- 1MARUM—Center for Marine Environmental Sciences, University of Bremen, Bremen, Germany
- 2Climate Lab, Institute of Geography, University of Bremen, Bremen, Germany
- 3National Drought Monitoring Centre, Pakistan Meteorological Department, Islamabad, Pakistan
This study investigates the potential impacts of future climate change on crop water requirements (CWR) in different climatic zones of Pakistan and the subsequent implications for agricultural water demand and supply. Using the latest CMIP6 climate projections, we focused on Rabi and Kharif crop seasons, which are crucial for the growth of major crops in Pakistan. An empirical climate-crop and a hydrological model based on the Budyko theory were modified and forced to project future CWR changes and the potential widening of the water demand-supply gap until 2,100. Our results indicate a significant rise in mean annual CWR across all zones and emissions scenarios, with increasing rates at 2.30–2.57 mm/yr under SSP585 and 1.0–1.26 mm/yr under SSP245. Both Rabi and Kharif seasons show rising CWR, notably more under SSP585 (Kharif: 8%–14%, Rabi: 12%–15%) than SSP245 (Kharif: 4%–7%, Rabi: 6%–8%). The demand-supply gap is expected to grow notably, with arid and semi-arid zones being the most affected. Compared to 2015–2025, by 2091–2,100, the gap increased by 7%–15% (SSP245) and 15%–28% (SSP585) for Kharif and 7%–13% (SSP245) and 13%–32% (SSP585) for Rabi. To address these challenges, we recommend strategies like enhancing irrigation efficiency, adjusting crop patterns, and developing heat-resilient crops. Our insights aim to inform policy decisions on agriculture and water management in Pakistan under future climate change.
1 Introduction
Climate change poses severe global challenges with substantial implications for agricultural systems. It threatens food security, rural livelihoods, and economic stability, thereby necessitating urgent and comprehensive responses (IPCC, 2021). The risks are particularly severe in regions where agriculture is heavily dependent on climate-dependent water availability, such as Pakistan (Elliott et al., 2014; Schewe et al., 2014; Immerzeel et al., 2020; Dars et al., 2021). In such contexts, understanding the potential impacts of future climate change on crop water requirements (CWR) and the changes in agricultural water demand and supply is critical.
Agriculture forms the backbone of Pakistan’s economy, with a significant proportion of the population engaged in farming and related activities. The sector is pivotal in national food security (FAO, 2018) and contributes substantially to the country’s economy. However, climate change, manifested through changes in temperature and precipitation patterns, increasingly threatens the sustainability of Pakistan’s agriculture (Munir et al., 2021), and has profound implications for water resources and agricultural productivity (Khan et al., 2021a).
Water, a critical input in agriculture, is predominantly determined by climate. Changes in climatic conditions can significantly alter the water requirements of crops, known as CWR. Rising temperatures due to climate change are known to increase evapotranspiration, subsequently increasing CWR (Liu et al., 2018). Shifts in precipitation patterns can also impact the timing and amount of water available for agriculture (Heureux et al., 2022), thereby affecting the balance of agricultural water demand and supply (Shafeeque et al., 2023).
Recent studies have employed climate-crop modeling approaches to project changes in CWR under future climate scenarios (Elliott et al., 2014; Chen et al., 2018; Liu et al., 2018; Elbeltagi et al., 2022). The dual crop coefficient approach, as described by the Food and Agriculture Organization (FAO) in their Irrigation and Drainage Paper No. 56, is a methodology used to calculate CWR (Allen et al., 2005). The dual crop coefficient splits the crop coefficient (Kc) into two components: basal crop coefficient (Kcb) and soil evaporation coefficient (Ks). The Ks factor is meant to adjust crop water use for the effects of water stress, which reduces transpiration and growth. Multiple studies have evaluated and improved the FAO-56 approach for calculating Ks. Yi (2010) found that the FAO approach underestimated soil water depletion for winter wheat but modifying the approach to account for crop evapotranspiration improved accuracy. Similarly, Hu et al. (2020) found that the FAO approach had reduced accuracy for alfalfa under water and salt stress, but incorporating a dynamic yield response factor improved simulations. Improving the climate-crop model by introducing a soil moisture adjustment factor (S) would enhance the accuracy of the calculated CWR. The S factor encompasses a broader spectrum of soil moisture dynamics, potentially accounting for not just water stress but also other soil-plant-atmosphere interactions that can impact CWR. Moreover, the simplicity of the empirical approach would make it transferrable to any geographical and climatic region.
Complementing these CWR projections based on climate-crop modeling with hydrological models to evaluate the agricultural water demand-supply balance can provide valuable insights (Schewe et al., 2014; Xavier et al., 2020). However, research gaps remain in investigating these dynamics in climate-sensitive agrarian societies like Pakistan. Much of the existing literature has focused on temperate regions and utilized earlier-generation climate models (Bhima, 2018; Chen et al., 2018). Studies incorporating the latest CMIP6 projections (Eyring et al., 2016) across Pakistan’s diverse climatic zones are limited. Moreover, research concurrently examining climate change impacts on CWR and agricultural water demand-supply at regional scales is lacking.
Few studies have incorporated CWR assessments with hydrological models to investigate potential changes in agricultural water demand and supply (Schewe et al., 2014; Ismail et al., 2020; Xing et al., 2020). Such integration is essential for understanding the interplay between future climate change, CWR, and agricultural water demand and supply (Zhang et al., 2021). This understanding, in turn, is critical for developing effective adaptation strategies in the agriculture sector.
This study addresses these gaps by (1) proposing an advanced empirical climate-crop model and employing it to estimate future CWR in Pakistan under CMIP6 projections, (2) proposing a modified version of hydrological model grounded in Budyko theory (Budyko, 1974) and using it to assess changes in agricultural water demand-supply, (3) Focusing the analysis on Pakistan’s four distinct climatic zones (extreme-arid, arid, semi-arid, and humid) and major cropping seasons (Rabi and Kharif). The latest CMIP6 climate projections under SSP245 and SSP585 scenarios were used to force the empirical climate-crop and Budyko hydrological models until 2,100. The study hypothesized that climate change would significantly impact CWR and further disrupt the agricultural water demand-supply gap across climatic zones and cropping seasons, with more pronounced effects under higher emissions scenarios and during high demand cropping season.
The study’s objectives are: (1) To estimate future changes in CWR under the SSP245 and SSP585 scenarios using an advanced empirical climate-crop model. (2) To evaluate potential changes in agricultural water demand and supply under different future climate conditions using the modified hydrological model based on the Budyko theory. (3) To identify the most vulnerable climatic zones in Pakistan and suggest adaptive agricultural water management strategies under future climate change scenarios. The findings are intended to provide robust scientific evidence to guide urgent climate adaptation efforts for securing Pakistan’s agricultural sustainability amidst intensifying climate risks and water constraints.
2 Materials and methods
2.1 Study area description
The study area of this research comprises various districts across all provinces of Pakistan, which represent four distinct climatic zones: extreme-arid, arid, semi-arid, and humid (Figure 1A; Table 1). Pakistan’s geographic location, marked by diverse topography and regional climatic influences, has led to distinct climatic characteristics across the country (Afzal, 2018; Nusrat et al., 2020). Given this heterogeneity, categorizing the country into these four climatic zones provides a comprehensive yet nuanced approach to examining the impacts of future climate change on crop water requirements and agricultural water supply.
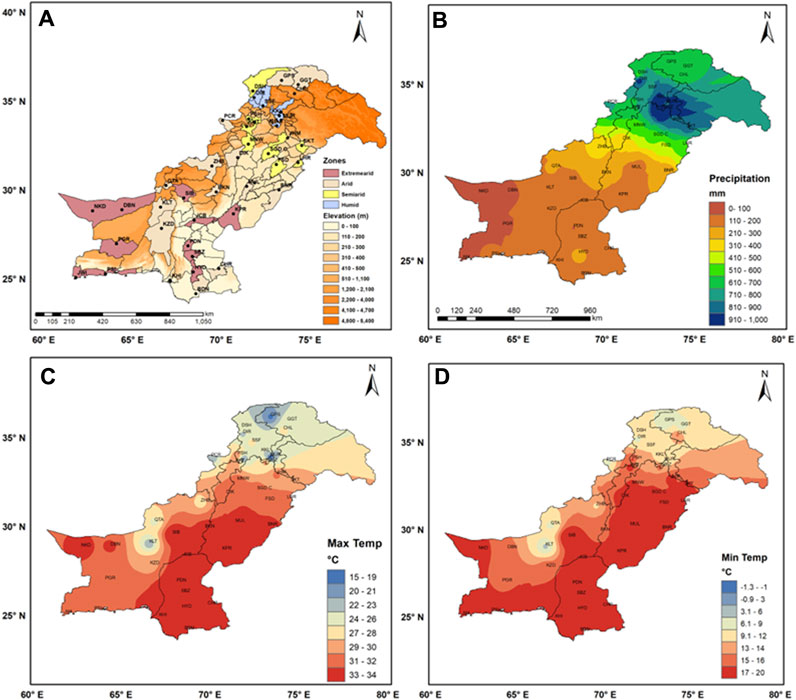
FIGURE 1. Overview of study area and climatology. (A) Geographic map indicating the study area’s elevation, meteorological stations, and representative districts for four distinct climatic zones. (B) Mean annual precipitation from 1951 to 2014. (C) Mean annual maximum temperature from 1951 to 2014. (D) Mean annual minimum temperature from 1951 to 2014. The full forms of the station names are provided in Table 1.
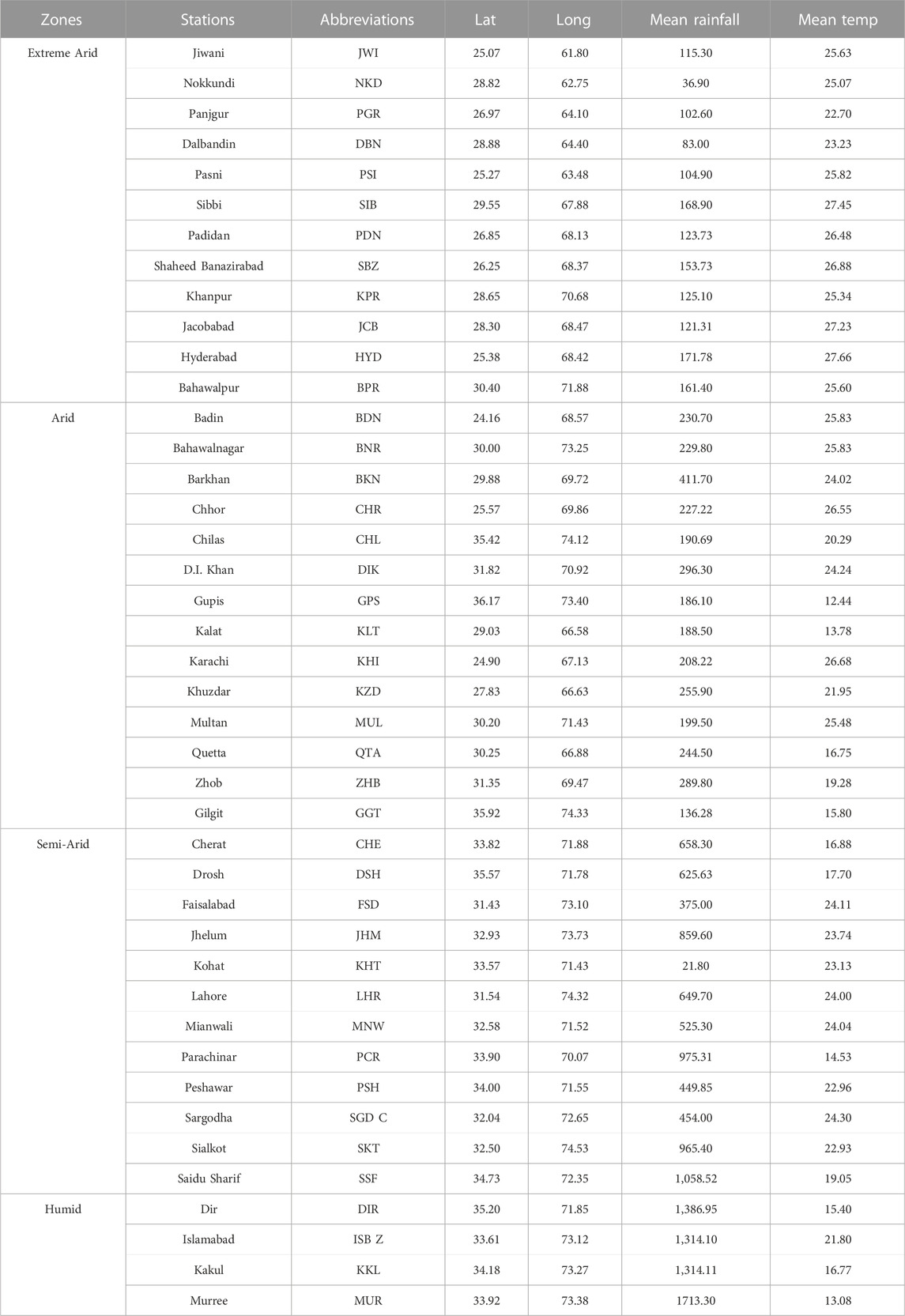
TABLE 1. Details of meteorological stations, their distribution in four climatic zones in Pakistan, and mean precipitation and temperature over (1951–2014). Abbreviations are the same as presented in Figure 1.
The Extreme Arid and Arid zones, located mostly in parts of Punjab, the eastern part of Sindh and Balochistan, are characterized by high temperatures ranging from 30°C to 35°C and scarce rainfall (Figures 1B, C). These conditions pose significant challenges to agriculture, primarily due to limited water availability (Haider and Ullah, 2020). In contrast, the semi-arid zone, spanning mostly parts of Khyber Pakhtunkhwa and Punjab, experiences moderate rainfall and temperatures, supporting a mix of farming systems. Lastly, the humid zone, mainly in Azad Kashmir and Gilgit Baltistan, receives ample rainfall of more than 800 mm (Figure 1B) and has a cooler climate, supporting a wide range of crops (Haider et al., 2017; Hasan and Khan, 2019). This classification is supported by previous studies that have recognized similar climatic divisions in Pakistan (Adnan et al., 2017; Hasan and Khan, 2019; Javid et al., 2019). Moreover, these climatic zones align with the agro-climatic zoning by the Pakistan Agricultural Research Council.
Using these climatic zones in our study allows for a more detailed and region-specific analysis of the impacts of future climate scenarios. This approach is advantageous over broader classifications, as it acknowledges the inherent climatic diversity across Pakistan, which significantly influences agricultural practices and water requirements. By considering these distinct zones, our study offers more precise and relevant insights for each region, thereby enhancing the utility of our findings for policy and planning purposes.
2.2 Data acquisition and preprocessing
The data utilized in the present study consisted of daily observations of maximum and minimum temperatures as well as precipitation. These data were obtained from the Pakistan Meteorological Department (PMD) for 42 meteorological stations across the country for the baseline period spanning 1951–2014 (http://www.pmd.gov.pk/cdpc/home.htm). The selection criteria for the meteorological stations were adapted from Adnan and Haider (2014) to ensure adequate geographic distribution and representation of Pakistan’s diverse climatic zones, including extremely-arid, arid, semi-arid, and humid zones.
Future climate data were derived from 10 general circulation models (GCMs) contained within the Coupled Model Intercomparison Project Phase 6 (CMIP6) dataset (https://esgf-node.llnl.gov/projects/cmip6/) (Table 2), with a specific focus on the Shared Socioeconomic Pathways SSP245 and SSP585 (Eyring et al., 2016). The CMhyd software (https://swat.tamu.edu/software/cmhyd/) was utilized to downscale and remove biases from the GCM data, adjusting it to align with the observed meteorological records before incorporation into empirical models. Quantile mapping represented the technique employed for empirical bias correction. Based on the evaluation of parameters from various quantile mapping strategies, the approach outlined by Gudmundsson et al. (2012) was selected due to exhibiting the fewest biases. The downscaled data were applied for both the baseline period (1951–2014) and the projected timeframe of 2015–2,100.
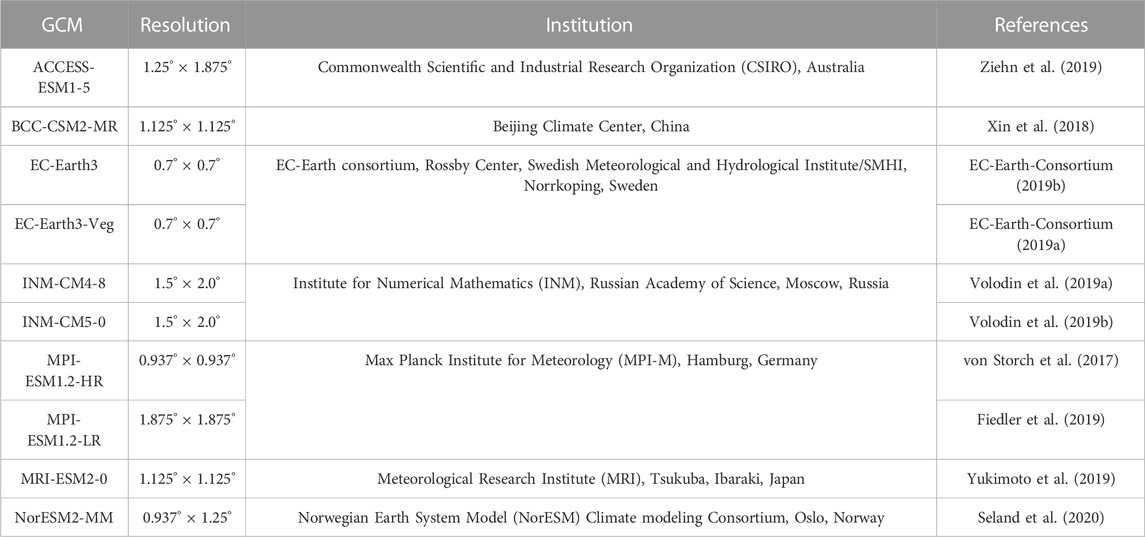
TABLE 2. Details of the selected GCMs from CMIP6 used in the study. The data was extracted for historical (1951–2014) and SSP245 and SSP585 (2015–2,100).
In addition to the meteorological parameters, data for the crop coefficient (Kc) and Budyko parameter (ω) were also included in the analysis. Specifically, the annual cycle of the crop coefficient (Kc) encompassing the typical duration from emergence to maturity for seasonal crops was obtained from previous studies by Rasul and Farooqi (1993); Naheed and Mahmood (2009); Naheed and Rasul (2010b). Concurrently, data for the Budyko parameter (ω), representing basin properties and climatic conditions, were referenced from research by Xu et al. (2013). These ω values indicate the irrigation effects on water and vegetation during this period. It was adjusted through calibration against observed precipitation-based supplies in each zone.
2.3 Improving empirical climate-crop model for calculating crop water requirements
The empirical climate-crop model was developed in this study to estimate the CWR for major crops grown in Pakistan’s varied climatic zones during the Rabi and Kharif cropping seasons. This empirical model integrates climate data, crop-specific information, and soil moisture factors to determine crop water demands systematically. The model can be expressed mathematically as:
Where, CWR = Crop water requirement (mm/day)
Kc = Crop coefficient (dimensionless). The Kc alues used in the model ranged from 0.45 in Rabi to 1.25 in Kharif.
ETp = Potential evapotranspiration (mm/day), calculated using the Hargreaves equation
S = Soil moisture adjustment factor (0–1)
The climate-crop model, incorporating the S factor, presents an advanced methodology for estimating CWR. In connection with existing methodologies, it is noteworthy to differentiate the S factor introduced in our empirical climate-crop model from the Ks factor in the dual crop coefficient approach (Allen et al., 2005). While both factors aim to capture the influence of soil moisture on CWR, their roles are distinct. The Ks factor in the dual crop coefficient primarily adjusts for water stress based on root zone soil water depletion. Conversely, our S factor encompasses a broader spectrum of soil moisture dynamics, potentially accounting for not just water stress but also other soil-plant-atmosphere interactions that can impact CWR. This advanced integration of the S factor directly into the CWR calculation allows us to refine CWR predictions based on real-time soil moisture conditions. Our model offers a more holistic view of the plant-soil-climate continuum by emphasizing both the climatic variables (through ETp and Kc) and soil moisture dynamics (through S). Including S in the climate-crop model also ensures our model’s adaptability to various soil types, moisture conditions, and irrigation practices, making it relevant and accurate across diverse agricultural scenarios.
The Hargreaves method (Hargreaves and Samani, 1985) was utilized to estimate ETp based on temperature data. This method provides a relatively simple approach for estimating ETp in areas with limited climatic records, requiring only maximum, minimum, and mean temperature data (Droogers and Allen, 2002). The Hargreaves equation is:
Where, Ra = Extraterrestrial radiation (mm/day)
T = Mean temperature (°C)
Td = Difference between mean maximum and mean minimum temperature (°C)
The extraterrestrial radiation (Ra) was computed based on the latitude and day of the year using standardized equations (Allen et al., 1998).
By integrating these key parameters pertaining to climatic conditions, crop characteristics, and soil moisture availability, the empirical climate-crop model provides a systematic methodology for determining CWR across Pakistan’s diverse agro-climatic zones during the cropping seasons.
2.4 Modifying hydrological model for calculating supply and demand gap
A hydrological model grounded in the Budyko framework (Budyko, 1974) was modified to estimate the demand-supply gap between CWR and precipitation-based supplies across Pakistan’s diverse climatic zones from 2015 to 2,100.
Budyko’s theory partitions precipitation into evapotranspiration and runoff based on the water and energy balances (Jones et al., 2012; Padrón et al., 2017), providing a robust approach for water balance assessments even with limited data (Greve et al., 2016). Although originally formulated for natural basins, this method has been extended to quantify anthropogenic impacts on water budgets (Wang and Hejazi, 2011; Lei et al., 2018). Successful applications in various basins have validated its efficacy (Han et al., 2011; Du et al., 2016; Gunkel and Lange, 2017; Shafeeque et al., 2022b). The mathematical equation of our hydrological model is:
Where:
P represents Precipitation,
ETp denotes Potential Evapotranspiration, CWR stands for Crop Water Requirements, and
ω is the Budyko parameter, indicative of catchment characteristics and climatic conditions. In this study, the values for ω ranged from 2 to 4. These values were selected based on prior research and calibrating the hydrological model based on the Budyko theory (Figure 2). The values varied for different climatic regions under investigation within the Indus Basin. It is worth noting that the choice of ω can influence the outcomes of the Budyko framework, especially when calculating the demand-supply gap.
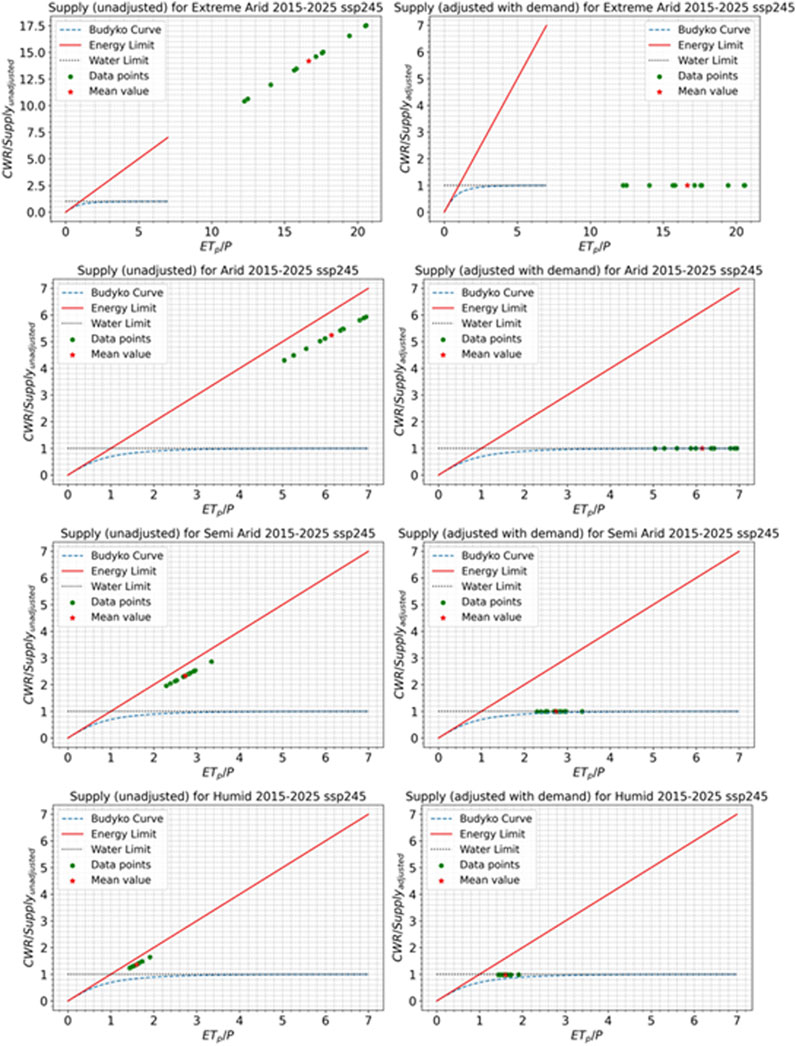
FIGURE 2. Validation of modified Budyko hydrological model for SS245 over all climatic zones during 2015–2025.
ETp was calculated using the Hargreaves equation (Hargreaves and Samani, 1985), requiring only temperature data (Eq. 2). CWR was computed using the empirical climate-crop model developed in this study.
Robust model validation involved two levels. First, supply estimates were validated against observed precipitation (supply unadjusted). Second, modeled demand-supply gaps were evaluated against measured values incorporating precipitation (supply adjusted with demand). This dual validation was conducted for all SSPs and climatic zones over 2015–2,100 and three periods: 2015–2025, 2051–2060, and 2091–2,100. For example, Figure 2 shows the validation of the hydrological model for SSP245 and the first period (2015–2025) over all zones. By integrating the Budyko theory with the latest climatology, the hydrological model provides a novel methodology to project future water deficits across Pakistan’s diverse agro-climatic zones, supporting informed water management.
2.5 Statistical analysis
Various statistical tests were utilized in this study for trend detection, significance testing, and comparative analysis of the different variables across climatic zones, time periods, and emissions scenarios. The presented results in subsequent sections are ensembled of 10 GCMs. The significance level was set at α=0.05 for all statistical tests unless otherwise specified.
The non-parametric Mann-Kendall test (Mann, 1945; Kendall, 1975) was applied to identify monotonic increasing or decreasing trends in the time series data on temperature, precipitation, CWR, and demand-supply gaps over 2015–2,100. Sen’s slope estimator (Sen, 1968) was computed in conjunction with the Mann-Kendall test to quantify the magnitude of significant trends.
Analysis of variance (ANOVA) tests (Fisher, 1925) were conducted to compare the means of different variables across the climatic zones, time slices, seasons, and SSP scenarios. Where significant differences were indicated by ANOVA, post hoc pairwise comparisons using Tukey’s HSD test (Tukey, 1949) were done to identify which specific zones, periods, or scenarios exhibited significant differences in the means.
Paired t-tests (Student, 1908) were utilized for comparing related samples, such as the differences in CWR between the Rabi and Kharif seasons for each climatic zone. For comparing independent samples, unpaired t-tests were applied.
Linear regression analyses (Draper and Smith, 1998) were performed to model the relationships between variables like CWR, precipitation, and time and quantify the change rates over the study period. The significance of regression slopes was tested to evaluate whether the trends differed statistically from zero.
For categorical data such as the direction of precipitation change, Pearson’s chi-square test (Pearson, 1900) was applied to determine if the proportions of increasing versus decreasing trends differed significantly across climatic zones.
3 Results
3.1 Future climate change
3.1.1 Projected temperature changes
The projected annual minimum and maximum temperatures exhibit statistically significant increasing trends across all climatic zones of Pakistan over 2015–2,100 under both SSP245 and SSP585 scenarios (ensemble of 10 GCMs) (Figures 3A, B; Figures 4A, B). The warming trends are time-progressive, with greater temperature anomalies in the end-century period than in the near term compared to baseline 1951–2014 (Figure 3C; Figure 4C).
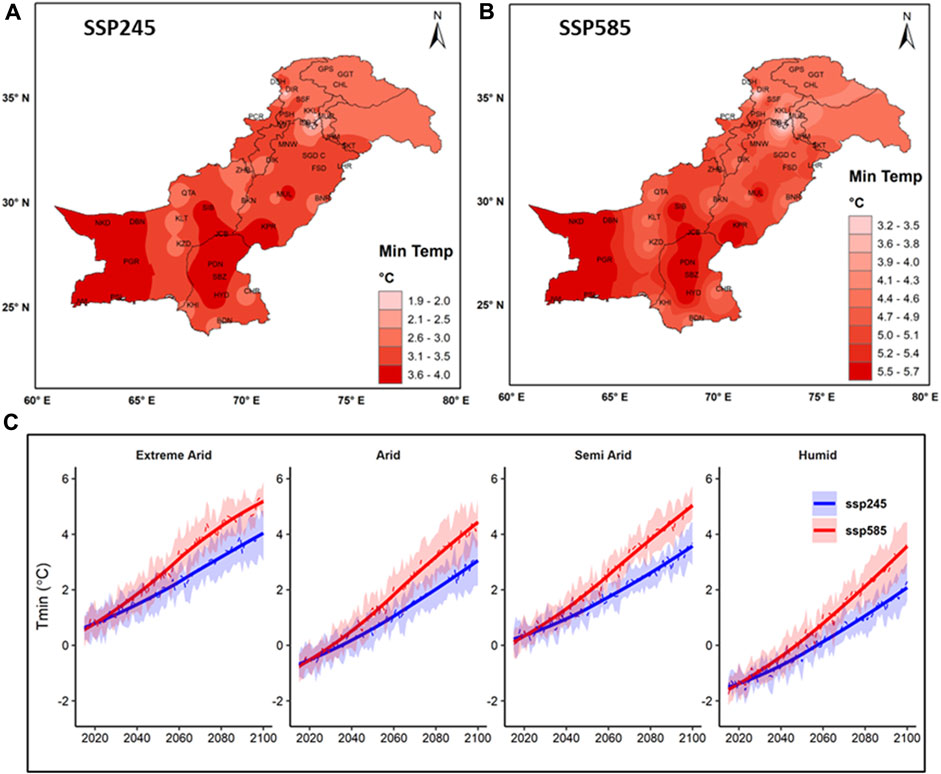
FIGURE 3. Spatiotemporal distribution of projected minimum temperature anomalies during 2091–2,100 compared to 1951–2014 over Pakistan. (A) Spatial distribution for emission scenario SSP245, (B) Spatial distribution for emission scenario SSP585, and (C) time series in four climatic zones for both emission scenarios.
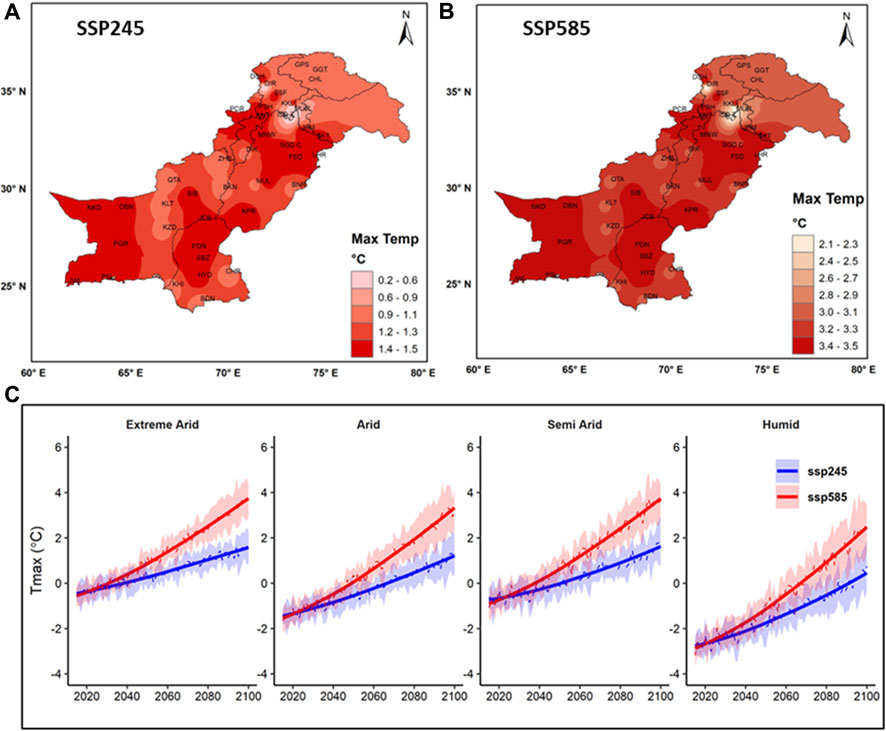
FIGURE 4. Spatiotemporal distribution of projected anomalies of maximum temperature during 2091–2,100 compared to 1951–2014 over Pakistan. (A) Spatial distribution for emission scenario SSP245, (B) Spatial distribution for emission scenario SSP585, and (C) time series in four climatic zones for both emission scenarios.
For minimum temperature, the anomalies by 2025 range from +0.57°C ± 0.43°C in the extreme-arid zone to −1.5°C ± 0.35°C in the humid zone under SSP245. By 2,100, the changes reach +3.62°C ± 0.58°C and +1.62°C ± 0.59°C in the extreme-arid and humid zones, respectively (Figure 3C). The minimum temperature warms at a greater rate under high emissions (SSP585), exceeding SSP245 anomalies in most periods. SSP585 exhibits minimum temperature anomalies of −1.76°C ± 0.45°C in the humid zone and +5.33°C ± 1.45°C in the extreme-arid zone by 2025 and 2,100, respectively (Figure 3C).
For maximum temperature, the anomalies vary from −0.5°C ± 0.34°C in the extreme-arid zone to −2.71°C ± 0.42°C in the humid zone by 2025 under SSP245. By 2,100, the changes reach +1.6°C ± 0.8°C and +2.43°C ± 1.1°C in the extreme-arid and humid zones, respectively. Again, SSP585 shows greater warming than SSP245 in most periods (Figure 4C).
The Mann-Kendall test indicates statistically significant increasing trends for minimum and maximum temperatures over 2015–2,100 across all zones and scenarios. The warming rate is steeper under high emissions (SSP585) versus low emissions (SSP245). The arid north/west regions exhibit greater warming than the humid east zone for both minimum and maximum temperatures.
The spatial asymmetry is statistically significant based on ANOVA tests. Under SSP245, the minimum temperature warming rate is 0.024°C/year in the extreme-arid zone versus 0.010°C/year in the humid zone over 2015–2,100 (Figure 3C). The maximum temperature exhibits a slope of 0.019°C/year in the extreme-arid zone compared to 0.008°C/year in the humid zone (Figure 4C). The differences are enhanced under SSP585. Period-wise analyses confirm that the zonal gradients persist through the 21st century under both scenarios.
3.1.2 Projected precipitation changes
The projected annual precipitation over 2015–2,100 exhibits high interannual variability across all climatic zones of Pakistan under both SSP245 and SSP585 scenarios. However, distinct spatiotemporal patterns emerge in the anomalies relative to the baseline period. The anomalies are enhanced under SSP585 across all zones (Figures 5A, B).
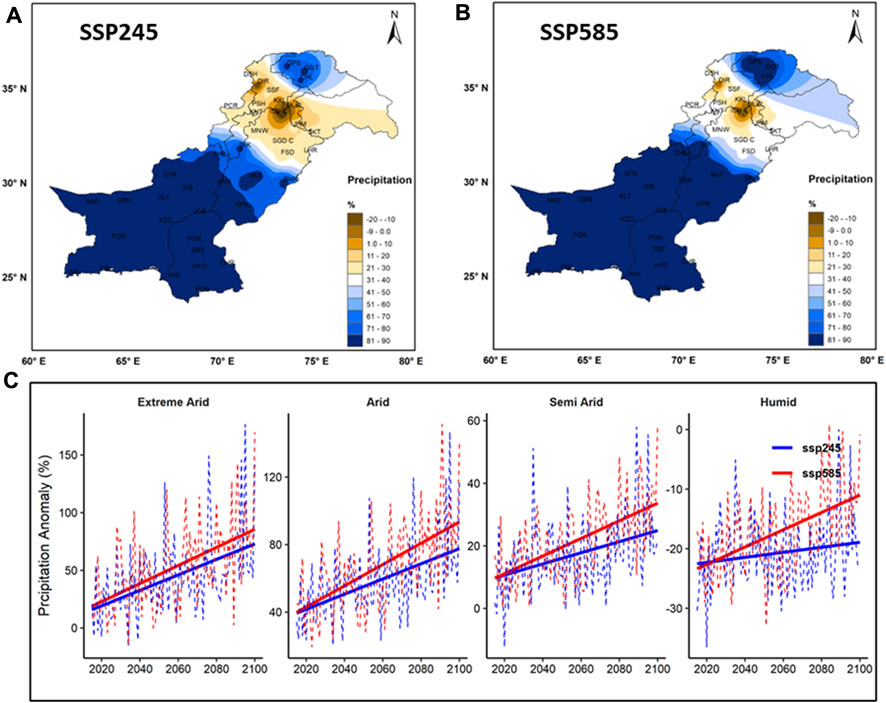
FIGURE 5. Spatiotemporal distribution of projected precipitation anomalies during 2091–2,100 compared to 1951–2014 over Pakistan. (A) Spatial distribution for emission scenario SSP245, (B) Spatial distribution for emission scenario SSP585, and (C) time series in four climatic zones for both emission scenarios.
The Mann-Kendall test indicates increasing but statistically insignificant trends in precipitation anomalies across all zones over 2015–2,100. Under SSP245, the slope is +0.13%/year (p=0.123) in the extreme-arid zone, +0.16%/year (p=0.081) in the arid zone, +0.08%/year (p=0.212) in the semi-arid zone, and +0.01%/year (p=0.732) in the humid zone (Figure 5C). The trends are slightly enhanced under SSP585 but remain statistically insignificant. ANOVA reveals no significant differences between zones in mean precipitation anomalies over 2015–2,100 under both SSPs.
By 2051–2060, anomalies turn positive (wetting) across all zones, but changes are insignificant. In the end-century period of 2091–2,100, most zones exhibit significant wetting (p<0.01), while the semi-arid zone shows insignificant wetting. Under SSP585, anomalies are enhanced, but trends remain insignificant due to high interannual variability.
3.2 Future crop water requirements
Annual CWR showed statistically significant increasing trends across all climatic zones and emission scenarios over 2015–2,100 (p<0.001 in all cases). The rate of increase in CWR was higher under SSP585 compared to SSP245 across the zones (Figure 6).
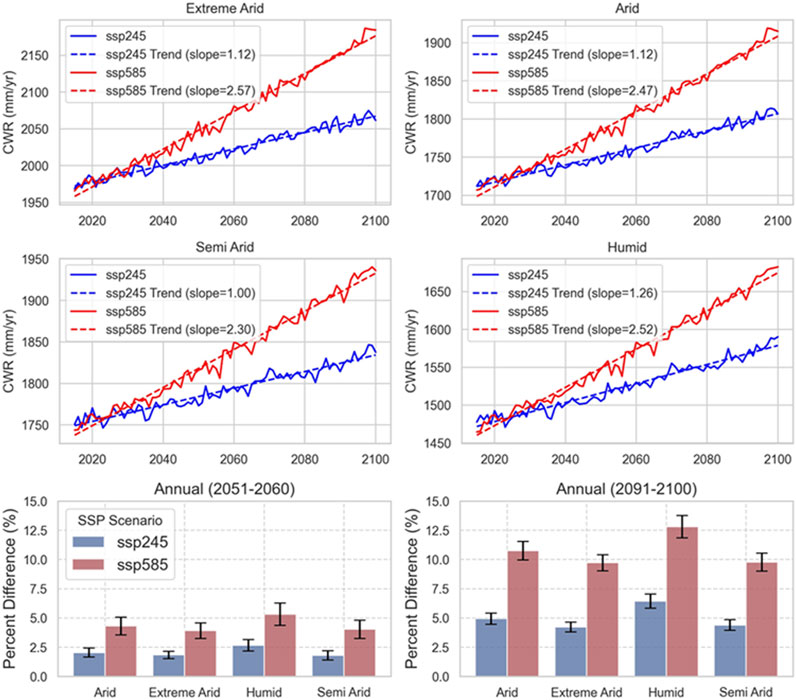
FIGURE 6. Projected annual crop water requirements (CWR) in four climatic zones under SSP245 and SSP585 from 2015 to 2,100. The dotted lines show the projected CWR trend. The bar plots show the differences (%) in CWR during 2051–2060 and 2091–2,100 compared to 2015–2025 at an annual scale.
Comparing the three time periods of 2015–2025, 2051–2060, and 2091–2,100, annual CWR increased significantly from the near-term period to the end-century period across zones, seasons, and emissions scenarios. In the semi-arid zone, mean CWR escalated by 2% under SSP245 (p<0.001). The increase was more pronounced under SSP585, by 3.5% in 2015–2025 to 9% in 2091–2,100 for this zone (p<0.001) (Figure 6).
The humid climatic zone showed the lowest increase in annual CWR overtime compared to the other zones. Under SSP245, the CWR in the extreme-arid, arid, semi-arid, and humid zones increased at 1.12, 1.12,1.00, and 1.26 mm/yr at an annual scale (p<0.001). Under SSP585, the CWR in the extreme-arid, arid, semi-arid, and humid zones increased at 2.57, 2.47, 2.30, and 2.52 mm/yr at annual scale (p<0.001) (Figure 6). The increasing CWR across zones can be attributed to rising temperatures and subsequent increases in evapotranspiration. The higher rate of increase under SSP585 versus SSP245 aligns with greater warming expected under higher emissions scenarios, as projected by the IPCC (2021). The lower sensitivity in the humid zone is likely due to the moderating effects of higher rainfall. In contrast, hot and dry zones like extreme-arid will likely be more vulnerable to warming impacts.
Relative to 2015–2025 under SSP245, the mean annual CWR rose by 2%–2.6 % and 4.2%–6.4 % for all zones in 2051–2060 and 2091–2,100. Under SSP585, the increases ranged from 4% to 5.3% (2051–2060) and 9%–10.7% in all zones (2091–2,100) (Figure 6).
Seasonal CWR exhibited significantly increasing trends in the Rabi and Kharif seasons across the four climatic zones and SSP scenarios over 2015–2,100 (p<0.001 in all cases). The rate of increase was markedly higher in the Kharif season compared to the Rabi season across zones and SSPs. For instance, under SSP245, the CWR in the extreme-arid, arid, semi-arid, and humid zones increased at 0.48, 0.64, 0.53, and 0.74 mm/yr in Kharif and 0.64, 0.48, 0.47, and 0.51 mm/yr in Rabi (p<0.001). Under SSP585, the CWR in the extreme-arid, arid, semi-arid, and humid zones increased at 1.26, 1.44, 1.29, and 1.54 mm/yr in Kharif and 1.21, 1.03, 1.01, and 0.98 mm/yr in Rabi (p<0.001) (Figure 7).
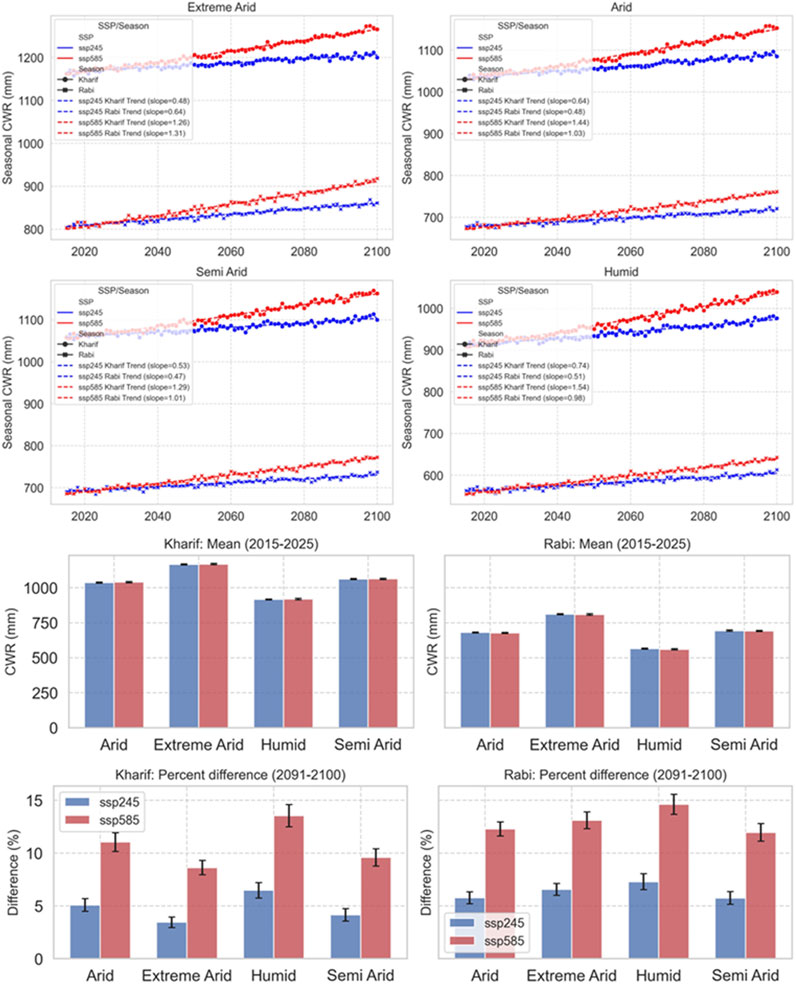
FIGURE 7. Projected seasonal crop water requirements (CWR) in four climatic zones under SSP245 and SSP585 from 2015 to 2,100. The dotted lines show the projected seasonal CWR trend. The bar plots show the CWR values for the Kharif and Rabi seasons during 2015–2025. The bar plots show the differences (%) in CWR during 2051–2060 and 2091–2,100 compared to 2015–2025 for Kharif and Rabi seasons.
The Kharif season exhibited significantly higher CWR than the Rabi season across all zones and timeframes. For instance, under SSP245 and SSP585, the mean CWR over 2015–2025 was 1,166 and 1,169 mm in Kharif versus 810 and 808 mm in Rabi for the extreme-arid zone (p<0.001). The Kharif-Rabi differential was most pronounced in the humid climatic zone, with mean CWR of 916 and 918 mm in Kharif versus 565 and 560 mm in Rabi under SSP245 and SSP585 (p<0.001).
Monthly CWR showed distinct seasonal patterns with higher values in summer (Kharif) months and lower values in winter (Rabi) months consistently across all climatic zones and scenarios. Monthly CWR was consistently higher under SSP585 compared to SSP245 across zones and months over 2015–2,100, with percent differences ranging from 3% to 14% depending on month and zone (p<0.01, paired t-test) (Figure 8).
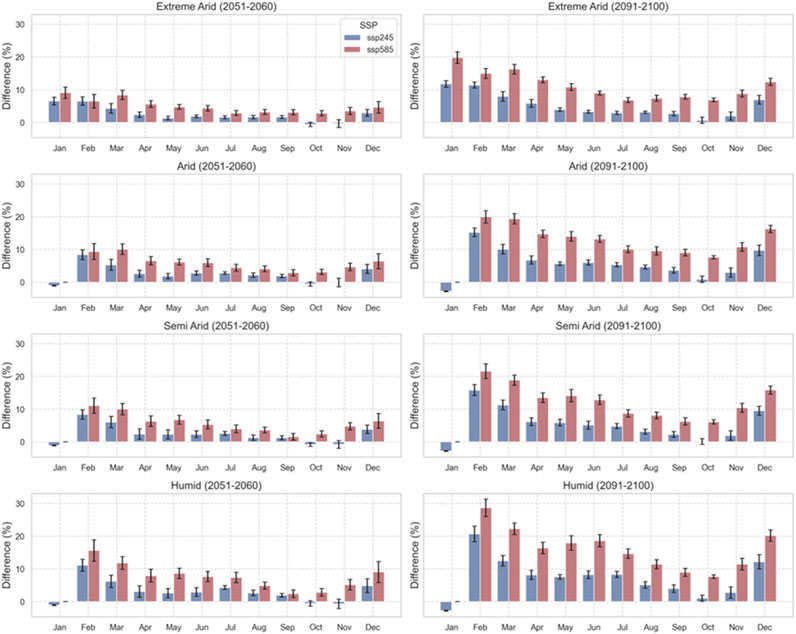
FIGURE 8. Projected changes (%) in monthly crop water requirements (CWR) in four climatic zones under SSP245 and SSP585 during 2051–2060 and 2091–2,100 compared to 2015–2025.
Average monthly CWR showed a negative percent difference in January for all climatic zones except extreme-arid under SSP245 and SSP585 from 2051 to 2060 to 2091–2,100 (Figure 8). For all zones, monthly CWR showed low changes in October under SSP245 over 2051–2060 and 2091–2,100. The changes in monthly CWR were higher in February for the arid, semi-arid, and humid zones, ranging from 15% to 19%, 15%–21%, and 20%–28% under both SSP245 and SSP585 over 2091–2,100 (Figure 8).
3.3 Future demand and supply gap
The projected annual demand-supply gap showed an increasing trend under both SSP scenarios across all climatic zones and periods compared to 2015–2025. The increase was more pronounced under SSP585 than SSP245, indicating greater vulnerability under the high emissions scenario. The humid zone exhibited the smallest rise in the demand-supply gap, while the arid zone displayed the most substantial increase under both SSPs.
In the 2015–2025 period under SSP245, the mean annual demand-supply gap was significantly higher (ANOVA, p<0.05) in the extreme arid zone compared to other zones. A similar pattern was observed under SSP585, with the extreme-arid zone showing the greatest gap trailed by arid, semi-arid, and humid (Table 3). Differences among zones were statistically significant for all periods under both SSPs (ANOVA, p<0.01).
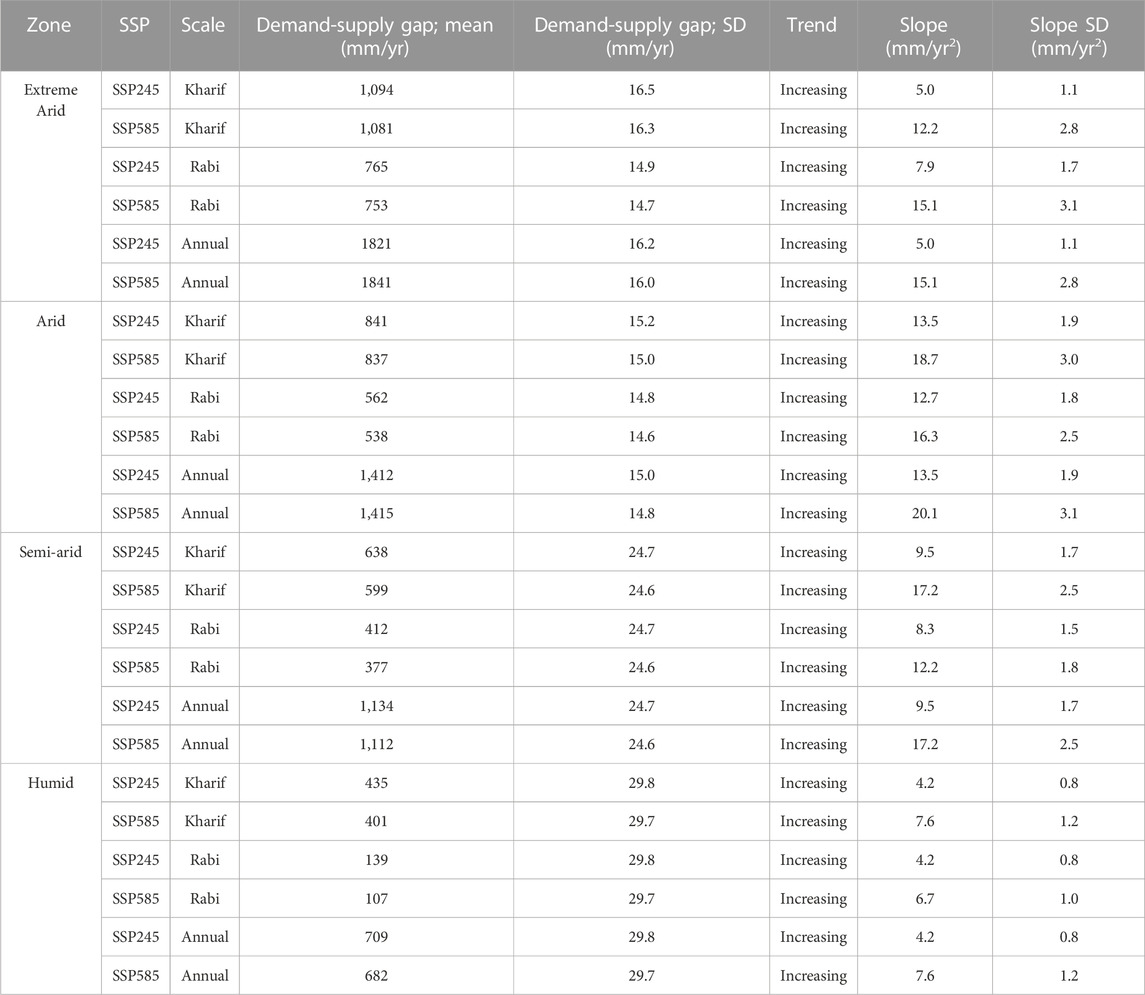
TABLE 3. Mean projected demand-supply gap and trend slope for four climatic zones under SSP245 and SSP585 at annual and seasonal scales from 2015 to 2,100.
Linear regression revealed statistically significant increasing trends in the demand-supply gap over 2015–2,100 across all zones and SSPs (p<0.001). Under SSP245, the slopes were 13.5, 9.5, 5.0, and 4.2 mm/yr2 for the arid, semi-arid, extreme-arid, and humid zones, respectively (Table 3). The slopes were steeper under SSP585 at 20.1, 17.2, 15.1, and 7.6 mm/yr2 for the respective zones.
Relative to 2015–2025 under SSP245, the mean annual demand-supply gap rose by 10% and 15% in the arid zone and 8% and 12% in the semi-arid zone during 2051–2060 and 2091–2,100 (Figure 9). Under SSP585, the increases were higher at 18% and 32 % in the arid zone and 14% and 24% in the semi-arid zone for the same future periods. Similar escalating patterns were seen in the extreme-arid and humid zones under both SSPs compared to 2015–2025.
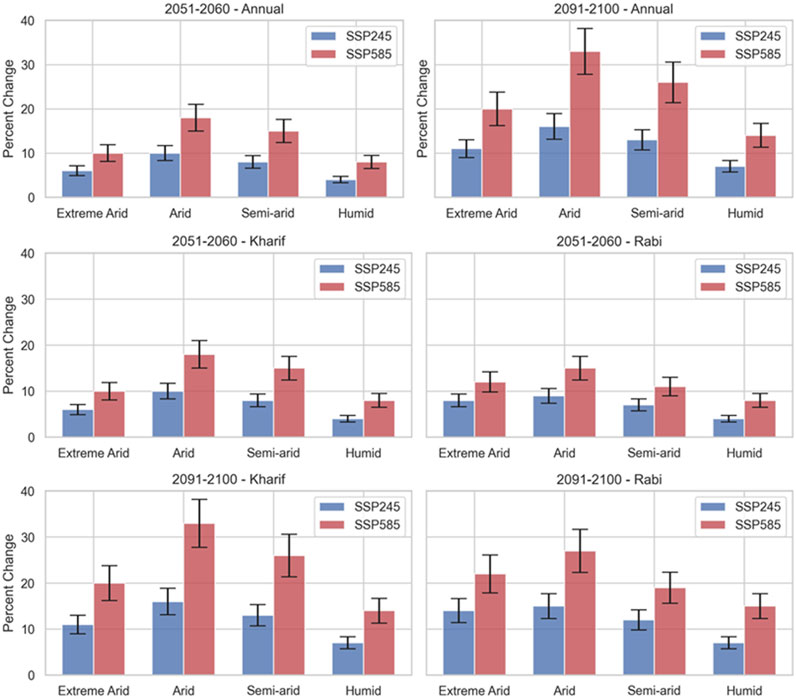
FIGURE 9. Projected changes (%) in annual and seasonal agricultural demand-supply gap in four climatic zones under SSP245 and SSP585 during 2051–2060 and 2091–2,100 compared to 2015–2025.
Under SSP245 in 2015–2025, the mean Kharif demand-supply gap was significantly higher in the arid zone (841 mm) compared to other zones (ANOVA, p<0.05). For Rabi, the arid zone also showed the highest mean gap (556 mm) in 2015–2025, markedly exceeding the extreme-arid, semi-arid, and humid zones (ANOVA, p<0.01) (Table 3). This pattern persisted across periods under SSP245, with the arid zone displaying the greatest Kharif and Rabi gaps followed by extreme-arid, semi-arid, and humid. Similar trends were seen under SSP585 of the arid zone having the most substantial gaps.
Regression analyses revealed significantly increasing linear trends in the Kharif demand-supply gap over 2015–2,100 for all zones and SSPs (p<0.001). The slopes ranged from 4.2 to 13.5 mm/yr2 under SSP245 and 7.6–18.7 mm/yr2 under SSP585 (Table 3). Significant increasing Rabi trends were also found across zones and scenarios (p<0.01), with steeper SSP585 slopes.
For the Rabi and Kharif seasons, the projected seasonal demand-supply gap increased over 2015–2,100 across all zones under SSP245 and SSP585 relative to 2015–2025 (Table 3). Mirroring the annual trend, the rise was more pronounced under SSP585 versus SSP245. The Kharif season exhibited a higher demand-supply gap than Rabi for most zones and periods (Figure 9), likely owing to greater crop water needs in summer.
Relative to 2015–2025 under SSP245, the mean Kharif demand-supply gap rose by 4%–10% in 2051–2060 and 7%–15% in 2091–2,100 for all zones (Figure 9). For Rabi, the increases were 4%–9% and 7%–13% in these zones over the same future periods. The rises were greater under SSP585, pointing to heightened vulnerability in the future. Relative to 2015–2025, under SSP585, the mean Kharif demand-supply gap rose by 8%–18% in 2051–2060 and 13%–32% in 2091–2,100 for all zones. Similarly, Rabi’s increases were 8%–15% and 15%–28% in these zones over 2051–2060 and 2091–2,100, respectively.
4 Discussion
4.1 Implications of asymmetric warming and precipitation variabilities on Pakistan’s agricultural adaptation
The statistically significant warming trends for both minimum and maximum temperatures are consistent with the thermodynamic effects of rising greenhouse gases under the SSP scenarios (Lund et al., 2020; Nazarenko et al., 2022). The higher emissions pathway (SSP585) leads to more significant warming, highlighting the potential benefits of mitigation efforts (Hasegawa et al., 2018).
The spatial analysis reveals greater warming rates for both variables in the arid north/west versus the humid east (Figures 3A, B; Figures 4A, B). This asymmetry likely reflects land-atmosphere feedbacks whereby soil moisture depletion amplifies summer daytime heating in drier zones (Khan et al., 2019; Nadeem et al., 2022). In contrast, atmospheric dynamics and higher moisture availability likely moderate the warming in the humid east region (Iqbal et al., 2016).
Uneven zonal warming (Figures 3, 4) has critical implications for agriculture. The hot and arid zones are at higher risk of extreme heat stress during sensitive crop growth stages (Adnan et al., 2017; Nasim et al., 2018). Targeted adaptation strategies such as heat-tolerant varieties, adjusted planting schedules, and efficient irrigation will be essential, especially in the food-producing arid and semi-arid regions.
The significant warming trends (Figure 3C; Figure 4C) will also alter evapotranspiration rates and CWR across Pakistan (Naheed and Rasul, 2010a; Zhu et al., 2013; Ahmad et al., 2021), exacerbating risks to food security. Sustainable agricultural water management will be crucial for building resilience to asymmetric regional warming. The results underscore the urgency of adaptation planning focused on the most sensitive arid and semi-arid agricultural zones (Mehmood, 2021), in conjunction with ambitious global mitigation efforts to limit the degree of warming.
The statistically insignificant precipitation trends highlight considerable uncertainties in regional climate projections over Pakistan, underscoring the modulating effects of natural variability (Gadiwala and Burke, 2019; Khan et al., 2020; Nusrat et al., 2022). However, significant drying of the humid zone in the early 21st century (Figure 5) reflects the suppressing effects of anthropogenic aerosols (Ahmed et al., 2019b; Li et al., 2020). The lack of robust signals can be attributed to countervailing dynamics - while thermodynamic factors promote wetting, circulation changes related to weakening monsoons may reduce precipitation (Ahmed et al., 2019a; Chand and Ahmad, 2020). Complex orography also complicates the simulation of spatial patterns of climatic variables (Ahmed et al., 2020), resulting in uncertainties in the projected CWR and demand-supply gap. Such uncertainties pose challenges for adapting Pakistan’s vulnerable agriculture sector (Waseem et al., 2020; Zhou et al., 2020). Advances in climate modeling and dynamical downscaling are essential to provide accurate climate projections for the region (Giorgi et al., 2011). The asymmetric precipitation responses across zones, with the wetting of arid regions and drying of humid areas (Figure 5), are consistent with expected thermodynamic responses under global warming (Ali et al., 2016; Athar et al., 2021). However, dynamical circulation changes can overturn these gradients, highlighting the complex interplay of driving factors (Giorgi et al., 2011). Targeted high-resolution modeling is critical to disentangling the thermodynamic and dynamic processes governing heterogeneous precipitation changes across Pakistan’s climatic zones under climate change.
4.2 Climate-driven variations in crop water requirements: impacts and adaptation in Pakistan’s agro-climatic zones
The projected changes in climate (Figures 3–5) are expected to impact CWR across the distinct climatic zones of Pakistan significantly. The increasing temperatures (Figures 3, 4) will likely enhance evapotranspiration, thereby raising CWR, consistent with previous studies (Liu et al., 2016; Arshad et al., 2019; Haider and Ullah, 2020; Dawadi et al., 2022). More markedly, the rate of increase in CWR is projected to be higher under the high emissions scenario SSP585 compared to SSP245 (Figure 6). This aligns with the greater warming expected under pathways with higher radiative forcing, as highlighted by the IPCC (2021).
The trends in CWR also vary across the climatic zones of Pakistan, with the humid zone exhibiting the highest percent changes over time (Figure 6). This could be attributed to the moderating influence of higher rainfall in the humid zone (Figure 1B; Figure 5), which may help offset some of the impacts of warming on evapotranspiration and crop water needs. In contrast, hot and dry zones like arid and extreme-arid are likely to be more sensitive to warming impacts, reflected in the higher rate of increase in CWR projected for these regions. Our findings agree with previous studies (Naheed and Rasul, 2010a; Haider and Ullah, 2020) that projected increasing CWR in the country’s northern half.
The implications of these projected changes in CWR on agricultural water demand and supply can be significant for a water-stressed country like Pakistan. Meeting the rising crop water needs would require additional water withdrawals for irrigation, putting further pressure on already limited water resources, especially in drier regions (Laghari et al., 2012; Kirby et al., 2017). Pakistan’s per capita water storage capacity is alarmingly low compared to other nations, and surface water supplies are projected to decrease by 30% in the coming decades due to climate change and reservoir silting, according to (Qureshi, 2015). To address this looming crisis, researchers recommend both supply-side and demand-side solutions. On the supply side, increasing water storage capacity and conjunctive use of surface and groundwater are suggested by Qureshi (2011) and Qureshi (2015). However, overexploitation of aquifers is already occurring, so groundwater use must be carefully managed, as Cheema et al. (2014) argue. On the demand side, increasing irrigation efficiency and crop productivity is critical. Zhu et al. (2013) and Kijne (2001) recommend transitioning from supply-based to demand-based water management, which involves forming water user associations and instituting water pricing reforms. Improving crop yields through agricultural research and development is also key, according to Zhu et al. (2013) and Yu et al. (2013). Carefully selecting cropping patterns and cultivars suited to water-limited conditions can significantly improve resilience. Debaeke and Aboudrare (2004) outline how farmers can choose drought-resistant crops and varieties, adjust planting dates and densities, and optimize irrigation timing to cope with drought. Bodner et al. (2015) argue that understanding site-specific hydrology and plant-soil interactions is necessary to choose appropriate management strategies. Flexible, adaptable management systems that can respond to unpredictable weather changes are ideal, according to Debaeke and Aboudrare (2004). Pakistan must act urgently to implement adaptive water management and agricultural strategies to address the worsening gap between supply and demand (Kirby et al., 2017). Improving irrigation efficiency, choosing appropriate cropping patterns, and developing drought-resistant cultivars can help build resilience to water scarcity and ensure future food security. With comprehensive action on both the supply and demand sides, Pakistan can overcome this crisis and protect its agricultural economy. Our findings (Figures 6–9) provide valuable agro-climatic zone-specific insights to guide efforts for navigating the climate change challenges facing Pakistan’s agricultural water sector.
Our results indicate that CWR in both the major Kharif and Rabi growing seasons are projected to rise markedly across Pakistan’s agro-climatic zones by 2,100 (Figure 7). The escalating trends are concerning as they signify increasing irrigation water demands in the future, which could considerably strain water resources.
Our results highlighted a higher rate of increase in CWR under SSP585 versus SSP245, demonstrating that CWR is responsive to the magnitude of climate change, with more significant warming driving higher evapotranspirative crop water needs (Ruan et al., 2020; Ahmad et al., 2021; Tuladhar et al., 2023). Given the existing challenges of water scarcity and imbalance between supply and demand (Table 3; Figure 9) in many parts of Pakistan, this has serious implications for agriculture and food security concerns.
While CWR rises across zones, the lower incremental rate of change seen in humid regions highlights the mitigating effects of higher precipitation. In contrast, hot and dry areas like the extreme-arid and arid zones display greater sensitivity to warming impacts that drive up CWR due to limitations in water availability for irrigation (Qureshi, 2015).
Our findings indicate that CWR exhibits a strong seasonal cycle in different climatic zones of Pakistan (Figures 7, 8), aligning with the summer monsoon climate when rainfall peaks, yet peaking specifically in August according to multiple studies (Naheed and Rasul, 2010a; Imran et al., 2013; Haider and Ullah, 2020). Despite this rainfall, which is often unevenly distributed, supplemental irrigation is essential, particularly in Pakistan’s drier regions, due to insufficient compensation for increased evapotranspiration during warmer months (Naheed and Rasul, 2010a; Ahmad and Choi, 2021; Khan et al., 2021b). Southern Pakistan, including south Punjab and Sindh, consistently requires irrigation throughout the growing season. Projected climate change scenarios featuring increased temperatures (Figures 3, 4) are expected to further elevate CWR, with some estimates suggesting increases up to 200 mm per season in upcoming decades (Naheed and Rasul, 2010a; Haider and Ullah, 2020). Notably, CWR in Pakistan varies significantly based on location and crop type, being higher in southern regions and differing among crops—cotton (Kharif crops), for instance, demands more water than Rabi crops, for example, wheat (Naheed and Rasul, 2010a; Haider and Ullah, 2020; Khan et al., 2021b). Specific environmental conditions, weather, and crop characteristics influence these requirements. The peak CWR during the summer monsoon months underscores this season’s crucial role in fulfilling crop water needs.
However, the projected rises in summer CWR under both emissions scenarios highlight the threat posed by climate change to the delicate monsoon-agriculture relationship in South Asia. Existing research points to an intensification of the regional hydrological cycle with global warming, resulting in increased variability in the South Asian monsoon (Kitoh et al., 2013). Our findings concur with studies that have warned about growing unpredictability in the timing and strength of monsoon rainfall under climate change (Wake, 2015; Langenbrunner, 2019). This unpredictability threatens the ability of Kharif season rainfall to adequately meet escalating peak irrigation requirements, increasing reliance on irrigation from other constrained water sources, for example, diverting canal water and abstracting groundwater.
The significantly higher monthly CWR projected for Pakistan’s arid regions corresponds to previous observations that agriculture in these already water-stressed arid and semi-arid zones is particularly vulnerable to climate change impacts. Our results align with global projections highlighting heightened risks to agriculture in water-scarce regions under climate change (Elliott et al., 2014; Liu et al., 2016). The disparity between the arid and humid zones underscores the need for regional nuance in climate adaptation planning to account for locational differences in impacts.
Coping with the additional pressure on water resources will involve enhancing the efficiency of surface irrigation that currently dominates Pakistan’s agricultural lands (Janjua et al., 2021). However, technical solutions must be accompanied by integrated water management approaches encompassing cropping pattern optimization, on-farm storage, precision irrigation technologies, institutional reforms, and strengthening smallholder farmers’ capacities (Siyal et al., 2023).
Our findings provide robust evidence that climate change poses significant risks to agriculture and water security sustainability in Pakistan’s arid regions. Spatiotemporal insights can inform targeted mitigation and adaptation policies to build resilience. However, addressing these complex challenges requires recognizing climate risks embedded within the larger social, economic, and political forces shaping regional water constraints.
4.3 Vulnerability assessment of climatic zones and strategic water management adaptations in Pakistan’s agriculture
The projected widening gap between CWR and precipitation-derived supply indicates growing water scarcity across climatic zones. The findings reveal concerning implications of climate change for agricultural water availability across Pakistan’s agroecological regions.
Under SSP245, the annual demand-supply gap increased significantly from the 2015–2025 baseline by 10%–15% in the arid zone and 8%–12% in the semi-arid zone during 2051–2060 and 2091–2,100. The rises exceeded 30% in certain zones under SSP585, indicating heightened vulnerability. Statistical analyses confirmed the significant spatiotemporal increases under both SSPs (p<0.01).
The increasing trends in the demand-supply gap have profound sustainability implications for Pakistani agriculture, which is heavily dependent on irrigation (∼90% of water use) (Qureshi, 2015). Our findings concur with studies predicting declining per capita water availability under climate change (Immerzeel et al., 2020).
The arid and semi-arid zones appear most vulnerable, exhibiting the largest absolute gap between CWR and supply annually and seasonally. These zones are the main agricultural regions of the country, and the food demand stress in these regions is enormously increasing, which increases the water demand in these regions (Qureshi, 2011; 2015; Rafiqul Islam, 2022; Hasan and Abed, 2023; Haseeb et al., 2023). This aligns with research showing heightened evapotranspiration in arid agricultural areas due to warming (Ahmed et al., 2021). However, the challenges extend beyond these regions, with significant rising trends across zones, underscoring the systemic nature of this issue.
The analysis highlights the urgent need for agricultural adaptation. Expanding irrigation infrastructure could help close the demand-supply gap (Qureshi, 2011). Improving conveyance efficiency and adopting precision irrigation techniques may substantially reduce the water demand. Diversifying toward drought-tolerant crops is another potential strategy (Rasul and Sharma, 2016).
Without strategic adaptation, climate change is projected to exacerbate Pakistan’s agricultural water challenges, with potential food security implications given the sector’s massive water needs. An integrated policy response focused on infrastructure, efficient technologies, and sustainable cropping patterns will be critical for climate resilience.
The findings also have serious seasonal water availability implications for major cropping periods. The Kharif demand-supply gap is projected to widen substantially, increasing by 8%–10% in the arid and semi-arid zones during 2051–2060 and 12%–15% in 2091–2,100 under SSP245 versus 2015–2025 (Figure 9). Rabi demand-supply gaps also rose markedly over time.
This indicates systemic intensifying constraints across regions, concurring with studies on declining water availability (Immerzeel et al., 2020). The arid and semi-arid zones also appeared to be the most vulnerable seasonally, displaying the highest Kharif and Rabi gaps, likely due to hot, dry conditions driving crop water needs (Haider and Ullah, 2020; Ahmad et al., 2021). However, significant rising trends across zones underscore the national-scale implications.
Our analysis highlights the need for strategic seasonal adaptation, like expanding reservoir storage for Kharif and improving irrigation efficiency for Rabi, as suggested by (Qureshi, 2015; Siyal et al., 2023). Adjusting cropping patterns towards less water-intensive varieties will also be critical (Rasul and Sharma, 2016). Without urgent adaptations, the projected rising seasonal gaps portend risks for Pakistan’s climate-vulnerable food production and security.
4.4 Implications: navigating climate and agriculture interplay, methodological advances, and pathways forward
Amidst an era of pronounced climatic shifts, the interplay between climate change and agricultural sustainability has become a focal point of global discourse. This study, rooted in Pakistan’s diverse agroclimatic canvas, offers an empirically robust exploration into this relationship by advancing the climate-crop model. Employing the cutting-edge CMIP6 (10 GCMs) climate projections under SSP245 and SSP585 scenarios, the research meticulously projects the future CWR and agricultural demand and supply gap across Pakistan. The findings, accentuated under the high emissions scenario SSP585, illuminate the impending challenges of meeting escalating CWR and widening the demand-supply gap in a warming landscape.
Complementing this, the study modified and improved the deployment of the hydrological model based on Budyko theory, offering a granular view of the widening agricultural water demand-supply gap. Notably, seasonal nuances, especially the exacerbated gaps during the Kharif season, underscore the complexities of managing agricultural water resources under shifting climate paradigms.
For policymakers and stakeholders, these findings translate into an urgent call for action. With Pakistan’s agrarian backbone facing potential water stress, strategic interventions—ranging from enhancing irrigation infrastructure to fostering drought-resilient crops—emerge as non-negotiable imperatives. Moreover, the study’s zone-specific insights provide a roadmap for tailoring interventions, ensuring that adaptation efforts are both localized and impactful.
For the broader academic community, this research serves as a benchmark, improving and blending rigorous methodologies with the latest climate projections to elucidate climate-agriculture dynamics in a developing nation context. Furthermore, the study’s findings align with and expand upon existing literature, offering fresh perspectives and data points for future research.
As the specter of climate change looms larger, this study illuminates the pressing challenges and potential pathways forward for Pakistan’s agricultural sector. Beyond its academic merit, the research stands as a clarion call, urging a synthesis of science, policy, and on-ground action to safeguard food security and agricultural sustainability in an era of unprecedented change.
4.5 Methodological constraints, uncertainties, and avenues for future research
Our study utilized projections from 10 GCMs to 2 SSPs (SSP245 and SSP585) of CMIP6. While capturing a breadth of potential futures, these models may exhibit varying sensitivities to greenhouse emission forcing, leading to divergent outputs for temperature and precipitation, particularly at regional scales (Deser et al., 2020). While multi-model ensembles like ours provide a comprehensive view, biases inherent to individual models may persist.
To refine GCM outputs for impact models, we adopted statistical downscaling and bias correction methods, notably quantile mapping. While widely accepted, these techniques carry statistical assumptions that might propagate uncertainties (Maraun et al., 2017). However, given the scale and scope of our study, they were the most suitable methods available.
The temperature-driven Hargreaves method was employed for potential evapotranspiration estimation, a choice made due to limited meteorological data despite its potential uncertainties compared to energy balance approaches (Droogers and Allen, 2002). It is evident that calibrating the empirical Hargreaves coefficient (Feng et al., 2017; Srivastava et al., 2018) and incorporating the soil moisture factor (S) in the climate-crop model (Eq. 1) improve the accuracy of the CWR projections when meteorological data are limited.
Our empirical climate-crop model made simplifying assumptions, such as using generalized crop coefficients for major crops, which might affect crop water requirement estimates (Steduto et al., 2012). However, given the data limitations, such a choice was rational and necessary. Furthermore, while interannual and decadal climate variabilities were not explicitly simulated, our utilization of a large ensemble aimed to encapsulate this source of uncertainty (Deser et al., 2020).
In the context of our application of the Budyko framework to the Indus Basin, it is crucial to acknowledge a methodological constraint related to water supplies. The Budyko framework traditionally operates under the assumption that local water supplies are predominantly derived from precipitation (Greve et al., 2015). While this assumption may hold in many regions globally, the Indus Basin exhibits a nuanced hydrological dynamic. Notably, a significant portion of the basin’s water supply originates from its river system, which is largely sustained by the melting of snow and glaciers (Lutz et al., 2016; Shafeeque et al., 2022a). This riverine input becomes even more pivotal during dry seasons when precipitation is at its lowest (Senbeta et al., 2023). For the purpose of this study, we chose to concentrate on precipitation-based supplies, thereby offering a foundational yet potentially oversimplified understanding of the water dynamics in the Indus Basin. Future research avenues should consider developing a more intricate model within the Budyko framework that encapsulates river discharge, glacier melt rates, and groundwater contributions, ensuring a holistic representation of the Indus Basin’s water availability dynamics.
While the Budyko framework provides a foundational understanding of hydrological dynamics, the choice of ω introduces uncertainty (Wang and Fu, 2018). The sensitivity of ω values in the Budyko framework is a recognized methodological concern (Singh and Kumar, 2015). The choice of ω significantly impacts predictions, with the greatest uncertainty under mild climate conditions (Guo et al., 2019). In our analysis, varying ω within the range of 2–4 can lead to different estimations of the demand-supply gap. A lower ω value tends to predict a smaller gap, implying a closer balance between demand and supply. Conversely, a higher ω suggests a larger gap, indicating potential water stress in the region. It is imperative to recognize that while the Budyko framework provides a foundational understanding of the hydrological dynamics, the choice of ω introduces a degree of uncertainty. While the Budyko framework is invaluable for assessing water availability, the sensitivity of ω necessitates approaches incorporating joint distributions and additional processes like vegetation dynamics, especially at small scales. Advancing the Budyko framework will require diverse, representative data, determining how the Budyko curve changes with climate change, and exploring laws for selecting variables and statistical methods (Gudmundsson et al., 2016). The Budyko framework can provide a foundational yet comprehensive understanding of hydrological dynamics across scales with these improvements. Future research might benefit from a more detailed sensitivity analysis of ω values to understand their implications better and refine predictions on water availability in the Indus Basin
Future research should consider more advanced downscaling techniques, detailed crop coefficients validated against field data, process-based crop growth models, and catchment-specific Budyko parameters. Additionally, integrated modeling platforms can comprehensively evaluate the ramifications of climate change on water resources and food security in Pakistan. It is paramount to continually validate and refine projections against observed data to enhance model performance and credibility.
5 Conclusion
This study offers vital projections on the impacts of climate change on CWR and the agricultural water demand-supply gap in Pakistan until 2,100. We improved and synergistically applied the empirical climate-crop model, which revealed significantly increasing trends in CWR across climatic zones, and the hydrological model based on the Budyko theory, which projects the widening gaps between rising CWR and precipitation-derived water supply. Increasing temperature trends were evident across all climatic zones, with a steeper rise under SSP585, especially in arid regions. Despite the uncertainty in precipitation trends, the hot and dry regions displayed heightened vulnerability to CWR increases due to climate change.
The widening gap between rising CWR and water supply indicates heightened risks on a national scale, with arid and semi-arid zones being the most affected. Such findings underscore Pakistan’s need for strategic climate adaptation, especially in its agriculture sector, which heavily relies on irrigation. Focused efforts on enhancing irrigation efficiency, adjusting cropping patterns, and developing resilient crops are essential. Furthermore, based on our zonal findings, localized strategies can optimize adaptation endeavors. Integrating scientific insights with community knowledge is crucial for crafting effective policies. Our study serves as a timely guide for prioritizing and tailoring adaptation efforts in Pakistan, emphasizing the cooperation between science and policy to address looming climate challenges.
Data availability statement
The original contributions presented in the study are included in the article/Supplementary Material, further inquiries can be directed to the corresponding author.
Author contributions
MS: Conceptualization, Data curation, Formal Analysis, Funding acquisition, Investigation, Methodology, Software, Supervision, Validation, Visualization, Writing–original draft, Writing–review and editing. AB: Conceptualization, Data curation, Formal Analysis, Writing–original draft, Writing–review and editing.
Funding
The author(s) declare financial support was received for the research, authorship, and/or publication of this article. The first author acknowledges the funding from Deutsche Forschungsgemeinschaft (DFG: MA 6966/6-1).
Acknowledgments
The authors are thankful to the Pakistan Meteorological Department (PMD) and Water and Power Development Authority (WAPDA) of Pakistan for providing the observational data. The links and references are provided in the text for CMIP6 and other data used in the study.
Conflict of interest
The authors declare that the research was conducted in the absence of any commercial or financial relationships that could be construed as a potential conflict of interest.
Publisher’s note
All claims expressed in this article are solely those of the authors and do not necessarily represent those of their affiliated organizations, or those of the publisher, the editors and the reviewers. Any product that may be evaluated in this article, or claim that may be made by its manufacturer, is not guaranteed or endorsed by the publisher.
References
Adnan, S., and Haider, S. (2014). Classification and Assessment of Aridity Over Pakistan Provinces (1960-2009). Int. J. Environ. 3, 24–35. doi:10.3126/ije.v3i4.11728
Adnan, S., Ullah, K., Khan, A. H., and Gao, S. (2017). Meteorological impacts on evapotranspiration in different climatic zones of Pakistan. J. Arid Land 9, 938–952. doi:10.1007/s40333-017-0107-2
Afzal, M. (2018). Impact of climate change on crop adaptation: current challenges and future perspectives.
Ahmad, M. J., Cho, G.-H., Kim, S.-H., Lee, S., Adelodun, B., and Choi, K.-S. (2021). Influence mechanism of climate change over crop growth and water demands for wheat-rice system of Punjab, Pakistan. J. Water Clim. Change 12, 1184–1202. doi:10.2166/wcc.2020.009
Ahmad, M. J., and Choi, K.-S. (2021). Climate-Induced Perspective Variations in Irrigation Schedules and Design Water Requirements for the Rice–Wheat System. Agronomy 11, 2006. doi:10.3390/agronomy11102006
Ahmed, K., Shahid, S., Chung, E.-S., Wang, X.-J., and Harun, S. B. (2019a). Climate change uncertainties in seasonal drought severity-area-frequency curves: case of arid region of Pakistan. J. Hydrology 570, 473–485. doi:10.1016/j.jhydrol.2019.01.019
Ahmed, K., Shahid, S., Nawaz, N., and Khan, N. (2019b). Modeling climate change impacts on precipitation in arid regions of Pakistan: a non-local model output statistics downscaling approach. Theor. Appl. Climatol. 137, 1347–1364. doi:10.1007/s00704-018-2672-5
Ahmed, M., Fayyaz Ul, H., Ahmad, S., Hayat, R., and Raza, M. A. (2020). “Application of Generalized Additive Model for Rainfall Forecasting in Rainfed Pothwar, Pakistan,” in Systems modeling. Editor M. Ahmed (Singapore: Springer Singapore), 403–414.
Ahmed, N., Lü, H., Ahmed, S., Nabi, G., Wajid, M. A., Shakoor, A., et al. (2021). Irrigation Supply and Demand, Land Use/Cover Change and Future Projections of Climate, in Indus Basin Irrigation System, Pakistan. Sustainability 13, 8695. doi:10.3390/su13168695
Ali, S., Khattak, M. S., Khan, D., Sharif, M., Khan, H. Z., and Ullah, A. (2016). Predicting Future Temperature And Precipitation Over Pakistan. 21st Century 35, 61–76.
Allen, R. G., Pereira, L. S., Raes, D., and Smith, M. (1998). Crop evapotranspiration - guidelines for computing crop water requirements - FAO Irrigation and drainage paper 56.
Allen, R. G., Pereira, L. S., Smith, M., Raes, D., and Wright, J. L. (2005). FAO-56. Dual Crop Coefficient Method for Estimating Evaporation from Soil and Application Extensions. J. Irrigation Drainage Eng. 131, 2–13. doi:10.1061/(asce)0733-9437(2005)131:1(2)
Arshad, A., Zhang, Z., Zhang, W., and Gujree, I. (2019). Long-Term Perspective Changes in Crop Irrigation Requirement Caused by Climate and Agriculture Land Use Changes in Rechna Doab, Pakistan. Water 11, 1567. doi:10.3390/w11081567
Athar, H., Nabeel, A., Nadeem, I., and Saeed, F. (2021). Projected changes in the climate of Pakistan using IPCC AR5-based climate models. Theor. Appl. Climatol. 145, 567–584. doi:10.1007/s00704-021-03651-8
Bhima, K. J. (2018). Climate Change Impact on Water Availability and Demand of Irrigation Water - A Review. Int. J. Curr. Microbiol. Appl. Sci. 7, 4349–4360. doi:10.20546/ijcmas.2018.707.507
Bodner, G., Nakhforoosh, A., and Kaul, H.-P. (2015). Management of crop water under drought: a review. Agron. Sustain. Dev. 35, 401–442. doi:10.1007/s13593-015-0283-4
Chand, S., and Ahmad, M. (2020). Appraisal of spatial and temporal behavior in monsoon precipitation series of Punjab-Pakistan using hierarchical Bayesian Models. Environ. Earth Sci. 79, 304. doi:10.1007/s12665-020-09049-5
Cheema, M. J., Immerzeel, W. W., and Bastiaanssen, W. G. (2014). Spatial quantification of groundwater abstraction in the irrigated Indus basin. Ground Water 52, 25–36. doi:10.1111/gwat.12027
Chen, Y., Zhang, Z., and Tao, F. (2018). Impacts of climate change and climate extremes on major crops productivity in China at a global warming of 1.5 and 2.0 °C. Earth Syst. Dyn. 9, 543–562. doi:10.5194/esd-9-543-2018
Dars, G. H., Lashari, B. K., Soomro, M. S., Strong, C., and Ansari, K. (2021). “Pakistan’s Water Resources in the Era of Climate Change,” in Water resources of Pakistan. Editors M. A. Watto, M. Mitchell, and S. Bashir (Cham: Springer International Publishing), 95–108.
Dawadi, S., Mishra, Y., Lamichhane, M., and Tamrakar, J. (2022). Impact of Climate Change on Crop Water Requirement in Kamala River Basin of Nepal. Adv. Eng. Technol. Int. J. 2, 47–58. doi:10.3126/aet.v2i01.50440
Debaeke, P., and Aboudrare, A. (2004). Adaptation of crop management to water-limited environments. Eur. J. Agron. 21, 433–446. doi:10.1016/j.eja.2004.07.006
Deser, C., Lehner, F., Rodgers, K. B., Ault, T., Delworth, T. L., Dinezio, P. N., et al. (2020). Insights from Earth system model initial-condition large ensembles and future prospects. Nat. Clim. Change 10, 277–286. doi:10.1038/s41558-020-0731-2
Droogers, P., and Allen, R. G. (2002). Estimating Reference Evapotranspiration Under Inaccurate Data Conditions. Irrigation Drainage Syst. 16, 33–45. doi:10.1023/a:1015508322413
Du, C., Sun, F., Yu, J., Liu, X., and Chen, Y. (2016). New interpretation of the role of water balance in an extended Budyko hypothesis in arid regions. Hydrology Earth Syst. Sci. 20, 393–409. doi:10.5194/hess-20-393-2016
Ec-Earth-Consortium (2019b). EC-Earth-Consortium EC-Earth3 model output prepared for CMIP6 CMIP. Earth System Grid Federation.
Ec-Earth-Consortium (2019a). EC-Earth-Consortium EC-Earth3-Veg model output prepared for CMIP6 ScenarioMIP. Earth System Grid Federation.
Elbeltagi, A., Kumar, N., Chandel, A., Arshad, A., Pande, C. B., and Islam, A. (2022). Modelling the reference crop evapotranspiration in the Beas-Sutlej basin (India): an artificial neural network approach based on different combinations of meteorological data. Environ. Monit. Assess. 194, 141. doi:10.1007/s10661-022-09812-0
Elliott, J., Deryng, D., Muller, C., Frieler, K., Konzmann, M., Gerten, D., et al. (2014). Constraints and potentials of future irrigation water availability on agricultural production under climate change. Proc. Natl. Acad. Sci. U. S. A. 111, 3239–3244. doi:10.1073/pnas.1222474110
Eyring, V., Bony, S., Meehl, G. A., Senior, C. A., Stevens, B., Stouffer, R. J., et al. (2016). Overview of the Coupled Model Intercomparison Project Phase 6 (CMIP6) experimental design and organization. Geosci. Model Dev. 9, 1937–1958. doi:10.5194/gmd-9-1937-2016
Fao (2018). The future of food and agriculture. Alternative pathways to 2050. Rome: Food and Agriculture Organization of the United Nations.
Feng, Y., Jia, Y., Cui, N., Zhao, L., Li, C., and Gong, D. (2017). Calibration of Hargreaves model for reference evapotranspiration estimation in Sichuan basin of southwest China. Agric. Water Manag. 181, 1–9. doi:10.1016/j.agwat.2016.11.010
Fiedler, S., Stevens, B., Wieners, K.-H., Giorgetta, M., Reick, C., Jungclaus, J., et al. (2019). MPI-M MPIESM1.2-LR model output prepared for CMIP6 RFMIP. Earth System Grid Federation.
Gadiwala, M. S., and Burke, F. (2019). Climate change and precipitation in Pakistan -A meteorological prospect.
Giorgi, F., Im, E. S., Coppola, E., Diffenbaugh, N. S., Gao, X. J., Mariotti, L., et al. (2011). Higher Hydroclimatic Intensity with Global Warming. J. Clim. 24, 5309–5324. doi:10.1175/2011jcli3979.1
Greve, P., Gudmundsson, L., Orlowsky, B., and Seneviratne, S. I. (2015). Introducing a probabilistic Budyko framework. Geophys. Res. Lett. 42, 2261–2269. doi:10.1002/2015gl063449
Greve, P., Gudmundsson, L., Orlowsky, B., and Seneviratne, S. I. (2016). A two-parameter Budyko function to represent conditions under which evapotranspiration exceeds precipitation. Hydrology Earth Syst. Sci. 20, 2195–2205. doi:10.5194/hess-20-2195-2016
Gudmundsson, L., Bremnes, J. B., Haugen, J. E., and Engen-Skaugen, T. (2012). Technical Note: downscaling RCM precipitation to the station scale using statistical transformations – a comparison of methods. Hydrology Earth Syst. Sci. 16, 3383–3390. doi:10.5194/hess-16-3383-2012
Gudmundsson, L., Greve, P., and Seneviratne, S. I. (2016). The sensitivity of water availability to changes in the aridity index and other factors-A probabilistic analysis in the Budyko space. Geophys. Res. Lett. 43, 6985–6994. doi:10.1002/2016gl069763
Gunkel, A., and Lange, J. (2017). Water scarcity, data scarcity and the Budyko curve—An application in the Lower Jordan River Basin. J. Hydrology Regional Stud. 12, 136–149. doi:10.1016/j.ejrh.2017.04.004
Guo, A., Chang, J., Wang, Y., Huang, Q., Guo, Z., and Li, Y. (2019). Uncertainty analysis of water availability assessment through the Budyko framework. J. Hydrology 576, 396–407. doi:10.1016/j.jhydrol.2019.06.033
Haider, K., Khokhar, M. F., Chishtie, F., Razzaqkhan, W., and Hakeem, K. R. (2017). Identification and future description of warming signatures over Pakistan with special emphasis on evolution of CO(2) levels and temperature during the first decade of the twenty-first century. Environ. Sci. Pollut. Res. Int. 24, 7617–7629. doi:10.1007/s11356-016-8359-5
Haider, S., and Ullah, K. (2020). Projected crop water requirement over agro-climatically diversified region of Pakistan.
Han, S., Hu, H., Yang, D., and Liu, Q. (2011). Irrigation impact on annual water balance of the oases in Tarim Basin, Northwest China. Hydrol. Process. 25, 167–174. doi:10.1002/hyp.7830
Hargreaves, H. G., and Samani, A. Z. (1985). Reference Crop Evapotranspiration from Temperature. Appl. Eng. Agric. 1, 96–99. doi:10.13031/2013.26773
Hasan, B. F., and Abed, B. S. (2023). Assessment of Climate Change Impact on Water Productivity and Yield of Wheat Cultivated Using Developed Seasonal Schedule Irrigation in the Nineveh Province. J. Eng. 29, 63–78. doi:10.31026/j.eng.2023.05.05
Hasan, M. U., and Khan, S. (2019). Climate Classification of Pakistan. Int. J. Econ. Environ. Geol. 10, 60–71. doi:10.46660/ojs.v10i2.264
Haseeb, M., Farid, H. U., Khan, Z. M., Anjum, M. N., Ahmad, A., and Mubeen, M. (2023). Quantifying irrigation water demand and supply gap using remote sensing and GIS in Multan, Pakistan. Environ. Monit. Assess. 195, 990. doi:10.1007/s10661-023-11546-6
Hasegawa, T., Fujimori, S., Havlík, P., Valin, H., Bodirsky, B. L., Doelman, J. C., et al. (2018). Risk of increased food insecurity under stringent global climate change mitigation policy. Nat. Clim. Change 8, 699–703. doi:10.1038/s41558-018-0230-x
Heureux, A. M. C., Alvar-Beltrán, J., Manzanas, R., Ali, M., Wahaj, R., Dowlatchahi, M., et al. (2022). Climate Trends and Extremes in the Indus River Basin, Pakistan: implications for Agricultural Production. Atmosphere 13, 378. doi:10.3390/atmos13030378
Hu, Y., Kang, S., Ding, R., Du, T., Tong, L., and Li, S. (2020). The Dynamic Yield Response Factor of Alfalfa Improves the Accuracy of Dual Crop Coefficient Approach under Water and Salt Stress. Water 12, 1224. doi:10.3390/w12051224
Immerzeel, W. W., Lutz, A. F., Andrade, M., Bahl, A., Biemans, H., Bolch, T., et al. (2020).Importance and vulnerability of the world's water towers. Nature 577, 364–369. doi:10.1038/s41586-019-1822-y
Imran, A., Zaman, Q., and Afzal, M. .(2013). Temporal Trends in the Peak Monsoonal Precipitation Events over Northeast Pakistan. Pak. Meteorol. Dep. 10.
IPCC (2021). Climate change 2021 – the physical science basis: working group I contribution to the sixth assessment report of the intergovernmental panel on climate change. Cambridge: Cambridge University Press.
Iqbal, W., Syed, F. S., Sajjad, H., Nikulin, G., Kjellström, E., and Hannachi, A. (2016). Mean climate and representation of jet streams in the CORDEX south asia simulations by the regional climate model RCA4. Theoretical and Applied Climatology.
Ismail, H., Kamal, M. R., Bin Abdullah, A. F., and Bin Mohd, M. S. F. (2020). Climate-Smart Agro-Hydrological Model for a Large Scale Rice Irrigation Scheme in Malaysia. Appl. Sci. 10, 3906. doi:10.3390/app10113906
Janjua, S., Hassan, I., Muhammad, S., Ahmed, S., and Ahmed, A. (2021). Water management in Pakistan's Indus Basin: challenges and opportunities. Water Policy 23, 1329–1343. doi:10.2166/wp.2021.068
Javid, K., Akram, M. a.N., Mumtaz, M., and Siddiqui, R. (2019). Modeling and mapping of climatic classification of Pakistan by using remote sensing climate compound index (2000 to 2018). Appl. Water Sci. 9, 152. doi:10.1007/s13201-019-1028-3
Jones, J. A., Creed, I. F., Hatcher, K. L., Warren, R. J., Adams, M. B., Benson, M. H., et al. (2012). Ecosystem Processes and Human Influences Regulate Streamflow Response to Climate Change at Long-Term Ecological Research Sites. BioScience 62, 390–404. doi:10.1525/bio.2012.62.4.10
Khan, A. U., Shah, A. H., and Iftikhar-Ul-Husnain, M. (2021a). Impact of Climate Change on the Net Revenue of Major Crop Growing Farmers in Pakistan: A Ricardian Approach. Clim. Change Econ. 12. doi:10.1142/s2010007821500068
Khan, M. J., Malik, A. J., Rahman, M., Afzaal, M., Mulk, S. J. I., and Engineering, D. S. (2021b). Assessment of Crop Water Requirement for Various Crops in Peshawar. Pak. Using Crop. Model 10, 1–10.
Khan, N., Shahid, S., Chung, E.-S., Behlil, F., and Darwish, M. S. J. (2020). Spatiotemporal changes in precipitation extremes in the arid province of Pakistan with removal of the influence of natural climate variability. Theor. Appl. Climatol. 142, 1447–1462. doi:10.1007/s00704-020-03389-9
Khan, N., Shahid, S., Ismail, T., Ahmed, K., and Nawaz, N. (2019). Trends in heat wave related indices in Pakistan. Stoch. Environ. Res. Risk Assess. 33, 287–302. doi:10.1007/s00477-018-1605-2
Kijne, J. W. (2001). Lessons learned from the change from supply to demand water management in irrigated agriculture: a case study from Pakistan. Water Policy 3, 109–123. doi:10.1016/s1366-7017(01)00010-1
Kirby, M., Ahmad, M.-U.-D., Mainuddin, M., Khaliq, T., and Cheema, M. J. M. (2017). Agricultural production, water use and food availability in Pakistan: historical trends, and projections to 2050. Agric. Water Manag. 179, 34–46. doi:10.1016/j.agwat.2016.06.001
Kitoh, A., Endo, H., Krishna Kumar, K., Cavalcanti, I. F. A., Goswami, P., and Zhou, T. (2013). Monsoons in a changing world: A regional perspective in a global context. J. Geophys. Res. Atmos. 118, 3053–3065. doi:10.1002/jgrd.50258
Laghari, A. N., Vanham, D., and Rauch, W. (2012). The Indus basin in the framework of current and future water resources management. Hydrology Earth Syst. Sci. 16, 1063–1083. doi:10.5194/hess-16-1063-2012
Langenbrunner, B. (2019). A stronger South Asian monsoon. Nat. Clim. Change 9, 352. doi:10.1038/s41558-019-0472-2
Lei, X., Zhao, J., Wang, D., and Sivapalan, M. (2018). A Budyko-type model for human water consumption. J. Hydrology 567, 212–226. doi:10.1016/j.jhydrol.2018.10.021
Li, X., Ting, M., You, Y., Lee, D. E., Westervelt, D. M., and Ming, Y. (2020). South Asian Summer Monsoon Response to Aerosol-Forced Sea Surface Temperatures. Geophys. Res. Lett. 47. doi:10.1029/2019gl085329
Liu, W., Sun, F., Lim, W. H., Zhang, J., Wang, H., Shiogama, H., et al. (2018). Global drought and severe drought-affected populations in 1.5 and 2°C warmer worlds. Earth Syst. Dyn. 9, 267–283. doi:10.5194/esd-9-267-2018
Liu, W., Yang, H., Folberth, C., Wang, X., Luo, Q., and Schulin, R. (2016). Global investigation of impacts of PET methods on simulating crop-water relations for maize. Agric. For. Meteorology 221, 164–175. doi:10.1016/j.agrformet.2016.02.017
Lund, M. T., Aamaas, B., Stjern, C. W., Klimont, Z., Berntsen, T. K., and Samset, B. H. (2020). A continued role of short-lived climate forcers under the Shared Socioeconomic Pathways. Earth Syst. Dyn. 11, 977–993. doi:10.5194/esd-11-977-2020
Lutz, A. F., Immerzeel, W. W., Kraaijenbrink, P. D., Shrestha, A. B., and Bierkens, M. F. (2016). Climate Change Impacts on the Upper Indus Hydrology: sources, Shifts and Extremes. PLoS One 11, e0165630. doi:10.1371/journal.pone.0165630
Mann, H. B. (1945). Nonparametric Tests Against Trend. Econometrica 13, 245–259. doi:10.2307/1907187
Maraun, D., Shepherd, T. G., Widmann, M., Zappa, G., Walton, D., Gutiérrez, J. M., et al. (2017). Towards process-informed bias correction of climate change simulations. Nat. Clim. Change 7, 764–773. doi:10.1038/nclimate3418
Mehmood, T. (2021). Impact of climate change on agriculture and water resources of Pakistan: A review. Pure Appl. Biol. 10. doi:10.19045/bspab.2021.100017
Munir, M. U., Ahmad, A., Hopmans, J. W., Belgacem, A. O., and Baig, M. B. (2021). “Water Scarcity Threats to National Food Security of Pakistan—Issues, Implications, and Way Forward,” in Emerging challenges to food production and security in asia, Middle East, and africa: climate risks and resource scarcity. Editors M. Behnassi, M. Barjees Baig, M. El Haiba, and M. R. Reed (Cham: Springer International Publishing), 241–266.
Nadeem, F., Jacobs, B., and Cordell, D. (2022). Mapping agricultural vulnerability to impacts of climate events of Punjab, Pakistan. Reg. Environ. Change 22, 66. doi:10.1007/s10113-022-01918-y
Naheed, G., and Mahmood, A. (2009). Water Requirement of Wheat Crop in Pakistan. Pak. J. Meteorology 6 (11).
Naheed, G., and Rasul, G. (2010a). Projections of Crop Water Requirement in Pakistan under Global Warming. Pak. J. Meteorology 7.
Naheed, G., and Rasul, G. (2010b). Recent Water Requirement of Cotton Crop in Pakistan. Pak. J. Meteorology 6.
Nasim, W., Amin, A., Fahad, S., Awais, M., Khan, N., Mubeen, M., et al. (2018). Future risk assessment by estimating historical heat wave trends with projected heat accumulation using SimCLIM climate model in Pakistan. Atmos. Res. 205, 118–133. doi:10.1016/j.atmosres.2018.01.009
Nazarenko, L. S., Tausnev, N., Russell, G. L., Rind, D., Miller, R. L., Schmidt, G. A., et al. (2022). Future Climate Change Under SSP Emission Scenarios With GISS-E2.1. J. Adv. Model. Earth Syst. 14. doi:10.1029/2021ms002871
Nusrat, A., Gabriel, H. F., Haider, S., Ahmad, S., Shahid, M., and Ahmed Jamal, S. (2020). Application of Machine Learning Techniques to Delineate Homogeneous Climate Zones in River Basins of Pakistan for Hydro-Climatic Change Impact Studies. Appl. Sci. 10, 6878. doi:10.3390/app10196878
Nusrat, A., Gabriel, H. F., E Habiba, U., Rehman, H. U., Haider, S., Ahmad, S., et al. (2022). Plausible Precipitation Trends over the Large River Basins of Pakistan in Twenty First Century. Atmosphere 13, 190. doi:10.3390/atmos13020190
Padrón, R. S., Gudmundsson, L., Greve, P., and Seneviratne, S. I. (2017). Large-Scale Controls of the Surface Water Balance Over Land: insights From a Systematic Review and Meta-Analysis. Water Resour. Res. 53, 9659–9678. doi:10.1002/2017wr021215
Pearson, K. (1900). X. On the criterion that a given system of deviations from the probable in the case of a correlated system of variables is such that it can be reasonably supposed to have arisen from random sampling. Lond. Edinb. Dublin Philosophical Mag. J. Sci. 50, 157–175. doi:10.1080/14786440009463897
Qureshi, A. S. (2015). Improving food security and livelihood resilience through groundwater management in Pakistan.
Qureshi, A. S. (2011). Water Management in the Indus Basin in Pakistan: challenges and Opportunities. Mt. Res. Dev. 31, 252–260. doi:10.1659/mrd-journal-d-11-00019.1
Rafiqul Islam, M. (2022). Impact of climate-induced extreme events and demand–supply gap on water resources in Bangladesh. J. Water Clim. Change 13, 1878–1899. doi:10.2166/wcc.2022.421
Rasul, G., and Farooqi, A. (1993). Water requirement of cotton crop in Pakistan. Pak. Meteorol. Dep. 4, 154–165.
Rasul, G., and Sharma, B. (2016). The nexus approach to water–energy–food security: an option for adaptation to climate change. Clim. Policy 16, 682–702. doi:10.1080/14693062.2015.1029865
Ruan, H., Yu, J., Wang, P., and Wang, T. (2020). Increased crop water requirements have exacerbated water stress in the arid transboundary rivers of Central Asia. Sci. Total Environ. 713, 136585. doi:10.1016/j.scitotenv.2020.136585
Schewe, J., Heinke, J., Gerten, D., Haddeland, I., Arnell, N. W., Clark, D. B., et al. (2014). Multimodel assessment of water scarcity under climate change. Proc. Natl. Acad. Sci. U. S. A. 111, 3245–3250. doi:10.1073/pnas.1222460110
Seland, Ø., Bentsen, M., Olivié, D., Toniazzo, T., Gjermundsen, A., Graff, L. S., et al. (2020). Overview of the Norwegian Earth System Model (NorESM2) and key climate response of CMIP6 DECK, historical, and scenario simulations. Geosci. Model Dev. 13, 6165–6200. doi:10.5194/gmd-13-6165-2020
Sen, P. K. (1968). Estimates of the Regression Coefficient Based on Kendall's Tau. J. Am. Stat. Assoc. 63, 1379–1389. doi:10.1080/01621459.1968.10480934
Senbeta, T. B., Karamuz, E., Kochanek, K., Napiórkowski, J. J., and Romanowicz, R. J. (2023). Budyko-based approach for modelling water balance dynamics considering environmental change drivers in the Vistula River basin, Poland. Hydrological Sci. J. 68, 655–669. doi:10.1080/02626667.2023.2187297
Shafeeque, M., Hafeez, M., Sarwar, A., Arshad, A., Khurshid, T., Asim, M. I., et al. (2023). Quantifying future water-saving potential under climate change and groundwater recharge scenarios in Lower Chenab Canal, Indus River Basin. Theor. Appl. Climatol. doi:10.1007/s00704-023-04621-y
Shafeeque, M., Luo, Y., Arshad, A., Muhammad, S., Ashraf, M., and Pham, Q. B. (2022a). Assessment of climate change impacts on glacio-hydrological processes and their variations within critical zone. Nat. Hazards 115, 2721–2748. doi:10.1007/s11069-022-05661-9
Shafeeque, M., Luo, Y., and He, H. (2022b). Involving Turc-Budyko formula in evaluating gridded precipitation datasets in glaciated catchments. J. Hydrology 614, 128482. doi:10.1016/j.jhydrol.2022.128482
Singh, R., and Kumar, R. (2015). Vulnerability of water availability in India due to climate change: A bottom-up probabilistic Budyko analysis. Geophys. Res. Lett. 42, 9799–9807. doi:10.1002/2015gl066363
Siyal, A. W., Gerbens-Leenes, W., Aldaya, M. M., and Naz, R. (2023). The importance of irrigation supply chains within the water footprint: an example from the Pakistani part of the Indus basin. J. Integr. Environ. Sci. 20, 2208644. doi:10.1080/1943815x.2023.2208644
Srivastava, A., Sahoo, B., Raghuwanshi, N. S., and Chatterjee, C. (2018). Modelling the dynamics of evapotranspiration using Variable Infiltration Capacity model and regionally calibrated Hargreaves approach. Irrigation Sci. 36, 289–300. doi:10.1007/s00271-018-0583-y
Steduto, P., Hsiao, T. C., Fereres, E., and Raes, D. (2012). Crop yield response to water. FAO Irrigation and drainage paper 66. Rome, Italy: Food and Agriculture Organization of the United Nations.
Tukey, J. W. (1949). Comparing Individual Means in the Analysis of Variance. Biometrics 5, 99–114. doi:10.2307/3001913
Tuladhar, S., Hussain, A., Baig, S., Ali, A., Soheb, M., Angchuk, T., et al. (2023). Climate Change, Water and Agriculture Linkages in the Upper Indus Basin: A Field Study From Gilgit-Baltistan and Leh-Ladakh. Front. Sustain. Food Syst. 6. doi:10.3389/fsufs.2022.1012363
Volodin, E., Mortikov, E., Gritsun, A., Lykossov, V., Galin, V., Diansky, N., et al. (2019b). INM INM-CM5-0 model output prepared for CMIP6 CMIP piControl. Earth System Grid Federation.
Volodin, E., Mortikov, E., Gritsun, A., Lykossov, V., Galin, V., Diansky, N., et al. (2019a). INM INM-CM4-8 model output prepared for CMIP6 CMIP piControl. Earth System Grid Federation.
Von Storch, J.-S., Putrasahan, D., Lohmann, K., Gutjahr, O., Jungclaus, J., Bittner, M., et al. (2017). MPI-M MPIESM1.2-HR model output prepared for CMIP6 HighResMIP. Earth System Grid Federation.
Wang, D., and Hejazi, M. (2011). Quantifying the relative contribution of the climate and direct human impacts on mean annual streamflow in the contiguous United States. Water Resour. Res. 47. doi:10.1029/2010wr010283
Wang, W., and Fu, J. (2018). Global assessment of predictability of water availability: A bivariate probabilistic Budyko analysis. J. Hydrology 557, 643–650. doi:10.1016/j.jhydrol.2017.12.068
Waseem, M., Ahmad, I., Mujtaba, A., Tayyab, M., Si, C., Lü, H., et al. (2020). Spatiotemporal Dynamics of Precipitation in Southwest Arid-Agriculture Zones of Pakistan. Sustainability 12, 2305. doi:10.3390/su12062305
Xavier, L. C. P., Silva, S. M. O. D., Carvalho, T. M. N., Pontes Filho, J. D., and Souza Filho, F. D. a.D. (2020). Use of Machine Learning in Evaluation of Drought Perception in Irrigated Agriculture: the Case of an Irrigated Perimeter in Brazil. Water 12, 1546. doi:10.3390/w12061546
Xin, X., Zhang, J., Zhang, F., Wu, T., Shi, X., Li, J., et al. (2018). BCC BCC-CSM2MR model output prepared for CMIP6 CMIP. Earth System Grid Federation.
Xing, Z., Ma, M., Wei, Y., Zhang, X., Yu, Z., and Yi, P. (2020). A new agricultural drought index considering the irrigation water demand and water supply availability. Nat. Hazards 104, 2409–2429. doi:10.1007/s11069-020-04278-0
Xu, X., Liu, W., Scanlon, B. R., Zhang, L., and Pan, M. (2013). Local and global factors controlling water-energy balances within the Budyko framework. Geophys. Res. Lett. 40, 6123–6129. doi:10.1002/2013gl058324
Yi, L. J. R. S. (2010). Evaluation and improvement of the FAO-56 soil water stress coefficient approach using field experimental data.
Yu, W., Yang, Y.-C., Savitsky, A., Alford, D., Brown, C., Wescoat, J., et al. (2013). The Indus Basin of Pakistan. The World Bank.
Yukimoto, S., Koshiro, T., Kawai, H., Oshima, N., Yoshida, K., Urakawa, S., et al. (2019). MRI MRI-ESM2.0 model output prepared for CMIP6 CMIP. Earth System Grid Federation.
Zhang, Y., Wu, Z., Singh, V. P., Su, Q., He, H., Yin, H., et al. (2021). Evolving CO2 Rather Than SST Leads to a Factor of Ten Decrease in GCM Convergence Time. J. Adv. Model. Earth Syst. 13, e2021MS002505. doi:10.1029/2021MS002505
Zhou, T., Lu, J., Zhang, W., and Chen, Z. (2020). The Sources of Uncertainty in the Projection of Global Land Monsoon Precipitation. Geophys. Res. Lett. 47. doi:10.1029/2020gl088415
Zhu, T., Ringler, C., Iqbal, M. M., Sulser, T. B., and Goheer, M. A. (2013). Climate change impacts and adaptation options for water and food in Pakistan: scenario analysis using an integrated global water and food projections model. Water Int. 38, 651–669. doi:10.1080/02508060.2013.830682
Keywords: climate change, crop water requirements, agricultural water demand-supply, Budyko hydrological model, climate-crop model, CMIP6
Citation: Shafeeque M and Bibi A (2023) Assessing the impact of future climate scenarios on crop water requirements and agricultural water supply across different climatic zones of Pakistan. Front. Earth Sci. 11:1283171. doi: 10.3389/feart.2023.1283171
Received: 25 August 2023; Accepted: 28 September 2023;
Published: 10 October 2023.
Edited by:
Muhammad Saifullah, Muhammad Nawaz Shareef University of Agriculture Multan, PakistanReviewed by:
Arfan Arshad, Oklahoma State University, United StatesAbid Sarwar, University of Agriculture, Faisalabad, Pakistan
Copyright © 2023 Shafeeque and Bibi. This is an open-access article distributed under the terms of the Creative Commons Attribution License (CC BY). The use, distribution or reproduction in other forums is permitted, provided the original author(s) and the copyright owner(s) are credited and that the original publication in this journal is cited, in accordance with accepted academic practice. No use, distribution or reproduction is permitted which does not comply with these terms.
*Correspondence: Muhammad Shafeeque, shafeequ@uni-bremen.de