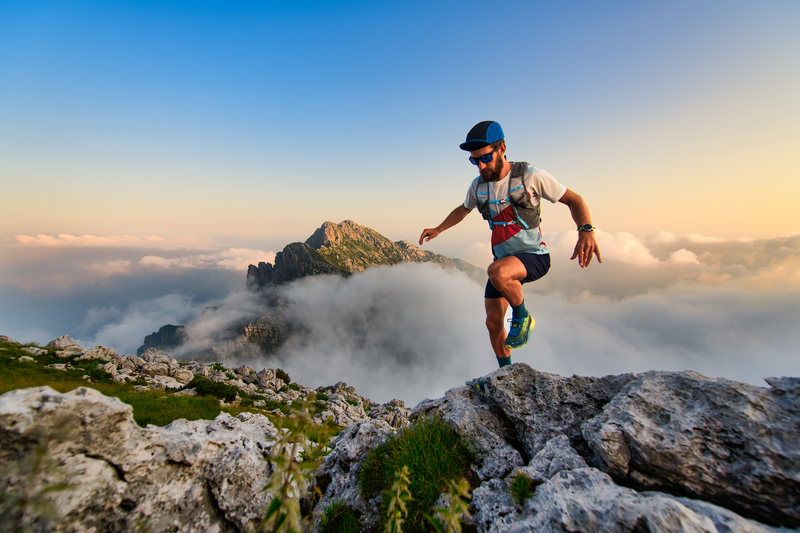
94% of researchers rate our articles as excellent or good
Learn more about the work of our research integrity team to safeguard the quality of each article we publish.
Find out more
ORIGINAL RESEARCH article
Front. Earth Sci. , 11 September 2023
Sec. Interdisciplinary Climate Studies
Volume 11 - 2023 | https://doi.org/10.3389/feart.2023.1256296
This article is part of the Research Topic Energy, Economy, and Climate Interactions: Challenges and Opportunities, volume II View all 13 articles
The relationship between the high-frequency time series of PM2.5 in the atmosphere and the air pollutants emitted by industrial firms is not yet fully understood. This study aimed to identify independent PM2.5 clustering regions in Shaanxi Province and to evaluate the spatio-temporal correlations of PM2.5 concentrations and pollutant emissions from industrial firms in these regions. To accomplish this, daily data on PM2.5 concentrations and air pollutants emitted by industrial firms were analyzed using the K-means spatial clustering method and cross-wavelet transformation. The results show that: 1) PM2.5 concentrations in Shaanxi Province can be divided into three independent clustering regions. 2) The lagged impact of industrial emissions on PM2.5 concentrations were about 1/4-1/2 period. 3) PM2.5 was mainly influenced by particulate matter (PM) emissions from industrial plants during the period of 16–32 days, while nitrogen oxides (NOx) significantly affected PM2.5 concentrations during the period of 32–64 days. 4) Emissions of PM, NOx, and sulfur dioxide (SO2) more significantly affect PM2.5 concentrations in northern and central Shaanxi, and pollutants emitted by firms in the thermal power generation, utility, and steel industries had more significant effects on PM2.5 concentrations than those emitted by the cement manufacturing and electric power industries. During the COVID-19 shutdown, the emissions of firms cannot significantly affect PM2.5 concentrations. These findings suggest that emission reduction initiatives should consider industrial, regional, and periodic differences to reduce PM2.5 pollution during winter.
Exposure to high level of fine particulate matter (PM2.5) in the atmosphere has been associated with severe adverse effects on human health (Barwick et al., 2017; Zhong et al., 2017; Chen et al., 2018; Liu and Salvo, 2018; Cheung et al., 2020; Fan et al., 2020; He et al., 2020), as well as substantial economic losses (Zhang and Mu, 2018; Sun et al., 2019; Chen et al., 2022; Ito and Zhang, 2020). While efforts have been made to manage PM2.5 concentrations in developing countries like China, with notable progress in recent decades (Greenstone et al., 2021), the annual average PM2.5 concentrations in China’s 339 cities are still expected to exceed the recommended level by the World Health Organization (MEE, 2022; WHO, 2021). Moreover, severe haze pollution remains a common occurrence in many Chinese cities during autumn and winter (Ma et al., 2020; Zhang et al., 2020b).
To effectively reduce ambient PM2.5 concentrations, it is crucial to identify the firms that should reduce emissions during periods of heavy pollution as well as during normal times (F. Wang et al., 2019; Zhao et al., 2018; Alari et al., 2021; Frankowski, 2020; Rivera, 2021), for the industrial sector significantly contributes to China’s economic growth but also emits a large amount of air pollutants while consuming fossil energy (Choi et al., 2021; MEE, 2020; Zhang et al., 2014). Various studies have analyzed the correlation between PM2.5 concentrations and yearly air pollutant emissions using a physicochemical model (Choi et al., 2021; Lu et al., 2021; Wang et al., 2022; Liu et al., 2018b; Zhang et al., 2014; Zhang et al., 2018), likely multiple regression (Zhang et al., 2020), geographically weighted regression model (Wang et al., 2018; Tu et al., 2019) and physicochemical model such as CMAQ (Wang et al., 2022b; Wang et al., 2019b), WRF-Chem (Spiridonov et al., 2019; Azmi et al., 2022; Zhang et al., 2023). Although an increasing body of research begins to concentrate on the interplay between business emissions and air quality (Li et al., 2016; Wu et al., 2023), there has been limited research conducted on the relationship between high-frequency scale (such as a week, day, or even an hour) air pollutant emissions from industrial firms and PM2.5 concentrations, which can help the government formulate proper strategies for reducing industrial emissions.
In order to better capture the high-frequency variability of PM2.5, some researchers have employed the wavelet analysis technique to identify the temporal patterns of PM2.5 changes (Chen et al., 2020; Kapwata et al., 2021; Li et al., 2017; Shi et al., 2014; Sun et al., 2017; Zhao et al., 2009; Zhao et al., 2016). Wavelet analysis has been shown to be effective in studying the periodicity and evolution characteristics of PM2.5 concentrations, as well as investigating the influence of natural factors on PM2.5 concentrations. Moreover, PM2.5 pollution exhibit significant regional variations that often do not align with administrative divisions (Tie et al., 2005; Tie and Cao, 2009; Wang et al., 2011; Zhao et al., 2020), the PM2.5 pollution areas must be identify before appropriate mitigation strategies can be established.
To fill the knowledge gap mentioned above, this study selects Shaanxi Province in China as a relatively independent study area to examine the correlation between firms’ emissions and PM2.5. For the unique topography Guanzhong Plain is one of China’s most polluted region for PM2.5 (Xu et al., 2018; Li et al., 2022); Southern Shaanxi has a good ecology; Northern Shaanxi has many polluting firms) and diverse types of firms (Miao et al., 2019; Wang et al., 2022c) in Shaanxi Province can provide effective lessons for pollutant management in other regions and countries. We collected a unique daily scale emission data from all continuous emission monitoring system (CEMS) installed firms in Shaanxi Province and air quality monitor site data at county level.
This paper are to make the following contributions to the field: 1) Establish a methodology for identifying PM2.5 pollution control areas using spatial clustering techniques; 2) Identify firms and industries with a significant impact on PM2.5 pollution areas by utilizing cross-wavelet analysis on high-frequency time scale data; 3) Provide evidence-based support for precisely requiring firms to adopt emission reduction measures during periods of heavy pollution, and effectively reducing socio-economic losses.
The rest of this paper is structured as follows. Part 2 introduces the study area, data, and methodology. Part 3, this study shows cluster and wavelet analysis. In the final part, this paper provides some concluding remarks.
Shaanxi Province, in northwestern China, spans between 105°29′E-111°15′E longitude and 31°42′N-39°35′N latitude, characterized by a complex and diverse terrain. Shaanxi Province, the most affluent among the five northwestern provinces of China, recorded a GDP of 2,980.1 billion RMB in 2021 (National Bureau of Statistics, 2023). The industrial sector played a substantial role, contributing 46.3% to the Shaanxi’s GDP. With a diverse industrial landscape encompassing high-end energy and chemical sectors, equipment manufacturing, aerospace, electronic information, and automobile production, Shaanxi Province’s industrial firms collectively consumed 92.8 million tonnes of standard coal in 2021. This consumption constituted 68.1% of the total standard coal usage within the province. Such considerable energy consumption consequently led to substantial pollutant emissions. Notably, industrial sources in Shaanxi Province contributed to over 60% of total emissions for both Total Suspended Particulates (TSP) and Sulfur Dioxide (SO2) pollutants in 2021 (Ministry of Ecology and Environment, 2021).
Shaanxi can be geographically divided into three parts: southern Shaanxi, Guanzhong and northern Shaanxi. Northern Shaanxi, which encompasses the cities of Yulin and Yan’an, is abundant in coal, oil, and natural gas resources and houses several large-scale energy chemical firms, including thermal power, coking, coal chemical, and petrochemical. The Guanzhong plain, comprising cities such as Xi’an, Xianyang, Weinan, Baoji, and Tongchuan, is recognized as one of China’s worst air pollution areas in China due to a significant number of thermal power, cement, and steel sectors (Bai et al., 2019). The Southern Shaanxi region, including the cities of Hanzhong, Shangluo, and Ankang, is rich in rare mineral resources, such as molybdenum, rhenium, and mercury, as well as abundant natural resources.
Figure 1 demonstrates the distribution of 169 air quality monitoring stations and 361 firms in Shaanxi Province. Specifically, Guanzhong has 60 air quality monitoring stations and 150 industrial firms, Northern Shaanxi has 59 air quality monitoring stations and 177 industrial firms, while Southern Shaanxi has 50 air quality monitoring stations and 34 industrial firms. Shaanxi’s average PM2.5 concentrations has decreased from 57 to 43 μg/m3 between 2017 and 2020. However, the current concentration remains above the national standard limit of 30 μg/m3.
The monitoring data of PM2.5 concentrations are from the ambient air quality monitoring stations in Shannxi Province (http://113.140.66.226:8024/sxAQIWeb/pagecity.aspx?cityCode=NjEwMTAw), and meteorological data including wind speed, wind direction, air temperature, air pressure, and humidity are from Shaanxi air quality real-time release system. The research period is from 1 January 2017 to 31 December 2020. The firms’ emissions data come from key pollutant discharge firms’ monitoring information release platforms (http://113.140.66.227:9777/envinfo_ps/zdyjbxxpublicity/list), including the three most important pollutants, TSP (total suspended particles), SO2 (sulfur dioxide) and NOx (nitrogen oxide). The pollutant emissions of the firms installing CEMS exceed 65% of the total pollutant emissions in the region (The State Council, 2007), which basically reflects the regional industrial pollutant emissions.
For air quality monitoring data, we remove outliers in the data and ensure the accuracy of the data by using data from neighbouring air quality monitoring points. For firm emission data, we firstly removed extreme outliers in the firms CEMS data; secondly, we determined whether the firms was in a shutdown state through the firms’ flue gas oxygen content, and for missing values in a non-shutdown state, we filled in the pollutant emissions of the firms neighboring time through the missing values to ensure the validity of the data.
PM2.5 concentrations exhibits spatial clustering on a daily basis. To identify regions that require targeted control during the study period, daily partitioning results of the clustering process are subjected to correlation clustering analysis. If the external transfer of pollutants is not taken into account, PM2.5 concentrations in the identified areas can be reduced by reducing all types of pollutant emissions, such as those from industrial, mobile and non-organized sources. Thiessen polygons are generated from the monitoring stations to ensure the continuity of the identified pollution area and to avoid the limitations imposed by administrative divisions. The resulting clustering categories are consistent with the geographical division, and further refine the control area for PM2.5 while smoothing out the boundary of the region.
This paper adopts the K-means clustering method, a fast clustering approach that abstracts clustering units into m-dimensional points and evaluates the similarity between units based on the distance between clustering units (Liu et al., 2018a), to conduct spatial clustering of PM2.5:
1) Optional K initial clustering centers: Z1(1), Z2(1), …, Zk(1), 1 is the subsequence of iteration.
2) The rest of the samples are allocated to one of the K cluster centers according to the principle of minimum distance:
X∈Si(k),k is the subsequence of iteration; K is the number of cluster centers.
3) Compute the new vector value of each cluster center: Zj (k+1) j=1, 2, … , K
4) If Zj(k+1)
The wavelet transform is a powerful method for the simultaneous analysis of time series in both the time and frequency domains. It involves the use of a variable window function and is generated from mother and child waves through time translation and scaling, as noted by Gao and Zhang (2016) and Mu et al. (2021). This decomposition results in a set of essential functions where increasing the scale is equivalent to decreasing the frequency and sacrificing the time resolution, while reducing the scale and increasing the frequency is equivalent to sacrificing the frequency resolution. By stretching and translational transform, the wavelet function is obtained from the wavelet generator function. The continuous wavelet function is expressed as:
where* represents a conjugate complex, N is the total number of the time series, (δt/s)/s is a factor used for the standardization of wavelet function.
The Morlet wavelet function is commonly used in wavelet analysis as it effectively separates and reconstructs waves of different frequency bands without losing time resolution. This function maintains its shape through frequency shift and has excellent temporal aggregation and high-frequency resolution (Morlet et al., 1982). Therefore, Morlet wavelet function is employed to analyze the periodicity of PM2.5 concentrations:
Where t is time,
The boundary effects and errors encountered when handling finite time series are addressed by testing the statistical significance of the wavelet power spectrum using an appropriate equation (Cazelles et al., 2008; Furon et al., 2008; Grinsted et al., 2004; Liu et al., 2018a). Therefore, the test measure display as follows:
Cross-wavelet analysis can compare the frequencies of two-time series Xt and Yt, and obtain the resonance period and phase of the two sequences in some periods. Cross wavelet spectra of two-time series XWT is
The phase difference can be transferred to the difference of [-π, π], and its value indicates the lag characteristics of the two sequences (Aguiar-Conraria and Joana Soares, 2011; Addesso et al., 2022). The phase difference formula is as follows:
The seasonal distribution of PM2.5 concentrations in each region is relatively even, with the highest levels in winter and the lowest in summer (Figure 2). During the winter and spring seasons, the PM2.5 concentrations in the three regions display significant variations, ranging from 65 to 95 μg/m3 and 29.5–47 μg/m3, respectively. This indicates that the concentrations during winter are approximately twice as high as those during spring. Conversely, PM2.5 concentrations slightly vary among the three regions during the summer and autumn seasons. Moreover, meteorological factors such as temperature, wind speed, and relative humidity significantly promote the diffusion of atmospheric pollutants. In mid-March, the cessation of heating results in a reduction of pollution sources, leading to lower PM2.5 concentrations during the spring season than during the winter season.
Annual PM2.5 concentrations in the three regions follow the order of Guanzhong > Northern Shaanxi > Southern Shaanxi. Guanzhong is the most economically developed region in Shaanxi Province and has several large coal-fired power plants, cement manufacturers, chemical plants, and metal smelters, which contribute to high PM2.5 concentrations. The Guanzhong area’s proximity to the Qinling Mountains in the South and the Loess Plateau in the North further hinders airflow movement and pollutant diffusion, thereby intensifying the pollutant concentration (Wang et al., 2015). In Northern Shaanxi, several coal-fired power plants and factories discharge pollutants, and the unique linear canyon terrain in Yan’an City tends to converge local pollutants in the area. Southern Shaanxi primarily relies on natural resources to develop the primary industry, has low urbanization, and has a less prominent contribution of firm emission sources to PM2.5 concentrations.
From Figure 2, it is obvious shows that PM2.5 pollution in Shaanxi Province has a significant regional feature, in order to accurately identify each PM2.5 pollution region in Shaanxi Province, so as to more accurate identification of the link between pollutant emissions from firms and PM2.5 in the air, this part use the PM2.5 data of the air monitoring points in the study time period, and the method of spatial clustering to perform spatial clustering. Figure 3 depicts the spatial clustering of air pollution areas in Shaanxi Province. By overlaying the zoning results onto the elevation map of Shaanxi Province, it becomes apparent that delimited regions of PM2.5 overlap with the locations of the Guanzhong Plain, Northern Shaanxi, and Southern Shaanxi, suggesting that PM2.5 concentrations are influenced by the topography. Three regions were identified based on their coverage area and PM2.5 concentration levels. Region 1 covers most of the Guanzhong area, region 2 includes the northern Shaanxi area and a portion of the Guanzhong area, while region 3 comprises the Southern Shaanxi area and a portion of the Guanzhong area. Region 1 had a mean PM2.5 of 57.4 μg/m3, and 335 days per year exceeded 24-h PM2.5 thresholds (<75 μg/m3), representing 20.41% of total days. In Region 2, the mean PM2.5 was 40.3 μg/m3, with 151 days exceeding the 24-h PM2.5 benchmark, representing 9.2% of the total number of days. Finally, the mean PM2.5 concentration in region 3 was 33.2 μg/m3, with 79 days exceeding the 24-h PM2.5 benchmark, representing 4.81% of the total number of days.
The analysis results presented in Figure 4 shows the variations in pollutant emissions among industrial firms in Shaanxi Province. Figure 4 shows that region 1 and region 2 emit about the same amount of TSP pollutants, while region 2 emits a much larger amount of SO2 and NOx than region 1 and region 3. This emission situation is closely related to the distribution of industries in different regions. This difference was attributed to the lots of power plants located in region 2, which accounts for 50% of the power plants in Shaanxi Province and contributes to over 70% of total pollutant emissions from power plants. Region 1 is distributed with many processing and manufacturing firms, which mainly emit TSP pollutants, making the emissions of TSP pollutants in region 1 and region 2 similar, although there are significant differences in SO2 and NOx emissions. Simultaneously, it can be observed that TSP emissions in region 3 saw a notable increase during the COVID-19 period in 2020. In contrast, emissions in regions 1 and 2 experienced a certain degree of decline. There’s a reasonable suspicion that regions 1 and 2 redirected a portion of their production capacity to region 3, which may account for this dynamic change.
FIGURE 4. Daily variation curves of air pollutants, including TSP, SO2, and NOx, emitted by industrial firms from 2017 to 2020.
This part we employ the Morlet wavelet function to conduct a wavelet transform of daily data on TSP, SO2, and NOx emissions from industrial firms in three regions of Shaanxi Province from January 2017 to December 2020. The wavelet spectrums of TSP, SO2, and NOx are analyzed, with time d as the horizontal axis and time scales as the vertical axis. A wavelet coefficient of 0 signifies a mutation point wherein the correlation coefficient shifts from higher to lower values. The white shadow area in the wavelet spectrum represents the influence cone of the wavelet boundary, while the area enclosed by thick white solid lines indicates that the noise test has passed at a 95% significance level.
Figure 5 shows that the pollutants emitted by industrial firms during the winter prevention period (November to February) in the three regions demonstrate substantial periodicities of 16–64 and 64–128 days, with the impact of contaminants on PM2.5 concentrations lagging 1/4-3/8 cycles. The TSP and PM2.5 manifest a strong correlation in the 16–32 days range, followed by NOx and PM2.5, while SO2 and PM2.5 exhibit the weakest correlation. These results coincide with the fact that TSP directly influences PM2.5 concentrations in the atmosphere, while NOx and SO2 necessitate a chemical reaction to generate nitrate and sulfate and form secondary particles. Simultaneously, the figure shows a notable correlation between pollutant emissions from firm and PM2.5 concentrations within short timeframes in both region 1 and region 2. This strong correlation strongly suggests that firms’ pollutant emissions play a pivotal role in driving fluctuations in PM2.5 concentrations, particularly during the winter months. However, this short-term influence on PM2.5 concentrations as a result of corporate emissions is not evident in region 3.
FIGURE 5. Spatiotemporal correlation between PM2.5 concentrations and industrial firms’ emissions in different regions of PM2.5 pollution. Note: region 1 represents Guanzhong, region 2 represents Northern Shaanxi, and region 3 represents Southern Shaanxi. (A,D,G) Region 1 TSP, Region 2 TSP, Region 3 TSP; (B,E,H) Region 1 S02, Region 2 S02, Region 3 S02; (C,F I) Region 1 NOx, Region 2 NOx, Region 3 NOx.
Figure 5 also shows that the emissions from industrial firms in region 1 strongly resonate with PM2.5 from November to February 2017 and 2018. However, during a short period of 32–64 days, the emissions negatively related with PM2.5 concentrations, implying that changes in PM2.5 concentrations lag behind alterations in TSP emissions from firms. In the winter of 2019, there is a significant correlation between firm emissions and PM2.5 concentrations, but no significant correlation is found in the spring of 2020 since industrial emissions reduced during the COVID-19 lockdown, which caused an unprecedented cessation of human activities that affected China’s industrial production and pollutant emissions. Therefore, controlling industrial emissions can alleviate PM2.5 pollution in Shaanxi.
Using region 1 as an example, the study found a strong correlation between PM2.5 concentrations and pollutants emitted by thermal production, supply, and steel industries within a short timeframe of 8–32 days. However, the correlation coefficient displays irregular fluctuations (Figure 6). Conversely, there was a weak correlation between PM2.5 and emissions from cement and power sectors. During a medium to long timeframe of 32–64 days, TSP emissions from heat production and supply firms in 2018 and 2019 exhibited correlations with PM2.5 concentrations. Moreover, SO2 positively related PM2.5 concentrations in 2018 and 2019, while NOx and PM2.5 displayed positive correlations in 2019 and 2020. Between 2017 and 2019, TSP, SO2, and NOx emissions from cement manufacturing firms demonstrated strong positive correlations with PM2.5. In contrast, there were weak correlations between PM2.5 concentrations and pollutants emitted by firms in the power and iron-steel industries. Within a long timeframe of 64–128 days, the correlation between emissions in the above four industries and PM2.5 concentrations gradually weakened, except for significant relationships between PM2.5 and NOx emitted by thermal production and supply firms.
FIGURE 6. Spatiotemporal correlation between PM2.5 concentration and the air pollutants emitted by different industrial firms in region1 (Guanzhong). (A,D,G,J) TSP steel industry, TSP thermal power industry, TSP cement manufacturing, PM heat production and supply; (B,E,H,K) S02 steel industry, S02 thermal power industry, S02 cement manufacturing, S02 heat production and supply; (C,F,I,L) NOx steel industry, NOx thermal power industry, NOx cement manufacturing, NOx heat production and supply.
In addition to firms’ emissions, meteorological factors are also an important driver of PM2.5 concentrations (Jia et al., 2020). This section takes region 1 as an example to further analyse whether meteorology further influences the changes in PM2.5 concentrations on basis of emissions from firms. Specifically, the study examines the periodic effects of atmospheric pressure, temperature, humidity, wind speed, and sunshine on PM2.5 using time-series data and Morlet cross-wavelet transform analysis. Figure 7 shows that the PM2.5 concentration has a negative correlation with atmospheric pressure, humidity, wind speed, and sunshine on an 8–32 days time scale, while no significant correlations are observed with air temperature. In addition, the degree of correlation between PM2.5 concentration and wind direction, air pressure, and humidity during the winter defense period in 2017–2018 and 2018–2019 is significantly higher than that in other years. In a certain extent, it can be inferred from Figures 5A–C that a connection exists between the heightened responsiveness of firms’ emissions to ambient PM2.5 and alterations in meteorological conditions. This dynamic suggests that firms emit comparable levels of pollutants but contribute to more PM2.5 pollution, possibly due to changing meteorological factors.
FIGURE 7. Spatiotemporal correlation between PM2.5 concentrations and meteorological factors in region 1 (Guanzhong). (A) Region 1 pressure, (B) Region 1 temperature, (C) Region 1 humidity, (D) Region 1 sunshine hours, (E) Region 1 wind speed.
This study utilizes data of PM2.5 concentrations collected from 169 air quality monitoring stations and 361 industrial firms situated in all county-level cities within Shaanxi Province. The spatial cluster analysis method is used to determine the clustering region of PM2.5, while the spatiotemporal correlation between PM2.5 concentrations and industrial firm emissions in different smog-contaminated areas is evaluated using the cross-wavelet analysis method.
The findings of this study indicate that the mean PM2.5 concentrations in Shaanxi Province decreased from 50.2 to 38.1 μg/m3 between 2017 and 2020, which can be attributed to the 3-year plan to control haze and improve air quality. The mean PM2.5 concentrations in summer and winter were 38.9 μg/m3 and 77.6 μg/m3, respectively, with higher concentrations observed during winter. Additionally, the mean PM2.5 concentrations in Guanzhong, Northern Shaanxi, and Southern Shaanxi were 57.4, 40.3, and 33.2 μg/m3, respectively, with Guanzhong having a higher PM2.5 concentration than Southern Shaanxi.
The K-means clustering method is employed to cluster daily PM2.5 concentrations in winter, and three pollution areas are identified based on ground elevation information and geographical subregions of Shaanxi Province. PM2.5 concentrations in the three regions of Shaanxi Province during winter show periodic changes, with pollutants emitted by firms lagging about 1/4-1/2 of the period. In the 16–32 days period, PM2.5 concentrations are significantly affected by PM, followed by NOx and SO2. NOx has significantly affected PM2.5 concentrations in the 32–64 days period, while in the 64–128 days period, the impact of NOx and SO2 on PM2.5 concentrations is similar. Industrial firms in regions 1 and 2 significantly affect local PM2.5 concentrations, while the impact of industrial firms in region 3 is minor.
We also found during the COVID-19 lockdown, industrial firm emissions, excluding those in the heat production and supply industry, had no significant impact on PM2.5 concentrations, indicating that controlling air pollutants emitted from firms can alleviate PM2.5 in Shaanxi. Furthermore, pollutants emitted by firms in the thermal production, supply, and steel industries have a greater impact on PM2.5 concentrations than those from the cement manufacturing and power industries.
The study findings suggest that the government should focus on reducing and eliminating backward production capacity, strengthening discharge control of thermal power production, supply, and steel-iron industries, and promoting industrial structure optimization and upgrading in Shaanxi Province to reduce PM2.5 concentrations during winter. Specifically, for the Guanzhong and northern Shaanxi regions, there is a particular need to control pollutant emissions from firms under unfavourable meteorological conditions in order to effectively mitigate the severe PM2.5 pollution caused by firms’ emissions. For the southern Shaanxi region, there is a need to prevent firms in the northern Shaanxi and Guanzhong regions from relocating or relocating some of their production orders to southern Shaanxi.
The original contributions presented in the study are included in the article/Supplementary material, further inquiries can be directed to the corresponding author.
JZ: Data curation, Formal Analysis, Methodology, Software, Writing–original draft. LY: Formal Analysis, Writing–original draft. CJ: Visualization, Writing–review and editing. PG: Visualization, Writing–review and editing.
We would like to acknowledge the reviewers for their valuable comments.
The authors declare that the research was conducted in the absence of any commercial or financial relationships that could be construed as a potential conflict of interest.
All claims expressed in this article are solely those of the authors and do not necessarily represent those of their affiliated organizations, or those of the publisher, the editors and the reviewers. Any product that may be evaluated in this article, or claim that may be made by its manufacturer, is not guaranteed or endorsed by the publisher.
Addesso, R., Bellino, A., and Baldantoni, D. (2022). Underground ecosystem conservation through high-resolution air monitoring. Environ. Manage. 69, 982–993. doi:10.1007/s00267-022-01603-0
Aguiar-Conraria, L., and Joana Soares, M. (2011). Business cycle synchronization and the euro: A wavelet analysis. J. Macroecon. 33, 477–489. doi:10.1016/j.jmacro.2011.02.005
Alari, A., Schwarz, L., Zabrocki, L., Le Nir, G., Chaix, B., Benmarhnia, T., et al. (2021). The effects of an air quality alert program on premature mortality: A difference-in-differences evaluation in the region of paris. Environ. Int. 156, 106583. doi:10.1016/j.envint.2021.106583
Azmi, S., Sharma, M., and Nagar, P. K. (2022). NMVOC emissions and their formation into secondary organic aerosols over India using WRF-Chem model. Atmos. Environ. 287, 119254. doi:10.1016/j.atmosenv.2022.119254
Bai, K., Ma, M., Chang, N.-B., and Gao, W. (2019). Spatiotemporal trend analysis for fine particulate matter concentrations in China using high-resolution satellite-derived and ground-measured PM2.5 data. J. Environ. Manage. 233, 530–542. doi:10.1016/j.jenvman.2018.12.071
Barwick, P. J., Li, S., Rao, D., and Zahur, N. (2017). Air pollution, health spending and willingness to pay for clean air in China. SSRN Electron. J. doi:10.2139/ssrn.2999068
Cazelles, B., Chavez, M., Berteaux, D., Ménard, F., Vik, J. O., Jenouvrier, S., et al. (2008). Wavelet analysis of ecological time series. Oecologia 156, 287–304. doi:10.1007/s00442-008-0993-2
Chen, S., Guo, C., and Huang, X. (2018). Air pollution, student health, and school absences: evidence from China. J. Environ. Econ. Manage. 92, 465–497. doi:10.1016/j.jeem.2018.10.002
Chen, C., Sun, Y., Lan, Q., and Jiang, F. (2020). Impacts of industrial agglomeration on pollution and ecological efficiency-A spatial econometric analysis based on a big panel dataset of China’s 259 cities. J. Clean. Prod. 258, 120721. doi:10.1016/j.jclepro.2020.120721
Chen, Z., Wang, F., Liu, B., and Zhang, B. (2022). Short-term and long-term impacts of air pollution control on China’s economy. Environ. Manage. 70, 536–547. doi:10.1007/s00267-022-01664-1
Cheung, C. W., He, G., and Pan, Y. (2020). Mitigating the air pollution effect? The remarkable decline in the pollution-mortality relationship in Hong Kong. J. Environ. Econ. Manage. 101, 102316. doi:10.1016/j.jeem.2020.102316
Choi, W. J., Jung, B., Lee, D., Kang, H., Kim, H., Hong, H., et al. (2021). An investigation into the effect of emissions from industrial complexes on air quality in the ulsan metropolitan city utilizing trace components in PM2.5. Appl. Sci. 11, 10003. doi:10.3390/app112110003
Fan, M., He, G., and Zhou, M. (2020). The winter choke: coal-fired heating, air pollution, and mortality in China. J. Health Econ. 71, 102316. doi:10.1016/j.jhealeco.2020.102316
Frankowski, J. (2020). Attention: smog alert! Citizen engagement for clean air and its consequences for fuel poverty in Poland. Energy Build. 207, 109525. doi:10.1016/j.enbuild.2019.109525
Furon, A. C., Wagner-Riddle, C., Smith, C. R., and Warland, J. S. (2008). Wavelet analysis of wintertime and spring thaw CO2 and N2O fluxes from agricultural fields. Agric. For. Meteorol. 148, 1305–1317. doi:10.1016/j.agrformet.2008.03.006
Greenstone, M., He, G., Li, S., and Zou, E. Y. (2021). China’s war on pollution: evidence from the first 5 years. Rev. Environ. Econ. Policy 15, 281–299. doi:10.1086/715550
Grinsted, A., Moore, J. C., and Jevrejeva, S. (2004). Application of the cross wavelet transform and wavelet coherence to geophysical time series. Nonlinear process. geophys. 11, 561–566. doi:10.5194/npg-11-561-2004
He, G., Liu, T., and Zhou, M. (2020). Straw burning, PM2.5, and death: evidence from China. J. Dev. Econ. 145, 102468. doi:10.1016/j.jdeveco.2020.102468
Ito, K., and Zhang, S. (2020). Willingness to pay for clean air: evidence from air purifier markets in China. J. Polit. Econ. 128, 1627–1672. doi:10.1086/705554
Jia, C., Wang, Y., and Yang, X. (2020). Analysis on the periodic variation of PM2.5 concentration and its causes in Guanzhong Plain. Arid. Zo. Res. 37. doi:10.13866/j.azr.2020.06.18
Kapwata, T., Wright, C. Y., du Preez, D. J., Kunene, Z., Mathee, A., Ikeda, T., et al. (2021). Exploring rural hospital admissions for diarrhoeal disease, malaria, pneumonia, and asthma in relation to temperature, rainfall and air pollution using wavelet transform analysis. Sci. Total Environ. 791, 148307. doi:10.1016/j.scitotenv.2021.148307
Li, G., Deng, S., Li, J., Gao, J., Lu, Z., Yi, X., et al. (2022). Levels, ozone and secondary organic aerosol formation potentials of BTEX and their health risks during autumn and winter in the Guanzhong Plain, China. Air Qual. Atmos. heal. 15, 1941–1952. doi:10.1007/s11869-022-01228-6
Li, M., Ren, X., Zhou, L., and Zhang, F. (2016). Spatial mismatch between pollutant emission and environmental quality in China — a case study of NOx. Atmos. Pollut. Res. 7, 294–302. doi:10.1016/j.apr.2015.10.005
Li, Z., Sun, Z., Shao, X., Liao, X., Zhang, X., Xiong, Y., et al. (2017). Using Morlet wavelet analysis to analyze multiple time scale periodically in PM2.5 in Beijing. China Environ. Sci. 37, 407–415. doi:10.3969/j.issn.1000-6923.2017.02.002
Liu, G., Yang, J., Hao, Y., and Zhang, Y. (2018a). Big data-informed energy efficiency assessment of China industry sectors based on K-means clustering. J. Clean. Prod. 183, 304–314. doi:10.1016/j.jclepro.2018.02.129
Liu, H., and Salvo, A. (2018). Severe air pollution and child absences when schools and parents respond. J. Environ. Econ. Manage. 92, 300–330. doi:10.1016/j.jeem.2018.10.003
Liu, Y., Wu, J., Yu, D., and Hao, R. (2018b). Understanding the patterns and drivers of air pollution on multiple time scales: the case of northern China. Environ. Manage. 61, 1048–1061. doi:10.1007/s00267-018-1026-5
Lu, W., Tam, V. W., Du, L., and Chen, H. (2021). Impact of industrial agglomeration on haze pollution: new evidence from bohai sea economic region in China. J. Clean. Prod. 280, 124414. doi:10.1016/j.jclepro.2020.124414
Ma, S., Xiao, Z., Zhang, Y., Wang, L., and Shao, M. (2020). Assessment of meteorological impact and emergency plan for a heavy haze pollution episode in a core city of the North China plain. Aerosol Air Qual. Res. 20, 26–42. doi:10.4209/aaqr.2019.08.0392
Mee, (2022). 2021 communiqué on the status of China’s ecology and environment. Avaialble at: http://www.gov.cn/xinwen/2022-05/28/content_5692799.htm (accessed May 28, 2022).
Miao, Z., Baležentis, T., Shao, S., and Chang, D. (2019). Energy use, industrial soot and vehicle exhaust pollution—China’s regional air pollution recognition, performance decomposition and governance. Energy Econ. 83, 501–514. doi:10.1016/j.eneco.2019.07.002
Ministry of Ecology and Environment, (2021). China ecological environment Statistics annual report 2021. Avaialble at: https://www.mee.gov.cn/hjzl/sthjzk/sthjtjnb/202301/W020230118392178258531.pdf.
Morlet, J., Arens, G., Fourgeau, E., and Giard, D. (1982). Wave propagation and sampling theory - Part II. Sampling theory and complex waves. Geophysics 47, 222–236. doi:10.1190/1.1441329
National Bureau of Statistics, (2023). China statistical yearbook 2022. Avaialble at: http://www.stats.gov.cn/sj/ndsj/2022/indexch.htm.
Rivera, N. M. (2021). Air quality warnings and temporary driving bans: evidence from air pollution, car trips, and mass-transit ridership in Santiago. J. Environ. Econ. Manage. 108, 102454. doi:10.1016/j.jeem.2021.102454
Shi, M., Wu, H., Zhang, S., Li, H., Yang, T., Liu, W., et al. (2014). Weekly cycle of magnetic characteristics of the daily PM2.5 and PM2.5-10 in Beijing, China. Atmos. Environ. 98, 357–367. doi:10.1016/j.atmosenv.2014.08.079
Spiridonov, V., Jakimovski, B., Spiridonova, I., and Pereira, G. (2019). Development of air quality forecasting system in Macedonia, based on WRF-Chem model. Air Qual. Atmos. heal. 12, 825–836. doi:10.1007/s11869-019-00698-5
Sun, Z., Lu, W., Wang, Y., Li, Y., Wei, H., Shi, J., et al. (2017). ER model based supervision system analysis using information disclosure. Clust. Comput. 20, 215–224. doi:10.1007/s10586-017-0779-6
Sun, C., Zheng, S., Wang, J., and Kahn, M. E. (2019). Does clean air increase the demand for the consumer city? Evidence from beijing. J. Reg. Sci. 59, 409–434. doi:10.1111/jors.12443
The State Council, (2007). Environmental Protection Bureau releases list of national key monitoring enterprises. Avaialble at: https://www.gov.cn/zfjg/content_566589.htm.
Tie, X., and Cao, J. (2009). Aerosol pollution in China: present and future impact on environment. Particuology 7, 426–431. doi:10.1016/j.partic.2009.09.003
Tie, X., Madronich, S., Walters, S., Edwards, D. P., Ginoux, P., Mahowald, N., et al. (2005). Assessment of the global impact of aerosols on tropospheric oxidants. J. Geophys. Res. D. Atmos. 110, D03204. doi:10.1029/2004JD005359
Torrence, C., and Compo, G. P. (1998). A practical guide to wavelet analysis. Bull. Am. Meteorol. Soc. 79, 61–78. doi:10.1175/1520-0477(1998)079<0061:APGTWA>2.0.CO;2
Torrence, C., and Webster, P. J. (1999). Interdecadal changes in the ENSO-monsoon system. J. Clim. 12, 2679–2690. doi:10.1175/1520-0442(1999)012<2679:icitem>2.0.co;2
Tu, M., Liu, Z., He, C., Fang, Z., and Lu, W. (2019). The relationships between urban landscape patterns and fine particulate pollution in China: A multiscale investigation using a geographically weighted regression model. J. Clean. Prod. 237, 117744. doi:10.1016/j.jclepro.2019.117744
Veleda, D., Montagne, R., and Araujo, M. (2012). Cross-wavelet bias corrected by normalizing scales. J. Atmos. Ocean. Technol. 29, 1401–1408. doi:10.1175/JTECH-D-11-00140.1
Wang, F., Zhao, H., Zhang, X., Niu, C., and Ma, J. (2019a). Understanding individual-level protective responses to air pollution warning: A case study of Beijing, China. Hum. Ecol. Risk Assess. Int. J. 25, 1473–1487. doi:10.1080/10807039.2018.1468995
Wang, K., Gao, J., Liu, K., Tong, Y., Dan, M., Zhang, X., et al. (2022a). Unit-based emissions and environmental impacts of industrial condensable particulate matter in China in 2020. SSRN Electron. J. doi:10.2139/ssrn.4035367
Wang, K., Gao, J., Liu, K., Tong, Y., Dan, M., Zhang, X., et al. (2022b). Unit-based emissions and environmental impacts of industrial condensable particulate matter in China in 2020. Chemosphere 303, 134759. doi:10.1016/j.chemosphere.2022.134759
Wang, M., Wang, W., and Wu, L. (2022c). Application of a new grey multivariate forecasting model in the forecasting of energy consumption in 7 regions of China. Energy 243, 123024. doi:10.1016/j.energy.2021.123024
Wang, P., Cao, J., Tie, X., Wang, G., Li, G., Hu, T., et al. (2015). Impact of meteorological parameters and gaseous pollutants on PM2.5 and PM10 mass concentrations during 2010 in Xi’an, China. Aerosol Air Qual. Res. 15, 1844–1854. doi:10.4209/aaqr.2015.05.0380
Wang, P., Guo, H., Hu, J., Kota, S. H., Ying, Q., and Zhang, H. (2019b). Responses of PM2.5 and O3 concentrations to changes of meteorology and emissions in China. Sci. Total Environ. 662, 297–306. doi:10.1016/j.scitotenv.2019.01.227
Wang, S., Liu, X., Yang, X., Zou, B., and Wang, J. (2018). Spatial variations of PM2.5 in Chinese cities for the joint impacts of human activities and natural conditions: A global and local regression perspective. J. Clean. Prod. 203, 143–152. doi:10.1016/j.jclepro.2018.08.249
Wang, Y., Wan, Q., Meng, W., Liao, F., Tan, H., and Zhang, R. (2011). Long-term impacts of aerosols on precipitation and lightning over the Pearl River Delta megacity area in China. Atmos. Chem. Phys. 11, 12421–12436. doi:10.5194/acp-11-12421-2011
Wegner, T., Hussein, T., Hämeri, K., Vesala, T., Kulmala, M., and Weber, S. (2012). Properties of aerosol signature size distributions in the urban environment as derived by cluster analysis. Atmos. Environ. 61, 350–360. doi:10.1016/j.atmosenv.2012.07.048
WHO (2021). Global air quality guidelines. Avaialble at: https://www.who.int/news-room/questions-and-answers/item/who-global-air-quality-guidelines.
Wu, Q., Hong, S., Yang, L., Mu, H., Huang, C., Niu, X., et al. (2023). Coupling coordination relationships between air pollutant concentrations and emissions in China. Atmos. Pollut. Res. 14, 101678. doi:10.1016/j.apr.2023.101678
Xu, Y., Ying, Q., Hu, J., Gao, Y., Yang, Y., Wang, D., et al. (2018). Spatial and temporal variations in criteria air pollutants in three typical terrain regions in Shaanxi, China, during 2015. Air Qual. Atmos. heal. 11, 95–109. doi:10.1007/s11869-017-0523-7
Zhang, R., Jing, J., Tao, J., Hsu, S.-C., Wang, G., Cao, J., et al. (2014). Corrigendum to “Chemical characterization and source apportionment of PM2.5 in Beijing: seasonal perspective” published in Atmos. Chem. Phys., 13, 7053–7074, 2013. Atmos. Chem. Phys. 14, 175. doi:10.5194/acp-14-175-2014
Zhang, H., Song, H., Wang, X., Wang, Y., Min, R., Qi, M., et al. (2023). Effect of agricultural soil wind erosion on urban PM2.5 concentrations simulated by WRF-chem and weps: A case study in kaifeng, China. Chemosphere 323, 138250. doi:10.1016/j.chemosphere.2023.138250
Zhang, J., and Mu, Q. (2018). Air pollution and defensive expenditures: evidence from particulate-filtering facemasks. J. Environ. Econ. Manage. 92, 517–536. doi:10.1016/j.jeem.2017.07.006
Zhang, L., An, J., Liu, M., Li, Z., Liu, Y., Tao, L., et al. (2020a). Spatiotemporal variations and influencing factors of PM2.5 concentrations in Beijing, China. Environ. Pollut. 262, 114276. doi:10.1016/j.envpol.2020.114276
Zhang, W., Wang, H., Zhang, X., Peng, Y., Zhong, J., Wang, Y., et al. (2020b). Evaluating the contributions of changed meteorological conditions and emission to substantial reductions of PM2.5 concentration from winter 2016 to 2017 in Central and Eastern China. Sci. Total Environ. 716, 136892. doi:10.1016/j.scitotenv.2020.136892
Zhang, X., Shi, M., Li, Y., Pang, R., and Xiang, N. (2018). Correlating PM2.5 concentrations with air pollutant emissions: A longitudinal study of the beijing-tianjin-hebei region. J. Clean. Prod. 179, 103–113. doi:10.1016/j.jclepro.2018.01.072
Zhao, H., Wang, F., Niu, C., Wang, H., and Zhang, X. (2018). Red warning for air pollution in China: exploring residents’ perceptions of the first two red warnings in beijing. Environ. Res. 161, 540–545. doi:10.1016/j.envres.2017.11.058
Zhao, S., Yu, Y., Yin, D., He, J., Liu, N., Qu, J., et al. (2016). Annual and diurnal variations of gaseous and particulate pollutants in 31 provincial capital cities based on in situ air quality monitoring data from China National Environmental Monitoring Center. Environ. Int. 86, 92–106. doi:10.1016/j.envint.2015.11.003
Zhao, X., Thomas, C. W., and Cai, T. (2020). The evolution of policy instruments for air pollution control in China: A content analysis of policy documents from 1973 to 2016. Environ. Manage. 66, 953–965. doi:10.1007/s00267-020-01353-x
Zhao, X., Zhang, X., Xu, X., Xu, J., Meng, W., and Pu, W. (2009). Seasonal and diurnal variations of ambient PM2.5 concentration in urban and rural environments in Beijing. Atmos. Environ. 43, 2893–2900. doi:10.1016/j.atmosenv.2009.03.009
Keywords: industrial firms’ emissions, PM2.5, cross-wavelet method, spatiotemporal clustering, China
Citation: Zhao J, Yuan L, Jia C and Guan P (2023) Relationship between PM2.5 pollution and firms’ emissions in Shaanxi Province, China. Front. Earth Sci. 11:1256296. doi: 10.3389/feart.2023.1256296
Received: 10 July 2023; Accepted: 28 August 2023;
Published: 11 September 2023.
Edited by:
Chuanbao Wu, Shandong University of Science and Technology, ChinaReviewed by:
Xiaowei Sun, China Waterborne Transport Research Institute, ChinaCopyright © 2023 Zhao, Yuan, Jia and Guan. This is an open-access article distributed under the terms of the Creative Commons Attribution License (CC BY). The use, distribution or reproduction in other forums is permitted, provided the original author(s) and the copyright owner(s) are credited and that the original publication in this journal is cited, in accordance with accepted academic practice. No use, distribution or reproduction is permitted which does not comply with these terms.
*Correspondence: Linjiang Yuan, eXVhbmxpbmppYW5nQHhhdWF0LmVkdS5jbg==
Disclaimer: All claims expressed in this article are solely those of the authors and do not necessarily represent those of their affiliated organizations, or those of the publisher, the editors and the reviewers. Any product that may be evaluated in this article or claim that may be made by its manufacturer is not guaranteed or endorsed by the publisher.
Research integrity at Frontiers
Learn more about the work of our research integrity team to safeguard the quality of each article we publish.