- 1Collaborative Innovation Center for Western Ecological Safety, College of Atmospheric Sciences, Lanzhou University, Lanzhou, China
- 2Shandong Climate Center, Jinan, China
- 3MOE Key Laboratory of Mechanics on Disaster and Environment in Western China, Department of Geological Engineering, Lanzhou University, Lanzhou, China
Rain-induced loess landslides are especially prevalent in the Chinese Loess Plateau (CLP). Some became fatal landslide disasters, leading to numerous casualties and significant socioeconomic losses. Extreme precipitation is the main cause of landslide occurrence. Therefore, in this study we discuss the correlation between seven extreme precipitation indices, single continuous precipitation events and fatal landslides in the CLP using Pearson correlation analysis. We also predict future precipitation under climate changes using five optimal CMIP6 models. During the period 2004–2016, fatal landslides in the CLP increased at a rate of 0.6 per year, with frequent landslide events occurring especially in the central and southwestern parts of the CLP. We find that SDII (simple daily intensity precipitation index) and R
1 Introduction
Global warming changes the water and energy system, which greatly contributed to the natural disasters in the past century and resulted in losses of life and millions in economic cost. These disasters include floods, droughts, hurricanes, and secondary disasters such as fatal landslides, heat waves and so on. For the direct disasters, their variability induced by global warming has received much attention (Johnson et al., 2018; Zhao et al., 2018). Wang et al. (2017) suggest that a warming of 0.5°C leads to significant increases in extreme temperature and precipitation events in most regions. However, the secondary disasters are harder to predict than the direct disasters, because they are triggered by direct disasters and influenced by multiple factors. Recent studies have demonstrated that there are physical cascading mechanisms between direct and secondary disasters, such as heat and forest fires, drought and heat waves, floods and landslides (AghaKouchak et al., 2018; Ren and Leslie, 2020; Carnicer et al., 2022). Quantifying the coupled risks arising from direct and secondary disasters is beneficial for risk prediction and loss reduction.
Landslides, as a typical secondary disaster, are always characterized by fast process, low predictability, and large losses of life, which have a strong impact on human society. The occurrence of landslides is generally associated with extreme rainfall (Crozier, 2005; Kirschbaum et al., 2012; Ren et al., 2014). When extreme rainfall inflow on a slope is faster than the outflow, that is, the infiltration rate is greater than the exfiltration rate, the water content and pore water pressure in the soil increase, and the strength of the soil decreases, leading to landslide events (Van Asch et al., 1999; Crozier, 2010). Previous studies have showed an increasing trend of fatal landslides globally, causing 156,268 deaths and 177,537 injuries from 1995–2014, with precipitation-induced landslides accounting for 85% of these events (Haque et al., 2019). Obviously this number does not include remote areas where fatal landslides occurred but were not reported and recorded. Many researchers found a highly correlated relationship between landslide events and extreme precipitation in several countries, such as Portugal, Italy, India, South Korea, and China (Wen et al., 2004; Peruccacci et al., 2017; Bhardwaj et al., 2019; Kim et al., 2021; Araujo et al., 2022). China, one of the countries suffering from heavy landslide casualties, accounts for more than half of the landslides due to extreme precipitation events, which is among the three main factors that cause fatal landslides: extreme precipitation, urbanization, and over-exploitation (Zhang et al., 2023). Therefore, determining precipitation threshold is a common means of predicting landslides that are not generated by earthquakes. Since Caine (1980) established global threshold for landslide triggering using precipitation intensity (I) and precipitation duration (D), more and more researchers have used physical or empirical methods to determine global or regional thresholds by precipitation intensity (I) and precipitation duration (D), and some choose to consider cumulative event precipitation (E) and precipitation duration (D) as independent variables for defining thresholds (Ran et al., 2018; Huang et al., 2022; Zhou et al., 2022). In addition, extreme precipitation tends to increase globally under global warming, and previous studies have confirmed that future landslide events can be predicted on the basis of future changes in precipitation in different climate scenarios (Araujo et al., 2022).
Among the regions prone to landslides in the world, the Chinese Loess Plateau (CLP) region has a fragile geological environment, with a unique combination of loess gully landforms and valley landforms such as loess tableland, loess ridges and hills. Furthermore, loess has large pore spaces, vertical joint development, high collapsibility and erodibility, and a very fragmented surface (Xu et al., 2008; Zhang and Li, 2011; Peng and Duan, 2018). Therefore, the loess structure and high water sensitivity are the endogenous causes of frequent geological hazards in the area. Geological hazards in the CLP occur throughout the year; landslides and loess mudslides occur frequently in up to 30% of Gansu Province (Wang et al., 2020). Lei. (2001) proposed that precipitation and human activities account for 30% and 23% of all loess landslides, respectively. According to statistics of landslides on the CLP by Xu et al. (2017), rainfall-induced landslides accounted for 40% of the 53 fatal landslides that caused 717 deaths during 1980–2015, indicating that rainfall was a direct indicator of landslides in the CLP. In recent years, studies on landslides and precipitation in the CLP focused on the mechanism of landslides triggered by precipitation and the role of precipitation in typical landslide events, and almost all of these discussions revolved around small areas of the CLP. Zhou et al. (2019) proposed that the main effect of rainfall is to fill a layer of loess through pores and fissures, which makes the water content and pore pressure in the CLP increase, and the subsequent decrease in effective stress reduces the landslide resistance of the slope. Qiu et al. (2020) considered the triggering factors of landslide events in Shaanxi and found that most of the non-earthquake-induced landslide events in Shaanxi were triggered by long-time pre-precipitation and short-term daily precipitation. By studying a landslide process in Gansu, Wang et al. (2020) pointed out that the occurrence of continuous precipitation can induce the resurgence of landslides caused by historical earthquakes. With wetting in West China in recent years (Yang and Li, 2008; Shang et al., 2019), more extreme weather and other catastrophic events will become commonplace, thus research into the various secondary hazards associated with precipitation is urgently needed. However, almost all these studies did not separately consider the fatal landslide events that caused the death and disappearance of many people. There are still relatively few studies quantitatively linking fatal landslides and precipitation in the entire CLP region. It remains unclear whether future landslide events will increase under global warming or not. Therefore, in this article we focus on the response of fatal landslides to precipitation in the CLP region under global warming.
The remainder of this paper is presented below. In Section 2, we introduce the study area and data. In Section 3, we introduce methods. We analyze the spatial and temporal distribution of fatal landslides as well as annual mean precipitation for the period 2004–2016, the association of landslides with extreme and continuous precipitation, and future changes in extreme precipitation in Section 4. The summary and discussion are presented in Section 5.
2 Study area and data
2.1 Overview of the study area
In this paper, the main body of the CLP (101°-114°E, 34°-41°N) is chosen as our study area (Figure 1). The CLP is located at a northern latitude in the middle reach of the Yellow River. It covers a region more than 1,000 km from east to west and 750 km from north to south, including a vast area west of the Taihang Mountains, east of the Riyue Mountains in Qinghai Province, north of the Qinling Mountains, and south of the Great Wall, spanning Shanxi, Shaanxi and Ningxia, including parts of Inner Mongolia, Gansu, Qinghai, and Henan. Its total area is approximately 630,000 km2, accounting for ∼6.6% of China’s landmass (Liu, 1985). With a population of more than 200 million, the CLP is the base of numerous forestry, agricultural and livestock industries (Lei. 2001). It has semi-arid and semi-humid climate. Its vegetation ecosystem shows a clear regional variation from northwest to southeast; and its ecosystem mainly includes desert, grassland, shrubs and forests, etc (Zhang et al., 2022). This fragile ecological environment is highly susceptible to soil erosion. Therefore, there are three geomorphological structures in the CLP: platform, ridge and dome. The geological structures are complex, mainly including bedrock, mudstone, river and lake strata, Wucheng loess, Lishi loess, Malan loess, and recently deposited loess (Peng et al., 2019b).
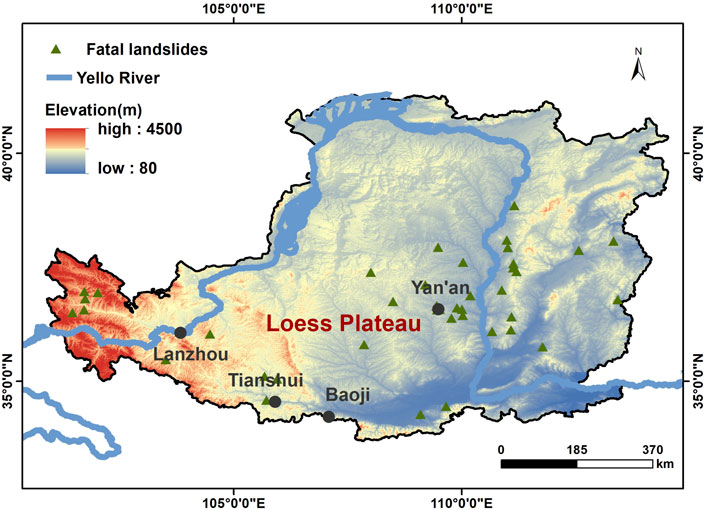
FIGURE 1. Geographical location, elevation map and distribution of fatal landslide sites in the CLP.
The thickness of loess deposition varies from a few meters to 400 m (Derbyshire et al., 2000; Xu et al., 2007). Moreover, the terrain is highly undulating with alternating rivers, tablelands, crisscrossed gullies and ravines. In addition, many studies showed that loess has unique mechanical properties, large porosity, vertical joints, loose texture, and sensitivity to suction stress (Dijkstra et al., 1995; Derbyshire et al., 2000; Xu et al., 2007; Zhang et al., 2009; Zhang and Liu, 2010) and is a typical type of unsaturated soil (Fredlund and Rahardjo, 1993; Nouaouria et al., 2008; Xia and Han, 2009; Muñoz-Castelblanco et al., 2011; Muñoz-Castelblanco et al., 2012). Therefore, the unique environmental characteristics in the CLP make it extremely sensitive to precipitation, earthquakes, vegetation and human activities, resulting in frequent occurrences of landslides and other catastrophic events. As mentioned above, there is a close relationship between precipitation and landslides. Factors other than precipitation also need our attention. Some landslides in the CLP originate from earthquakes, which are in the seismic sensitive zone and cause soil liquefaction due to earthquakes (Zhang and Wang, 2007; Wang et al., 2014). At the same time, the influence of vegetation on landslides is reflected by the fact that the increase of vegetation reduces pore pressure and the reinforcement of roots can improve the strength of soil (Guo et al., 2020; Luo et al., 2023). Therefore, bare vegetation is more prone to trigger landslides. Human activities are another important influencing factor for landslides. In recent years, irrigation and engineering activities have increased the susceptibility to catastrophic events in the CLP. Irrigation makes the groundwater level rise, which increases pore pressure and destabilizes the slope, thus contributing to the occurrence of landslides (Lian et al., 2020). Human activities such as excavation and mining can also weaken the stability of slopes and even revive old landslides, leading to frequent occurrence of catastrophic events in the CLP, resulting in a large number of casualties (Li et al., 2014; Peng et al., 2019a; Tang et al., 2020).
2.2 Data
The precipitation data used in this paper are from the CN05.1 dataset provided by the Climate Change Research Center of the Chinese Academy of Sciences (CAS-CCRC, https://ccrc.iap.ac.cn/resource/detail?id=228), which is obtained by the anomaly app-roach method with the interpolation and superposition of the climate field and the anomaly field (Wu and Gao, 2013), using observations from more than 2,400 stations in China. The data first included daily mean temperature, maximum and minimum temperature, and precipitation, and later added three variables-humidity, wind speed, and surface evaporation (Wu et al., 2017). Among them, the daily precipitation data have a resolution of 0.25° and the time range is 1961–2021. The data have high resolution and long-time scale, which is suitable for comparative analysis of model simulation results. In this paper, we mainly use these data from 1980 to 2021 for the calculation of extreme precipitation indices and the comparison of model simulation results.
To analyze the characteristics of landslide change, we use data on non-earthquake-induced fatal landslides provided by Zhang and Huang (2018). Zhang and Huang (2018) collected the data on landslides from China’s geological environment information site (CGEIS, http://www.cigem.gov.cn) and Ministry of Natural Resources of China (MNR, http://www.mnr.gov.cn) from 2004 to 2016, including the type of hazards, triggering mechanism, approximate locations, economic losses, and casualties. Since almost all casualties or fatalities were from landslides, they organized the specific quantity of landslide events that resulted in casualties in different geographical areas and provinces and total losses of fatal landslides and excluded earthquake-induced landslide events. It is worth noting that the fatal landslides are landslide events that result in the death or disappearance of people.
Regarding simulating and predicting the frequency of continuous precipitation for historical and future periods, we used the daily precipitation data from the Coupled Model Intercomparison Project Phase 6 (CMIP6). We selected 25 models covering daily precipitation data for the historical simulations (1980–2014) and the SSP1-2.6 and SSP5-8.5 simulations (2015–2100) for comparative analysis (Table 1). The data available at https://esgf-node.llnl.gov/search/cmip6/. Since the resolutions of these models differ, the bilinear interpolation method was used to interpolate the models to a uniform resolution of (0.25° × 0.25°).
3 Methodology
3.1 Definition of extreme precipitation indices
In this paper, we use the extreme precipitation index recommended by the Expert Team on Climate Change Detection and Indices (ETCCDI) (etccdi.pacificclimate.org/list_27_indices.shtml), which is available for the analysis of extreme precipitation and for climate change studies. In our study, we considered the duration, intensity and frequency of precipitation to select seven of these extreme precipitation indicators to study the effects on fatal landslides. In operational forecasting in China, a precipitation event with daily precipitation ≥50 mm is called torrential rain, and a precipitation event with daily precipitation between 25 and 50 mm is called heavy rain. Because of the uneven distribution of precipitation in the CLP, considering that the sample size satisfied by considering the effects of extreme precipitation with 50 mm as the criterion for heavy rainfall is too small, in the paper, we have chosen a criterion of 25 mm to discuss the effects of heavy rainfall on fatal landslides. The method to define the threshold of extreme precipitation events at each station (Zhai and Pan, 2003) is that the samples of daily precipitation series from 1981 to 2010 are arranged in an ascending order for each station. The 30-year average of the 95th (99th) percentile of subsamples with daily precipitation
All extreme precipitation indices used in the paper are included in the following table (Table 2).
3.2 CMIP6 modes introduction
The Coupled Model Intercomparison Project (CMIP), which was initiated and organized by the WCRP Working Group on Coupled Modeling (WGCM), is an international program to compare coupled models. Its objective is to compare the performances of global coupled climate models, which has evolved to “advance model development and improve scientific understanding of the Earth’s climate system” with the rapid development of coupled sea-air models (Zhou et al., 2019). In this paper, we use historical precipitation simulations from the Historical experiment, in addition to daily precipitation results from the ScenarioMIP, a subprogram of 23 models from various countries approved by the CMIP6. The two extreme emission scenarios, SSP1-2.6 and SSP5-8.5, were selected for our study. SSP stands for socioeconomic pathway, with SSP1-2.6 representing the combined effects of low vulnerability, low mitigation pressure and low radiative forcing, and SSP5-8.5 considering higher CO2 emissions and representing the development of high fossil fuel consumption. Thirty-three institutions are involved in the development of the CMIP6 models. By eliminating models with incomplete precipitation data in the historical phase and in SSP1-2.6 and SSP5-8.5 scenarios, we finally retained 25 CMIP6 models. Institutions and resolutions of the 25 models are shown in Table 1.
3.3 Statistical parameters for model performance evaluation
To evaluate the simulation capability of the CMIP6 model for extreme precipitation indices, we considered statistical indicators on spatial and temporal scales. Among them, the spatial correlation coefficient (
The closer the RMSE is to 0, the better the mode simulation capability.
On the time scale,
The above equation reflects the formula for the linear trend equation, where
4 Results
4.1 Spatial and temporal distribution of fatal landslides and annual mean precipitation
The CLP, as one of the oldest loess, is the birthplace of the ancient civilization of the Chinese nation. The development of culture illustrated its suitability for human living. The CLP is typical with loess soil, with weak water conservation. A new study shows that the annual accumulated precipitation has an abrupt increase since 2000 (Huang et al., 2022). In the high stage of annual precipitation, it shows the CLP region became wetter (Figure 2A), and the annual mean precipitation increased by 14.38 mm/year, which is consistent with previous studies and proves that there is a trend of wetting in Northwest China (Yang and Li, 2008; Shang et al., 2019). Additionally, the annual mean precipitation dropped to its lowest (402.4 mm) in 2005 and reached its highest (506.8 mm) in 2013. With the wetting of the CLP, fatal landslides from 2004 to 2016 increased by 0.6 events/year. It is worth noting that before 2007, only one fatal landslide occurred, which was in 2004, but after 2007, the occurrence of fatal landslides became more frequent and peaked in 2013, when 17 fatal landslides occurred over the CLP.
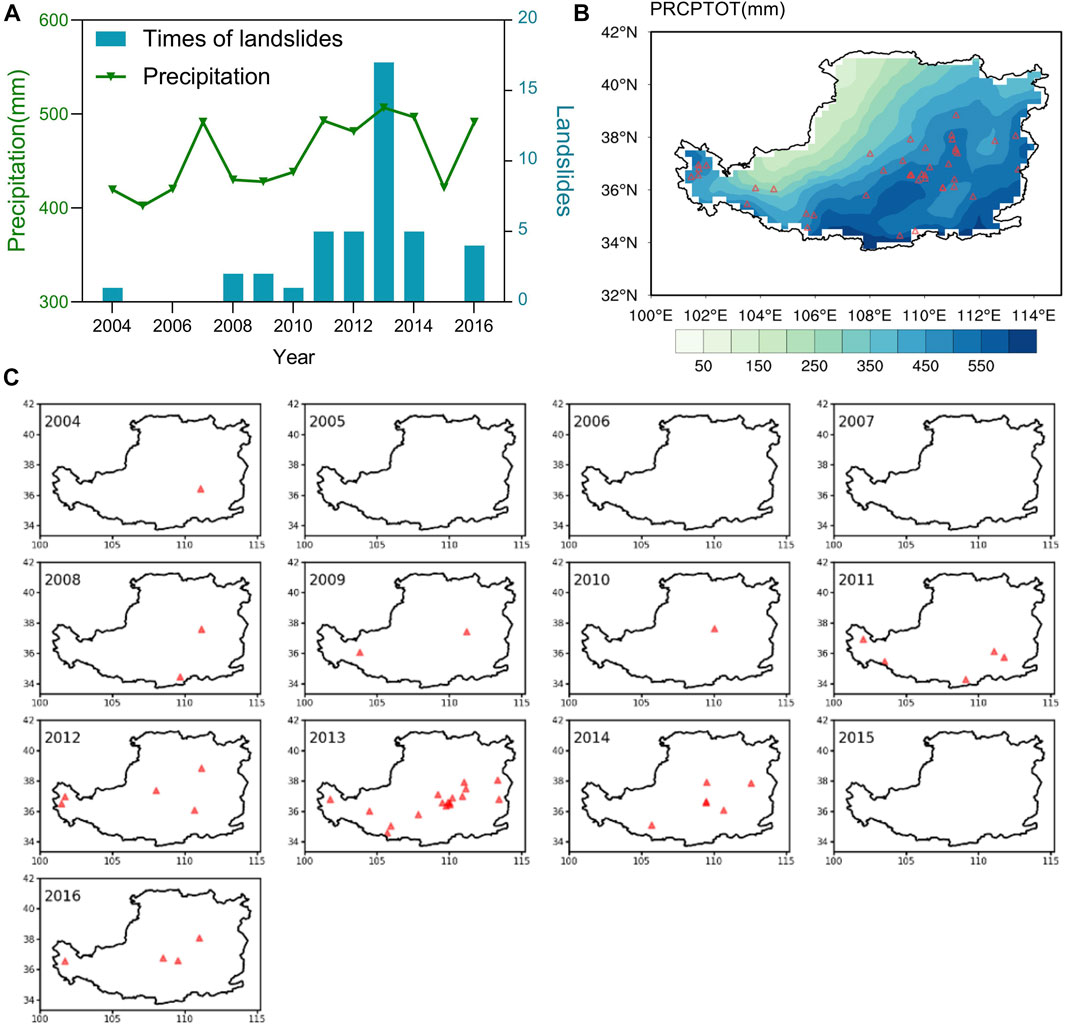
FIGURE 2. Spatial and temporal distribution of fatal landslides and annual mean precipitation in the CLP region. (A) Time series of the number of fatal landslides and annual precipitation from 2004 to 2016. (B) Distribution of fatal landslides and annual mean precipitation from 2004 to 2016. Red triangles are the locations of fatal landslides. (C) Spatial distribution of fatal landslide events from 2004 to 2016.
Precipitation is uneven in spatial distribution with significant regional differences in the CLP. As seen from the spatial distribution of fatal landslides and annual mean precipitation from 2004 to 2016 (Figure 2B), precipitation over the CLP decreased from southeast to northwest, with the highest annual mean precipitation of 810.22 mm occurring in the southeastern part of the CLP, and the lowest of 122.21 mm occurring in the northwestern part. The fatal landslides were mainly distributed in the central and southwestern CLP (Shaanxi and Gansu), especially the central part; and no fatal landslides occurred in the northwestern part, where precipitation was scarce, from 2004 to 2016. Overall, landslides occur more frequently in places with high annual precipitation. However, annual precipitation greater than 600 mm is mainly in the southernmost part of the CLP, but the location of frequent fatal landslides is mainly concentrated in the central part (Figure 2B).
The evolution of the spatial distribution of fatal landslide events from 2004 to 2016 (Figure 2C) reveals location changes of fatal landslide events during those 13 years. The landslide that occurred in 2004 was concentrated in the southeastern CLP. After 2008, fatal landslides occurred more frequently, and most of them were distributed in the southeastern and southwestern areas. The number of fatal landslides peaked in 2013, with a spatial distribution from the southwestern to the eastern and central part of the CLP. The number of sites where landslides occurred showed a decreasing trend with a spatially sparse distribution as a result of the sharp drop in precipitation in 2015 (Figure 2A).
4.2 Effects of extreme precipitation on fatal landslides
Previous landslide model tests on loess areas showed that rainfall has obvious effect on the soil water content in loess areas, up to approximately 3 m below the surface (Tu et al., 2009; Zhang et al., 2012), and that precipitation significantly changes the matrix suction in the upper part of the loess but barely affects that in the lower part (Huang and Qi, 2004). Thus, the landslides caused by short, intense rainfall are mostly shallow landslides (Li et al., 2008; Zhuang et al., 2014). With these results, we analyzed the explicit relationship between extreme rainfall and fatal landslides. We considered the frequency, intensity and duration of precipitation and investigated the effect of precipitation on fatal landslides. By analyzing the spatial distribution of anomalies of the six extreme precipitation indices from 2004 to 2016 based on the period from 1981 to 2010, we find that SDII is almost positive anomaly except for a small part of the western CLP where there is obvious negative anomaly (Figure 3A). The range of value of SDII anomalies is between −0.5 and 0.5 mm/day. The distribution of R95pTOT is similar to that of SDII (Figure 3B), but the difference is that R95pTOT exhibits a smaller range of positive anomalies than SDII, with negative anomaly in the southwest of the CLP. The maximum anomaly value is located in the middle east of the CLP, and the average R95pTOT from 2004 to 2006 is 40 mm more than the average in the base period. The distribution of R25mm is basically identical to that of CWD and R
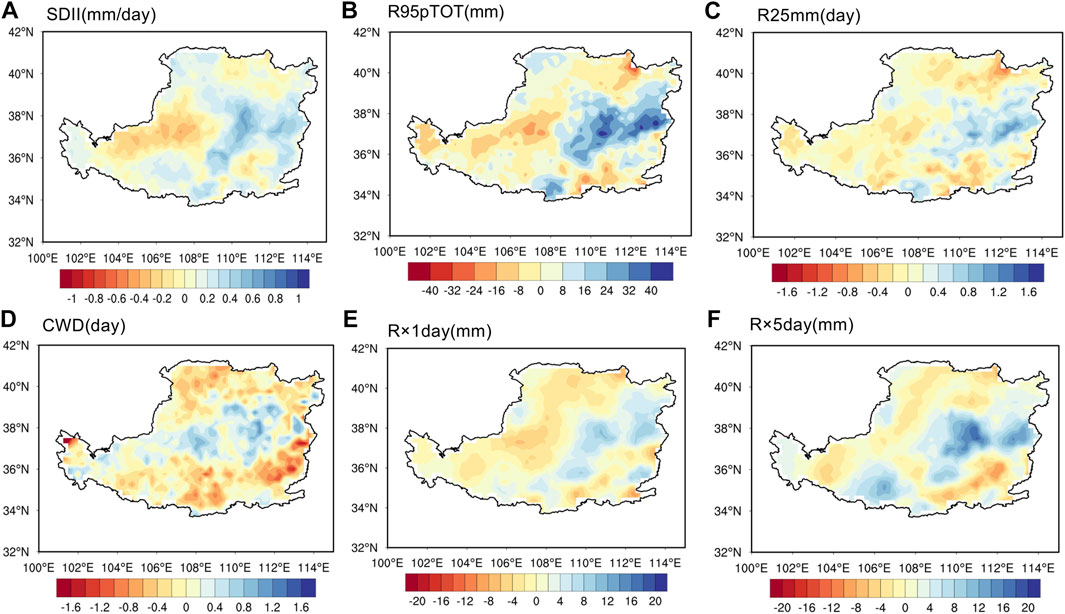
FIGURE 3. Spatial anomalous distribution of SDII (A), R95pTOT (B), R25mm (C), CWD (D), R
From the time series distribution of the six extreme precipitation indices in 1980–2021, we can find that SDII, R95pTOT, R25mm, R
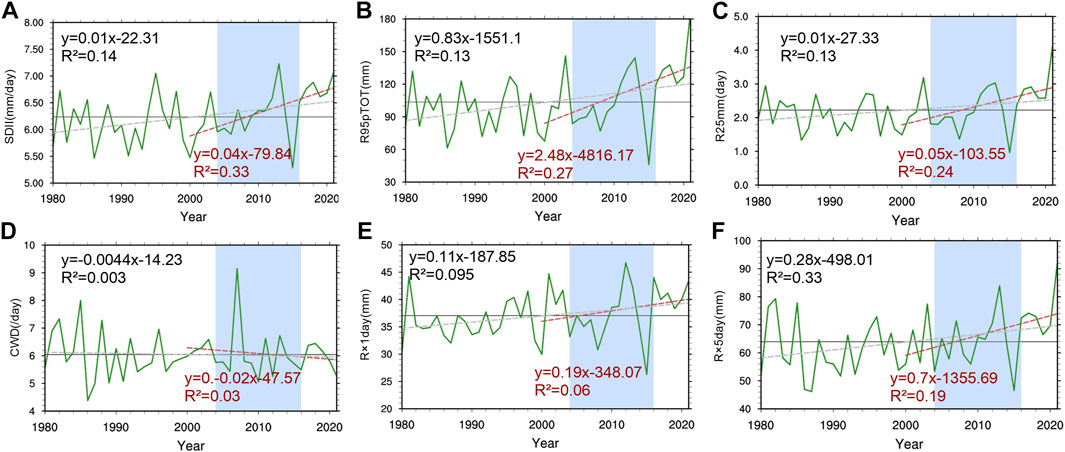
FIGURE 4. Time series distribution of SDII (A), R95pTOT (B), R25mm (C), CWD (D), R
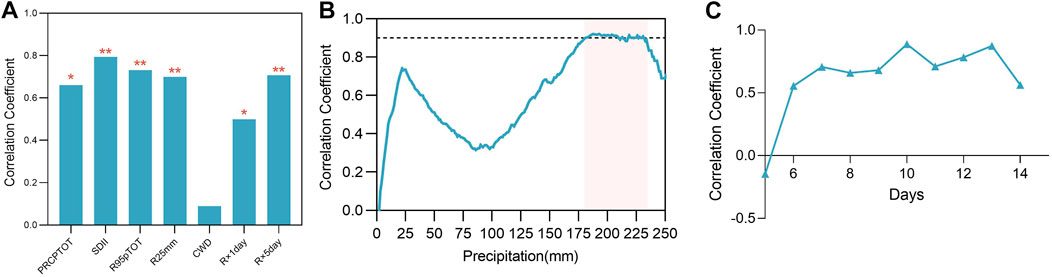
FIGURE 5. Effects of extreme and continuous precipitation events on landslides (A) The correlation coefficient between fatal landslides and extreme precipitation index. “*” represents passes 0.05 confidence level based on Student’s t-test. “**” represents passes 0.01 confidence level based on Student’s t-test. (B) Correlation coefficient between precipitation events with different cumulative precipitation and fatal landslides. (C) Correlation coefficients between cumulative precipitation events of different durations for 185–235 mm and fatal landslides.
It has been indicated that deep landslides are mainly influenced by long, antecedent effective precipitation (Li et al., 2008), and the reduction of negative pore pressure in dry soils by antecedent precipitation is a precondition for strong rainfall to induce landslides (Ma et al., 2014). Similarly, Tu et al. (2009) found that long, continuous, light rainfall is more likely to induce loess landslides than short, heavy rainfall. Zhuang and Peng (2014) investigated a loess landslide event caused by long rainfall, and found that long rainfall led to multiple landslides at the same location; and after the first landslide occurred, cracks formed at the rear edge of the landslide, after which more precipitation filled the cracks and induced large landslides (Zhang and Li, 2011).
From the above study we have found that fatal landslides have a better correlation with R
4.3 Changes of sensitive precipitation by anthropogenic effects
Based on the above analysis, we found that SDII has a high correlation with fatal landslides in both spatial and temporal distributions, therefore, we analyzed the spatial and temporal variation of SDII in the CLP under SSP1-2.6 and SSP5-8.5 scenarios, and then predicted future landslide events. Previously, we calculated
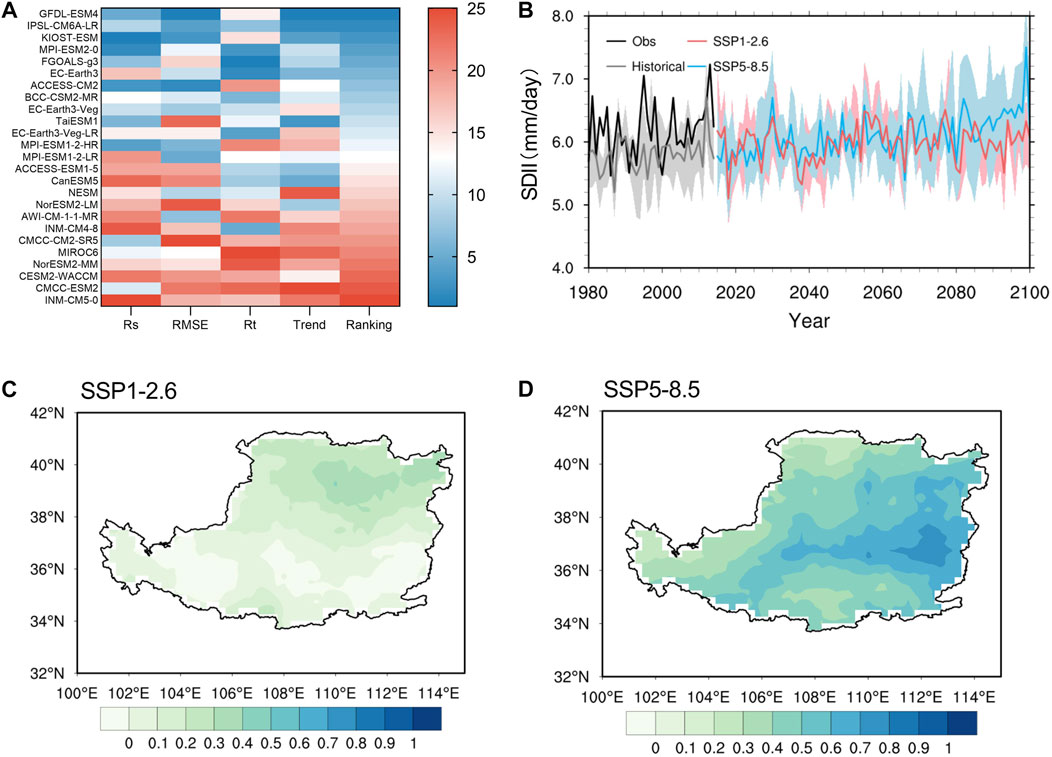
FIGURE 6. Prediction of future SDII (A)
The simulation results for SDII showed that GFDL-ESM4, IPSL-CM6A-LR, KIOST-ESM, MPI-ESM2-0, and FGOAL-g3 performed the best and ranked in the top five. It shows that the above five models can better simulate the spatial and temporal distribution characteristics of SDII. Therefore, we calculate the ensemble mean of the historical simulation results of the above five optimal models. The results of the ensemble averaging are better than those of the individual models, with
Similarly, we investigated the future changes of R
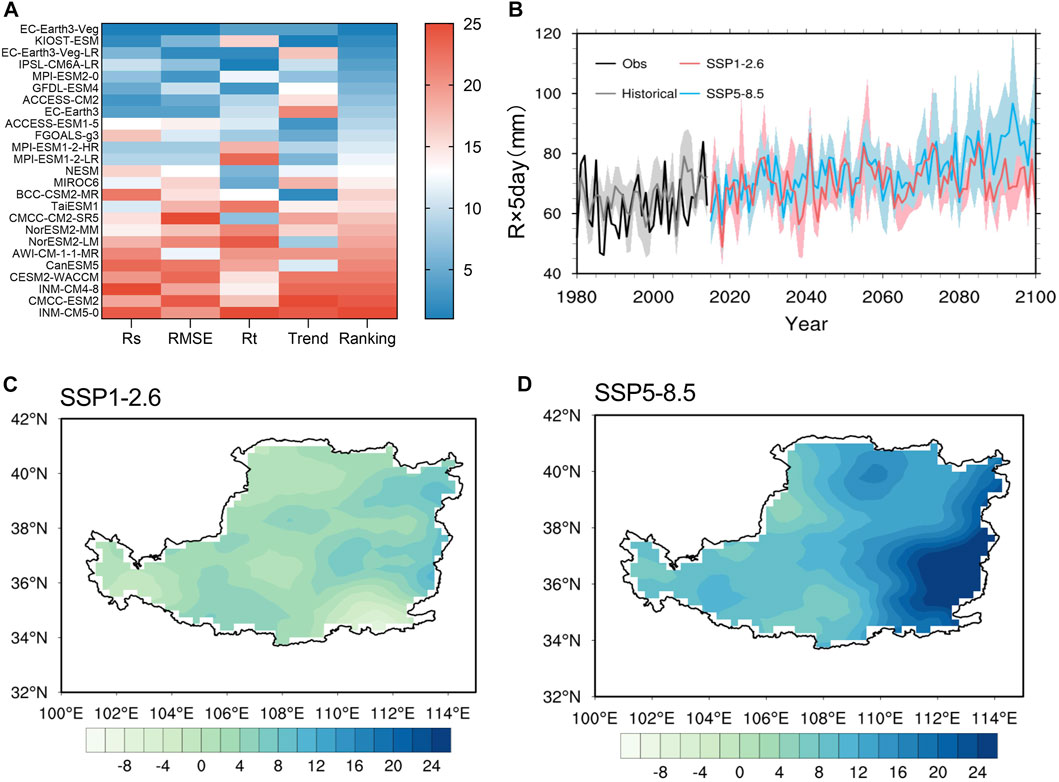
FIGURE 7. Same as Figure 6, except that the extreme precipitation index studied is R
5 Discussion
In this paper, we consider the relationship between extreme precipitation (including continuous precipitation) and fatal landslides during 2004–2016; and find that SDII and R×5day are well correlated with fatal landslides, a finding that suggests that fatal landslides in the CLP are mainly caused by continuous precipitation events. Sun et al. (2021) stated that rainfall intensity affects the time scale of development of slope deformation damage. When a heavy rainfall occurs suddenly, the safety factor of the slope decreases rapidly; as the rainfall continues, the safety factor decreases to 1.05 by the sixth day, and the rainfall penetrates into the interior of the slope, which is exacerbated by the initial rainfall, thus causing the slope to lose stability and resulting in landslide events (Zhou et al., 2019). By comparing the correlation between precipitation and landslides with different cumulative precipitation and duration, we find that the correlation was stable above 0.5 when the precipitation amount reached 185–235 mm and the duration of precipitation is 6 days or longer, which is consistent with previous findings. On 21 July 2013, the initial effective rainfall in 7 days for the landslide in Tianshui, Gansu Province was 239 mm, which caused significant casualties and losses (Peng et al., 2015). Moreover, the pre-cumulative precipitation of the landslide event that caused casualties in the Nanxiaohegou Basin in 2018 also reached 232.2 mm with a duration of more than 10 days (Luo et al., 2023).
Under global warming, different regions of the world have experienced different degrees of increase in annual precipitation and, notably, in extreme precipitation events. As atmospheric water resources grow and the changes of dynamic circulation become stronger (Pendergrass et al., 2017), extreme precipitation events occur more frequently especially in the late 21st century. For such a unique terrain as the CLP, the increase in extreme and cumulative precipitation will significantly increase the frequency of fatal landslides, leading to a serious threat to the local economy and human life, especially in the central CLP. This is relatively consistent with the findings of previous studies. Lin et al. (2022) suggested that the extent of landslide occurrence would increase under the influence of climate change, and the frequency of reaching the precipitation threshold for landslides would increase significantly in the future, especially in the northwestern region.
Therefore, the results obtained in this study provide better insight into the link between heavy precipitation events and fatal landslides, which can help local governments adopt relevant policies to actively avoid disaster risks. However, more work is needed to study the response of global warming precipitation to landslides. The prediction of future precipitation relies on different climate models, and the uncertainty of model prediction needs to be further verified for the reliability of future precipitation trends. Human activities enhance the probability of occurrence of extreme precipitation, but it is still a challenge to quantify the contribution of anthropogenic emissions and urbanization. In addition, there are multiple factors triggering the occurrence of fatal landslides in the CLP; we can only predict landslides simply by using rainfall thresholds. In fact, precipitation is only one key factor leading to landslides. In recent years, landslide events due to loess excavation and irrigation deserve equal attention. With the implementation of policies such as the western development, many engineering projects in the CLP have caused serious soil erosion; and road construction and over-mining have further increased the risk for disasters in the area. According to Yang et al. (2020), reference evapotranspiration will increase in most regions of Northwest China in the future, which may exacerbate plant water stress and thus lead to more widespread anthropogenic irrigation and indirectly induce landslides. In addition, it is worth noting that another factor leading to the high incidence of landslides in the western CLP may be related to sparse vegetation and weak root protection in the CLP, which are actually indirectly regulated by low precipitation. These issues need further research.
6 Conclusion
The CLP as a landslide-prone area has landslides that cause casualties almost every year. Many studies pointed out the great influence of short, heavy precipitation and antecedent precipitation on landslide events over the region. In this study, we found that fatal landslides occurred mainly in the central and southwestern CLP (Shaanxi and Gansu). Fatal landslides showed an increasing trend of 0.6 events/year on average from 2004 to 2016.
It was found that the effect of extreme precipitation on fatal landslides was more significant compared to the annual mean precipitation. On the spatial scale, the multi-year average results of multiple extreme precipitation indices from 2004–2016 in the central CLP showed positive anomalies compared to the climatic average, reflecting that the key to the frequency of fatal landslides in the central CLP is the increase in extreme precipitation events. Meanwhile, in the southwestern CLP, R
By analyzing the future changes of SDII and R×5day under SSP1-2.6 and SSP5-8.5 scenarios, we found that the risk of extreme precipitation events will further increase under the global warming, especially under the high emission scenario, which is mainly reflected in the central and southeastern CLP. This could assist in the prediction of future landslides.
Data availability statement
Publicly available datasets were analyzed in this study. This data can be found here: http://www.mnr.gov.cn http://www.cigem.gov.cn https://ccrc.iap.ac.cn/resource/detail?id=228 https://esgf-node.llnl.gov/search/cmip6/.
Author contributions
XG proposed the general idea of the manuscript and draft of manuscript. WS and XK: Methodology. WS: Wrote the first draft of the manuscript and visualization. XK performed the statistical analysis. YH: Supervised and edited the manuscript. FZ and JH gave the resources. All authors contributed to the article and approved the submitted version.
Funding
This work was supported by the National Science Foundation of China (42041004 and 42041006) and the Fundamental Research Funds for the Central Universities (No. lzujbky-2022-ct06).
Acknowledgments
The authors thank CAS-CCRC for daily precipitation data, FZ and XH for fatal landslide data, and also CMIP6 for daily precipitation data from their website at https://esgf-node.llnl.gov/search/cmip6/. Thanks to the help of all the reviewers and editors. The authors also thank all the institutions that provided the data for this research.
Conflict of interest
The authors declare that the research was conducted in the absence of any commercial or financial relationships that could be construed as a potential conflict of interest.
Publisher’s note
All claims expressed in this article are solely those of the authors and do not necessarily represent those of their affiliated organizations, or those of the publisher, the editors and the reviewers. Any product that may be evaluated in this article, or claim that may be made by its manufacturer, is not guaranteed or endorsed by the publisher.
Supplementary material
The Supplementary Material for this article can be found online at: https://www.frontiersin.org/articles/10.3389/feart.2023.1146724/full#supplementary-material
References
AghaKouchak, A., Huning, L. S., Chiang, F., Sadegh, M., Vahedifard, F., Mazdiyasni, O., et al. (2018). How do natural hazards cascade to cause disasters? Nature 561 (7724), 458–460. doi:10.1038/d41586-018-06783-6
Araujo, J. R., Ramos, A. M., Soares, P. M. M., Melo, R., Oliveira, S. C., and Trigo, R. M. (2022). Impact of extreme rainfall events on landslide activity in Portugal under climate change scenarios. Landslides 19 (10), 2279–2293. doi:10.1007/s10346-022-01895-7
Ayar, P. V., Vrac, M., and Mailhot, A. (2021). Ensemble bias correction of climate simulations: Preserving internal variability. Sci. Rep. 11 (1), 3098. doi:10.1038/s41598-021-82715-1
Bhardwaj, A., Wasson, R. J., Ziegler, A. D., Chow, W. T. L., and Sundriyal, Y. P. (2019). Characteristics of rain-induced landslides in the Indian himalaya: A case study of the mandakini catchment during the 2013 flood. Geomorphology 330, 100–115. doi:10.1016/j.geomorph.2019.01.010
Bhatia, U., and Ganguly, A. R. (2019). Precipitation extremes and depth-duration-frequency under internal climate variability. Sci. Rep. 9, 9112. doi:10.1038/s41598-019-45673-3
Caine, N. (1980). The rainfall intensity-duration control of shallow landslides and debris flows. Geogr. Ann. 62A, 23–27. doi:10.2307/520449
Carnicer, J., Alegria, A., Giannakopoulos, C., Di Giuseppe, F., Karali, A., Koutsias, N., et al. (2022). Global warming is shifting the relationships between fire weather and realized fire-induced CO2 emissions in Europe. Sci. Rep. 12 (1), 10365. doi:10.1038/s41598-022-14480-8
Crozier, M. J. (2010). Deciphering the effect of climate change on landslide activity: A review. Geomorphology 124 (3), 260–267. doi:10.1016/j.geomorph.2010.04.009
Crozier, M. J. (2005). Multiple-occurrence regional landslide events in New Zealand: Hazard management issues. Landslides 2 (4), 247–256. doi:10.1007/s10346-005-0019-7
Derbyshire, E., Meng, X., and Dijkstra, T. A. (2000). Landslides in the thick loess terrain of north-west China. New York: John Wiley and Sons Ltd. doi:10.1046/j.1365-2451.2002.03438.x
Dijkstra, T. A., Rogers, C. D. F., and van Asch, T. W. J. (1995). “Cut slope and terrace edge failures in Malan loess, Lanzhou, PR China,” in Proceedings of the XI ECSMFE Conference, Copenhagen, 28 May - 1 June 1995, 61–67.
Fredlund, D. G., and Rahardjo, H. (1993). Soil mechanics for unsaturated soils. New York: John Wiley and Sons. doi:10.1002/9780470172759
Guo, W., Chen, Z., Wang, W., Gao, W., Guo, M., Kang, H., et al. (2020). Telling a different story: The promote role of vegetation in the initiation of shallow landslides during rainfall on the Chinese Loess Plateau. Geomorphology 350, 106879. doi:10.1016/j.geomorph.2019.106879
Haque, U., da Silva, P. F., Devoli, G., Pilz, J., Zhao, B. X., Khaloua, A., et al. (2019). The human cost of global warming: Deadly landslides and their triggers (1995-2014). Sci. Total Environ. 682, 673–684. doi:10.1016/j.scitotenv.2019.03.415
Huang, F., Chen, J., Liu, W., Huang, J., Hong, H., and Chen, W. (2022). Regional rainfall-induced landslide hazard warning based on landslide susceptibility mapping and a critical rainfall threshold. Geomorphology 408, 108236. doi:10.1016/j.geomorph.2022.108236
Huang, R., and Qi, G. (2004). Observation of suction in a landslide. Chin. J. Geotechnical Eng. 26 (2), 216–219. (in Chinese).
Huang, X., Guan, X., Zhu, K., Gu, T., Huang, J., and He, Y. (2022). Influence of water vapor influx on interdecadal change in summer precipitation over the source area of the Yellow River Basin. Atmos. Res. 276, 106270. doi:10.1016/j.atmosres.2022.106270
Johnson, N. C., Xie, S., Kosaka, Y., and Li, X. (2018). Increasing occurrence of cold and warm extremes during the recent global warming slowdown. Nat. Commun. 9, 1724. doi:10.1038/s41467-018-04040-y
Kim, S. W., Chun, K. W., Kim, M., Catani, F., Choi, B., and Seo, J. I. (2021). Effect of antecedent rainfall conditions and their variations on shallow landslide-triggering rainfall thresholds in South Korea. Landslides 18 (2), 569–582. doi:10.1007/s10346-020-01505-4
Kirschbaum, D., Adler, R., Adler, D., Peters-Lidard, C., and Huffman, G. (2012). Global distribution of extreme precipitation and high-impact landslides in 2010 relative to previous years. J. Hydrometeorol. 13 (5), 1536–1551. doi:10.1175/Jhm-D-12-02.1
Lei, X. (2001). Geo-hazards in Loess Plateau and human activity. Beijing: Science Press, 258–264. (in Chinese).
Li, C., Ma, T., and Zhu, X. (2008). Forecasting of landslides triggered by rainfall: Theory, methods and applications. Beijing: Geology Press. (in Chinese).
Li, P., Qian, H., and Wu, J. (2014). Environment: Accelerate research on land creation. Nature 510, 29–31. doi:10.1038/510029a
Lian, B., Peng, J., Zhan, H., Huang, Q., Wang, X., and Hu, S. (2020). Formation mechanism analysis of irrigation-induced retrogressive loess landslides. Catena 195, 104441. doi:10.1016/j.catena.2019.104441
Lin, Q., Steger, S., Pittore, M., Zhang, J., Wang, L., Jiang, T., et al. (2022). Evaluation of potential changes in landslide susceptibility and landslide occurrence frequency in China under climate change. Sci. Total Environ. 850, 158049. doi:10.1016/j.scitotenv.2022.158049
Luo, L., Guo, W., Tian, P., Liu, Y., Wang, S., and Luo, J. (2023). Unique landslides (loess slide-flows) induced by an extreme rainstorm in 2018 on the Loess Plateau: A new geological hazard and erosion process. Int. J. Sediment Res. 38 (2), 228–239. doi:10.1016/j.ijsrc.2022.07.009
Ma, S., Zheng, A., and Li, C. (2014). Mechanism of rainfall-induced landslides and its implications to landslide prediction. Bull. Sci. Technol. 1, 39–43. doi:10.13774/j.cnki.kjtb.2014.01.050
Muñoz-Castelblanco, J. A., Delage, P., Pereira, J. M., and Cui, Y. (2011). Some aspects of the compression and collapse behaviour of an unsaturated natural loess. Géotechnique Lett. 1, 17–22. doi:10.1680/geolett.11.00003
MuñOz-Castelblanco, J. A., Pereira, J. M., Delage, P., and Cui, Y. (2012). The water retention properties of a natural unsaturated loess from Northern France. Géotechnique 62, 95–106. doi:10.1680/geot.9.P.084
Nouaouria, M. S., Guenfoud, M., and Lafifi, B. (2008). Engineering properties of loess in Algeria. Eng. Geol. 99 (1-2), 85–90. doi:10.1016/j.enggeo.2008.01.013
Pendergrass, A. G., Knutti, R., Lehner, F., Deser, C., and Sanderson, B. M. (2017). Precipitation variability increases in a warmer climate. Sci. Rep. 7, 17966. doi:10.1038/s41598-017-17966-y
Peng, J., and Duan, Z. (2018). Landslides in the words of little loess-grain. Chin. J. Nat. 40 (04), 285–289. (in Chinese).
Peng, J., Fan, Z., Wu, D., Huang, Q., Wang, Q., Zhuang, J., et al. (2019). Landslides triggered by excavation in the Loess Plateau of China: A case study of middle pleistocene loess slopes. J. Asian Earth Sci. 171, 246–258. doi:10.1016/j.jseaes.2018.11.014
Peng, J., Fan, Z., Wu, D., Zhuang, J., Dai, F., Chen, W., et al. (2015). Heavy rainfall triggered loess-mudstone landslide and subsequent debris flow in Tianshui, China. Eng. Geol. 186, 79–90. doi:10.1016/j.enggeo.2014.08.015
Peng, J., Wang, S., Wang, Q., Zhuang, J., Huang, W., Zhu, X., et al. (2019). Distribution and genetic types of loess landslides in China. J. Asian Earth Sci. 170, 329–350. doi:10.1016/j.jseaes.2018.11.015
Peruccacci, S., Brunetti, M. T., Gariano, S. L., Melillo, M., Rossi, M., and Guzzetti, F. (2017). Rainfall thresholds for possible landslide occurrence in Italy. Geomorphology 290, 39–57. doi:10.1016/j.geomorph.2017.03.031
Pimonsree, S., Kamworapan, S., Gheewala, S. H., Thongbhakdi, A., and Prueksakorn, K. (2022). Evaluation of CMIP6 GCMs performance to simulate precipitation over Southeast Asia. Atmos. Res. 282, 106522. doi:10.1016/j.atmosres.2022.106522
Qiu, H., Cui, Y., Pei, Y., Yang, D., Hu, S., Wang, X., et al. (2020). Temporal patterns of nonseismically triggered landslides in Shaanxi Province, China. Catena 187, 104356. doi:10.1016/j.catena.2019.104356
Ran, Q., Hong, Y., Li, W., and Gao, J. (2018). A modelling study of rainfall-induced shallow landslide mechanisms under different rainfall characteristics. J. Hydrology 563, 790–801. doi:10.1016/j.jhydrol.2018.06.040
Ren, D., and Leslie, L. M. (2020). Climate warming enhancement of catastrophic southern California debris flows. Sci. Rep. 10 (1), 10507. doi:10.1038/s41598-020-67511-7
Ren, D., Leslie, L. M., and Lynch, M. J. (2014). Trends in storm-triggered landslides over southern California. J. Appl. Meteorology Climatol. 53 (2), 217–233. doi:10.1175/Jamc-D-12-0253.1
Rivera, J. A., and Arnould, G. (2020). Evaluation of the ability of CMIP6 models to simulate precipitation over Southwestern South America: Climatic features and long-term trends (1901–2014). Atmos. Res. 241, 104953. doi:10.1016/j.atmosres.2020.104953
Shang, H., Xu, M., Zhao, F., and Tijjani, S. B. (2019). Spatial and temporal variations in precipitation amount, frequency, intensity, and persistence in China, 1973-2016. J. Hydrometeorol. 20 (11), 2215–2227. doi:10.1175/Jhm-D-19-0032.1
Shiferaw, A., Tadesse, T., Rowe, C., and Oglesby, R. (2018). Precipitation extremes in dynamically downscaled climate scenarios over the greater horn of africa. Atmosphere 9 (3), 112. doi:10.3390/atmos9030112
Sun, P., Wang, H., Wang, G., Li, R., Zhang, Z., and Huo, X. (2021). Field model experiments and numerical analysis of rainfall-induced shallow loess landslides. Eng. Geol. 295, 106411. doi:10.1016/j.enggeo.2021.106411
Tang, Y., Feng, F., Guo, Z., Feng, W., Li, Z., Wang, J., et al. (2020). Integrating principal component analysis with statistically-based models for analysis of causal factors and landslide susceptibility mapping: A comparative study from the Loess Plateau area in Shanxi (China). J. Clean. Prod. 277, 124159. doi:10.1016/j.jclepro.2020.124159
Tu, X., Kwong, A. K. L., Dai, F., Tham, L. G., and Min, H. (2009). Field monitoring of rainfall infiltration in a loess slope and analysis of failure mechanism of rainfall-induced landslides. Eng. Geol. 105 (1-2), 134–150. doi:10.1016/j.enggeo.2008.11.011
Van Asch, T. W. J., Buma, J., and Van Beek, L. P. H. (1999). A view on some hydrological triggering systems in landslides. Geomorphology 30 (1-2), 25–32. doi:10.1016/S0169-555x(99)00042-2
Wang, G., He, Y., Zhang, B., Wang, X., Cheng, S., Xie, Y., et al. (2022). Historical evaluation and projection of precipitation phase changes in the cold season over the Tibetan Plateau based on CMIP6 multimodels. Atmos. Res. 281, 106494. doi:10.1016/j.atmosres.2022.106494
Wang, G., Zhang, D., Furuya, G., and Yang, J. (2014). Pore-pressure generation and fluidization in a loess landslide triggered by the 1920 haiyuan earthquake, China: A case study. Eng. Geol. 174, 36–45. doi:10.1016/j.enggeo.2014.03.006
Wang, H., Sun, P., Zhang, S., Han, S., Li, X., Wang, T., et al. (2020). Rainfall-induced landslide in loess area, northwest China: A case study of the changhe landslide on september 14, 2019, in Gansu province. Landslides 17 (9), 2145–2160. doi:10.1007/s10346-020-01460-0
Wang, Z., Lin, L., Zhang, X., Zhang, H., Liu, L., and Xu, Y. (2017). Scenario dependence of future changes in climate extremes under 1.5 °C and 2 °C global warming. Sci. Rep. 7 (1), 46432. doi:10.1038/srep46432
Wen, B., Wang, S., Wang, E., and Zhang, J. (2004). Characteristics of rapid giant landslides in China. Landslides 1 (4), 247–261. doi:10.1007/s10346-004-0022-4
Wu, J., and Gao, X. (2013). A gridded daily observation dataset over China region and comparison with the other datasets. Chin. J. Geophys. 56 (4), 1102–1111. (in Chinese).
Wu, J., Gao, X., Giorgi, F., and Chen, D. (2017). Changes of effective temperature and cold/hot days in late decades over China based on a high resolution gridded observation dataset. Int. J. Climatol. 37 (S1), 788–800. doi:10.1002/joc.5038
Xia, J., and Han, A. (2009). Cyclic variability in microstructure and physio-mechanical properties of the Xiashu Loess-palaeosol sequence in Nanjing, China. Eng. Geol. 104 (3-4), 263–268. doi:10.1016/j.enggeo.2008.11.002
Xu, L., Li, H., and Wu, D. (2008). Discussion on infiltration of surface water and their significance to terrace loess landslides. Chin. J. Geol. Hazard Control 02, 32–35. (in Chinese).
Xu, X., Guo, W., Liu, Y., Ma, J., Wang, W., Zhang, H., et al. (2017). Landslides on the Loess Plateau of China: A latest statistics together with a close look. Nat. Hazards 86 (3), 1393–1403. doi:10.1007/s11069-016-2738-6
Xu, Z., Lin, Z., and Zhang, M. (2007). Loess in China and loess landslides. Chin. J. Rock Mech. Eng. 26 (7), 1297–1312. (in Chinese).
Yang, L., Feng, Q., Adamowski, J., Yin, Z., Wen, X., Wu, M., et al. (2020). Spatio-temporal variation of reference evapotranspiration in northwest China based on CORDEX-EA. Atmos. Res. 238, 104868. doi:10.1016/j.atmosres.2020.104868
Yang, L., Feng, Q., Yin, Z., Wen, X., Deo, R., Si, J., et al. (2019). Application of multivariate recursive nesting bias correction, multiscale wavelet entropy and AI-based models to improve future precipitation projection in upstream of the Heihe River, Northwest China. Theor. Appl. Climatol. 137 (1-2), 323–339. doi:10.1007/s00704-018-2598-y
Yang, X., and Li, D. (2008). Precipitation variation characteristics and arid climate division in China. J. Arid Meteorology 26 (2), 17–24. (in Chinese).
Zhai, P., and Pan, X. (2003). Change in extreme temperature and precipitation over northern China during the second harf of the 20th century. Acta Geogr. Sinca 58 (S1), 1–10. (in Chinese).
Zhang, D., Wang, G., Luo, C., Chen, J., and Zhou, Y. (2009). A rapid loess flowslide triggered by irrigation in China. Landslides 6 (1), 55–60. doi:10.1007/s10346-008-0135-2
Zhang, D., and Wang, G. (2007). Study of the 1920 Haiyuan earthquake-induced landslides in loess (China). Eng. Geol. 94 (1-2), 76–88. doi:10.1016/j.enggeo.2007.07.007
Zhang, F., and Huang, X. (2018). Trend and spatiotemporal distribution of fatal landslides triggered by non-seismic effects in China. Landslides 15 (8), 1663–1674. doi:10.1007/s10346-018-1007-z
Zhang, F., Liu, G., Chen, W., Liang, S., Chen, R., and Han, W. (2012). Human-induced landslide on a high cut slope: A case of repeated failures due to multi-excavation. J. Rock Mech. Geotechnical Eng. 4 (4), 367–374. doi:10.3724/SP.J.1235.2012.00367
Zhang, M., Li, T., Eintracht, S., and Hoffer, L. J. (2011). Vitamin C provision improves mood in acutely hospitalized patients. J. Eng. Geol. 19 (04), 530–533. (in Chinese). doi:10.1016/j.nut.2010.05.016
Zhang, M., and Liu, J. (2010). Controlling factors of loess landslides in Western China. Environ. Earth Sci. 59 (8), 1671–1680. doi:10.1007/s12665-009-0149-7
Zhang, S., Li, C., Peng, J., Zhou, Y., Wang, S., Chen, Y., et al. (2023). Fatal landslides in China from 1940 to 2020: Occurrences and vulnerabilities. Landslides 20, 1243–1264. doi:10.1007/s10346-023-02034-6
Zhang, Y., Jiang, X., Lei, Y., and Gao, S. (2022). The contributions of natural and anthropogenic factors to NDVI variations on the Loess Plateau in China during 2000-2020. Ecol. Indic. 143, 109342. doi:10.1016/j.ecolind.2022.109342
Zhao, J., Zhan, R., and Wang, Y. (2018). Global warming hiatus contributed to the increased occurrence of intense tropical cyclones in the coastal regions along East Asia. Sci. Rep. 8, 6023. doi:10.1038/s41598-018-24402-2
Zhou, D., Zhang, Z., Li, J., and Wang, X. (2019). Seepage-stress coupled modeling for rainfall induced loess landslide. Theor. Appl. Mech. Lett. 9 (1), 7–13. doi:10.1016/j.taml.2019.02.006
Zhou, T., Zou, L., and Chen, X. (2019). Commentary on the coupled model Intercomparison project phase 6 (CMIP6). Clim. Chang Res. 15 (5), 446–456. (in Chineae).
Zhou, W., Qiu, H., Wang, L., Pei, Y., Tang, B., Ma, S., et al. (2022). Combining rainfall-induced shallow landslides and subsequent debris flows for hazard chain prediction. Catena 213, 106199. doi:10.1016/j.catena.2022.106199
Zhuang, J., Iqbal, J., Peng, J., and Liu, T. (2014). Probability prediction model for landslide occurrences in Xi’an, Shaanxi Province, China. J. Mt. Sci. 11 (2), 345–359. doi:10.1007/s11629-013-2809-z
Keywords: Chinese Loess Plateau, extreme precipitation, fatal landslides, global warming, continuous precipitation
Citation: Guan X, Sun W, Kong X, Zhang F, Huang J and He Y (2023) Response of fatal landslides to precipitation over the Chinese Loess Plateau under global warming. Front. Earth Sci. 11:1146724. doi: 10.3389/feart.2023.1146724
Received: 17 January 2023; Accepted: 25 May 2023;
Published: 07 June 2023.
Edited by:
Liew Juneng, National University of Malaysia, MalaysiaReviewed by:
Linshan Yang, Chinese Academy of Sciences (CAS), ChinaPeiyue Li, Chang’an University, China
Lishan Ran, The University of Hong Kong, Hong Kong SAR, China
Copyright © 2023 Guan, Sun, Kong, Zhang, Huang and He. This is an open-access article distributed under the terms of the Creative Commons Attribution License (CC BY). The use, distribution or reproduction in other forums is permitted, provided the original author(s) and the copyright owner(s) are credited and that the original publication in this journal is cited, in accordance with accepted academic practice. No use, distribution or reproduction is permitted which does not comply with these terms.
*Correspondence: Fanyu Zhang, emhhbmdmeUBsenUuZWR1LmNu