- 1Geomatics Research Center, A.O. Kovalevsky Institute of Biology of the Southern Seas of RAS, Sevastopol, Russia
- 2Department of Subtropical and Tropical Ecology, Institute of Environmental Engineering, Peoples’ Friendship University of Russia (RUDN University), Moscow, Russia
Since the end of the 20th century, the use of geographic information systems and digital elevation models has reduced the time required for and improved the quality of morphometric analysis of the relief within river basins. However, researchers are constantly faced with the problem of choosing the most accurate and suitable digital terrain model for their task. Many global, regional, and local digital elevation models are available. In this study, we comparatively analyzed the accuracy of the ASTER GDEM, ALOS World 3D, Copernicus DEM, and SRTM DEM spatial datasets for the purpose of catchment basin modeling for the river basins of the northwestern slope of the Crimean Mountains (Zapadnyy Bulganak, Alma, Kacha, Belbek, and Chernaya Rivers) as an example. For each river basin, we calculated the systematic, root mean square, mean absolute, standard root mean square (Bessel’s correction), and centered mean absolute errors by comparing ASTER GDEM, ALOS World 3D, Copernicus DEM, and SRTM DEM data with a 1:100,000 topographic map within the considered river basins. We found the smallest error values for the Copernicus DEM and ALOS World 3D datasets; furthermore, we used the Copernicus DEM dataset to model the river basins and sub-basins of the northwestern slope of the Crimean Mountains. As a result, we identified these river basins and sub-basins for the Zapadnyy Bulganak, Alma, Kacha, Belbek, and Chernaya Rivers, which are represented by stream basins, valleys, gullies, and ravine systems.
1 Introduction
Digital elevation model (DEM) is a generic term for digital topographic and/or bathymetric data in all their forms (Manune, 2007). A large number of studies have been devoted to assessing the accuracy of DEM, considering both the practical and theoretical aspects of this issue (del Rosario Gonzalez-Moradas and Viveen, 2020; Mesa-Mingorance and Ariza-López, 2020; Uuemaa et al., 2020; Yamazaki et al., 2017). The number of these scientific studies has been constantly increasing due to the improvement in existing geodatasets and the market entry of new sets of geodata, which is of considerable interest to researchers. In recent years, DEMs created using unmanned aerial vehicles (UAVs) (Uysal et al., 2015; Hashemi-Beni et al., 2018; Escobar Villanueva et al., 2019; Annis et al., 2020) have been extensively used. UAVs have high accuracy but, in almost all cases, are inaccessible to a wide range of researchers. DEMs are actively used in the study of glaciers (Fischer et al., 2015; Bodin et al., 2018), forests (Balzter et al., 2015; Liu et al., 2018), and celestial bodies (Florinsky and Filippov, 2017; Fawdon et al., 2018), among others. One of the largest niche areas is occupied by studies of the morphometry of river basins (Pyankov and Shikhov, 2017; Fang et al., 2019; Sarkar et al., 2020; Yermolaev et al., 2021; Zhao et al., 2021), in which DEMs are actively used to identify and characterize river basins.
ASTER and SRTM DEMs have been thoroughly compared. For example, Rajasekhar et al. (2018) studied lineament extraction from ASTER DEM, SRTM, and Cartosat for the Jilledubanderu River basin, Anantapur district, India. Thomas and Prasannakumar, 2015 studied basin morphometry derived from topographic maps, ASTER, and SRTM DEMs, considering an example from Kerala, India. Nikolakopoulos et al. (2006) compared ASTER and SRTM DEMs in Greece using two regions of Crete Island. Zhao et al. (2021) compared the performance among typical open global DEM datasets for the Fenhe River Basin in China.
Due to the emergence of a large number of new open datasets, researchers have been increasingly using Copernicus DEM and comparing different datasets (Karlson et al., 2021; Garrote, 2022; Yuan et al., 2022). Mutar et al. (2021) considered the river basins flowing into the Mosul reservoir (Iraq), finding that the Copernicus DEM model was more accurate than SRTM DEM and ASTER GDEM. However, other authors (Kramm and Hoffmeister, 2021) reported that Copernicus DEM data can produce ambiguous results. Many studies (Karionov, 2010; Yeritsian, 2013; Trofimov and Filippova, 2014) have described the accuracy of the SRTM dataset. Nevertheless, other authors (Karionov, 2010; Yeritsian, 2013) have emphasized that the accuracy of the cartographic material obtained using the SRTM datasets is equivalent or close to that of topographic map. In recent years, ALOS World 3D DEM (Tadono et al., 2016) was also introduced and its accuracy, as well as advantages and disadvantages compared with other DEMs (Courty et al., 2019; Viel et al., 2020), have been analyzed. For the Crimean Peninsula, SRTM are mainly used by researchers, but comparisons with other DEMs are not given and measurement errors are not evaluated.
Several global datasets contain information on river basins on a global scale (Tang and Lettenmaier, 2012; Lehner and Grill G., 2013; Dallaire et al., 2019). For example, the HydroBASINS Version 1.0 dataset contains information on river basins and sub-basins worldwide. This dataset is not suitable for the Crimean Peninsula due to a large number of errors, in particular, incorrect allocation of the catchment basins’ boundaries (for example, merged boundaries of the South Coast of the Crimean Peninsula river basins, unreasonable basin division of the largest rivers of the Crimean Peninsula into logically unreasonable parts, etc.). Most regional models in Europe do not include the Crimean Peninsula in the research area, which complicates further analysis and comparison of catchment basins (Vanham and Bidoglio, 2014). Additionally, the identification of small river basins or sub-basins for most large rivers of the Crimean Peninsula is limited only to the main tributaries, whereas the catchment basins of tributaries and their tributaries are practically not considered, with the exception of the most studied and largest river of the Crimean Peninsula, the Salgir River, as well as a small number of Crimean rivers.
Three groups can be distinguished among the studies on the catchment basins of the Crimean Peninsula. The first small group consists of studies (Dunaieva and Kovalenko, 2013; Narozhnyaya, 2021) that considered the river basins of the Crimean Peninsula as a whole. Almost always (with the exception of one study (Pozachenyuk, 2009), which is of historical value), river basins have been automatically identified using geographic information systems and DEMs. The second group consists of studies devoted to river basin groups in certain regions of the Crimean Peninsula: the river basins of the northwestern slope of the Crimean Mountains (Vermaat et al., 2012; Tabunshchik, 2018), the Kerch Peninsula (Krivoguz, 2016), the Sivash region (Timchenko et al., 2020), and the northern macroslope of the Crimean Mountains (Timchenko, 2000). The third and most numerous groups of studies has focused on the catchment basins of different separate rivers of the Crimean Peninsula (Vlasova, 2011; Pozachenyuk et al., 2014; Ergina and Timchenko, 2016; Kayukova, 2016; Amelichev et al., 2017). Moreover, in the third group, the most studied river basins are the basins of the largest rivers and their main tributaries.
The purpose of the study is to select the most accurate DEMs and, on its basis, to identify the basins and sub-basins of the rivers within northwestern slope of Crimean Mountains. Specifically, the main contents of this study are as follows. In the Section 2 “Materials and Methods,” four DEMs are compared with a topographic map and a general scheme of research using geoinformation research methods are presented. In the Section 3 “Results,” the calculation of measurement errors typical for various DEMs are shown. Also, the result of modeling the allocation of basins and sub-basins of the rivers of the northwestern slope of the Crimean Mountains are presented. In the Section 4, discussion of the obtained results and their comparison with other regions of the world are shown. Also, in the Section 4, the difficulties that the authors encountered while working on the article and ways to solve them are described. In the Section 5, conclusions and implications are given.
2 Materials and methods
2.1 Study area
The basins of the Zapadnyy Bulganak, Alma, Kacha, Belbek, Chernaya Rivers are located in the southwestern part of the Crimean Peninsula (Figure 1). The area of the studied territory comprises approximately 2,299 sq km. The rivers originate in the Crimean Mountains and flow into the Black Sea (Tabunshchik et al., 2022).
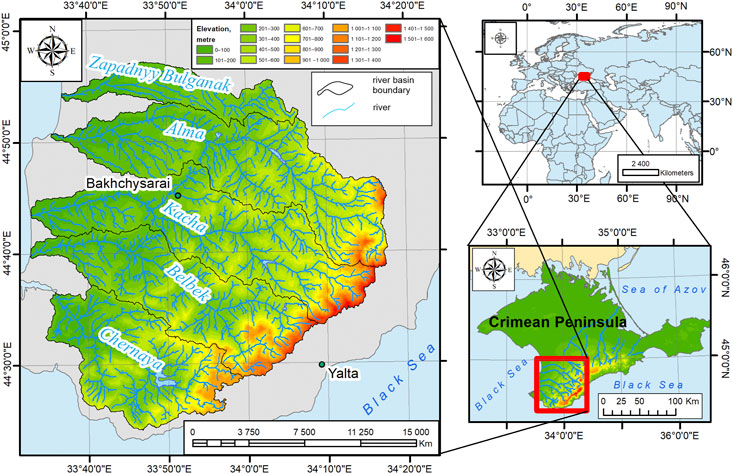
FIGURE 1. Geographical location of the study area (Tabunshchik et al., 2022).
2.2 Materials and methods
We chose the DEMs for identifying river basins by selecting those most suitable for the study geodatasets, with the highest spatial resolution, and distributed under an open license. We thus selected ASTER GDEM (Version 3, 2019), ALOS World 3D (Version 3.2, 2021), Copernicus DEM (Version 3, 2021), and SRTM DEM (Version 3, 2013) for this study among all DEMs available to us. The spatial resolution of these DEMs is 30 m/pixel.
As a DEM differs from the real terrain elevation, as for each pixel, an average value is given, we verified the selected DEMs by comparing the height marks and elevation values obtained from the topographic map. From a topographic map with a scale of 1:100,000 [which was previously linked to the WGS 84 UTM zone 36 N (EPSG: 32,636) projection], we obtained sample values of several peaks (mountains, points) and isohypses, which we then compared with the elevation values of the same points on each of the considered DEMs through a simple spatial relationship. For these purposes, a point shapefile was created containing the elevation values of points from a topographic map. Then, using the tools “Spatial Join” and “ Extract Values to Points,” the elevation values from each DTM for each point were obtained. In total, we selected 100 points for each catchment basin, and then we compared the obtained data. Accuracy was calculated according to a previously reported method (Onkov, 2011). An additive error model was adopted during statistical data processing. According to this additive error model, we calculated the difference in heights of the DEM H DEM and the topographic relief H ТОРО as
Land was considered as the sum of systematic ΔH and random Δh errors:
After eliminating the systematic error from the measurement results using
the parameters of the random component Δh were estimated.
The following types of errors were calculated in the study (where n is the number of measurements):
1. Average elevation difference (systematic error), m;
2. Root mean square error, m;
3. Mean absolute error, м;
4. Standard root mean square error (Bessel’s correction), m;
5. Centered mean absolute error, m.
The methodology for delineating river basins is based on the utilization of the ArcGIS software suite in conjunction with the DEM. It encompasses a systematic algorithm comprising a series of steps executed using the “Hydrology” toolbox within the “Spatial Analyst” tool.
1. The DEM is imported into the ArcGIS software suite.
2. The “Fill” tool from the “Hydrology” toolbox in the “Spatial Analyst” toolset is employed to rectify erroneous depressions within the DEM.
3. The “Flow Direction” tool from the “Hydrology” toolbox in the “Spatial Analyst” toolset is applied to ascertain the flow direction for each pixel of the DEM, which has been preprocessed (in step 2) using the “Fill” tool.
4. The “Flow Accumulation” tool from the “Hydrology” toolbox in the “Spatial Analyst” toolset is utilized to compute the cumulative flow, representing the aggregated weight of all pixels that drain into each downslope pixel in the resulting raster. The flow direction raster created in step 3 serves as the input.
5. The “Raster Calculator” tool from the “Map Algebra” toolbox in the “Spatial Analyst” toolset is used to select pixels with a flow accumulation value exceeding 25. As a result, a new raster is generated with flow accumulation values above 25.
6. The “Stream Link” tool from the “Hydrology” toolbox in the “Spatial Analyst” toolset is employed to create a raster linear network wherein each section of the network is assigned unique values, representing individual stream links. The input rasters consist of the flow direction raster generated in step 3 and the flow accumulation raster with values exceeding 25, produced in step 5.
7. The “Stream Order” tool from the “Hydrology” toolbox in the “Spatial Analyst” toolset is utilized to assign a stream order to each link within the stream network raster created in step 6.
8. The “Basin” tool from the “Hydrology” toolbox in the “Spatial Analyst” toolset is employed to generate a raster depicting river basins based on the constructed flow direction raster (step 3).
9. The “Raster to Polygon” tool from the “Conversion” toolbox within the “From Raster” toolset is applied to transform the stream network raster obtained in step 7 into a polygon shapefile.
We supplemented and implemented the method for identifying river basins and their sub-basins using ArcGIS 10.8 software and the builtin model editor “Model Builder,” which allowed us to automate and speed up the delineation process (Figure 2). Our identification of river basins is based on a previously described method (Elkhrachy, 2018; Bajirao et al., 2019; Garrote, 2022; Samsonov, 2022). The theoretical and methodological foundations of delineating river basins and sub-basins were extensively discussed by Bai et al. (2015a) (Bai et al., 2015b). We automated it using the built-in ArcGIS Model Builder (Figure 2).
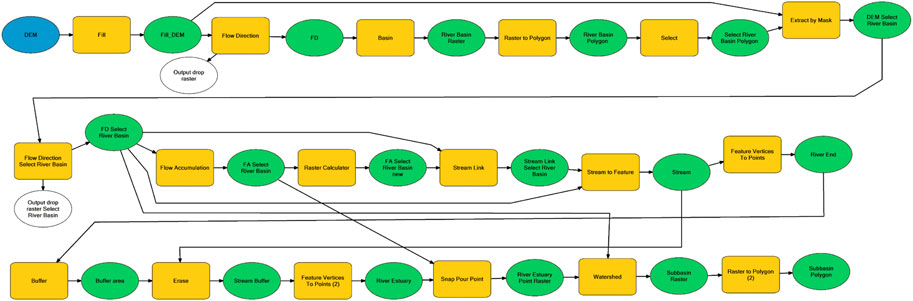
FIGURE 2. Model for identifying river basins on the northwestern slope of Crimean Mountains and their sub-basins within basins of Zapadnyy Bulganak, Alma, Kacha, Belbek, and Chernaya Rivers [compiled by us using (Samsonov, 2022)].
We note the sensitivity of this method to the incoming sets of spatial data: the type of DEM and its accuracy, as well as the accuracy of tying the points of the river mouths.
3 Results
We calculated the values of five different types of errors for the territory of the river basins of the northwestern slope of the Crimean Mountains. These values are presented in Table 1. Table 1 shows that the errors for ASTER GDEM and SRTM DEM were the largest; those of ALOS World 3D and Copernicus DEM were the smallest. Additionally, the values of errors within the basins of the Zapadnyy Bulganak, Alma, Kacha, Belbek, and Chernaya Rivers were analyzed (Table 2, Table 3, Table 4, Table 5, Table 6).
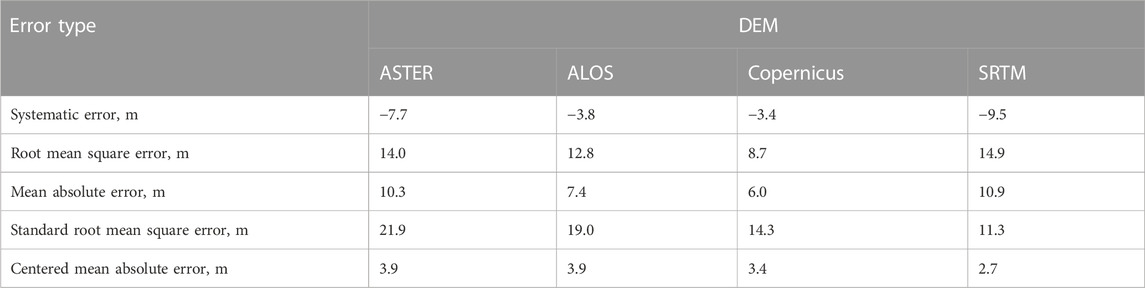
TABLE 1. Comparison of accuracy of absolute heights in study area according to a topographic map at scale of 1:100,000 for various DEM sets.
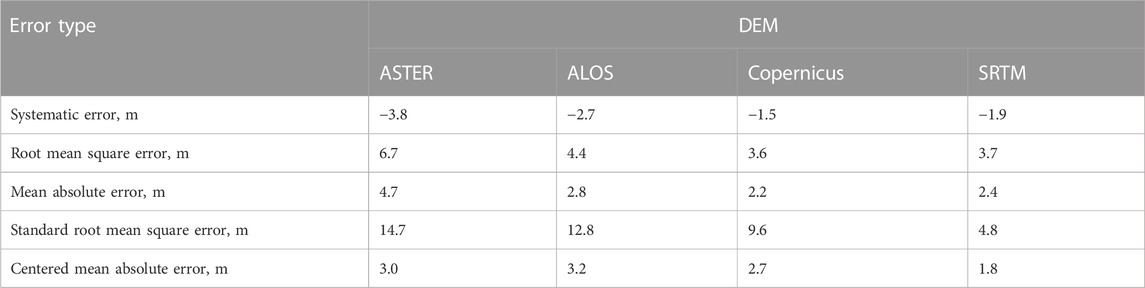
TABLE 2. Comparison of accuracy of absolute heights in Zapadnyy Bulganak River basin according to topographic map at scale of 1:100,000 for various DEM sets.
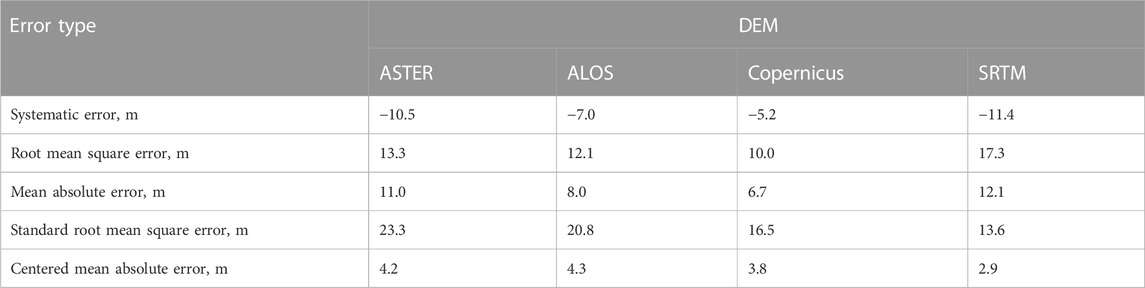
TABLE 3. Comparison of accuracy of absolute heights in Alma River basin according to topographic map at scale of 1:100,000 for various DEM sets.
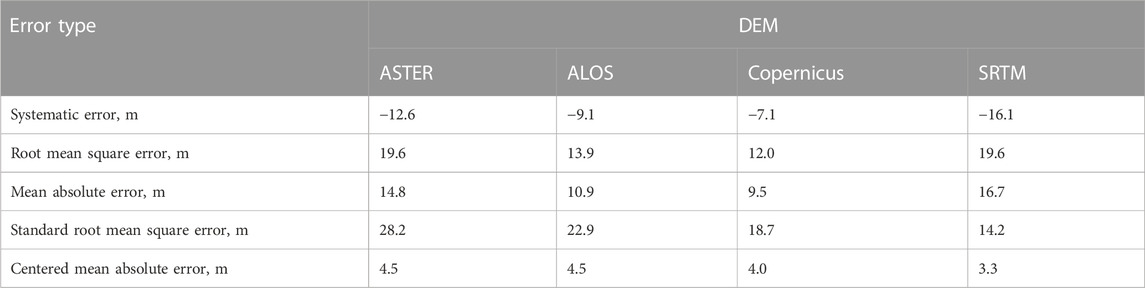
TABLE 4. Comparison of accuracy of absolute heights in Kacha River basin according to topographic map at scale of 1:100,000 for various DEM sets.

TABLE 5. Comparison of accuracy of absolute heights in Belbek River basin according to topographic map at scale of 1:100,000 for various DEM sets.
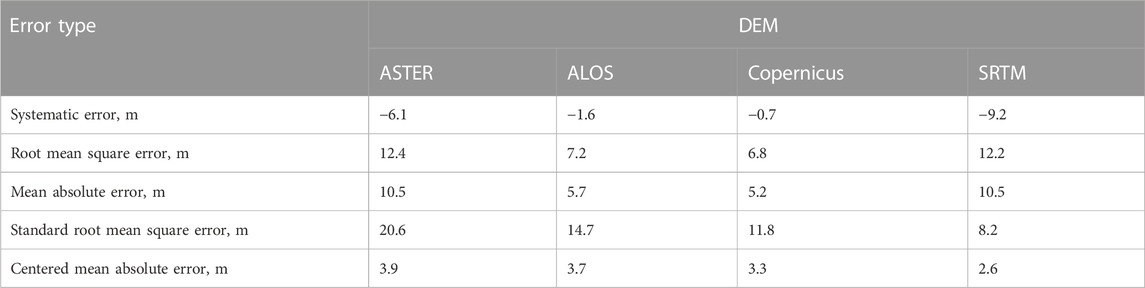
TABLE 6. Comparison of accuracy of absolute heights in Chernaya River basin according to topographic map at scale of 1:100,000 for various DEM sets.
The Copernicus DEM is most suitable for the analysis of the morphometric characteristics of the river basins of the northwestern slope of the Crimean Mountains. This DEM has a spatial resolution of 30 m/pixel.
Based on the Copernicus DEM, the boundaries of the streams sub-basins within the basins of the Zapadnyy Bulganak, Alma, Kacha, Belbek, and Chernaya Rivers were identified as a result of modeling and partial manual correction of the obtained model results (we selectively checked for the presence of errors in the boundaries of the selected sub-basins). The obtained results are shown in Figure 3.
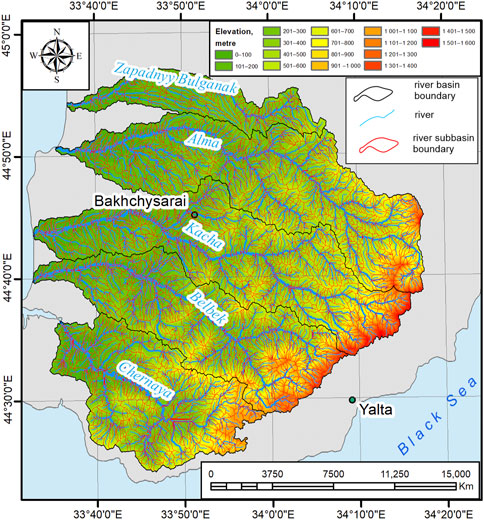
FIGURE 3. Stream sub-basins on the northwestern slope of the Crimean Mountains within the basins of the Zapadnyy Bulganak, Alma, Kacha, Belbek, and Chernaya Rivers.
Figure 3 shows that we identified 3,293 sub-basins in the study area, which form the valley, gully, and ravine systems of the study area. The basin of the Western Bulganak River has 207 sub-basins, the Alma River has 860 sub-basins, the Kacha River has 855 sub-basins, the Belbek River has 747 sub-basins, and the Chernaya River has 624 sub-basins.
4 Discussion
After analyzing the data presented in Table 1, Table 2, Table 3, Table 4, Table 5, Table 6, we found that the error values of the Copernicus DEM, which has a spatial resolution of 30 m/pixel, were the lowest. Using the same technique with different data as the input to the model may have led to the obtained results slightly differing. This may concern both the boundaries of the study region and the software products on which the data were processed. For example, if we compare our earlier calculations of the area of catchment basins using SRTM DEM (Tabunshchik, 2021a) with those obtained using Copernicus DEM and data from the literature, insignificant differences are observed. Here, on the entire-basin scale, these changes are insignificant, and the differences are mainly related to the number of points along which the outer boundaries of the river basins are drawn.
We compared our data with those calculated by researchers for other regions. Karwel and Ewiak (2008) reported that the accuracy of SRTM within the flat part of the territory of Poland is 2.9 m, and 5.4 m for mountainous and foothill areas. Calculations (Orlyankin and Aleshina, 2019) showed that within the river basins of the northwestern slope of the Crimean Mountains, the systematic error of elevation calculated from the SRTM dataset, with a spatial resolution of 90 × 90 m, is +1 m.
Mutar et al. (2021) indicated that the RMSE of Copernicus DEM is 1.3 m in Iraq, which is 2.6 times more accurate than the SRTM DEM dataset and 5.2 times more accurate than the ASTER GDEM dataset. The accuracy of the Copernicus DEM dataset in China is 6.73 m (Li et al., 2022). Santillan and Makinano-Santillan (2016) found that when comparing datasets within the Philippines, the AW3D30 dataset most accurately represents true heights compared with the SRTM and ASTER GDEM datasets, because the AW3D30 dataset has the lowest mean error, RMSE, and standard deviation. Elkhrachy (2018), for the territory of Saudi Arabia, reported that when comparing DEM and a topographic map at a scale of 1: 10,000, which was chosen as a reference, the vertical accuracy of the SRTM and ASTER datasets is ±6.87 and ±7.97 m, respectively. Dong et al. (2015) conducted an accuracy assessment of ZY-3, SRTM, DLR-SRTM, and GDEM in Northeast China. GPS data was used as the accuracy evaluation criterion for ZY-3, and the RMSE for SRTM was found to be ±2.82 m. Zhang et al. (2019) compared ASTER, SRTM, ALOS, and TanDEM-X for flood risk mapping on the island of Hispaniola, using GPS and LiDAR measurements. They found that ASTER had the highest errors, while ALOS and TanDEM-X had the lowest errors. Karabörk et al. (2021) compared AlosPalsar, Sentinel-1A, AW3D30, SRTM, and ASTER GDEM with ground control points (GCP) obtained from digital aerial photographs, photogrammetric maps, or orthophotos. They found that the mean error values for ALOS were 1.1 m on flat terrain and 8.2 m in mountainous areas, while SRTM had mean errors of 1.8 m on flat terrain and 7.9 m in mountainous areas. ASTER had mean errors of 1.0 m on flat terrain and 8.4 m in mountainous areas. Purinton and Bookhagen (2021) compared the accuracy of SRTM, ASTER, ALOS, TanDEM-X, and Copernicus DEM in the Arid Central Andes. They found that the Copernicus DEM provided the most accurate representation of the landscape and should be the preferred DEM model for topographic analysis in areas where local high-quality DEM coverage is not available.
To demonstrate the changes in the areas and morphometric characteristics of river basins, Table 7 presents the results of a comparison of the basins area of the Zapadnyy Bulganak, Alma, Kacha, Belbek, and Chernaya rivers, calculated using the SRTM DEM and Copernicus DEM datasets, as well as a comparison with the data on river basin area given in the literature.
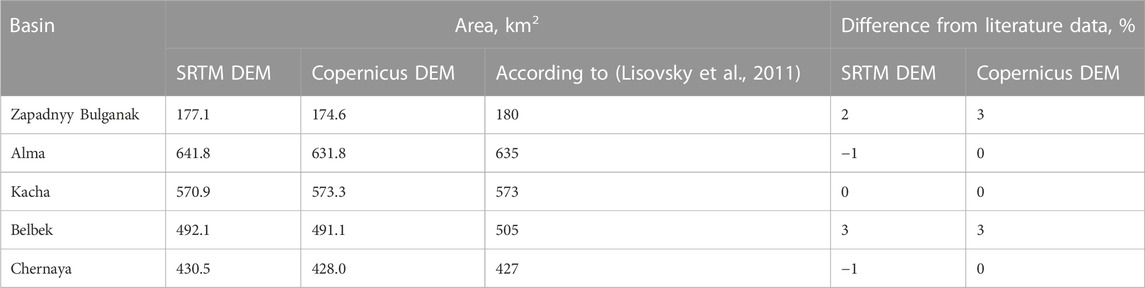
TABLE 7. Comparison of basin areas of the Zapadnyy Bulganak, Alma, Kacha, Belbek, and Chernaya Rivers.
Using GIS, both the river basins (Ermolaev et al., 2014; Ali et al., 2023; Sharma et al., 2023) and sub-basins (Vanham and Bidoglio, 2014; Dallaire et al., 2019) of large rivers can be identified. However, the low accuracy of the DEM and new techniques can often lead to distortion of the output results. As an example, consider a previously described technique (Tabunshchik, 2021b; Samsonov, 2022), which is based on the PCRaster Python Library and automated by Van der Kwast as a PCRaster Tools plugin for QGIS. The application of this technique to the river basins of the northwestern slope of the Crimean Mountains showed a rather mixed picture that defies logical classification (Figure 4).
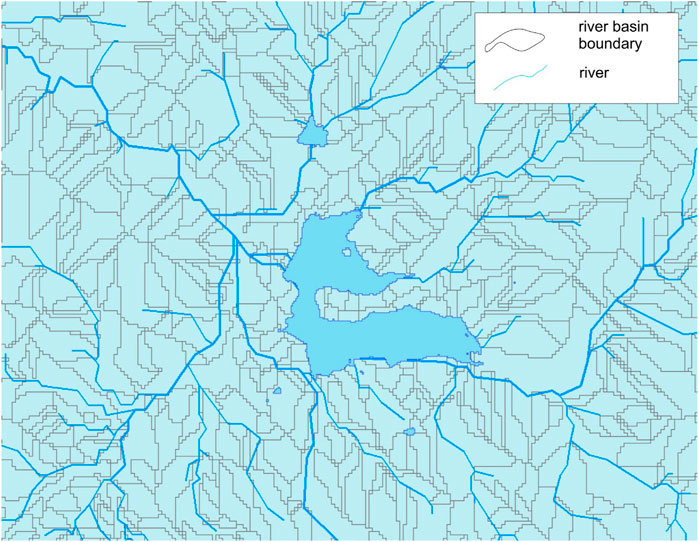
FIGURE 4. Example of the unsuccessful selection of sub-basins using PCRaster Tools plugin for QGIS on catchment basin fragment of Chernaya River.
Figure 4 shows that the sub-basins of the rivers were not identified. The resulting processing result contained many errors, and the boundaries of sub-basins were identified without considering watersheds, which indicates the impossibility of applying this method in the study area.
The method proposed by Samsonov (2022) produced the best result of modeling the sub-basins of the five largest rivers of the northwestern slope of the Crimean Mountains (Zapadnyy Bulganak, Alma, Kacha, Belbek, and Chernaya Rivers). We propose using a buffer value that exceeds twice the DEM resolution’s value to separate the points of the mouth of smaller streams flowing into the main stream. In our measurements, we found that this value should be equal to the pixel resolution, given the large error in constructing and visualizing data with a buffer size of two pixels (Figure 5).
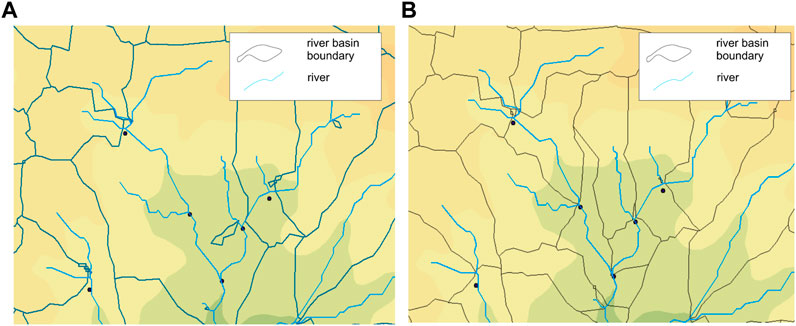
FIGURE 5. Fragment of a map of sub-basins compiled using a 60 m buffer (A), containing construction errors and 30 m (B) with minimal construction errors.
In some areas, we identified single image pixels that did not belong to catchment basins, so we then manually identified them. Additionally, the resolution of various open DEM datasets impacts the accuracy of sub-basin identification. When comparing DEM data with a resolution of 90 m/pixel with those with a resolution of 30 m/pixel, the most accurate results were achieved by the latter.
Many studies have focused on the identification of river basins using SRTM DEM for the Crimean Peninsula (Vlasova, 2012; Pozachenyuk et al., 2014; Pozachenyuk et al., 2015; Tabunshchik, 2021a; Narozhnyaya, 2021; Drygval, 2022). Other DEMs have practically not been used, which is probably due to the popularity and widespread use of SRTM DEM.
Narozhnyaya (2021) provided a detailed description of the morphometric analysis of the river basins of the Crimean Peninsula; however, the description is based on the use of SRTM DEM. A detailed description of the individual basins of the large rivers of the Crimean Peninsula was not provided, including the five basins that we considered. Narozhnyaya (2021) did not distinguish the sub-basins of the rivers: only separate maps were presented that allowed judging the distribution of certain morphometric indicators of river basins.
We identified the sub-basins within the basins of the Zapadnyy Bulganak, Alma, Kacha, Belbek, and Chernaya Rivers for the first time, and we substantially refined the available information on the quantitative characteristics of the basins of these rivers. This, in turn, creates many opportunities for researchers of the nature of the Crimean Peninsula to solve many problems with more accurate initial data. Additionally, the use of DEMs is advisable when conducting complex engineering, geological, hydrological, and hydrogeological studies. Several directions for further research of the river basins and sub-basins of the northwestern slope of the Crimean Mountains emerge from our study, in particular, their anthropogenic transformation, searching for relationships between climate change and changes in vegetation and land cover types; assessing their geoecological state; preparing landscape planning maps; and developing and implementing recommendations for sustainable development.
In further research, it is necessary to continue comparing different DEM datasets and identifying the most accurate and suitable ones for specific tasks. While the study focused on analyzing specific DEM datasets for the studied region, there is potential in future research to examine the accuracy of various DEM datasets in different regions and compare them to each other. Additionally, advancements in remote sensing technologies and data processing methods present opportunities to improve the accuracy of DEM datasets. Further research can explore the integration of data from multiple sources, such as LiDAR, satellite imagery, and ground-based measurements, to enhance the accuracy and reliability of DEMs. The use of Unmanned Aerial Vehicles (UAVs) at the local level of investigation also holds great interest.
5 Conclusion
Despite more than two centuries of hydrological studies of the rivers and river basins of the Crimean Peninsula, many unsolved problems remain. In general, the Crimean Peninsula remains insufficiently hydrologically studied. This primarily applies to important tasks such as the hydrological characteristics of rivers and the morphometric characteristics of river basins, determining the types of water management use of rivers and developing schemes for optimizing river and river basin use, studying and predicting possible ecogeodynamic processes under the influence of anthropogenic factors within river basins, studying the degree of anthropogenic transformation of the river basin, etc. Only at the end of the 20th to the beginning of the 21st century did detailed work begin on the identification and description of the basins and sub-basins of the rivers of the Crimean Peninsula, which continues to this day. However, these studies are still extremely scarce, and the data are scattered. This study of the river basins and sub-basins identifying the northwestern slope of the Crimean Mountains provides a distinctive contribution to the unresolved history of hydrological research within the Crimean Peninsula.
The use of DEMs enables the study of the main morphometric characteristics of the river basins of the northwestern slope of the Crimean Mountains. However, when choosing the initial data, the least error-prone datasets should be used. The performed calculations showed that the smallest errors in the selection of DEM were obtained for Copernicus DEM, which has a resolution of 30 m/pixel. Copernicus DEM provides a sufficiently high level of accuracy and detail, which was shown in the calculation model of the Zapadnyy Bulganak, Alma, Kacha, Belbek, and Chernaya River basins, as well as their sub-basins.
Data availability statement
The original contributions presented in the study are included in the article/supplementary material, further inquiries can be directed to the corresponding author.
Author contributions
VT conceived and designed the experiments; TG performed the experiments; VT and RG analyzed the data and contributed to the analysis tools; VT, TG, CP, AK, and RG wrote the paper, but all authors discussed the results and enhanced the final draft of the manuscript. All authors contributed to the article and approved the submitted version.
Funding
The research was conducted within the framework of the research topic “Studying the spatial and temporal organisation of aquatic and terrestrial ecosystems in order to develop an operational monitoring system based on remote sensing data and GIS technologies. Registration number: 121040100327-3.” The RUDN University Strategic Academic Leadership Program supported this research.
Acknowledgments
The authors are grateful to Makarova V.I. and Kolesnikova E.M. (Don State Public Library) for support of the study.
Conflict of interest
The authors declare that the research was conducted in the absence of any commercial or financial relationships that could be construed as a potential conflict of interest.
Publisher’s note
All claims expressed in this article are solely those of the authors and do not necessarily represent those of their affiliated organizations, or those of the publisher, the editors and the reviewers. Any product that may be evaluated in this article, or claim that may be made by its manufacturer, is not guaranteed or endorsed by the publisher.
References
Ali, F., Srisuwan, C., Techato, K., and Bennui, A. (2023). Assessment of small hydropower in songkhla lake basin, Thailand using GIS-MCDM. Sustain. Water Resour. Manag. 9 (1), 25–22. doi:10.1007/s40899-022-00788-w
Amelichev, G. N., Oliferov, A. N., and Novikova, F. N. (2017). Hydrological features of the river abdalka (simferopol) in recharge area of the plain crimea artesian basin. Sci. Notes V.I. Vernadsky Crime. Fed. Univ. Geogr. Geol. 3 (1), 160–175.
Annis, A., Nardi, F., Petroselli, A., Apollonio, C., Arcangeletti, E., Tauro, F., et al. (2020). UAV-DEMs for small-scale flood hazard mapping. Water 12, 1717. doi:10.3390/w12061717
Bai, R., Li, T. J., Huang, Y. F., Li, J. Y., and Wang, G. Q. (2015a). An efficient and comprehensive method for drainage network extraction from DEM with billions of pixels using a size-balanced binary search tree. Geomorphology 238, 56–67. doi:10.1016/j.geomorph.2015.02.028
Bai, R., Li, T. J., Huang, Y., Li, J. Y., Wang, G. Q., and Yin, D. Q. (2015b). A hierarchical pyramid method for managing large-scale high-resolution drainage networks extracted from DEM. Comput. Geosciences 85 (PA), 234–247. doi:10.1016/j.cageo.2015.06.019
Bajirao, T. S., Kumar, P. K., Kumar, P. K., Tarate, C., and Bajirao, S. (2019). Application of remote sensing and GIS for morphometric analysis of watershed: A review. IJCS 7.2, 709–713.
Balzter, H., Cole, B., Thiel, C., and Schmullius, C. (2015). Mapping CORINE land cover from sentinel-1A SAR and SRTM digital elevation model data using random forests. Remote Sens. 7, 14876–14898. doi:10.3390/rs71114876
Bodin, X., Thibert, E., Sanchez, O., Rabatel, A., and Jaillet, S. (2018). Multi-annual kinematics of an active rock glacier quantified from very high-resolution DEMs: An application-case in the French alps. Remote Sens. 10, 547. doi:10.3390/rs10040547
Courty, L. G., Soriano-Monzalvo, J. C., and Pedrozo-Acuña, A. (2019). Evaluation of open-access global digital elevation models (AW3D30, SRTM, and ASTER) for flood modelling purposes. J. Flood Risk Manag. 12, e12550. doi:10.1111/jfr3.12550
Dallaire, C. O., Lehner, B., Sayre, R., and Thieme, M. (2019). A multidisciplinary framework to derive global river reach classifications at high spatial resolution. Environ. Res. Let 14 (2), 024003. doi:10.1088/1748-9326/aad8e9
del Rosario Gonzalez-Moradas, M., and Viveen, W. (2020). Evaluation of ASTER GDEM2, SRTMv3. 0, ALOS AW3D30 and TanDEM-X DEMs for the Peruvian Andes against highly accurate GNSS ground control points and geomorphological-hydrological metrics. Remote Sens. Environ. 237, 111509. doi:10.1016/j.rse.2019.111509
Dong, Y., Chang, H. C., Chen, W., Zhang, K., and Feng, R. (2015). Accuracy assessment of GDEM, SRTM, and DLR-SRTM in northeastern China. Geocarto Int. 30 (7), 779–792. doi:10.1080/10106049.2014.985744
Drygval, A. V. (2022). “Morphometric analysis of the Shelen river basin (Southern coast of Crimea),” in Geology and water resources of Crimea (LEMA Publishing House).
Dunaieva, Y., and Kovalenko, P. (2013). River basins typification of crimea by agrolandscapes and ecological load. Sci. J. Russ. Sci. Res. Inst. Land Improv. Problems 4 (12), 157–167.
Elkhrachy, I. (2018). Vertical accuracy assessment for SRTM and ASTER digital elevation models: A case study of najran city, Saudi Arabia. Ain Shams Eng. J. 9 (4), 1807–1817. doi:10.1016/j.asej.2017.01.007
Ergina, E. I., and Timchenko, Z. V. (2016). The soil of the basin of the angara river. Sci. Notes V.I. Vernadsky Crime. Fed. Univ. Geogr. Geol. 2 (2), 88–96.
Ermolaev, O. P., Maltsev, K. A., and Ivanov, M. A. (2014). Automated construction of boundaries of basin geosystems for the Volga. Fed. Dist. Geogr. Nat. Resour. 3, 32–39.
Escobar Villanueva, J. R., Iglesias Martínez, L., and Pérez Montiel, J. I. (2019). DEM generation from fixed-wing UAV imaging and LiDAR-derived ground control points for flood estimations. Sensors 19, 3205. doi:10.3390/s19143205
Fang, G., Yuan, T., Zhang, Y., Wen, X., and Lin, R. (2019). Integrated study on soil erosion using RUSLE and GIS in yangtze River Basin of jiangsu province (China). Arabian J. Geosciences 12 (5), 173–213. doi:10.1007/s12517-019-4331-2
Fawdon, P., Gupta, S., Davis, J. M., Warner, N. H., Adler, J. B., Balme, M. R., et al. (2018). The Hypanis Valles delta: The last highstand of a sea on early Mars? Earth Planet. Sci. Let 500, 225–241. doi:10.1016/j.epsl.2018.07.040
Fischer, M., Huss, M., and Hoelzle, M. (2015). Surface elevation and mass changes of all Swiss glaciers 1980–2010. Cryosphere 9 (2), 525–540. doi:10.5194/tc-9-525-2015
Florinsky, I. V., and Filippov, S. V. (2017). A desktop system of virtual morphometric globes for Mars and the Moon. Planet. Space Sci. 137, 32–39. doi:10.1016/j.pss.2017.01.005
Garrote, J. (2022). Free global DEMs and flood modelling—a comparison analysis for the january 2015 flooding event in mocuba city (Mozambique). Water 14, 176. doi:10.3390/w14020176
Hashemi-Beni, L., Jones, J., Thompson, G., Johnson, C., and Gebrehiwot, A. (2018). Challenges and opportunities for UAV-based digital elevation model generation for flood-risk management: A case of princeville, North Carolina. Sensors 18 (11), 3843. doi:10.3390/s18113843
Karabörk, H., Makineci, H. B., Orhan, O., and Karakus, P. (2021). Accuracy assessment of DEMs derived from multiple SAR data using the InSAR technique. Arabian J. Sci. Eng. 46, 5755–5765. doi:10.1007/s13369-020-05128-8
Karlson, M., Bastviken, D., and Reese, H. (2021). Error characteristics of pan-arctic digital elevation models and elevation derivatives in northern Sweden. Remote Sens. 13, 4653. doi:10.3390/rs13224653
Karwel, A. K., and Ewiak, I. (2008). Estimation of the accuracy of the SRTM terrain model on the area of Poland. Int Archives Photogrammetry Remote Sens Spatial Inf Sci XXXVII (7), 169–172.
Kayukova, E. P., (2016). Using hydrochemical data to estimate the components of the water balance (the Bodrak river basin, southwestern Crimea). Ser. 7. Geol. Geogr 4, 25–36. Vestnik of Saint Petersburg University. doi:10.21638/11701/spbu07.2016.403
Kramm, T., and Hoffmeister, D. (2021)., 37. Germany, 7774–7799. Comprehensive vertical accuracy analysis of freely available DEMs for different landscape types of the Rur catchment, GermanyGeocarto Int. doi:10.1080/10106049.2021.1984588
Krivoguz, D. O. (2016). The role of hydrological processes in the processes of landslide formation of the Kerch Peninsula. Yekaterinburg, Russia: Aeterna Limited Liability Company.
Lehner, B., and Grill, G. (2013). Global river hydrography and network routing: Baseline data and new approaches to study the world’s large river systems. Hydrol. Process. 27 (15), 2171–2186. doi:10.1002/hyp.9740
Li, H., Zhao, J., Yan, B., Yue, L., and Wang, L. (2022). Global DEMs vary from one to another: An evaluation of newly released Copernicus, NASA and AW3D30 DEM on selected terrains of China using ICESat-2 altimetry data. Int. J. Digital Earth 15 (5), 1149–1168. doi:10.1080/17538947.2022.2094002
Lisovsky, A. A., Novik, V. A., Timchenko, Z. V., and Gubskaya, U. A. (2011). Surface water bodies of the Crimea. Management and use of water resources. Krymuchpedgiz Publishing House.
Liu, Y., Gong, W., Hu, X., and Gong, J. (2018). Forest type identification with random forest using sentinel-1A, sentinel-2A, multi-temporal landsat-8 and DEM data. Remote Sens. 10, 946. doi:10.3390/rs10060946
Manune, D. F. (2007). Digital elevation model. Technologies and applications: The DEM users manual. Bethesd: American Society for Photogrammetry and Remote Sensing.
Mesa-Mingorance, J. L., and Ariza-López, F. J. (2020). Accuracy assessment of digital elevation models (DEMs): A critical review of practices of the past three decades. Remote Sens. 12 (16), 2630. doi:10.3390/rs12162630
Mutar, A. Q., Mustafa, M. T., and Hameed, M. A. (2021). The impact of (DEM) Accuracy on the Watersheds areas as a function of spatial data. Periodicals Eng. Nat. Sci. (PEN) 9 (4), 1118–1130. doi:10.21533/pen.v9i4.2585
Narozhnyaya, A. G. (2021). Morphometric analysis of the Crimean river basins relief by using GIS. InterCarto. InterGIS. 27 (2), 218–232. doi:10.35595/2414-9179-2021-2-27-218-232
Nikolakopoulos, K. G., Kamaratakis, E. K., and Chrysoulakis, N. (2006). SRTM vs ASTER elevation products. Comparison for two regions in Crete, Greece. Int. J. Remote Sens. 27, 4819–4838. doi:10.1080/01431160600835853
Onkov, I. (2011). Evaluation of SRTM height accuracy for the orthotransformation of high resolution satellite images. Geomatics 3, 40–46.
Orlyankin, V. N., and Aleshina, A. R. (2019). The use of matrixes of heights srtm in the preliminary calculations and mapping of depths and potential flood inundation of fluvial plain. Issled. Zemli iz Kosmosa 5, 72–81. doi:10.31857/S0205-96142019572-81
Pozachenyuk, E. A., Ergina, E. I., Oliferov, A. N., Mikhailov, V. A., Vlasova, A. N., Kudrjan', E. A., et al. (2014). Analysis of factors of the Salgir River's water resources formation under the condition of climate changing. Sci. Notes V.I 2, 118–138.
Pozachenyuk, E. A., Lisetskii, F. N., Vlasova, A. N., Buryak, Z. A., and Marinina, O. A., (2015). Model of position-dynamic structure of river basins. Res. J. Pharm. Biol. Chem. Sci. 6, 1776–1780.
Pozachenyuk, E. A. (2009). “Modern landscapes of the crimea and adjacent water areas,” in 19th International Multidisciplinary Scientific GeoConference SGEM 2019, At: Albena, Bulgaria.
Purinton, B., and Bookhagen, B. (2021). Beyond vertical point accuracy: Assessing inter-pixel consistency in 30 m global dems for the arid central andes. Front. Earth Sci. 9, 758606. doi:10.3389/feart.2021.758606
Pyankov, S. V., and Shikhov, A. N. (2017). Geoinformation support for modeling hydrological processes and phenomena. Perm State National Research University.
Rajasekhar, M., Raju, G. S., Raju, R. S., Ramachandra, M., and Kumar, B. P. (2018). Data on comparative studies of lineaments extraction from ASTER DEM, SRTM, and Cartosat for Jilledubanderu River basin, Anantapur district, AP, India by using remote sensing and GIS. Data brief 20, 1676–1682. doi:10.1016/j.dib.2018.09.023
Samsonov, T. E. (2022). Fundamentals of geoinformatics: Practicum. Moscow, Russia: Faculty of Geography of Moscow State University. doi:10.5281/zenodo.1167857
Santillan, J. R., and Makinano-Santillan, M. (2016). Vertical accuracy assessment of 30-m resolution ALOS, ASTER and SRTM global DEMS over Northeastern Mindanao, Philippines. XXIII ISPRS Congress. Comission IV. Int. Archives Photogrammetry Remote Sens. Spatial Inf. Sci. 41 (B4), 149–156. doi:10.5194/isprsarchivesXLI-B4-149-2016
Sarkar, D., Mondal, P., Sutradhar, S., and Sarkar, P. (2020). Morphometric analysis using SRTM-DEM and GIS of nagar River Basin, indo-Bangladesh barind tract. J. Indian. Soc. Remote Sens. 48, 597–614. doi:10.1007/s12524-020-01106-7
Sharma, P., Singh, M. M., Chaurasia, R. S., and Sabir, M. (2023). Remote sensing and GIS based approach in morphometric analysis of birma River Basin (central India). Sustain. Agri, Food Environ. Res. 11. doi:10.7770/safer-V11N1-art2387
Tabunshchik, V. A. (2021b). Estimation of stream power index (SPI) in the River Basins of the north-western slopes of the Crimean Mountains (River Basins Zapadnyy Bulganak, Alma, Kacha, Belbek, Chernaya). Geopolit. i ecogeodinamika Reg. 7 (2), 344–354.
Tabunshchik, V. A. (2021a). Morphometric characteristics of River Basins of the northwestern slope of the Crimean Mountains (Zapadnyy Bulganak, Alma, Kacha, Belbek, Chernaya River Basins). Sci. Notes V.I. Vernadsky Crime. Fed. Univ. Geogr. Geol. 7 (3), 267–278. doi:10.37279/2413-1717-2021-7-3-267-278
Tabunshchik, V. A. (2018). Relief of river basins of the north-western slope of the Crimean Mountains (river basins Zapadnyy Bulganak, Alma, Kacha, Belbek, Chernaya). Geopolit. ecogeodynamics regions 4 (3), 78–87.
Tabunshchik, V., Gorbunov, R., and Gorbunova, T. (2022). Anthropogenic transformation of the River Basins of the northwestern slope of the Crimean Mountains (the crimean Peninsula). Land 11, 2121. doi:10.3390/land11122121
Tadono, T., Nagai, H., Ishida, H., Oda, F., Naito, S., Minakawa, K., et al. (2016). Generation of the 30 M-mesh global digital surface model by ALOS PRISM. International Archives of the Photogrammetry. Remote Sens. Spatial Inf. Sci. 41 (XLI-B4), 157–162. doi:10.5194/isprs-archives-XLI-B4-157-2016
Tang, Q., and Lettenmaier, D. P. (2012). 21st century runoff sensitivities of major global river basins. Geophys. Res. Let 39 (6), L06403. doi:10.1029/2011GL050834
Thomas, J., and Prasannakumar, V. (2015). Comparison of basin morphometry derived from topographic maps, ASTER and SRTM DEMs: An example from Kerala, India. Geocarto Int. 30 (3), 346–364. doi:10.1080/10106049.2014.955063
Timchenko, Z. V. (2000). Assessment of the geoecological state of water resources of small rivers (on the example of small rivers of the northern macroscline of the Crimean Mountains), V.I. Simferopol: Vernadsky Taurida National University.
Timchenko, Z. V., Tabunshchik, V. A., and Zelentsova, M. G. (2020). The characteristics of the dzhankoy region rivers and dzhankoy town okrug of the republic of the crimea. IOP Conf. Ser. Earth Environ. Sci. 548 (5), 052038. doi:10.1088/1755-1315/548/5/052038
Trofimov, A. A., and Filippova, A. V. (2014). Assessment of the accuracy of the SRTM DEM based on topographic surveys. Geoprofi 6, 13–17.
Uuemaa, E., Ahi, S., Montibeller, B., Muru, M., and Kmoch, A. (2020). Vertical accuracy of freely available global digital elevation models (ASTER, AW3D30, MERIT, TanDEM-X, SRTM, and NASADEM). Remote Sens. 12 (21), 3482. doi:10.3390/rs12213482
Uysal, M., Toprak, A. S., and Polat, N. (2015). DEM generation with UAV Photogrammetry and accuracy analysis in Sahitler hill. Measurement 73, 539–543. doi:10.1016/j.measurement.2015.06.010
Vanham, D., and Bidoglio, G. (2014). The water footprint of agricultural products in European river basins. Environ. Res. Let 9 (6), 064007. doi:10.1088/1748-9326/9/6/064007
Vermaat, J. E., Broekx, S., Van Eck, B., Guy, E., Hellmann, F., Jean Luc De Kok, , et al. (2012). Nitrogen source apportionment for the catchment, estuary, and adjacent coastal waters of the river scheldt. Ecol. Soc. 17 (2), 30. doi:10.5751/ES-04889-170230
Viel, J. A., Rosa, K. K. D., and Mendes Junior, C. W. (2020). Avaliação da Acurácia Vertical dos Modelos Digitais de Elevação SRTM, ALOS World 3D e ASTER GDEM: Um Estudo de Caso no Vale dos Vinhedos, RS – brasil. Rev. Bras. Geogr. Física 13 (5), 2255–2268. doi:10.26848/rbgf.v13.5.p2255-2268
Vlasova, A. N. (2012). The management of river basins and the Black Sea coastal zone by landscape planning instruments (The Crimea, the Voron river basin as an example). Turkish J. Fish. Aquatic Sci. 12 (5), 535–537.
Vlasova, A. (2011). The estimation of ecological state of the Salgir river basin. Sci. Notes V.I. Vernadsky Taurida Natl. Univ. Geogr. 24 (1), 66–71.
Yamazaki, D., Ikeshima, D., Tawatari, R., Yamaguchi, T., O'Loughlin, F., Neal, J. C., et al. (2017). A high-accuracy map of global terrain elevations. Geophys. Res. Let 44 (11), 5844–5853. doi:10.1002/2017GL072874
Yeritsian, H. H. (2013). Comparison of the DEMS generated from 1:50000, 1:100000 and 1:200000 topo-maps with the SRTM DEM data. Proc. NAS RA - Earth Sci. 66 (1), 39–47.
Yermolaev, O., Mukharamova, S., and Vedeneeva, E. (2021). River runoff modeling in the European territory of Russia. Catena 203, 105327. doi:10.1016/j.catena.2021.105327
Yuan, F., Repse, M., Leith, A., Rosenqvist, A., Milcinski, G., Moghaddam, N. F., et al. (2022). An operational analysis ready radar backscatter dataset for the african continent. Remote Sens. 14, 351. doi:10.3390/rs14020351
Zhang, K., Gann, D., Ross, M., Robertson, Q., Sarmiento, J., Santana, S., et al. (2019). Accuracy assessment of ASTER, SRTM, ALOS, and TDX DEMs for Hispaniola and implications for mapping vulnerability to coastal flooding. Remote Sens. Environ. 225, 290–306. doi:10.1016/j.rse.2019.02.028
Keywords: GIS, digital elevation model, ASTER GDEM, ALOS World 3D, Copernicus DEM, SRTM, river, river basin
Citation: Tabunshchik V, Gorbunov R, Gorbunova T, Pham CN and Klyuchkina A (2023) Identification of river basins within northwestern slope of Crimean Mountains using various digital elevation models (ASTER GDEM, ALOS World 3D, Copernicus DEM, and SRTM DEM). Front. Earth Sci. 11:1218823. doi: 10.3389/feart.2023.1218823
Received: 08 May 2023; Accepted: 30 June 2023;
Published: 13 July 2023.
Edited by:
Jie Luo, Zhejiang Lab, ChinaReviewed by:
Jiaye Li, Dongguan University of Technology, ChinaHasan Bilgehan Makineci, Konya Technical University, Türkiye
Ahmed Sefelnasr, United Arab Emirates University, United Arab Emirates
Copyright © 2023 Tabunshchik, Gorbunov, Gorbunova, Pham and Klyuchkina. This is an open-access article distributed under the terms of the Creative Commons Attribution License (CC BY). The use, distribution or reproduction in other forums is permitted, provided the original author(s) and the copyright owner(s) are credited and that the original publication in this journal is cited, in accordance with accepted academic practice. No use, distribution or reproduction is permitted which does not comply with these terms.
*Correspondence: Vladimir Tabunshchik, tabunshchyk@ibss-ras.ru