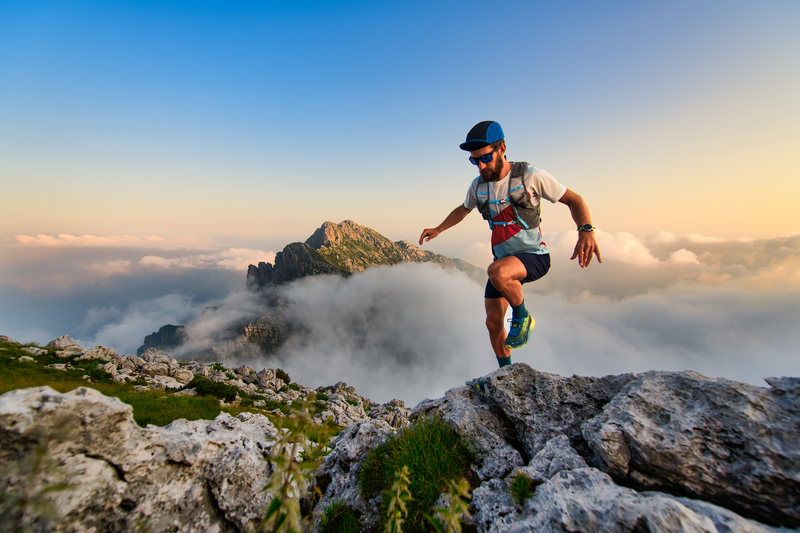
95% of researchers rate our articles as excellent or good
Learn more about the work of our research integrity team to safeguard the quality of each article we publish.
Find out more
EDITORIAL article
Front. Earth Sci. , 18 August 2023
Sec. Cryospheric Sciences
Volume 11 - 2023 | https://doi.org/10.3389/feart.2023.1266810
This article is part of the Research Topic Pan-Arctic Snow Research View all 8 articles
Editorial on the Research Topic
Pan-Arctic snow research
Snowpacks are a key component of the Earth’s environment and play a fundamental role in regulating both the Earth’s energy budget and the Arctic hydrological cycle (Bolibar et al., 2022). Polar snowpacks constitute the largest interface between the atmosphere and the Earth’s surface during winter (Bennett et al., 2022) and cover between 40% and 50% of the land surface during winter in the northern hemisphere (excluding the snow over sea ice). Although the seasonal snow cover represents a crucial component of the cryosphere (van Pelt et al., 2019), it is also the most variable and short-lived. Snow deposited on land or ice surfaces is thermodynamically unstable and is in constant change due to snow metamorphism, which is mainly controlled by temperature gradients (Pinzer and Schneebeli, 2009; Gallet et al., 2018; Domine et al., 2019). Snowpack affects the hydrological cycle by acting as a water reservoir during the wintertime while it contributes to the replenishment of water tables and rivers during the spring and summer melt periods (Bennett et al., 2022). The annual snowpacks are also critical for the mass balance of glaciers, providing them with water mass input (in the solid form) required for their growth, or at least helping to decelerate the speed at which they are disappearing by protecting the ice surface from solar radiation and melting (Bolibar et al., 2022). Snowpacks are not only reservoirs, but they are also ecosystems too, harboring a variety of microorganisms, such as prokaryotes, fungi, algae, protists, and viruses, that vary in abundance, physiological characteristics, community composition and diversity depending on both season and location (Maccario et al., 2015). These snow microbial communities are not only active at temperatures below 0°C, but also dynamic and interact with their chemical environment (Maccario et al., 2015). Therefore, the biotic compartment of snow in the Arctic has a crucial role in the production, degradation, and transformation of contaminants and nutrients and is a key player in biogeochemical cycles.
The main Research Topic of this Research Topic was to collect research proposing new and improved methodologies and techniques for physical, chemical, and biological snowpack observations. Since the snowpack is the most dynamic portion of the cryosphere, understanding and monitoring its evolution through space and time has become critical to disentangle the interplay of processes affecting the rapidly changing Arctic region.
The widespread coverage of the seasonal snow cover in the Northern Hemisphere and specifically in the Arctic region during the winter, make the study of its spatial evolution and dynamics difficult, largely due to the scattered nature of measurements and their location generally around accessible places nearby towns or research infrastructure. These measurements provide local information but do not always give the spatial and temporal resolution needed to understand how snow cover dynamics, timing, spatial variability, and properties change and evolve over the season and over larger spatial scales. To overcome this, new techniques for snowpack measurements emerged in the last decade and are presented in the papers by Vickers et al.; Messerli et al.
The paper by Vickers et al. proposed a method based on the Synthetic Aperture Radar based method for long term monitoring of seasonal snowmelt and wintertime Rain-On-Snow (ROS) events in Svalbard. The occurrence of wintertime ROS events is expected to increase in frequency and intensity across the Arctic because of climate change. ROS events can dramatically alter snow cover characteristics, by saturating the snowpack, changing its density profile, and enhancing surface runoff, as well as causing the widespread formation of basal ice and preferential flow paths, which impact ecosystems as well as infrastructure. Their approach is based on the usage of the C-band Synthetic Aperture Radar (SAR) observations to produce a 17-year dataset (2004–2020) about snow melting for Svalbard, from where a method for detecting and mapping both spring melt onset and ROS frequency has been developed. Thanks to the development of the above-mentioned methods, Vickers et al. found a general asymmetry in the timing of spring melt onset across the archipelago, with the earliest dates of onset taking place in the south and west of Svalbard and the latest melt onset in the north and east. Satellite measurements are enabling a new generation of techniques for monitoring the spatial evolution of the snowpack through time in locations where field measurements are rare.
Another remote technique that is now used to estimate the evolution of the snowpack is the terrestrial time-lapse photogrammetry. The use of this approach is limited in space compared to satellites but can produce a much higher resolution dataset of snow cover. In the original research submitted to this Research Topic, Messerli et al. proposed an innovative study for evaluating the snow cover evolution at Qasigiannguit Glacier, southwest Greenland using a comparison of time-lapse imagery and mass balance data. Using a manual snowline extraction method coupled with terrestrial time-lapse photogrammetry, they determine the snowlines at daily to sub-weekly timescales on the Qasigiannguit glacier in Kobbefjord, Nuuk. The dataset was used to produce a time series of maximum snowline elevations using a high-resolution Digital Elevation Model (DEM) and the extent of snow and bare ice during the 2020–2021 melt seasons. The measurements were validated using concurrent automatic weather station (AWS) and surface mass balance measurements and, the result obtained, supports a good agreement between the snowline elevation from the time-lapse data and the stake mass balance data. The study of Messerli et al. underlines the necessity for testing and developing new measurement techniques of snow cover and its properties that integrate field measurements from operators and automatic measuring stations. This is important since remote measurement techniques have limitations; some of the main snowpack parameters are difficult to retrieve using such approaches, for example, snow depth and snow water equivalent (SWE).
Automatic measuring stations and, more specifically, automated nivometric stations (ANS) can be defined as devices able to host different instrumentation and sensors for continuous measurements of the snowpack. The ANS can produce long-term continuous records of snowpack physical properties and can be equipped, for example, with cameras to measure the fractional snow-cover area (fSCA) using NIR (Near infrared) images, snow depth (SD), internal snow temperature and liquid water content (LWC) profiles at different depths with a 10 min time resolution and snow water equivalent with high precision. The ANS presented in Scoto et al. describes in detail the advantage of the usage of this device, the installation set-up, and the future possible implementation to extend the range of instruments\sensors installed on the station and hence enhance the possible snowpack continuous observation. The data from the ANS are useful to produce a long-term monitoring of the snowpack conditions and provide background data to validate snow physical models and satellite measurements. A limitation of these types of automatic measuring stations is the energy supply required to run them making their use usually limited to areas near research stations. The first attempt to overcome this Research Topic was presented in Filhol et al. where they proposed a new approach to meteorological observations on remote polar glaciers. Thanks to the availability of Internet-of-Things (IoT) technologies and low-cost electronics, they were able to assemble a snowpack monitoring system collecting spatially distributed, in situ data with real-time connectivity to online servers for immediate and long-term usage at costs comparable to those of a single autonomous weather station. The snowpack monitoring system integrates commercial and custom-built sensors connected to a node (main device) that manages power, data, and radio communication. Data is automatically sent to a server enabling a controlled and systematic handling and storage procedure. The proposed system collected reliable data and had sufficient power resources to survive 4–5 months of darkness during the polar night, partially overcoming the challenges to retrieve continuous snowpack data all year round from remote areas accessible only in specific seasons.
Automatic nivometric stations can be assembled for continuous measurement of snowpack properties. However, as underlined by Scoto et al.; Filhol et al. and in the introduction of this editorial, attention must be given to the history of the meteorological conditions in the selected study areas. Snowpack evolution is dependent on the meteorological conditions such as temperature, wind, relative humidity, and precipitation and on the specific weather events occurring at the study site. The solid precipitation amount is fundamental to building the snowpack and it is a fundamental parameter to be considered for studying the snowpack evolution during the Arctic winter season. During the winter period, the Arctic region is experiencing more frequent liquid precipitation (rain) that has a strong impact on the snowpack and, in general, on the whole Arctic ecosystem. A specific case study has been considered by Schot et al. where they analyze the precipitation trends (1958–2021) of Ammassalik island, south-east Greenland. To assess past changes in air temperature and precipitation, output from a regional polar climate model (RACMO2.3p2) was evaluated together with measurements from automatic weather stations in Tasiilaq and on Mittivakkat glacier. In addition, RACMO2.3p2 was used to assess past seasonal changes in air temperature, precipitation amount, precipitation phase and the altitude of the rain/snow boundary. The proposed approach contributed to an increased understanding of precipitation and temperature changes on Ammassalik island and suggests that air temperature has been increasing on Ammassalik island since 1958 with a trend that becomes more pronounced in the 1990–2019 subperiod. The total precipitation (solid and liquid) did not increase significantly for the investigated periods however, due to trends in precipitation as well as air temperature, the liquid to total precipitation ratio on Ammassalik island is increasing in the summer. Schot et al. suggested that higher elevated regions on the Ammassalik island have recently received more liquid precipitation combined with an upward migration of the rain-to-snow boundary altitude. A monthly increase in the altitude of the rain-to-snow boundary of +25, +23, +20 m/decade in July, August, and September respectively has been calculated.
The type and amount of precipitation constitute fundamental controls on snowpack evolution through the winter season and have the capacity to transfer impurities from the atmosphere into the snowpack (Spolaor et al., 2021). Fresh snow contains impurities that can accumulate and be preserved over time in the snowpack (Barbaro et al., 2021). The presence and abundance of elements, ionic compounds, organic compounds (natural and anthropogenic) black carbon and dust in the snowpack can be studied to understand specific transport events from the mid-latitude to the Arctic and evaluate the presence of possible contamination. There are multiple studies investigating the chemical content of the annual snowpack in different regions of the Arctic, providing important information on atmospheric processes, transport events, forest fire frequency, and local versus long range transport, but an emerging question that is still under investigation is the microbiological composition of the snowpack (Keuschnig et al., 2023). Much of the recent work on measuring the impact of microbial communities in the cryosphere is based on DNA and RNA analyses. However, the preservation of nucleic acids for high-throughput sequencing is an ongoing challenge for field scientists. The DNA molecules can be extracted from samples to evaluate both the composition and functional potential of communities, while the RNA, which is a less stable molecule, can be used to infer which members of the community are active at the time of sampling and which genes are being transcribed. Many different preservation techniques have been proposed to extract these molecules and in Trivedi et al. several of these were compared to assess their effectiveness on low biomass samples collected in Iceland. Snow and ice samples were melted and filtered upon Research Topic, and filters were preserved using: 1) liquid nitrogen flash freezing, 2) storage in RNAlater (a commercially available chemical stabilization reagent), or 3) storage in Zymo DNA/RNA Shield. Comparative statistics covering nucleic acid recovery, sequencing library preparation, genome assembly, and taxonomic diversity were used to determine best practices for the preservation of DNA and RNA samples from these environments. The results revealed that microbial community composition based on DNA was comparable across preservation types. However, when considering extracted RNA, the taxonomic composition of the active community was primarily driven by the filtered sample volume (i.e., biomass content). Overall, all three methods provided comparable results both for DNA and RNA, but the main factor to consider is collecting a sufficiently high quantity of biomass from snow and ice samples to environmentally relevant microbial community data.
Pigmented algae inhabiting glacier and ice sheet surfaces are part of the light absorbing particles present on snow and ice surfaces. Such particulates have been shown to decrease surface albedo and increase surface melt rates of both snow and bare ice surfaces. The study of Winkel et al. focused on the Seasonality of Glacial Snow and Ice Microbial Communities by assessing the microbial community composition and nutrient variations of glacier surface ice and snow habitats on various Icelandic glaciers using Illumina sequencing of the 16S rRNA, 18S rRNA, and ITS marker genes and correlating them with geochemical signals in the snow and ice. Winkel et al. show a clear shift in the microbial community structure and corresponding geochemical environmental characteristics from the summer to the winter season. Summer periods were characterized by a higher availability of microbially derived DOC and low nutrient concentrations, whereas winter was characterized by lower DOC and high nutrient concentrations. The summer eukaryotic algae inhabiting the glacier surface ice and snow were distinct and were closely associated with a diverse community of heterotrophic bacteria likely relying on algal-delivered DOC. Winter microbial communities contained bacteria resembling communities typically found in air and snow and lacked eukaryotic algae. The results from this study highlight the potential role of microorganisms in biogeochemical cycling as well as the impact of seasonality on these processes.
Different approaches, based on in situ and/or remote observations, can contribute to the description of the snow seasonality in Arctic areas. Snowpack observations performed directly in the field by operators or ANS are the most reliable, however, this type of data can only be collected from specific accessible regions and periods throughout the winter season. Should be noted that the technological improvement proposed by Filhol et al. is partially minimizing this problem; yet the ambition of covering the entire Arctic with ground ANS remains a major challenge. However, automatic acquisition systems (both the automatic weather station and the automated nivometric station) are essential for producing long-term datasets that can be used to establish in which way and how fast the Arctic is changing in recent decades as well as test the accuracy of the climate and meteorological models. The remote sensing approach can overcome the limitation of pointed field measurements with low spatial representativity and, by validating satellite and terrestrial time-lapse photogrammetry with ground measurements, it is possible to widen and expand the area where the snowpack is observed and studied and explore the consequence of specific meteorological events (such as the Rain-on-snow) on the snowpack properties. In addition, the impurities present in the snow contribute to the snowpack’s ability to absorb light and modify its physical characteristics. The presence of microorganisms in the snowpack is still a little-studied Research Topic, but studies that are coming out suggest a non-negligible role of the microbiological community in the evolution and biogeochemistry of snowpacks, highlighting the need for interdisciplinary studies over longer time scales.
The advances in snowpack monitoring shown in the research papers presented in this Research Topic demonstrate the attention that the Research Topic has been receiving in the past decade. From this article Research Topic it has become apparent that there is an increasing attention to improve and develop automatic measuring system, data logging, and telemetry (IoT) for real-time monitoring of snowpack properties and glacier mass balance dynamics. Similarly, the focus on modeling is likely to continue, particularly on the development of more automated community data collection-modeling to help increase model forecast capabilities regarding snowpack dynamics, glacier evolution, and impacts of climate change.
AS: Writing–original draft, Writing–review and editing. CL: Writing–original draft, Writing–review and editing. BL: Writing–original draft, Writing–review and editing. J-CG: Writing–original draft, Writing–review and editing. RS: Writing–original draft, Writing–review and editing. VP: Writing–original draft, Writing–review and editing. DC: Writing–original draft, Writing–review and editing.
The authors declare that the research was conducted in the absence of any commercial or financial relationships that could be construed as a potential conflict of interest.
The authors declared that they were an editorial board member of Frontiers, at the time of submission. This had no impact on the peer review process and the final decision.
All claims expressed in this article are solely those of the authors and do not necessarily represent those of their affiliated organizations, or those of the publisher, the editors and the reviewers. Any product that may be evaluated in this article, or claim that may be made by its manufacturer, is not guaranteed or endorsed by the publisher.
Barbaro, E., Koziol, K., Björkman, M. P., Vega, C. P., Zdanowicz, C., Martma, T., et al. (2021). Measurement report: spatial variations in ionic chemistry and water-stable isotopes in the snowpack on glaciers across svalbard during the 2015–2016 snow accumulation season. Atmos. Chem. Phys. 21, 3163–3180. doi:10.5194/acp-21-3163-2021
Bennett, K. E., Miller, G., Busey, R., Chen, M., Lathrop, E. R., Dann, J. B., et al. (2022). Spatial patterns of snow distribution in the sub-Arctic. Cryosphere 16, 3269–3293. doi:10.5194/tc-16-3269-2022
Bolibar, J., Rabatel, A., Gouttevin, I., Zekollari, H., and Galiez, C. (2022). Nonlinear sensitivity of glacier mass balance to future climate change unveiled by deep learning. Nat. Commun. 13, 409. doi:10.1038/s41467-022-28033-0
Domine, F., Picard, G., Morin, S., Barrere, M., Madore, J.-B., and Langlois, A. (2019). Major issues in simulating some arctic snowpack properties using current detailed snow physics models: consequences for the thermal regime and water budget of permafrost. J. Adv. Model Earth Syst. 11, 34–44. doi:10.1029/2018MS001445
Gallet, J. C., Bjorkman, M. P., Larose, C., Luks, B., Martma, T., and Zdanowicz, C. (2018). Protocols and recommendations for the measurement of snow physical properties, and sampling of snow for black carbon, water isotopes, major ions and microorganisms. Norwegian Polar Institute 46. Available at: https://www.polarknow.us.edu.pl/wp-content/uploads/Kortrapport46.pdf.
Keuschnig, C., Vogel, T. M., Barbaro, E., Spolaor, A., Koziol, K., Björkman, M. P., et al. (2023). Selection processes of Arctic seasonal glacier snowpack bacterial communities. Microbiome 11, 35. doi:10.1186/s40168-023-01473-6
Maccario, L., Sanguino, L., Vogel, T. M., and Larose, C. (2015). Snow and ice ecosystems: not so extreme. Res. Microbiol. 166, 782–795. doi:10.1016/j.resmic.2015.09.002
Pinzer, B. R., and Schneebeli, M. (2009). Snow metamorphism under alternating temperature gradients: morphology and recrystallization in surface snow. Geophys Res. Lett. 36, L23503. doi:10.1029/2009GL039618
Spolaor, A., Moroni, B., Luks, B., Nawrot, A., Roman, M., Larose, C., et al. (2021). Investigation on the sources and impact of trace elements in the annual snowpack and the firn in the hansbreen (southwest spitsbergen). Front. Earth Sci. (Lausanne) 8, 664. doi:10.3389/feart.2020.536036
Keywords: Arctic, snow, measurement techniques, proprieties, composition
Citation: Spolaor A, Larose C, Luks B, Gallet J-C, Salzano R, Pohjola VA and Costa D (2023) Editorial: Pan-Arctic snow research. Front. Earth Sci. 11:1266810. doi: 10.3389/feart.2023.1266810
Received: 25 July 2023; Accepted: 07 August 2023;
Published: 18 August 2023.
Edited and reviewed by:
Michael Lehning, Swiss Federal Institute of Technology Lausanne, SwitzerlandCopyright © 2023 Spolaor, Larose, Luks, Gallet, Salzano, Pohjola and Costa. This is an open-access article distributed under the terms of the Creative Commons Attribution License (CC BY). The use, distribution or reproduction in other forums is permitted, provided the original author(s) and the copyright owner(s) are credited and that the original publication in this journal is cited, in accordance with accepted academic practice. No use, distribution or reproduction is permitted which does not comply with these terms.
*Correspondence: Andrea Spolaor, YW5kcmVhLnNwb2xhb3JAdW5pdmUuaXQ=
Disclaimer: All claims expressed in this article are solely those of the authors and do not necessarily represent those of their affiliated organizations, or those of the publisher, the editors and the reviewers. Any product that may be evaluated in this article or claim that may be made by its manufacturer is not guaranteed or endorsed by the publisher.
Research integrity at Frontiers
Learn more about the work of our research integrity team to safeguard the quality of each article we publish.