www.riegl.com/nc/products/terrestrial-scanning/produktdetail/product/scanner/48/ (accessed 5.13.22).
Russell, A. J., Van Tatenhove, F. G. M., and Van De Wal, R. S. W. (1995). Effects of ice-front collapse and flood generation on a proglacial river channel near kangerlussuaq (Søndre Strømfjord), west Greenland. Hydrol. Process. 9, 213–226. doi:10.1002/hyp.3360090207
Sauer, V. B. (2002). Standards for the analysis and processing of surface-water data and information using electronic methods, standards for the analysis and processing of surface-water data and information using electronic methods. USGS numbered series 2001–4044. Reston, VA: U.S. Geological Survey. doi:10.3133/wri20014044
Sauer, V. B., and Turnipseed, D. P. (2010). Stage measurement at gaging stations (USGS Numbered Series No. 3-A7), Stage measurement at gaging stations, Techniques and Methods. Reston, VA: U.S. Geological Survey. doi:10.3133/tm3A7
Shepherd, A., Ivins, E., Rignot, E., Smith, B., van den Broeke, M., Velicogna, I., et al. (2020). Mass balance of the Greenland ice sheet from 1992 to 2018. Nature 579, 233–239. doi:10.1038/s41586-019-1855-2
Smith, L. C., Andrews, L. C., Pitcher, L. H., Overstreet, B. T., Rennermalm, Å. K., Cooper, M. G., et al. (2021). Supraglacial River forcing of subglacial water storage and diurnal ice sheet motion. Geophys. Res. Lett. 48, e2020GL091418. doi:10.1029/2020GL091418
Smith, L. C., Chu, V. W., Yang, K., Gleason, C. J., Pitcher, L. H., Rennermalm, A. K., et al. (2015). Efficient meltwater drainage through supraglacial streams and rivers on the southwest Greenland ice sheet. PNAS 112, 1001–1006. doi:10.1073/pnas.1413024112
Smith, L. C., Isacks, B. L., Bloom, A. L., and Murray, A. B. (1996). Estimation of discharge from three braided rivers using synthetic aperture radar satellite imagery: Potential application to ungaged basins. Water Resour. Res. 32, 2021–2034. doi:10.1029/96WR00752
Smith, L. C., Isacks, B. L., Forster, R. R., Bloom, A. L., and Preuss, I. (1995). Estimation of discharge from braided glacial rivers using ERS 1 synthetic aperture radar: First results. Water Resour. Res. 31, 1325–1329. doi:10.1029/95WR00145
Smith, L. C. (1997). Satellite remote sensing of river inundation area, stage, and discharge: A review. Hydrol. Process. 11, 1427–1439. doi:10.1002/(SICI)1099-1085(199708)11:10<1427:AID-HYP473>3.0.CO;2-S
Smith, L. C. (2000). Trends in Russian arctic river-ice formation and breakup, 1917 to 1994. Phys. Geogr. 21, 46–56. doi:10.1080/02723646.2000.10642698
Smith, L. C., Yang, K., Pitcher, L. H., Overstreet, B. T., Chu, V. W., Rennermalm, Å. K., et al. (2017). Direct measurements of meltwater runoff on the Greenland ice sheet surface. PNAS 114, E10622–E10631. doi:10.1073/pnas.1707743114
Stumpf, A., Augereau, E., Delacourt, C., and Bonnier, J. (2016). Photogrammetric discharge monitoring of small tropical mountain rivers: A case study at rivière des pluies, réunion island. Water Resour. Res. 52 (6), 4550–4570. doi:10.1002/2015WR018292
Tedstone, A. J., Nienow, P. W., Sole, A. J., Mair, D. W. F., Cowton, T. R., Bartholomew, I. D., et al. (2013). Greenland ice sheet motion insensitive to exceptional meltwater forcing. Proc. Natl. Acad. Sci. 110, 19719–19724. doi:10.1073/pnas.1315843110
Tedstone, A. (2017). Proglacial discharge measurements. south-west Greenland: Leverett Glacier, 2009–2012. doi:10.5285/17C400F1-ED6D-4D5A-A51F-AAD9EE61CE3D
van As, D., Andersen, M. L., Petersen, D., Fettweis, X., Angelen, J. H. V., Lenaerts, J. T. M., et al. (2014). Increasing meltwater discharge from the Nuuk region of the Greenland ice sheet and implications for mass balance (1960–2012). J. Glaciol. 60, 314–322. doi:10.3189/2014JoG13J065
van As, D., Bech Mikkelsen, A., Holtegaard Nielsen, M., Box, J. E., Claesson Liljedahl, L., Lindbäck, K., et al. (2017). Hypsometric amplification and routing moderation of Greenland ice sheet meltwater release. Cryosphere 11, 1371–1386. doi:10.5194/tc-11-1371-2017
van As, D., Fausto, R. S., Meierbachtol, T., Box, J. E., and Claesson Liljedahl, L. (2020). Greenland ice sheet meltwater runoff from the Kangerlussuaq and Isunnguata Sermia catchments using observation-based surface energy balance modeling, 2009–2019. doi:10.22008/FK2/GUW0Y8
van As, D., Hasholt, B., Ahlstrøm, A. P., Box, J. E., Cappelen, J., Colgan, W., et al. (2018). Reconstructing Greenland ice sheet meltwater discharge through the watson river (1949–2017). Arct. Antarct. Alp. Res. 50, S100010. doi:10.1080/15230430.2018.1433799
van den Broeke, M. R., Enderlin, E. M., Howat, I. M., Kuipers Munneke, P., Noël, B. P. Y., van de Berg, W. J., et al. (2016). On the recent contribution of the Greenland ice sheet to sea level change. Cryosphere 10, 1933–1946. doi:10.5194/tc-10-1933-2016
Yang, K., Smith, L. C., Fettweis, X., Gleason, C. J., Lu, Y., and Li, M. (2019a). Surface meltwater runoff on the Greenland ice sheet estimated from remotely sensed supraglacial lake infilling rate. Remote Sens. Environ. 234, 111459. doi:10.1016/j.rse.2019.111459
Yang, K., Smith, L. C., Karlstrom, L., Cooper, M. G., Tedesco, M., van As, D., et al. (2018). A new surface meltwater routing model for use on the Greenland Ice Sheet surface. Cryosphere 12 (12), 3791–3811. doi:10.5194/tc-12-3791-2018
Yang, K., Smith, L. C., Sole, A., Livingstone, S. J., Cheng, X., Chen, Z., et al. (2019b). Supraglacial rivers on the northwest Greenland ice sheet, devon ice cap, and barnes ice cap mapped using sentinel-2 imagery. Int. J. Appl. Earth Observation Geoinformation 78, 1–13. doi:10.1016/j.jag.2019.01.008
Yang, X., Pavelsky, T. M., and Allen, G. H. (2020). The past and future of global river ice. Nature 577, 69–73. doi:10.1038/s41586-019-1848-1
Keywords: non-contact river gauge, terrestrial LiDAR scanner (TLS), photogrammetry, Greenland ice sheet, meltwater runoff, hydrology
Citation: Goldstein SN, Ryan JC, How PR, Esenther SE, Pitcher LH, LeWinter AL, Overstreet BT, Kyzivat ED, Fayne JV and Smith LC (2023) Proglacial river stage derived from georectified time-lapse camera images, Inglefield Land, Northwest Greenland. Front. Earth Sci. 11:960363. doi: 10.3389/feart.2023.960363
Received: 02 June 2022; Accepted: 29 May 2023;
Published: 09 June 2023.
Edited by:
Ramanathan Alagappan, Jawaharlal Nehru University, IndiaReviewed by:
Marin Kneib, Swiss Federal Institute for Forest, Snow and Landscape Research (WSL), SwitzerlandCopyright © 2023 Goldstein, Ryan, How, Esenther, Pitcher, LeWinter, Overstreet, Kyzivat, Fayne and Smith. This is an open-access article distributed under the terms of the Creative Commons Attribution License (CC BY). The use, distribution or reproduction in other forums is permitted, provided the original author(s) and the copyright owner(s) are credited and that the original publication in this journal is cited, in accordance with accepted academic practice. No use, distribution or reproduction is permitted which does not comply with these terms.
*Correspondence: Seth N. Goldstein, c2V0aF9nb2xkc3RlaW5AYWx1bW5pLmJyb3duLmVkdQ==
Disclaimer: All claims expressed in this article are solely those of the authors and do not necessarily represent those of their affiliated organizations, or those of the publisher, the editors and the reviewers. Any product that may be evaluated in this article or claim that may be made by its manufacturer is not guaranteed or endorsed by the publisher.
Research integrity at Frontiers
Learn more about the work of our research integrity team to safeguard the quality of each article we publish.