- 1School of Earth Sciences and Resources, China University of Geosciences, Beijing, China
- 2Institute of Geomechanics, Chinese Academy of Geological Sciences, Beijing, China
- 3Key Laboratory of Active Tectonics and Geological Safety, Ministry of Natural Resources, Beijing, China
- 4Research Center of Neotectonism and Crustal Stability, China Geological Survey, Beijing, China
On September 5, 2022, a magnitude 6.8 earthquake occurred along the Xianshuihe Fault Zone in Luding County, Tibetan Plateau, China, leading to a significant outbreak of landslides. The urgent need for a swift and accurate evaluation of earthquake-induced landslides distribution in the affected area prompted this study. This research delves into regional geological data, scrutinizes post-earthquake Peak Ground Acceleration (PGA) and Arias Intensity (Ia) associated with the Luding earthquake, and conducts earthquake-induced landslides risk assessments within the Luding earthquake zone using the Newmark model. Validation of the earthquake-induced landslides risk assessment outcomes rooted in PGA and Ia relies on an earthquake-induced landslides database, revealing Area Under the Curve (AUC) values of 0.73 and 0.84 in respective ROC (Receiver Operating Characteristic) curves. These results unequivocally affirm the exceptional accuracy of earthquake-induced landslides evaluation using Ia calculations, emphasizing its suitability for the swift prediction and evaluation of earthquake-induced landslides. The earthquake-induced landslides risk assessment based on Ia computation reveals the area with extremely high-risk and high-risk of earthquake-induced landslides encompass 0.71% of the entire study area. Notably, these areas are predominantly clustered within seismic intensity VII zones and primarily trace the Moxi fault zone, extending from the southern portion of the middle east along the Dadu River and the Moxi fault, with reach up to Dewei Township in the north and Caoke Township in the south. Hazard-prone regions predominantly align with slopes featuring gradients of 30°–45° and bear a strong correlation with fault activity. Furthermore, the results of this evaluation are harmonious with the findings from remote sensing interpretation and on-site field investigations pertaining to the earthquake-induced landslides. This body of knowledge can serve as a crucial reference for expedited assessment, emergency response and subsequent supplementation of earthquake-induced landslide databases when confronting similar earthquake-induced landslide scenarios.
1 Introduction
On September 5, 2022, a strong earthquake of Ms6.8 occurred in Luding County, Sichuan Province (An et al., 2022; Xie et al., 2022), leading to the loss of life and damage to infrastructure properties. As of September 14, 118 people were reported missing or dead in areas with seismic intensity degree VIII and above, covering an area of 785 km2, primarily in Luding County and Shimian County (Han et al., 2022; Qu et al., 2022; Yang et al., 2022; Zhou et al., 2023). According to the China Seismic Network, the epicenter of the earthquake was located in the southwestern part of Moxi Town, Luding County (102.08°E, 29.59°N), with a focal depth of 16 km and a maximum seismic intensity of IX degrees (Qu et al., 2022; Sun et al., 2023). The earthquake occurred in the eastern part of the Tibetan Plateau, which has a complex topography and geological structure. The epicenter was located on the Moxi fault, which is situated in the southeastern section of the Xianshuihe fault zone. The Moxi fault is known for its sinistral strike-slip activity since the late Quaternary period and has clear fault traces. It has experienced at least five paleoseismic events since the Holocene period and is a fault with high activity and frequent earthquakes (Chen et al., 2016; An et al., 2022; Bai et al., 2022; Li et al., 2022). The landslides induced by the Luding earthquake were densely distributed along high and steep slopes such as the Xianshuihe fault zone and Dadu River, and were mainly small and medium-sized in the shallow surface layer (Fan et al., 2022a; Huang et al., 2022).
Earthquake-induced landslides are the result of slope sliding under the effect of earthquakes, and their distribution and development are influenced by internal dynamic geological processes (Zhang et al., 2013; Song et al., 2020a; Song et al., 2020b; Song et al., 2021). Earthquake-induced landslides are particularly severe and significant during strong earthquakes (Wang and Lin, 2010; Xu et al., 2014; Zhang et al., 2017; Fan et al., 2018). For instance, the 2005 Kashmir earthquake in India triggered 1,293 landslides (Owen et al., 2008; Shafique et al., 2016), the 2008 Wenchuan earthquake caused more than 200,000 landslides (Yin, 2008; Xu et al., 2014; Froude and Petley, 2018), the 2015 Lushan earthquake in China triggered 22,528 landslides (Xu et al., 2015; Yang et al., 2015), and the 2015 Nepal earthquake triggered more than 25,000 landslides (Gallen et al., 2016; Roback et al., 2018). The impact of geological disasters can last for decades after a strong earthquake, and the characteristics of mass disasters and chain effects of geological disasters become more pronounced, with the scale increasing significantly (Shen ad Wang, 2016; Tie et al., 2020). Therefore, accurately and rapidly identifying the distribution range of earthquake-induced geological disasters is crucial for emergency rescue and reconstruction efforts following an earthquake (Huang and Li, 2008; Yang et al., 2017; Iqbal et al., 2018).
Earthquake-induced landslides risk assessment is the determination of the probability of earthquake-induced landslides occurring in a particular area and time period. Researchers have made significant progress in developing methods for earthquake-induced landslides risk assessment, ranging from analyzing the relationship between earthquake-induced landslides and seismic faults, magnitude, and epicentral distance to using expert experience, data mining, and statistical analysis-based index system methods (Nowicki Jessee et al., 2018; Tang et al., 2020; Jafarian et al., 2021; Fan et al., 2022b; Liu et al., 2022) and the Newmark cumulative displacement method based on mechanical principles (Chousianitis et al., 2014; Zang et al., 2020; Gupta and Satyam, 2022).
However, earthquake-induced landslides are influenced by various factors, and the mechanism of formation is complex, making it challenging to quickly obtain a complete catalog of earthquake-induced landslides and the macrodisaster situation in the earthquake zone. This difficulty makes it challenging to perform rapid earthquake-induced landslides risk assessment using statistical analysis methods after an earthquake (Xu et al., 2013; Chen et al., 2020; Chen et al., 2022; Chen et al., 2022; Zhao et al., 2022).
The Newmark cumulative displacement model is an earthquake-induced landslides risk assessment method proposed based on the limit equilibrium theory of infinite slope (Newmark, 1965), which is an effective approach to assess the risk of earthquake-induced landslides by calculating the cumulative displacement of slopes under earthquake action. Unlike the index system approach, this model does not rely on cataloging earthquake-induced landslides, and it is not limited by differences in geological background, allowing for a rapid predictive evaluation of post-earthquake-induced landslides groups (Jibson, 1993; Wang et al., 2015; Du et al., 2017; Liu et al., 2018; 2022; Gu o et al., 2021; Guo et al., 2021; Maharjan et al., 2021). Due to the scarcity of historical earthquake acceleration records, the strictly Newmark model, calculated based on acceleration integration, lacks widespread adaptability, so many scholars have made improvements to the Newmark model based on extensive statistical analysis results. By considering the characteristics of earthquake-induced landslides in different regions, relationships have been established between seismic slope displacement and earthquake-induced landslide occurrence probability, thereby enhancing the model’s adaptability (Jibson et al., 2000; Xu et al., 2012; Dreyfus et al., 2013). At present, the Newmark model has been well applied in the earthquake-induced landslides risk assessment in Chi-Chi Mw 7.6 earthquake, Wenchuan Ms 8.0 earthquake, China (Wang et al., 2013; Chen et al., 2014), Lushan Ms 7.0 earthquake (Yuan et al., 2016; Jin et al., 2019; Li et al., 2022), and Ludian Ms 6.5 earthquake (Zhao et al., 2022). Among the refined Newmark models, the simplified models developed by Rathje and Sayg. (2008) and Pareek et al. (2014), based on the study of statistical patterns of earthquake-induced landslides, exhibit good practicality (Rathje and Sayg, 2008; Chousianitis et al., 2014; Nowicki et al., 2014; Pareek et al., 2014). These models are applicable to the prediction of earthquake-induced landslides in high-mountain canyon areas with strong seismic activity.
After Luding earthquake, some research institutions and scholars have conducted extensive studies on the characteristics, distribution patterns, and earthquake-induced landslides induced by the Luding Ms6.8 earthquake (Fan et al., 2022a; Liu et al., 2022). These studies have generally clarified the spatial development and distribution characteristics of earthquake-induced landslides triggered by the Luding Ms6.8 earthquake. However, there remains considerable controversy regarding the rapid predictive assessment results and accuracy of earthquake-induced landslides. In order to better guide the rapid earthquake-induced landslides risk assessment in the future, this study, based on the establishment of an earthquake-induced landslides database, utilizes a simplified Newmark model, and conducts a comparative analysis of earthquake-induced landslides risk assessments under two conditions: Peak Ground Acceleration (PGA) and Arias intensity. The aim is to figure out a method that can better predict and recognize earthquake-induced landslides.
The results were compared with the earthquake-induced landslides distribution obtained through remote sensing interpretation. As a result, a more applicable method for the rapid prediction and evaluation of earthquake-induced landslides is proposed. The study aims to analyze the spatial analysis and development characteristics of earthquake-induced landslides in key areas and to discuss the rapid prediction and evaluation methods of earthquake-induced landslides. The research findings can provide insights into the development pattern of earthquake-induced landslides and guide disaster prevention and mitigation work.
2 Geological background
2.1 Active fault
The Luding earthquake epicenter is situated on the eastern boundary between the Bayankela Block and the Sichuan-Yunnan Rhombus Block, forming a “Y”-shaped intersection with the Longmenshan fault zone, Xianshuihe fault zone, Dadu River fault zone, and Anning River fault zone (Figure 1). The Xianshuihe fault zone has undergone a complex tectonic evolution history since the Paleozoic era and is known to have formed during this time (Roger et al., 1995; Xu et al., 2007; Guo et al., 2015a; Guo et al., 2015b; Li et al., 2022). This fault zone extends approximately 400 km, stretching from Ganzi Donggu in the north to the Anshunchang area of Shimian County in the south, with an overall trend of 320°–330°, forming an arc that curves toward the northeast (Qian, 1988; Xiong et al., 2010; Bai et al., 2014). The Xianshuihe fault zone consists of several faults, including the Luhuo fault, Daofu fault, Qianning fault, Yalahe fault, Zhonggu fault, Selaha fault, Zheduotang fault, Mugecuonan fault, and Moxi fault (Figure 1; Pan et al., 2020; Bai et al., 2021).
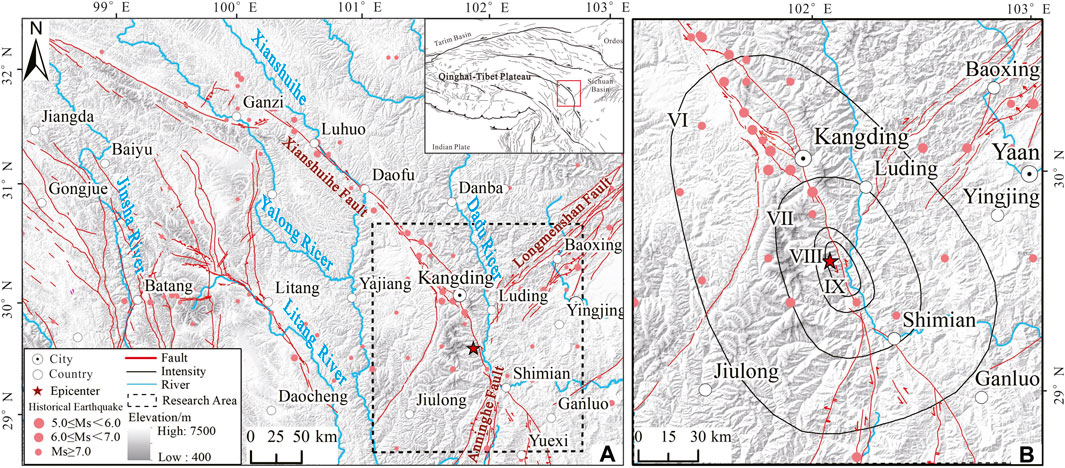
FIGURE 1. Structural location map of the Sichuan Luding earthquake on September 5, 2022: (A) Regional location map of the Xianshuihe fault zone, and (B) Luding earthquake research area.
Throughout history, the Xianshuihe fault zone has been frequently impacted by strong earthquakes. There have been 8 recorded earthquakes with a magnitude of Ms≥7.0 since 1725, making it a seismically active region (Li and Du, 1997; Cheng and Yang, 2002; Liu et al., 2022). The Luhuo Ms7.9 earthquake in 1973 was the most significant recorded earthquake in the area, and the closest strong earthquake was the Daofu Ms6.9 earthquake in 1981. The Moxi fault is characterized by high-speed left-lateral strike-slip movement, accompanied by a certain vertical component, since the late Quaternary period. The fault has a clear trace, and the strike-slip rate is estimated to be between 9.3–13.4 mm/a, while the vertical rate is between 1.5–3.2 mm/a. At least five paleoseismic events have occurred since the Holocene, indicating its seismogenic potential (Xu et al., 2003; Chen et al., 2016; Bai et al., 2022).
2.2 Characteristics of the Luding Ms6.8 earthquake
According to measurements recorded by the China Seismic Network, on September 5th, 2022, an earthquake of magnitude Ms6.8 occurred in Luding County, Sichuan Province, with a focal depth of 16 km and a maximum intensity of IX degrees (Qu et al., 2022; Sun et al., 2023). The United States Geological Survey (USGS) has reported the scalar seismic moment of this earthquake to be 1.158 × 1019 N m, with a moment magnitude of Mw6.64, and the centroid coordinates to be 29.726°N and 102.279°E, with a centroid depth of 15.5 km. The rupture model obtained by inversion indicates that the earthquake rupture surface is in the NNW‒SSE direction, with a rupture duration of 15 s. The entire rupture extends from the epicenter to the southeast direction, from deep to shallow, with a maximum slip of approximately 0.8 m (Dai et al., 2022; Li et al., 2022; Yang et al., 2022).
2.3 Basic characteristics of landslides induced by the Ms6.8 Luding earthquake
The Luding Ms6.8 earthquake triggered a significant number of earthquake-induced landslides. According to Tie et al. (2020) and Sun et al. (2023), the earthquake mainly consisted of medium and small clusters of high-level collapses and landslides, with a concentrated distribution of seismic intensity Ⅸ zone and high and steep slopes on both sides of the Dadu River. Specifically, the affected areas included Moxi Town, Detuo Town, Shimian County, Caoke Township, and Wanggangping Township (Figure 2A; Xie et al., 2022; Fan et al., 2022a; Huang et al., 2022; Xiao et al., 2023). While shallow surface slip occurred at the top of the Mogangling landslide, the overall stability of the landslide was not affected (Figure 2B). The Wandong River Basin, located in the seismic intensity Ⅸ zone, showed a dense development of geological disasters, with high-level collapses mostly occurring in the shallow surface layer. These collapses displayed extremely high density and were characterized by distribution along fault zones and river valleys (Figures 2C,D; Wang et al., 2022).
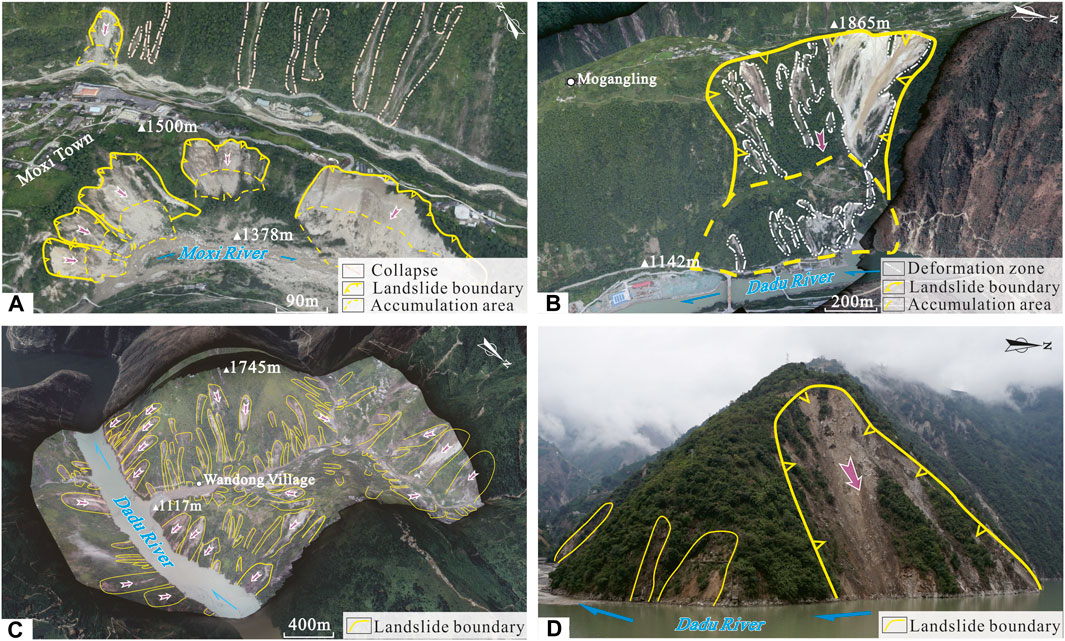
FIGURE 2. Typical landslides triggered by the Luding Ms6.8 earthquake: (A) Earthquake-induced landslides in the Moxi Platform; (B) Mogangling earthquake-induced landslides; (C) Earthquake-induced landslides in the Wandong River Basin, and (D) Earthquake-induced landslides in the estuary of the Wandong River (mirror-oriented NE).
3 Methodology and data
3.1 Data and sources
Seismic and geological data were utilized to evaluate the earthquake-induced slope displacement. The seismic data were sourced from the China Seismic Network, with the surface wave magnitude of the Luding earthquake recorded as 6.8 and the focal depth at 16 km. The digital elevation model (DEM) data were derived from the Panchromatic Remote Sensing Stereo Mapping Instrument (PRISM) aboard the ALOS satellite, with a resolution of the Luding zone. Terrain data with a resolution of 12.5 m were obtained from the NASA Earth Science Data Website (https://nasadaacs.eos.nasa.gov).
The regional stratigraphic data were acquired from the 1:200,000 geological map of the Yingjing map, and the stratigraphic units were divided into 13 engineering geological rock groups based on factors such as geological structure, stratum age, rock-soil mass type, and rock mass weathering and fragmentation degree (Figure 3B). The physical and mechanical parameters of the engineering geological rock groups were obtained by referring to the “Engineering Geology Handbook” (fifth edition) (Hua and Zheng, 2018) and combining the results of mechanical strength tests (Table 1).
To calculate the slope displacement induced by the Luding earthquake, the peak ground acceleration (PGA) and Arias intensity (Ia) were obtained from the State Key Laboratory of Chengdu University of Technology and the seismic moment magnitude and source distance of the earthquake, respectively. The cumulative displacement of the slope was then calculated based on the correlation expressions between earthquake-induced slope cumulative displacement (Dn), critical acceleration (ac), and PGA (Eq. 5), as well as the correlation expression between the Newmark cumulative displacement and critical acceleration (ac) and Arias intensity (Ia) (Eq. 6) proposed by previous studies (Harp EL Wilson, 1995; Jibson et al., 2000; Jibson, 2007) (Figures 4A,B).
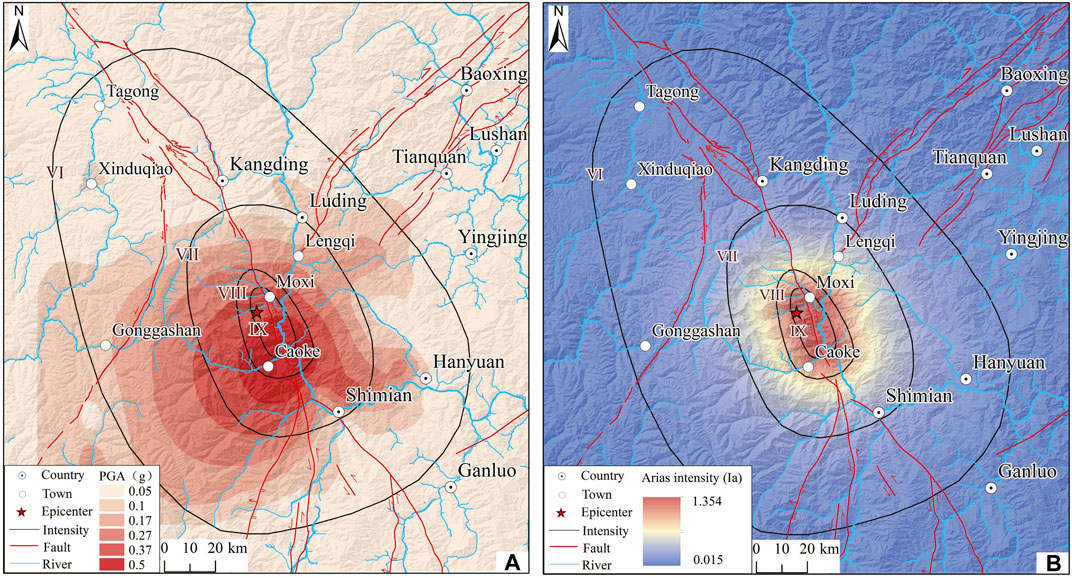
FIGURE 4. Peak ground acceleration (PGA) and Arias intensity (Ia) in the Luding earthquake zone: (A) Distribution map of peak ground acceleration (PGA) in the Luding earthquake zone (according to the Key Laboratory of Chengdu University of Technology), and (B) distribution map of the Arias intensity (Ia) in the Luding earthquake zone (calculated by earthquake data).
3.2 Earthquake-induced landslides risk assessment based on the Newmark model
The Newmark slope displacement model is a method for assessing the risk of earthquake-induced landslides. The method was proposed by British scientist Newmark in 1965 based on the limit equilibrium theory of infinite slopes. It is commonly used when the seismic structure is well understood or when earthquake intensity and parameter records are relatively complete. Wang et al. (2015) conducted an analysis of the factors affecting earthquake-induced landslides and risk inversion assessment using the Newmark model.
However, using the Newmark model based on the acceleration integral can be challenging in regional earthquake-induced landslides risk assessments. To address this issue, some scholars have introduced the regression model method. The relationship between ground motion parameters and Newmark cumulative displacement (Jibson, 2007; Jibson, 2011; Du et al., 2017), as well as the relationship between Newmark cumulative displacement and landslide probability (Jibson, 2011), have been studied.
To conduct a rapid risk assessment of regional earthquake-induced landslides, this paper uses the probabilistic seismic hazard analysis method to calculate the seismic probability and intensity of potential earthquake-affected slopes. With the help of empirical relations, the Newmark cumulative displacement model is used to obtain an approximate cumulative displacement value (Dn) for any slope in the region. The evaluation process is shown in Figure 5.
The aim of this study is to evaluate the landslide occurrence probability under earthquake action by analyzing the slope static safety factor (Fs), critical acceleration, and cumulative displacement. The calculation process is divided into four main steps, as follows: First, the slope static safety factor (Fs) is calculated using the slope safety factor formula based on the slider limit equilibrium theory proposed by Miles and Ho. (1999) and Jibson et al. (2000). This formula takes into account the physical and mechanical parameters of the rock and soil mass and the slope. Second, the limit equilibrium state equation of the slider under earthquake action is established by comparing the stress state of the slider under no external force and earthquake action. The critical acceleration of the slope is then calculated using the safety factor (Fs) and the equation proposed by Wilson and Keefer. (1983). Third, the relationship between the Arias intensity (Ia) and the peak ground acceleration (PGA) and the slope critical acceleration (ac) is calculated using the formulae proposed by Wang et al. (2013), Zhang et al. (2017), and Gupta and Satyam. (2022). This calculation enables the evaluation of the slope in the two cases of cumulative displacement (Dn). Finally, the landslide occurrence probability (P) is predicted and evaluated under earthquake action, according to the statistical law of slope cumulative displacement (Dn) and landslide occurrence probability (Jibson et al., 2000).
(1) Static safety factor (Fs)
In this study, the slope safety factor formula based on the slider limit equilibrium theory (Eq. 1; Jibson et al., 2000; Miles and Ho, 1999) is used to calculate the static safety factor (Fs) of the slope. This formula takes into account the physical and mechanical parameters of the rock and soil mass and slope, which are essential factors for the stability analysis of slopes.
where, c′ is the effective internal cohesion of the rock masses (kPa), φ′ is the effective internal friction angle of the rock masses (°), γ is the weight of the rock masses (kN/m3), γw is the weight of the groundwater (kN/m3), t is the thickness of the potential sliding masses (m), α is the dip angle of the potential sliding surface (°), and m is the proportion of the saturated portion in the total potential sliding masses.
(2) Critical acceleration (ac)
The stress state of the slider is compared under no external force and under the earthquake to establish the limit equilibrium state equation of the slider under earthquake conditions. The calculation formula of the critical acceleration (ac) is derived by using the safety factor (Fs) (Eq. 2; Wilson and Keefer, 1983).
where, g is the acceleration of gravity (m/s2) and α is the dip angle of the potential sliding surface (°).
(3) Arias intensity (Ia)
The Arias intensity (Ia) is used to determine the ground motion parameters in strong earthquake records. The Arias intensity is calculated by integrating the square of the ground motion acceleration over time and multiplying it by a constant (Arias, 1970). This method has the advantage of reflecting the amplitude, frequency, and duration of the ground motion, providing a comprehensive assessment of the seismic hazard. The Arias intensity is calculated by using the seismic moment magnitude Mw and the field source distance R (Eq. 3), and the moment Mw is converted by Eq. 4 (Wilson and Keefer, 1985; Zhang et al., 2017).
where Mw is the moment magnitude, Ms is the surface wave magnitude, and R is the source distance (km).
(4) Slope displacement (Dn)
Many studies have analyzed a large number of seismic acceleration records and earthquake-induced landslides examples to establish the correlation expressions of earthquake-induced slope cumulative displacement (Dn), critical acceleration (ac), and peak ground acceleration (PGA). The resulting correlation expressions (Eq. 5; Jibson, 2007) are important for understanding the behavior of slopes during earthquakes and for assessing the potential risk of landslides.
The Newmark cumulative displacement generated by regional earthquake-induced landslides and the critical acceleration (ac) and Arias intensity (Ia). This correlation is shown in Eq. 6, which was proposed by Jibson et al. (2000), Jibson (2007), and Harp EL Wilson. (1995). The correlation provides a useful tool for predicting the potential for landslides in areas affected by earthquakes.
In addition, this study also found a good functional correlation between the Newmark cumulative displacement generated by regional earthquake-induced landslides and the critical acceleration ac and Arias intensity (Ia). This correlation is shown in Eq. 6, which was proposed by Jibson et al. (2000), Jibson. (2007), and Harp EL Wilson. (1995). The correlation provides a useful tool for predicting the potential for landslides in areas affected by earthquakes.
where, ac is the critical acceleration of the slope (g), PGA is the peak ground acceleration (g), and Ia is the Arias intensity (m/s).
(5) Landslide occurrence probability (P)
According to the correlation between seismic slope displacement and landslide occurrence probability (Eq. 7; Jibson et al., 2000), the landslide occurrence probability (P) under earthquake action was calculated.
4 Rapid assessment of earthquake-induced landslide hazards
4.1 Static safety factor
Based on earthquake-induced landslides risk assessment experience, the slopes with slope degree of less than 5° are typically stable, and large-scale landslides are infrequent. Therefore, the calculations exclude slopes with slopes of less than 5° (Gupta and Satyam, 2022). Model parameters are adjusted through multiple cycle calculations to achieve a static safety factor (Fs) greater than 1 without external force. The parameters used are listed in Table 1 and include c', φ′, and γ, with γw set to 10 kN/m3, t set to 2.5 m, m set to 0.3, and α representing the terrain slope. Steep slopes, such as the banks of the Dadu River and the Moxi River, have lower static safety. In contrast, slopes with high static safety are typically distributed in areas with gentle slopes, such as the Tagong Grassland, Xinduqiao, and Yingjing County, where the static safety factor exceeds 5 (Figure 6A).
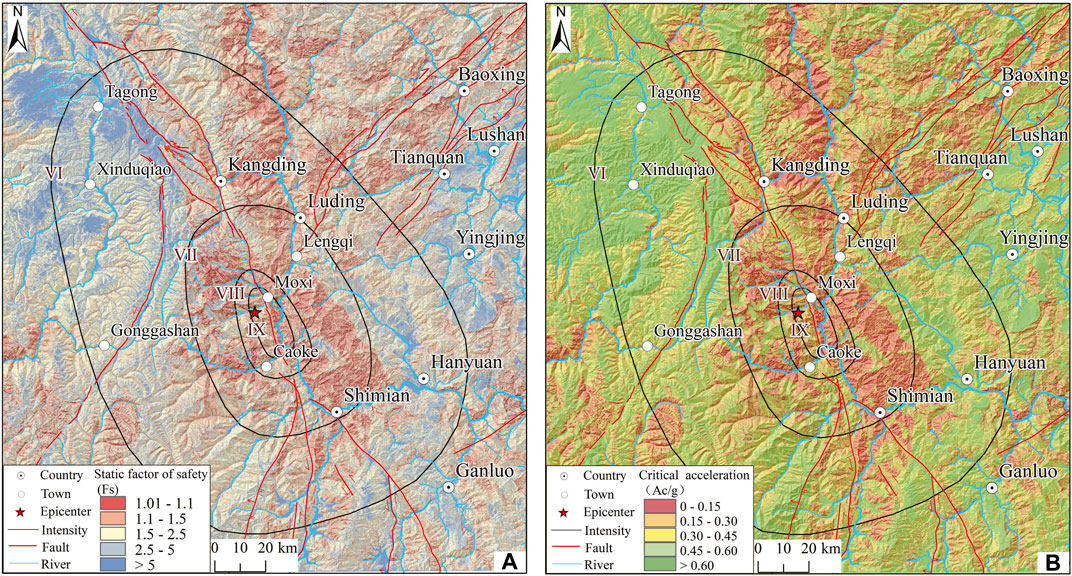
FIGURE 6. Distribution map of the static safety factor and critical acceleration of slope instability: (A) Static safety factor distribution map, and (B) Slope instability critical acceleration distribution map.
4.2 Critical acceleration of slope instability
The spatial distribution characteristics of the critical acceleration in the region have been calculated based on the static safety factor (Fs) and slope gradient. The stability of the region with a higher slope is found to be worse in correspondence to the static safety factor, indicating that the lower the static safety factor is, the smaller the critical acceleration required to induce slope instability. The critical acceleration on steep slopes along the Dadu River and near the Moxi River is less than 0.15 g, while in the valleys, basins, and Tagong Grasslands with gentle slopes, the critical acceleration is greater than 0.6 g, and the maximum can reach 0.93 g (Figure 6B).
4.3 Cumulative displacement of slope
(1) Based on the slope cumulative displacement calculated by PGA
Based on the PGA-based calculations, the cumulative displacement of the slope indicates that the displacement area is primarily distributed in the VII intensity zone (Figure 7A). The region with significant slope displacement is predominantly situated in the southeast direction of the earthquake, and it extends along the Dadu River and the Moxi Fault. The farthest affected area extends northward to Dewei Township and southward to the vicinity of Shimian County and the western side of Caoke Township along both sides of the Dadu River. The cumulative displacement of the slope in these areas can reach over 5 cm, and the cumulative displacement of the Wandong River Basin exceeds 10 cm.
(2) Cumulative slope displacement calculated based on the Arias intensity
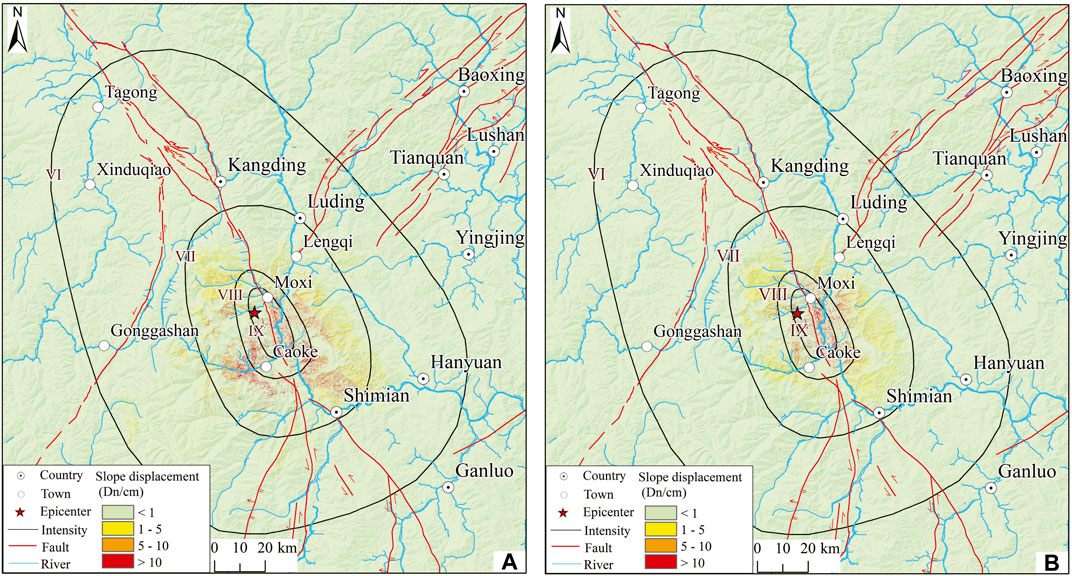
FIGURE 7. Slope cumulative displacement distribution of the Luding Ms6.8 earthquake (A) Slope cumulative displacement calculated based on PGA, and (B) Slope cumulative displacement calculated based on Arias.
The cumulative displacement of the slope calculated based on Ia indicates that the displacement area is limited to the VII intensity zone (Figure 7B). The slope displacement area is predominantly concentrated in the VIII intensity zone and distributed on the eastern side of the Moxi Fault Zone along both sides of the Dadu River. The farthest influence range extends up to Dewei Township in the north and Caoke Township in the south. It is noteworthy that the cumulative displacement of the slope can exceed 5 cm, in particular, the cumulative displacement of the Moxi Platform and the Wandong River Basin exceeds 10 cm, indicating that the potential risk of landslide and slope instability in the region should not be underestimated.
4.4 Earthquake-induced landslides risk assessment
Based on the Newmark model, this study uses PGA and Ia to calculate the landslide risk of the Luding Ms6.8 earthquake. The study area is divided into low-risk, medium-risk, high-risk, and extremely high-risk areas based on the probability of earthquake-induced landslides. The results indicate that the high-risk and extremely high-risk areas of earthquake-induced landslides are mainly distributed along both sides of the Dadu River and the Moxi fault. The prediction and evaluation results of earthquake-induced landslides risk are consistent with the landslide hazard predictions of the Luding Ms6.8 earthquake made by Chen et al. (2022) and Fan et al. (2022b).
The earthquake-induced landslides risk divisions calculated using PGA indicate that earthquake-induced landslides are extremely high, and the distribution of high-risk areas is relatively large, accounting for 1.81% of the total study area. They are mainly distributed along the Dadu River and on both sides of the Moxi fault, reaching to the north of the Jiajun Township, reaching south to the vicinity of Shimian County and the west side of Caoke Township (Figure 8A). The extremely high-risk zone area fluctuates and generally decreases as the distance from the fault increases, Within the slope range of 30°–45°, the extremely high-risk area occupies a larger area (Figure 9A).
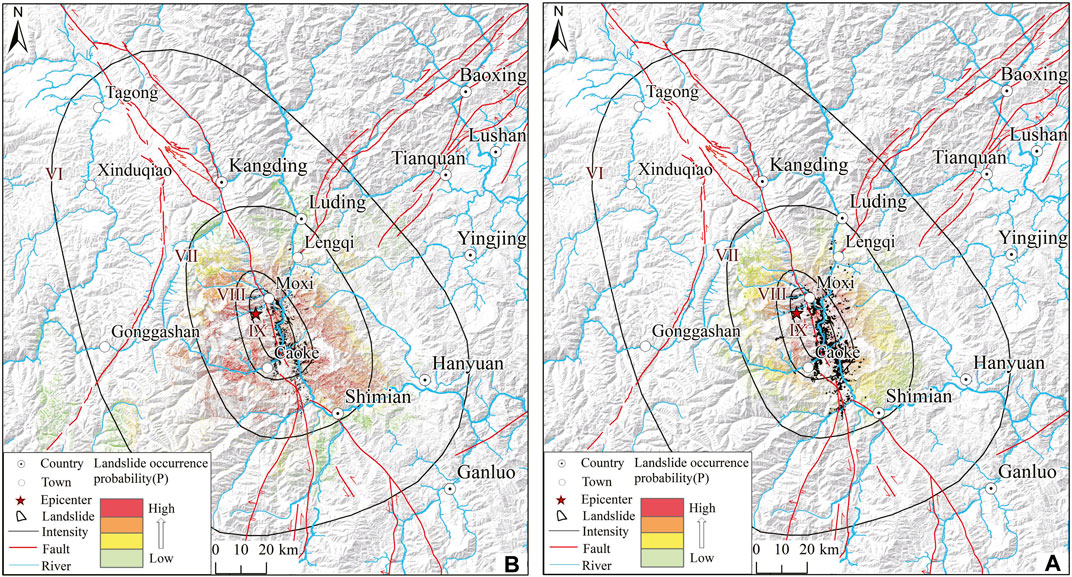
FIGURE 8. Landslide occurrence probability in the Luding Ms6.8 earthquake: (A) Distribution probability map of earthquake-induced landslides calculated based on PGA, and (B) Distribution probability map of earthquake-induced landslides calculated based on Ia.
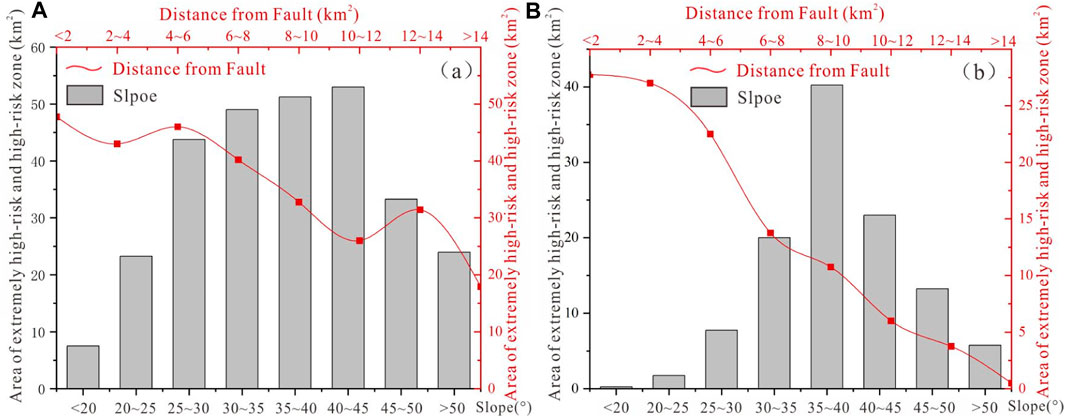
FIGURE 9. Statistical map of the distribution of extremely high-risk and high-risk areas of the Luding Ms6.8 Earthquake-induced landslides: (A) Statistical map of distribution characteristics of extremely high-risk and high-risk areas calculated based on PGA, and (B) Statistical map of distribution characteristics of extremely high-risk and high-risk areas calculated based on Ia.
The earthquake-induced landslides risk division calculated using Ia shows that the extremely high-risk and high-risk areas of earthquake-induced landslides are distributed over 0.71% of the total study area, mainly in intensity zone VII along the Dadu River and the Moxi fault, with the northernmost area being Dewei Township and the southern end extending to Caoke Township (Figure 8B). The extremely high-risk area of earthquake-induced landslides decreases as the distance from the fault increases, and the extremely high-risk area decreases within the slope range of 30°–45° (Figure 9B). The extremely high-risk area of earthquake-induced landslides is consistent with the distribution of earthquake-induced landslides interpreted by Huang et al. (2022).
5 Discussion
5.1 Discussion on the effect of PGA and Arias intensity on earthquake-induced landslides risk assessment
This paper aimed to assess the accuracy of landslide hazard assessment induced by the Luding Ms6.8 earthquake using PGA and Ia calculations based on the Newmark model. To verify the hazard assessment results, the paper used earthquake-induced landslides data shared by Huang et al. (2022). The success rate (ROC) curve method was used to test the earthquake-induced landslides risk assessment results. The closer the AUC value under the ROC curve is to 1.0, the higher the accuracy of the model. The study found that the area under the ROC curve of the earthquake-induced landslides risk assessment results obtained by PGA calculation was 0.73 (Figure 10), while the AUC value of the earthquake-induced landslides risk assessment results obtained by Ia calculation was 0.84. Therefore, the earthquake-induced landslides risk assessment method based on Ia calculation has high accuracy and reliability.
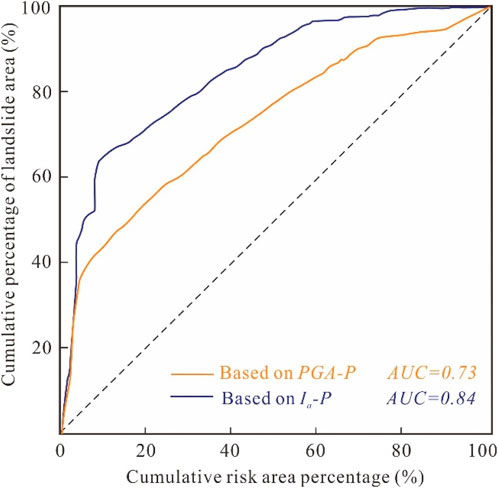
FIGURE 10. Success rate curve of earthquake-induced landslides risk assessment results induced by the Luding Ms6.8 earthquake.
Previous studies have also conducted risk assessments of earthquake-induced landslides using the Newmark cumulative displacement model and the Arias intensity based on the Newmark model. Wang et al. (2013) and Chousianitis et al. (2014) obtained accurate results that showed overall consistency between the hazard zones and the earthquake-induced landslides intensive areas revealed by post-earthquake surveys. However, Liu et al. (2022) conducted a rapid prediction assessment of Luding Ms6.8 earthquake-induced landslides based on the Newmark model using the peak ground acceleration obtained by estimation. The evaluation results showed that the risk of earthquake-induced landslides on both sides of the Dadu River was low and differed from the actual earthquake-induced landslides distribution.
The use of appropriate ground motion parameters is crucial for accurate earthquake-induced landslides risk assessment. In the Luding Ms6.8 earthquake, PGA and Ia were used to calculate earthquake-induced landslides risk assessment based on the Newmark model. It was found that the earthquake-induced landslides risk assessment method based on the Ia calculation has high accuracy and reliability. The reason for this is that Ia can reflect comprehensive information on earthquake amplitude and frequency at the same time and can more accurately reflect the real earthquake level compared to PGA. Therefore, it is suggested that when analyzing the relationship between regional earthquake-induced landslides and ground motion intensity, Arias intensity should be used rather than peak ground acceleration to avoid missing important ground motion information. Combining the established earthquake-induced landslides database, this study validates the results of earthquake-induced landslides risk assessments based on both PGA and Ia methods. The validation indicates that the earthquake-induced landslides risk assessment results calculated using Ia are more accurate. The model proves effective in predicting the hazard of earthquake-induced landslides and is applicable to earthquake-induced landslides risk assessments in tectonically active regions, such as the Tibetan Plateau. This is consistent with the findings of previous studies (Harp EL Wilson, 1995; Jibson, 2007; Jibson, 2011; Wang et al., 2013).
5.2 Discussion of the spatial characteristics of the earthquake-induced landslides
To analyze the spatial distribution of landslide risk zones induced by the Luding Ms6.8 earthquake based on Arias intensity calculations, several key characteristics were identified:
First, the findings suggest that earthquake-induced landslides tend to be concentrated along the fault zone, particularly in the NNW‒SSE Xianshuihe fault zone and the adjacent Dadu River region. As the distance from the fault increases, the area of extremely high-risk of earthquake-induced landslides decreases (Figure 9A). These observations can be attributed to the formation of structural landforms such as mountains and valleys due to faulting activities, which lead to relatively high seismic parameters in the fault zone area and the fracturing of the rock mass. These conditions increase the likelihood of landslide disasters. Therefore, it is important to take into account the proximity of a region to a fault zone and fractured rock mass when assessing landslide risks induced by earthquakes.
Second, the risk of landslides induced by the Luding earthquake exhibits a characteristic distribution along rivers and high and steep slopes. Slopes of 30°–45° are predominant in the extremely high-risk areas of earthquake-induced landslides. Specifically, the areas with high and steep slopes on both sides of the Dadu River, Wandong River and the Moxi Platform greater risks. High and steep slope areas experience unloading cracks due to the long-term effect of their own gravity, and some slopes undergo creep deformation. In the presence of earthquake action, these conditions contribute to an increased risk of landslides.
Huang et al. (2022) conducted a comprehensive study on the Luding Ms6.8 earthquake-induced landslides by establishing an earthquake-induced landslides database using field investigations and human-computer interaction visual interpretation of optical satellite images. The database includes at least 5007 earthquake-induced landslides, covering a total landslide area of 17.36 km2. This paper compared the results of earthquake-induced landslides risk assessment based on the earthquake-induced landslides database with those obtained using Ia calculations. The analysis shows that 2672 earthquake-induced landslides were distributed in extremely high-risk and high-risk areas of earthquake-induced landslides, accounting for 53.37% of the total number of landslides. Moreover, these landslides were distributed over an area of 13.34 km2, accounting for 76.95% of the total landslide area. The average area of earthquake-induced landslides decrease as the risk of earthquake-induced landslides decreases, as shown in Figure 11. The results of the risk prediction and evaluation are more consistent with the actual distribution of earthquake-induced landslides.
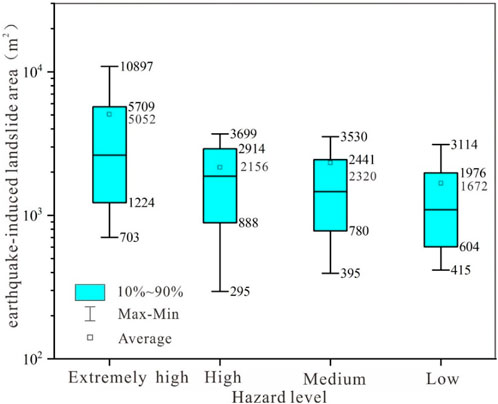
FIGURE 11. The area distribution of earthquake-induced landslides in the landslide hazard zone induced by the Luding Ms6.8 earthquake.
6 Conclusion
This study aimed to develop a rapid prediction and evaluation method for Luding Ms6.8 earthquake-induced landslides based on the PGA and Arias intensity.
First, the PGA and Arias intensity based on the Newmark cumulative displacement model were used to carry out rapid prediction and evaluation of earthquake-induced landslides. The results showed that the earthquake-induced landslides were extremely high, and high-risk areas were mainly distributed along the Dadu River, on both sides of the Moxi fault, to the north of Jiajun Township, to the south near Shimian County, and west of Caoke Township. The earthquake-induced landslides risk distribution obtained by the Ia calculation indicated that the earthquake-induced landslides were extremely high, and high-risk areas were mainly distributed in intensity zone VII, mainly along the Dadu River and Moxi fault distribution.
Second, the accuracy of the earthquake-induced landslides risk assessment results was verified using the earthquake-induced landslides database. The AUC value of the risk assessment results calculated by Ia was 0.84, which was more accurate than the risk assessment based on PGA and was more suitable for the rapid prediction and evaluation of earthquake-induced landslides.
Third, the regional risk distribution of Luding earthquake-induced landslides was highly influenced by active faults and had a tendency to concentrate along the fault zone, especially the NNW‒SSE to Xianshuihe fault zone. The areas with extremely high-risk and high-risk of earthquake induced landslides are mainly located on high and steep slopes affected by Dadu river incision and with relatively broken lithology, and in the dip range of 30–45°, the area occupied by the extremely high-risk zone was the largest. So, in the tectonically active regions, special attention should be directed towards areas controlled by faults, characterized by highly fragmented rock structures and steep slopes.
The research findings provide scientific guidance for subsequent remote sensing interpretation of earthquake-induced landslides and geological investigations, contributing to the establishment of a more comprehensive catalog of earthquake-induced landslides.
Data availability statement
The original contributions presented in the study are included in the article/Supplementary Material, further inquiries can be directed to the corresponding author.
Author contributions
CL: Writing–original draft. CG: Writing–review and editing. XZ: Conceptualization, Writing–review and editing. YY: Investigation, Writing–review and editing. JN: Investigation, Writing–review and editing. WZ: Investigation, Writing–review and editing.
Funding
The author(s) declare financial support was received for the research, authorship, and/or publication of this article. This research was supported by the National Natural Science Foundation of China (No. 42372339), the China Geological Survey Project (Nos DD20190319 and DD20221816), the Outstanding Young Scientists and Technologists Project of the Ministry of Natural Resources (No. 12110600000018003911).
Acknowledgments
The authors would like to thank Associate Prof. Zhihua Yang, Associate Prof. Ruian Wu from the Chinese Academy of Geological Sciences for their guidance on this paper, and Postgraduate students Deguang Song and Yanan Zhang for data processing. And thanks to Sichuan Provincial Institute of Geological Survey and Chengdu Zongheng Dapeng UAV Technology Co., Ltd. for providing field UAV remote sensing images for this research.
Conflict of interest
The authors declare that the research was conducted in the absence of any commercial or financial relationships that could be construed as a potential conflict of interest.
Publisher’s note
All claims expressed in this article are solely those of the authors and do not necessarily represent those of their affiliated organizations, or those of the publisher, the editors and the reviewers. Any product that may be evaluated in this article, or claim that may be made by its manufacturer, is not guaranteed or endorsed by the publisher.
References
An, Y., Wang, D., Ma, Q., Xu, Y. R., Li, Y., Zhang, Y. Y., et al. (2022). Preliminary report of the september 5, 2022 MS 6.8 luding earthquake, sichuan, China. Earthq. Res. Adv. 3, 100184. doi:10.1016/j.eqrea.2022.100184
Arias, A. (1970). “A measure of earthquake intensity,” in Seismic design for nuclear power plants (Cambridge, MA: Massachussetts Institute of Technology Press), 438–483.
Bai, M. K., Chevalier, M. L., Li, H. B., Pan, J. W., Wang, S. G., Li, K. Y., et al. (2021). Spatial slip rate distribution along the SE Xianshuihe Fault, eastern tibet, and earthquake hazard assessment. Tectonics 40, 1–14. doi:10.1029/2021tc006985
Bai, M. K., Chevalier, M. L., Li, H. B., Pan, J. W., Wu, Q., Wang, S. G., et al. (2022). Late Quaternary slip rate and earthquake hazard along the Qianning segment Xianshuihe fault. Acta Geol. Sin. 96 (7), 2312–2332. doi:10.19762/j.cnki.dizhixuebao.2022144
Bai, Y. J., Tie, Y. B., Ni, H. Y., and Li, M. H. (2014). Temporal spatial distribution and environment pregnant of geohazards in Xianshuihe of sichuan, China. J. Catastrophology 29 (4), 69–75. doi:10.3969/j.issn.1000-811X.2014.04.014
Chen, B., Li, Z. H., Huang, W. B., Liu, Z. J., Zhang, C. L., Du, J. T., et al. (2022a). Spatial distribution and influencing factors of Geohazards induced by the 2022 Mw6.6 Luding (Sichuan, China) earthquake. J. Sci. Environ. 44 (6), 971–985. doi:10.19814/j.jese.2022.10012
Chen, G. H., Xu, X. W., Wen, X. Z., and Chen, Y. G. (2016). Late Quaternary slip-rates and slip partitioning on the southeastern Xianshuihe fault system, eastern Tibetan Plateau. Acta Geol. Sinica-English Ed. 90 (2), 537–554. doi:10.1111/1755-6724.12689
Chen, S., Miao, Z. L., and Wu, L. X. (2022b). A method for using simplified Newmark displacement model based on modified strength parameters of rock mass. Acta Seismol. Sin. 44 (3), 512–527. doi:10.11939/jass.20210008
Chen, S., Miao, Z. L., Wu, L. X., and He, Y. G. (2020a). Application of an incomplete landslide inventory and one class classifier to earthquake induced landslide susceptibility mapping. IEEE J. Sel. Top. Appl. Earth Observations Remote Sens. 13, 1649–1660. doi:10.1109/jstars.2020.2985088
Chen, X. L., Liu, C. G., Yu, L., and Lin, C. X. (2014). Critical acceleration as a criterion in seismic landslide susceptibility assessment. Geomorphology 217, 15–22. doi:10.1016/j.geomorph.2014.04.011
Chen, X. L., Shan, X. J., Wang, M. M., Liu, C. G., and Han, N. N. (2020b). Distribution pattern of coseismic landslides triggered by the 2017 jiuzhaigou Ms7.0 earthquake of China: control of seismic landslide susceptibility. ISPRS Int. J. Geo-Information 9 (4), 198. doi:10.3390/ijgi9040198
Cheng, W. Z., and Yang, Y. L. (2002). Deformation rate changes of tectonic belts along boundaries of Yunnan-Sichuan block and their relation to grouped strong earthquakes. J. Geodesy Geodyn. 4, 21–25. doi:10.3969/j.issn.1671-5942.2002.04.005
Chousianitis, K., Gaudio, D., Kalogeras, I., and Ganas, A. (2014). Predictive model of Arias intensity and Newmark displacement for regional scale evaluation of earthquake induced landslide hazard in Greece. Soil Dyn. Earthq. Eng. 65, 11–29. doi:10.1016/j.soildyn.2014.05.009
Dai, D. Q., Sun, L., and Yang, Z. G. (2022). Rupture process of the 5 september 2022 Mw6.6 luding earthquake in sichuan. Seismic geomagnetic observation Res. 43 (05), 186–192.
Dreyfus, D., Rathje, E. M., and Jibson, R. W. (2013). The influence of different simplified sliding-block models and input parameters on regional predictions of seismic landslides triggered by the Northridge earthquake. Eng. Geol. 163, 41–54. doi:10.1016/j.enggeo.2013.05.015
Du, G. L., Zhang, Y. S., Yang, Z. H., Javed, I., Tong, B., Guo, C. B., et al. (2017). Estimation of seismic landslide hazard in the eastern himalayan syntaxis region of Tibetan plateau. Acta Geol. Sin. 91 (2), 658–668. doi:10.1111/1755-6724.13124
Fan, X. M., Fang, C. Y., Dai, L. X., Wang, X., Luo, Y. H., Wei, T., et al. (2022a). Near real time prediction of spatial distribution probability of earthquake-induced landslides take the Lushan Earthquake on June 1, 2022 as an example. J. Eng. Geol. 30 (3), 729–739. doi:10.13544/j.cnki.jeg.2022-0328
Fan, X. M., Scaringi, G., Xu, Q., Zhan, W. W., Dai, L. X., Li, Y. S., et al. (2018). Coseismic landslides triggered by the 8th August 2017 Ms7.0 Jiuzhaigou earthquake (Sichuan, China): factors controlling their spatial distribution and implications for the seismogenic blind fault identification. Landslides 15, 967–983. doi:10.1007/s10346-018-0960-x
Fan, X. M., Wang, X., Dai, L. X., Fang, C. Y., Deng, Y., Zou, C. B., et al. (2022b). Characteristics and spatial distribution pattern of Ms6.8 Luding earthquake occurred on September 5, 2022. J. Eng. Geol. 30 (5), 1504–1516. doi:10.13544/j.cnki.jeg.2022-0665
Froude, M. J., and Petley, D. N. (2018). Global fatal landslide occurrence from 2004 to 2016. Nat. Hazards Earth Syst. Sci. 18, 2161–2181. doi:10.5194/nhess-18-2161-2018
Gallen, S. F., Clark, M. K., Godt, J. W., Roback, K., and Niemi, N. A. (2016). Application and evaluation of a rapid response earthquake-triggered landslide model to the 25 April 2015 Mw 7.8 Gorkha earthquake, Nepal. Tectonophysics 714, 173–187. doi:10.1016/j.tecto.2016.10.031
Guo, C., Xu, Q., Dong, X. J., Li, W. L., Zhao, K. Y., Lu, H. Y., et al. (2021a). Geohazard recognition and inventory mapping using airborne LiDAR data in complex mountainous areas. J. Earth Sci. 32 (5), 1079–1091. doi:10.1007/s12583-021-1467-2
Guo, C. B., Du, Y. B., Zhang, Y. S., Zhang, G. Z., Yao, X., Wang, K., et al. (2015a). Geohazard effects of the Xianshuihe fault and characteristics of typical landslides in western Sichuan. Geol. Bull. China 34 (1), 121–134. doi:10.3969/j.issn.1671-2552.2015.01.010
Guo, C. B., Montgomery, D. R., Zhang, Y. S., Wang, K., and Yang, Z. H. (2015b). Quantitative assessment of landslide susceptibility along the Xianshuihe fault zone, Tibetan plateau, China. Geomorphology 248 (1), 93–110. doi:10.1016/j.geomorph.2015.07.012
Guo, X. Y., Fu, B. H., Du, J., Shi, P. L., Li, J. X., Li, Z., et al. (2021b). Monitoring and assessment for the susceptibility of landslide changes after the 2017 Ms7.0 Jiuzhaigou earthquake using the remote sensing technology. Front. Earth Sci. 9, 633117. doi:10.3389/feart.2021.633117
Gupta, K., and Satyam, N. (2022). Co-seismic landslide hazard assessment of Uttarakhand state (India) based on the modified Newmark model. J. Asian Earth Sci. X 8, 100120. doi:10.1016/j.jaesx.2022.100120
Han, B. Q., Liu, Z. J., Chen, B., Li, Z. H., Yu, C., Zhang, Y., et al. (2022). Coseismic deformation and slip distribution of the 2022 luding Mw 6.6 earthquake revealed by InSAR observations. Geomatics Inf. Sci. Wuhan Univ. 48 (1), 36–46. doi:10.13203/j.whugis20220636
Harp El Wilson, R. C. (1995). Shaking intensity thresholds for rock falls and slides: evidence from 1987 Whittier Narrows and superstition hills earthquake strong-motion records. Bull. Seismol. Soc. Am. 85 (6), 1739–1757. doi:10.1785/BSSA0850061739
Hua, J. X., and Zheng, J. G. (2018). Handbook of engineering Geology. Fifth Edition. Beijing: China Construction Industry Press.
Huang, R. Q., and Li, W. L. (2008). Research on development and distribution rules of geohazards induced by Wenchuan earthquake on 12 th May, 2008. Chin. J. Rock Mech. Eng. 27 (12), 2585–2592. doi:10.3321/j.issn:1000-6915.2008.12.028
Huang, Y. D., Xie, C. C., Li, T., Xu, C., He, X. L., Shao, X. Y., et al. (2022). An open-accessed inventory of landslides triggered by the MS 6.8 Luding earthquake, China on September 5, 2022. Earthq. Res. Adv. 3, 100181. doi:10.1016/j.eqrea.2022.100181
Iqbal, J., Dai, F. C., Hong, M., Tu, X. B., and Xie, Q. Z. (2018). Failure mechanism and stability analysis of an active landslide in the xiangjiaba reservoir area, southwest China. J. Earth Sci. 29 (3), 646–661. doi:10.1007/s12583-017-0753-5
Jafarian, Y., Lashgari, A., and Miraiei, M. (2021). Multivariate fragility functions for seismic landslide hazard assessment. J. Earthq. Eng. 25, 579–596. doi:10.1080/13632469.2018.1528909
Jibson, R. W. (1993). Predicting earthquake induced landslide displacements using Newmark's sliding block analysis. Transp. Res. Rec. 1411, 9–17.
Jibson, R. W. (2007). Regression models for estimating coseismic landslide displacement. Eng. Geol. 91 (2-3), 209–218. doi:10.1016/j.enggeo.2007.01.013
Jibson, R. W. (2011). Methods for assessing the stability of slopes during earthquakes: a retrospective. Eng. Geol. 122 (1-2), 43–50. doi:10.1016/j.enggeo.2010.09.017
Jibson, R. W., Harp, E. L., and Michael, J. A. (2000). A method for producing digital probabilistic seismic landslide hazard maps. Eng. Geol. 58 (3-4), 271–289. doi:10.1016/s0013-7952(00)00039-9
Jin, K. P., Yao, L. K., Cheng, Q. G., and Xing, A. G. (2019). Seismic landslides hazard zoning based on the modified Newmark model: a case study from the lushan earthquake, China. Nat. Hazards 99 (1), 493–509. doi:10.1007/s11069-019-03754-6
Li, G. H., Wang, A. J., and Gao, Y. (2022). Source rupture characteristics of the September 5, 2022 Luding M 6.8 earthquake at the Xianshuihe fault zone in southwest China. Earthq. Res. Adv. 3, 100201. doi:10.1016/j.eqrea.2022.100201
Li, T. Y., and Du, Q. F. (1997). Xianshuihe active fault zone and strong earthquake risk assessment. Chengdu: Chengdu Map Publishing House.
Liu, J. M., Shi, J. S., Wang, T., and Wu, S. (2018). Seismic landslide hazard assessment in the Tianshui area, China, based on scenario earthquakes. Bull. Eng. Geol. Environ. 77, 1263–1272. doi:10.1007/s10064-016-0998-8
Liu, J. M., Wang, T., Du, J. J., Chen, K., Huang, J. H., Wang, H. J., et al. (2022). Emergency rapid assessment of landslides induced by the Luding Ms6.8 earthquake, Sichuan, China. Hydrogeology Eng. Geol. 50, 1–12. doi:10.16030/j.cnki.issn.1000-3665.202209057
Maharjan, S., Gnyawali, K. R., Tannant, D. D., Chong, X., and Lacroix, P. (2021). Rapid terrain assessment for earthquake-triggered landslide susceptibility with high-resolution DEM and critical acceleration. Front. Earth Sci. 9, 689303. doi:10.3389/feart.2021.689303
Miles, S. B., and Ho, C. L. (1999). Rigorous landslide hazard zonation using Newmark's method and stochastic ground motion simulation. Soil Dyn. Earthq. Eng. 18, 305–323. doi:10.1016/s0267-7261(98)00048-7
Newmark, N. M. (1965). Effects of earthquakes on dams and embankments. Géotechnique 15 (2), 139–160. doi:10.1680/geot.1965.15.2.139
Nowicki, M. A., Wald, D. J., Hamburger, M. W., Hearne, M., and Thompson, E. M. (2014). Development of a globally applicable model for near real time prediction of seismically induced landslides. Eng. Geol. 173, 54–65. doi:10.1016/j.enggeo.2014.02.002
Nowicki Jessee, M. A., Hamburger, M. W., Allstadt, K., Wald, D. J., Robeson, S. M., Tanyas, H., et al. (2018). A global empirical model for near-real-time assessment of seismically induced landslides. J. Geophys. Res. Earth Surf. 123 (8), 1835–1859. doi:10.1029/2017jf004494
Owen, L. A., Kamp, U., Khattak, G. A., Harp, E. L., Keefer, D. K., and Bauer, M. A. (2008). Landslides triggered by the 8 october 2005 Kashmir earthquake. Geomorphology 94, 1–9. doi:10.1016/j.geomorph.2007.04.007
Pan, J. W., Li, H. B., Chevalier, M. L., Bai, M. K., Liu, F. C., Liu, D. L., et al. (2020). A newly discovered active fault on the Selaha Kangding segment along the SE Xianshuihe fault: the South Mugecuo fault. Acta Geol. Sin. 94 (11), 3178–3188. doi:10.19762/j.cnki.dizhixuebao.2020196
Pareek, N., Pal, S., Kaynia, A. M., and Sharma, M. L. (2014). Empirical-based seismically induced slope displacements in a geographic information system environment: a case study. Geohazards 8 (4), 258–268. doi:10.1080/17499518.2014.980273
Qian, H. (1988). Geological determination of potential source area on Xianshuihe fault zone. Sichuan Earthq. 2, 20–28.
Qu, Z., Zhu, B. J., Cao, Y. T., and Ru, H. R. (2022). Rapid report of seismic damage to buildings in the 2022 M 6.8 Luding earthquake, China. Earthq. Res. Adv. 3, 100180. doi:10.1016/j.eqrea.2022.100180
Rathje, E. M., and Sayg, G. (2008). Probabilistic seismic hazard analysis for the sliding displacement of slopes: scalar and vector approaches. J. Geotechnical Geoenvironmental Eng. 134 (6), 804–814. doi:10.1061/(asce)1090-0241(2008)134:6(804)
Roback, K., Clark, M. K., West, A. J., Zekkos, D., Li, G., Gallen, S. F., et al. (2018). The size, distribution, and mobility of landslides caused by the 2015 Mw7.8 Gorkha earthquake. Nepal. Geomorphol 301, 121–138. doi:10.1016/j.geomorph.2017.01.030
Roger, F., Calassou, S., Lancelot, J., Malavieille, J., Hou, L., Zhiqin, X., et al. (1995). Miocene emplacement and deformation of the Konga Shan granite (Xianshuihe fault zone, west Sichuan, China): geodynamic implications. Earth Planet. Sci. Lett. 130 (1-4), 201–216. doi:10.1016/0012-821x(94)00252-t
Shafique, M., Mark, V., and Khan, M. A. (2016). A review of the 2005 Kashmir earthquake induced landslides; from a remote sensing prospective. J. Asian Earth Sci. 118, 68–80. doi:10.1016/j.jseaes.2016.01.002
Shen, T., and Wang, Y. S. (2016). Development pattern of collapses in Wenchuan earthquake epicentral area. Sci. Technol. Eng. 16 (9), 7–14. doi:10.3969/j.issn.1671-1815.2016.09.002
Song, D. Q., Chen, Z., Ke, Y. T., and Nie, W. (2020a). Seismic response analysis of a bedding rock slope based on the time-frequency joint analysis method: a case study from the middle reach of the Jinsha River, China. Eng. Geol. 274, 105731. doi:10.1016/j.enggeo.2020.105731
Song, D. Q., Liu, X. L., Huang, J., and Zhang, J. M. (2020b). Energy-based analysis of seismic failure mechanism of a rock slope with discontinuities using Hilbert-Huang transform and marginal spectrum in the time-frequency domain. Landslides 18, 105–123. doi:10.1007/s10346-020-01491-7
Song, D. Q., Liu, X. L., Huang, J., Zhang, Y. F., Zhang, J. M., and Nkwenti, N. B. (2021). Seismic cumulative failure effects on a reservoir bank slope with a complex geological structure considering plastic deformation characteristics using shaking table tests. Eng. Geol. 286, 106085. doi:10.1016/j.enggeo.2021.106085
Sun, D., Yang, T., Cao, N., Tang, L., Hu, X., Wei, M., et al. (2023). Characteristics and prevention of coseismic geohazard induced by luding Ms6.8 earthquake, sichuan, China. Geosci. Front. 49, 1–18. doi:10.13745/j.esf.sf.2022.12.50
Tang, Y., Che, A., Cao, Y., and Zhang, F. (2020). Risk assessment of seismic landslides based on analysis of historical earthquake disaster characteristics. Bull. Eng. Geol. Environ. 79, 2271–2284. doi:10.1007/s10064-019-01716-7
Tie, Y. B., Zhang, X. Z., Lu, J. Y., Liang, J. T., Wang, D. H., Ma, Z. G., et al. (2020). Characteristics of geological hazards and it’s mitigations of the Ms6.8 earthquake in luding county, sichuan Province. Hydrogeology Eng. Geol. 49 (6), 1–12. doi:10.16030/j.cnki.issn.1000-3665.202209023
Wang, K. L., and Lin, M. L. (2010). Development of shallow seismic landslide potential map based on newmark's displacement: the case study of chi-chi earthquake, taiwan. Environ. Earth Sci. 60 (4), 775–785. doi:10.1007/s12665-009-0215-1
Wang, T., Wu, S. R., Shi, J. S., and Xin, P. (2013). Application and validation of seismic landslide displacement analysis based on Newmark model: a case study in wenchuan earthquake. Acta Geol. Sin. Engl. Ed. 87, 393–397.
Wang, T., Wu, S. R., Shi, J. S., and Xin, P. (2015). Concepts and mechanical assessment method for hazard: a review. J. Eng. Geol. 23 (1), 93–104. doi:10.13544/j.cnki.jeg.2015.01.014
Wang, X., Fang, C. Y., Tang, X. C., Dai, L. X., Fan, X. M., and Xu, Q. (2022). Research on emergency evaluation of landslides induced by luding Ms6.8 earthquake. Wuhan: Geomatics and Information Science of Wuhan University, 1–15.
Wilson, R. C., and Keefer, D. K. (1983). Dynamic analysis of a slope failure from the 6 August 1979 Coyote Lake, California, earthquake. Bull. Seismol. Soc. Am. 73 (3), 863–877. doi:10.1785/bssa0730030863
Wilson, R. C., and Keefer, D. K. (1985). Predicting areal limits of earthquake induced landsliding. Geol. Surv. Prof. Pap. 1360, 317–345.
Xiao, Z., Xu, C., Huang, Y., He, X., Shao, X., Chen, Z., et al. (2023). Analysis of spatial distribution of landslides triggered by the Ms6.8 Luding earthquake in China on September 5, 2022. Geoenvironmental Disasters 10 (1), 3–15. doi:10.1186/s40677-023-00233-w
Xie, F., Liang, C., Dai, S. G., Shao, B., Huang, H. B., Ouyang, J. H., et al. (2022). Preliminary results on a near-real-time rock slope damage monitoring system based on relative velocity changes following the September 5, 2022 MS 6.8 Luding, China earthquake. Earthq. Res. Adv. 3, 100202. doi:10.1016/j.eqrea.2022.100202
Xiong, T. Y., Yao, X., and Zhang, Y. S. (2010). A review on study of activity of Xianshuihe fault zone since the Holocene. J. Geomechanics 16 (02), 176–188. doi:10.3969/j.issn.1006-6616.2010.02.007
Xu, C., Xu, X., and Shyu, J. B. H. (2015). Database and spatial distribution of landslides triggered by the Lushan, China Mw 6.6 earthquake of 20 April 2013. Geomorphology 248, 77–92. doi:10.1016/j.geomorph.2015.07.002
Xu, C., Xu, X. W., Dai, F. C., Wu, Z. D., He, H. L., Shi, F., et al. (2013). Application of an incomplete landslide inventory, logistic regression model and its validation for landslide susceptibility mapping related to the May 12, 2008 Wenchuan earthquake of China. Nat. hazards 68 (2), 883–900. doi:10.1007/s11069-013-0661-7
Xu, C., Xu, X. W., Yao, X., and Dai, F. C. (2014). Three (nearly) complete inventories of landslides triggered by the May 12, 2008 Wenchuan Mw7.9 earthquake of China and their spatial distribution statistical analysis. Landslides 11, 441–461. doi:10.1007/s10346-013-0404-6
Xu, G. X., Yao, L. K., Li, Z. H., and Wang, X. F. (2012). Predictive models for permanent displacement of slopes based on recorded strong-motion data of Wenchuan Earthquake. J. Geotechnical Eng. 34 (6), 1131–1136.
Xu, X. W., Wen, X. Z., Zheng, R. Z., Ma, W. T., Song, F. M., and Yu, G. H. (2003). Pattern of latest tectonic motion and its dynamics for active blocks in Sichuan-Yunnan region, China. Sci. China Ser. D Earth Sci. 33 (S1), 151–162. doi:10.3321/j.issn:1006-9267.2003.z1.017
Xu, Z. Q., Li, H. Q., Hou, L. W., Fu, X. F., Chen, W., Zeng, L. S., et al. (2007). Uplift of the Longmen- Jinping orogenic belt along the eastern margin of the Qinghai-Tibet Plateau: large-scale detachment faulting and extrusion mechanism. Geol. Bull. China 26 (10), 1262–1276. doi:10.3969/j.issn.1671-2552.2007.10.005
Yang, Z. G., Dai, D. Q., Zhang, Y., Zhang, X. M., and Liu, J. (2022). Rupture process and aftershock mechanisms of the 2022 Luding Ms6.8 earthquake in Sichuan, China. Earthq. Sci. 35, 1–2. doi:10.1016/j.eqs.2022.12.005
Yang, Z. H., Lan, H. X., Gao, X., Li, L. P., Meng, Y. S., and Wu, Y. M. (2015). Urgent landslide susceptibility assessment in the 2013 lushan earthquake-impacted area, sichuan Province, China. Nat. Hazards 75 (3), 2467–2487. doi:10.1007/s11069-014-1441-8
Yang, Z. H., Lan, H. X., Zhang, Y. S., and Guo, C. B. (2017). Research review on long term activity of post earthquake geohazard in strong seismic disturbed regions. J. Geomechanics 23 (5), 743 753. doi:10.3969/j.issn.1006-6616.2017.05.011
Yin, Y. P. (2008). Studies on the geo-hazards triggered by wenchuan earthquake, sichuan. J. Eng. Geol. 16 (4), 433–444.
Yuan, R., Deng, Q., Cunningham, D., Han, Z., Zhang, D., and Zhang, B. (2016). Newmark displacement model for landslides induced by the 2013 Ms7.0 lushan earthquake, China. Front. Earth Sci. 10 (4), 740–750. doi:10.1007/s11707-015-0547-y
Zang, M., Qi, S. W., Zou, Y., Sheng, Z. P., and Zamora, B. S. (2020). An improved method of Newmark analysis for mapping hazards of earthquake-induced landslides. Nat. Hazards Earth Syst. Sci. Discuss. 20, 1–44. doi:10.5194/nhess-20-713-2020
Zhang, D., Wu, Z. H., Li, J. C., and Jiang, Y. (2013). An overview on earthquake induced landslide research. J. Geomechanics 19 (03), 225–241. doi:10.3969/j.issn.1006-6616.2013.03.001
Zhang, Y. S., Yang, Z. H., Guo, C. B., Wang, T., Wang, D. H., and Du, G. L. (2017). Predicting landslide scenes under potential earthquake scenarios in the Xianshuihe fault zone, Southwest China. J. Mt. Sci. 14 (07), 1262–1278. doi:10.1007/s11629-017-4363-6
Zhao, H. J., Ma, F. S., Li, Z. Q., Guo, J., and Zhang, J. X. (2022). Optimization of parameters and application of probabilistic seismic landslide hazard analysis model based on newmark displacement model: a case study in Ludian earthquake area. Earth Sci. 47 (12), 4401–4416. doi:10.3799/dqkx.2022.289
Keywords: Luding Ms6.8 earthquake, earthquake-induced landslides, earthquake-induced landslides risk assessment, Arias intensity, Newmark model
Citation: Li C, Guo C, Zhang X, Yan Y, Ni J and Zhao W (2023) Rapid evaluation of earthquake-induced landslides by PGA and Arias intensity model: insights from the Luding Ms6.8 earthquake, Tibetan Plateau. Front. Earth Sci. 11:1324773. doi: 10.3389/feart.2023.1324773
Received: 20 October 2023; Accepted: 27 November 2023;
Published: 29 December 2023.
Edited by:
Chong Xu, Ministry of Emergency Management, ChinaReviewed by:
Amod Mani Dixit, Universal Engineering and Science College (UESC), NepalDanqing Song, South China University of Technology, China
Yuandong Huang, Ministry of Emergency Management, China
Copyright © 2023 Li, Guo, Zhang, Yan, Ni and Zhao. This is an open-access article distributed under the terms of the Creative Commons Attribution License (CC BY). The use, distribution or reproduction in other forums is permitted, provided the original author(s) and the copyright owner(s) are credited and that the original publication in this journal is cited, in accordance with accepted academic practice. No use, distribution or reproduction is permitted which does not comply with these terms.
*Correspondence: Changbao Guo, Z3VvY2hhbmdiYW9AY2Fncy5hYy5jbg==