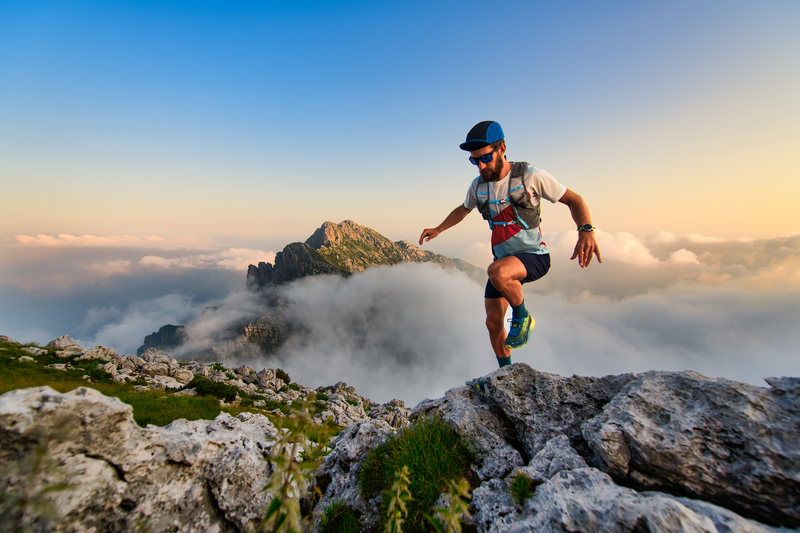
94% of researchers rate our articles as excellent or good
Learn more about the work of our research integrity team to safeguard the quality of each article we publish.
Find out more
ORIGINAL RESEARCH article
Front. Earth Sci. , 24 April 2023
Sec. Environmental Informatics and Remote Sensing
Volume 11 - 2023 | https://doi.org/10.3389/feart.2023.1133751
This article is part of the Research Topic Seafloor Mapping Using Underwater Remote Sensing Approaches View all 7 articles
Marine litter is a growing threat to the marine environment. Mapping of marine litter is becoming increasingly important to detect its potential hotspots and prevent their spread. In this paper, the applicability of the multibeam echo sounder (MBES) WASSP S3 and remotely operated underwater vehicle (ROV) Chasing M2 was tested in the detection and mapping of marine litter on the seafloor within the wider area of the St. Ante Channel (Šibenik, Croatia). Also, the precision assessment of WASSP S3 was tested at different cruising speeds. Results have shown that Chasing M2 can be used effectively for the initial detection of marine debris in shallow waters. However, if the underwater navigation and positioning system and auxiliary measurement scales are not used, the ROV has limited capabilities in deriving morphometric parameters of marine litter on the seafloor. This was determined by comparing the 3D model of a tire which was derived using video photogrammetry captured with ROV and the 3D model of a tire which was produced using a hand-held 3D scanner. Furthermore, the results have shown the WASSP S3 is not suitable for identifying marine litter smaller than 1 m at depths up to 10 m. The MBES WASSP S3 can detect marine litter that has a minimum area of 100 * 100 cm and a height of around 40 cm at depths up to 10 m. The results pointed to the need for caution when choosing an adequate sensor to detect and map marine litter on the seafloor. In addition, MBES interval measurements have shown that WASSP S3 precision is in the centimeter range (<10 cm) at different cruising speeds. The obtained results have helped to establish the guidelines for the integrated use of MBES, ROV, and UAV in the detection of marine litter on the seafloor.
Today, marine litter is recognized as one of the main ecological problems (Jeftić et al., 2009; Pham et al., 2014; Ioakeimidis et al., 2015; Kühn et al., 2015; Lusher, 2015; Tubau et al., 2015; Löhr et al., 2017; Consoli et al., 2018; Vlachogianni et al., 2018; Galgani et al., 2019; Funduk et al., 2021), which is not surprising given the fact that more than a half of world’s population lives 100 m from the sea (Brown, Blondel, 2009). Since marine litter is found in every ocean (Tubau et al., 2015; Galgani et al., 2019), it is not only an aesthetic problem, but represents a threat to the economy, environment, health, and more activities all around the world (Jeftić et al., 2009; Avio et al., 2017; Funduk et al., 2021). In addition to developed tourism and high population density, the Mediterranean Sea is characterized by highly developed industrial, tourism and fishing activities, which greatly affects the amount of marine litter (Cincinelli et al., 2018). As a result, much research has been conducted to map marine litter (Haarr et al., 2022). Marine litter is becoming a growing environmental problem in the Adriatic Sea. The Croatian side of the Adriatic coast is particularly at risk because it has about 6,000 km of karst coastline and over 1,240 islands, islets, and reefs and its position acts like a floating sieve that accumulates marine litter (Funduk et al., 2021).
The detection and classification of marine litter on the seafloor are based on three different approaches, most of which are combined altogether (Madricado et al., 2020). They include:
A. bottom trawling (Maes et al., 2018; Gerigny et al., 2019; Spedicato et al., 2019)
B. visual mapping (Oliveira et al., 2015; Huvenne et al., 2018; Pierdomenico et al., 2019)
C. acoustic (MBES) mapping (Mayer et al., 2007; Hughes Clarke, 2018; Madricardo et al., 2019).
Method (A) has the following limitations: invasiveness to the seabed, impossibility of application on the rocky seabed, and inaccurate information on the spatial distribution of the detected marine litter (Madricardo et al., 2020). Method (B) optical (visual) mapping methods rely on photographs and videos, that can usually be taken in shallow coastal areas. It can be collected by divers (Bauer et al., 2008), remotely operated underwater vehicles (ROV) (Oliveira et al., 2019), and autonomous underwater vehicles (AUV). There are many advantages of this method: non-invasive, provides quantitative data, applies to all types of the seafloor, and allows the creation of orthophoto mosaic of the seafloor, and 3D models that can be used to analyze the morphometric characteristics of the detected objects. However, its application may be limited by visibility, hydrodynamic conditions of the sea, and a complex set of parameters related to the processing of underwater photogrammetry (Kalacska et al., 2018). With ROV, mapping of macro (large) marine litter is possible with (C) multi-beam echo sounders (MBES) (Madricardo et al., 2019). Numerous studies were carried out using it, which resulted in high data resolution, and helped in detecting marine litter (Kostylev et al., 2001; Mayer et al., 2007; Madricardo et al., 2019). Despite the rapid development of MBES technology, resolution (detail of data collection) remains the major limitation of acoustic methods (Šiljeg et al., 2016; Šiljeg et al., 2018). It primarily depends on the specifications of the sonar, distance from the seabed (depth), and speed of the boat (Madricardo et al., 2020). Along with the MBES, classifying marine litter can also be done using backscatter data (Pierdomenico et al., 2019). Backscatter data can be used for seafloor classification and detection of different objects (Simons, Snellen, 2009; Lacharité et al., 2018). Collected data can be used to explore seafloor characteristics such as hardness, sediment properties, and even sediment grain size.
In the Republic of Croatia (RH), due to the specific sea current (counterclockwise) (URL1), the islands in southern Dalmatia (Vis, Korčula, Mljet, Lastovo) are the most affected by marine litter. Previous studies on marine litter in the Adriatic Sea have shown that plastic as a type of marine litter is the most widespread, which is not only an aesthetic problem but also seriously endangers the entire marine environment (Kwokal, Štefanović, 2009). The increasing amounts of marine debris and its slow decomposition pose a great threat to the marine environment (Ioakeimidis et al., 2015), and its mapping is important to identify potential hotspots and prevent the spread of such areas.
In this paper, the possibility of application of the basic package of the remotely operated underwater vehicle (ROV) Chasing M2 and the MBES WASSP S3 in a mapping (detection and quantification) of marine litter was tested in the wider area of the St. Ante Channel (Šibenik), near the research station Martinska (Ruđer Bošković Institute). The main goal of the research was to determine the advantages and limitations of using a specific MBES and ROC in marine litter mapping. To achieve the main goal, it was necessary to set several secondary objectives:
• Generate textured 3D models of marine debris at selected test locations.
• Derive the digital bathymetric models (DBM) using a MBES.
• Assess the MBES precision regarding the different speeds of a boat.
From the set objectives the following hypotheses emerged:
(H1). MBES WASSP S3 will enable the mapping of marine litter with a minimum width of 20 cm at depths down to 10 m.
(H2). The precision of the MBES WASSP S3 will be >10 cm at depths down to 10 m at different directions and sailing speeds.
The survey was conducted on the test area of 400 m2 in the wider area of the St. Anthony’s Channel near the Martinska research station (Ruđer Bošković Institute) and the city of Šibenik (Figure 1).
This area was selected as a suitable test area for several reasons. First, Martinska is one of the research stations of the Ruđer Bošković Institute, which is the leading scientific institution in the Republic of Croatia. Underwater cameras are installed there, and the presence of marine litter has been detected for a long time, which is primarily a consequence of the large concentration of maritime traffic, which entails discarded waste. Furthermore, summer festivals and fishing are the reason for the gathering of the people in this area who take advantage of the remoteness of the place to dispose of waste that often ends up in the sea.
The research methodology can be divided into six main steps that included the following activities:
• UAV photogrammetry of the wider research area
• 3D scanning of a car tire using a manual 3D scanner
• Interval MBES survey of the test area
• ROV recording of the test area
• Detection and quantification of marine litter
• Comparison of generated results.
UAV photogrammetry included imaging of a wider surface around the test area at sea (400 m2) where a bathymetric and ROV survey was conducted. The test area was marked with buoys and, together with the wider surface, had a total area of 7.6 ha. UAV photogrammetry was conducted using a UAV Phantom 4 Pro V2.0.10 Ground Control Points were collected with GNNS Trimble R12i. The image workflow process was done in Agisoft Metashape. The purpose of the UAV survey was to create a digital orthophoto (DOP) and a digital surface model (DSM), which were used to visualize the wider area of Martinska, visualize the test area at sea and try to detect marine litter from the air, given that the transparency of the sea was relatively good, and the MBES survey within the defined test area was done down to a depth of 10 m.
Before surveying the test area (400 m2) with a Chasing M2 ROV and WASSP S3, scanning was performed with a handheld 3D scanner Artec Eva (URL 2) of a car tire that was found in the immediate vicinity of the coast.
The purpose of this scan was to determine the relative accuracy of the mentioned sensors. Testing of the relative accuracy was performed based on the morphometric parameters of the tire models derived from ROV and MBES as well as by the handheld 3D scanner. Of course, a comparison of the morphometric parameters of all three sensors would be possible if MBES successfully detects this tier on the seafloor. In this case, the model made with the Artec Eva handheld 3D scanner would serve as reference data. With an accuracy of up to 0.1 mm, it is an excellent sensor for recording objects of medium-small size (minimum object size 10 cm). After 3D scanning of the mentioned car tire with an Artec Eva, a professional diver placed the tire within a defined test area which was then recorded by the MBES and ROV system. At the end of the survey, the tire was taken out of the sea.
During the scanning of the tire, several 3D scans were collected, which were then processed in Artec Studio Professional 15 through five steps: (1) global registration, (2) crop surroundings, (3) outliner removal, (4) sharp fusion, and apply texture. Then simple morphometric parameters of the scanned tire were generated from the derived 3D model using the toolset from the Measure option (Figure 2).
The MBES survey was conducted on 16 May 2022, and it included the mapping of marine litter in the 20 x 20 m defined test area. This area was surveyed eight times. The surveys differed in sailing speed and direction. Precision testing using the Multiscale Model to Model Cloud Comparison (M3C2) plugin was performed for the first 6 MBES surveys performed in NE - SW, and SE - NW directions and at speeds of 1.5, 3, and 4.5 knots. M3C2 is a plugin within CloudCompare that computes signed (and robust) distances directly between two point clouds. This technique enables fast comparisons of large point clouds with complex surfaces that span a range of surface orientations (Barnhart, Crosby, 2013). The goal of this method of interval recording was to determine the reliability of MBES in the context of performing bathymetry. Eight MBES surveys were performed with these parameters (Figure 3):
A) Speed 1.5 knots (2.78 km/h) direction NE - SW from the S part of the area
B) Speed 1.5 knots (2.78 km/h) direction SE - NW movement from the S part of the area
C) Speed 4.5 knots (8.33 km7h) direction NE - SW from the S part of the area
D) Speed 4.5 knots (8.33 km/h) direction SE - NW movement from the S part of the area
E) Speed 3 knots (5.55 km/h) direction SE - NW movement from the S part of the area
F) Speed 3 knots (5.55 km/h) direction NE - SW movement from the S part of the area
G) Speed 3 knots (5.55 km/h) direction N - S moving from the S part of the surface
H) Speed 3 knots (5.55 km/h) direction E - W from the S part of the surface
All the components of the MBES were installed on the rubber boat Luna, which has repeatedly proven to be effective in this kind of research due to the easy installation of the necessary components on it, as well as its relatively short length (Šiljeg et al., 2022) (Figure 4A).
FIGURE 4. (A) Installation of MBES integrated measurement system on Luna and (B) underwater survey using ROV Chasing M2.
There were six main components of the MBES integrated measurement system:
a) WASSP S3 Multibeam Wideband Sounder c/w DRX
b) WASSP Sensor Box with integrated Spatial IMU
c) Hemisphere V320 GNSS Smart Antenna (mFreq, mGNSS, RTK, SBAS)
d) accumulator and power cord
e) configuration of computer and cable
f) configuration software (PoketMax, NtripClient, DRX Setup Webpages)
g) software for guidance (CDX) and data export (Data Manager)
A transducer is a device that converts an electronic pulse into sound and sends it toward the seafloor, and it is attached to a steel pole and immersed in water (de Jong et al., 2002). The depth of the seafloor is measured based on the time delay of the sound wave. For the purposes of the MBES survey, the WMB-160 transducer was used, which is characterized by an operating frequency of 160 kHz. The use of very low frequencies (90 kHz or 100 kHz) enables exploration of great depths (several hundred meters), while recordings at very high frequencies (e.g., 700 kHz) enable the collection of ultra-high-resolution data thanks to the higher acoustic resolution (vertical resolution) and smaller bandwidth (horizontal resolution). The WMB-160 transducer is attached to a steel pole with the aim of having as little influence on the output results as possible, which is helped by the fact that during the bathymetric survey, it does not move. Given that it is immersed in water (50 cm), it is important to emphasize that the vertical difference between the transducer and the water surface was considered during the measurement. To achieve the shortest path of the transmitted sound signal and its accurate georeferencing using the antenna placed directly above the transducer, it is oriented at an angle of 90⁰.
During the MBES bathymetric survey, the sea was in ideal conditions. The waves were almost imperceptible in size.
Despite the almost ideal conditions on the sea, the Luna ship was always in motion along the X, Y, and Z-axes, which, if not properly registered, can affect the output results. Therefore, the inertial measurement system (IMU) was used, which registers every movement of the ship along the X, Y, and Z-axes. Advanced navigation spatial IMU WSP002-INU is a navigation system that, in combination with the Hemisphere V320 GNSS antenna (URL 3), enables obtaining data on speed, location, acceleration, and orientation during the MBES survey.
IMU WSP002-INU is in a sensory box and contains an inert GPS navigation system and AHRS (attitude and heading reference system). The used system is characterized by high sensitivity to movements along different axes; therefore, it provides data on the angular tilt and direction of the ship’s movement regardless of its size (URL3).
Before the MBES survey, it was necessary to collect secondary data to accurately calibrate the system. Sound velocity (SV) is derived from direct data on seawater temperature (◦C) and salinity (ppt). Seawater temperature and salinity data were measured using the EXO2 multiparameter sonde. The SV parameter is crucial in canceling measurement range inaccuracies caused by sound speed variations in water. It is considered one of the main factors that can affect the quality of MBES data. Furthermore, the derived SV was verified by a visual component at the CDX interface above the flat seabed. Namely, the visual effect of an incorrect SV is manifested by the concave or convex curvature of the flat seabed. Depending on the location, the SV was regularly adjusted and manually checked using the sound screen. At the time of the recording, the research area was marked by a water temperature of 17°C and a salinity of 33‰, which calculated the speed of sound in water to be 1,510.54 m/s. Changes in temperature and salinity in the water column can affect the change in sound wave speed, which can result in a distortion of the seabed in a concave or convex shape (if the speed of sound on the sea surface is lower than the sound in deeper parts, there will be a concave distortion) (Jin et al., 2015). Collected data was controlled and visualized in WASSP’s operating system CDX. The CDX consists of six components: (1) home access; (2) screen frames; (3) CS (context-sensitive) menu; (4) information screen; (5) control tools; (6) optional tools. During the bathymetric survey, this system enables the visualization of the recorded area through four views: a) two-dimensional, b) perspective (3D), c) sonar field, and d) profile.
The vessel’s central reference point (CRP) was determined after the installation of the MBES. The defined settings (beam aperture, pulse length, beam width) were controlled by the onboard software and remained constant during multibeam depth sounder imaging. The ping rate during interval MBES recordings was about 12/s, while the operating beam width was about 40 kHz. An important phase of the system calibration process was the configuration of the GPS antenna, for which the PocketMax3 software was used. The Hemisphere V320 GNSS antenna was connected to the CROPOS_VRS_RTCM31 system via the NtripClient software, which enables data registration in real-time. It is a multi-frequency, multi-constellation GNSS antenna based on Eclipse Vector™ technology, which provides an RTK-level position and precise heading. It achieves a heading accuracy of up to 0.17° RMS and offers a robust positioning performance. The Hemisphere Vector V320 was connected to the CROPOS (Croatian Positioning System) via the GSM network.
The underwater survey with ROV Chasing M2 was conducted in duration of 15–20 min (Figure 4B). The acquired video was processed in the Agisoft Metashape 1.5.1 software. A total of 7,875 photos were derived from the video. In this case the underwater photogrammetry was conducted with the basic sensor package that comes with the ROV. Therefore, the survey was characterized by many limiting factors compared to the regular UAV photogrammetry. They are:
• the difficult process of camera calibration
• absence of light
• difficult ROV orientation
• light refraction
• bad image texture which makes the process of photo aligment more difficult
• the impossibility of determining the appropriate overlap between images
• difficult absolute orientation of the reconstructed model
• absence of EXIF1 photo data regarding location of sensor within specific global coordinate system
After the photos were extracted from the video, a test alignment of all photos in one chunk was performed to try to obtain a unique model of the seafloor. As expected, the process failed because of this reasons:
• many photos had similar textures (dominant blue color)
• absence of EXIF data (XYZ)
• the quality of underwater photos was worse than UAV photos
• insufficient overlap between photos
Therefore, the photos were divided into several chunks. Each chunk contained photos of one or more pieces of marine litter. Considering that the camera of the used ROV has a small focal length, which generates a large field of view (FOV), the photos at the edges had distortions. Although during the alignment Agisoft solves the relative orientation problem and performs self-calibration, it was decided that edges of the photos needs to be masked. Therefore, only central part of the images is used in the process of the alignment and derivation of the dense cloud (Kwasnitschka et al., 2013). Young et al. (2017) stated that uncalibrated cameras produced accurate centimeter-scale reconstructions, suggesting that Agisoft can adequately work with uncalibrated cameras and photographs of larger distortions. Considering that the use of the basic ROV package does not include the possibility of obtaining EXIF data for each photo (XYZ), it was decided that the data written on each photo (depth, heading, pitch) needs to be converted into a text document and re-imported into individual chunks as EXIF data. In this way, photos would at least have depth data and two angular offsets. Namely, in other research, where the USBL method of underwater acoustic positioning is available the linking of each photo frame with its USBL position is performed, which enables the direct execution of georeferenced models (Price et al., 2019).
The image workflow process consisted of several steps. The first step refers to (1) photo aligment, which included identifying and extracting the tie points. The accuracy variable was set to high, while the key point limit and tie point limit parameters were set to 0 to generate the maximum number of tie points. This was done considering the charateristics of the environment (low light conditions, absence of differences in texture). Then, selective deletion (2) of tie points (sparse cloud) was performed to improve the relative orientation of the reconstructed model. A reconstruction uncertainty and reprojection error parameters were used within the Gradual Selection. Within each parameter 10% of the total number of derived tie points were deleted. Then iterative optimization of the tie points was performed, which re-estimated the camera’s intrinsic calibration parameters. The next step was (3) the derivation of a dense point cloud. A high-quality parameter was selected during processing. After derivation of dense point cloud (6) digital surface models (DEM) and (7) digital orthophoto (DOP) models of the marine litter were created. In the final step, the individual chunks were aligned and merged into a single chunk for better visualization and comparison of the models. In the aligment process the parameter quality was set to highest. Considering the lack of ground control points, the alignment method was set to point-based, while the limit of the tie points involved in the connection was set to 10,000.
The processing of underwater photos derived from videos acquired by ROV was successfully performed for each of the defined chunks. The following marine litter was detected (Figure 5):
1) Car tire (scanned by Artec Eva) - inserted by the authors
2) Two glass bottles
3) A piece of PVC (Polyvinyl chloride)
4) Plastic tube
5) Sheet metal
6) Old car tire
Figure 6 shows the digital surface models (DEMs) and digital orthophotos (DOPs) of the detected marine litter on the seafloor. From the DOP derived using UAV photogrammetry only sheet metal can be marginally detected from the air (Figure 6 – red circle). The reasons for this were the slightly shallower depth at which it is located and the shiny surface that reflects sunlight. A hillshade model was added to the DEMs to highlight the boundaries of the marine litter itself (Figure 6).
FIGURE 6. Digital surface models (DEMs) and digital orthophotos (DOPs) of the detected marine litter.
Although the processing of all chunks has been completed and all marine litter elements have been detected on the seafloor, it is important to note that the application of the basic ROV package does not allow accurate calculation of the morphometric parameters of the detected objects. This was best illustrated in the example of a car tire that was captured by the Artec Eva handheld 3D scanner. The width and length of the car tire calculated from the 3D model by Artec Eva was 76 cm, while in the reconstructed model from the underwater images it was 560 cm.
Figure 7 shows the unfiltered data of the acquired dense point clouds cut according to the boundaries of the test area (20 * 20 m) for the selected six MBES interval surveys (NE - SW, SE -NW at speeds of 1.5, 3, and 4.5 knots). The derived parameters of the point number and point density best illustrate how the vessel speed and the direction of travel can affect MBES sampling density.
FIGURE 7. Unfiltered data of the acquired dense point clouds in different MBES survey (A) speed 1.5 knots direction NE - SW; (B) speed 1.5 knots direction SE - NW; (C) speed 4.5 knots direction NE - SW; (D) speed 4.5 knots direction SE - NW; (E) speed 3 knots direction SE - NW; (F) speed 3 knots direction NE - SW.
As expected, most points were collected in scenarios (A) and (B) where the vessel’s speed was the lowest, i.e., 1.5 knots. In scenario (B) 201,841 points were collected, and in scenario (A) 173,294 points. The average number of points collected in the scenarios with the lowest possible speed of vessel was 187,685 points. Thus, the sampling density of the test area at a speed of 1.5 knots was 470 points/m2.
As expected, the lowest number of points was collected in scenarios (C) and (D) where the vessel speed was the highest, i.e. 4.5 knots. In scenario (C) 66,220 points were acquired and in scenario (D) 84,381 points. The average number of points collected in the scenarios with the highest possible speed of the vessel was 75,300 points. Thus, the sampling density of the test area at a speed of 4.5 knots was 188 points/m2, or 2.5 times lower than the sampling density at the lowest speed. This roughly corresponds to the differences in sailing speed.
Table 1 shows a summary of the distance between the MBES dense clouds of six interval measurements. The distance analysis was performed using the M3C2 algorithm in CloudCompare. The largest SD equal to 10 cm refers to the point cloud derived from scenario B (1.5 knots, SE - NW) and the point cloud derived from scenario F (3 knots, NE - SW), where point cloud B was considered as a reference. The smallest SD was measured between point cloud F (3 knots, NE - SW) and point cloud A (1.5 knots, NE - SW), and it was 3.7 cm. In this case, point cloud F was set as a reference. The example of M3C2 calculation is given in Figure 8 where scenarios (A) and (C) are compared.
FIGURE 8. Example of dense cloud comparison using M3C2 tool (A) speed 1.5 knots direction NE - SW; (B) speed 1.5 knots direction SE - NW; (C) speed 4.5 knots direction NE - SW.
The average SD, when the point clouds from the scenarios with the highest speed (4.5 knots) of sailing (C, D) are used as reference models, was 7 cm. This proves that the point clouds acquired at the maximum speed vessel do not differ significantly from the point clouds recorded at the minimum speed vessel of 1.5 knots, despite the much smaller point density. The mean SD of all acquired point clouds was 7.4 cm. The M3C2 analysis confirmed the significant coincidence of all compared point clouds, i.e., the high precision of MBES WASSP S3. Such high precision (<10 cm) is not surprising considering that the interval MBES surveys were performed one after the other, in the same, relatively stable sea conditions, and that the MBES system was connected to the CROPOS system - the national network of reference GNSS stations of the RH.
Digital bathymetry models (DBMs) of a test area were created from dense point clouds obtained from MBES dense clouds acquired from different directions and at the lowest (1.5 knots) and highest (4.5 knots) speed of a vessel. The objective of the study was to determine if marine litter can be detected on the produced DBMs or dense clouds acquired in different scenarios of MBES surveys. The detection of marine debris was attempted on the lowest (A, B) and highest speed (C, D) vessel scenarios. The process was performed for a larger acquisition area and after was cut according to the polygonal boundaries of the test area. Before performing DBMs, filtering of dense point clouds was performed. A new methodological approach based on semi-automatic error removal was applied (Šiljeg et al., 2022). The approach was extended by a new step of applying the Cloud to Cloud tool which removed all points with an absolute distance greater than 15 cm between the two dense point clouds used (same speed vessel but different direction).
The Moving Least Squares (MLS) tool in Cloud Compare then was used to smooth the dense point cloud. The SR parameter was optimized to 0.5 as it gave the best results after the iterative method. After filtering the points, the point clouds of the lowest vessel speed (A, B) had over 1 million points, while the contiguous point clouds of the highest vessel speed (C, D) had several hundred thousand of points. Rasterization of both merged point clouds was performed in ArcMap using the LAS Dataset to Raster tool. The Natural Neighbor (NN) interpolation method was used to convert depth points into a raster format (Šiljeg et al., 2022). It uses triangulation to estimate z-value by applying area-based weights to the terrain’s natural neighbors of a query point. Coleman et al. (2011) tested four interpolation techniques, including Kriging, NN, inverse distance weighted (IDW), and spline in the ability to accurately represent shallow-water bathymetry. The NN was founded to be the most effective.
From both generated DBMs (slow vessel speed and the highest possible vessel speed) it was possible to see that the marine litter was not detected on the seafloor. This was confirmed by performing different morphometric analyzes (isohypses, slope) (Figure 9). This applies to the filtered integral model and the unfiltered integral models. Therefore, a visual inspection was conducted of the unfiltered point clouds in Cloud Compare to detect certain deviations that could indicate the specific objects on the seafloor.
FIGURE 9. (A) Unfiltered DBM from scenario A; (B) unfiltered DBM from scenario B; (C) unfiltered DBM of A and B scenario; (D) filtered DBM of A and B scenario; (E) unfiltered DBM from scenario C; (F) unfiltered DBM from scenario D; (G) unfiltered DBM of C and D scenario; (H) filtered DBM of C and D scenario.
Since the MBES survey covered a larger area outside the defined test area on the unfiltered point cloud of the smallest speed vessel concrete blocks with dimensions of 100 cm * 100 cm * 40 cm were detected northeast of the test area. Buoys attached to them were visible at DOP derived from UAV photogrammetry. One concrete block was located at a depth of 6.5 m and has the above-mentioned dimensions, while the other block was located slightly further east at a depth of 9 m and has slightly smaller dimensions. Considering the given dimensions of the objects and the fact that the concrete blocks were detected with 100% certainty, which was also confirmed by a diver who knows the morphology of the recorded seabed, it can be said that the minimum mapping unit for a marine litter of the WASSP S3 at this specific depth (10 m) was 100 cm (width) * 100 cm (length), and a height of about 40 cm. In other words, only objects with a surface area greater than 1 m2 and a height of 40 cm on the seafloor can be effectively detected from the unfiltered point cloud (Figure 10).
FIGURE 10. Detection of concrete blocks on the unfiltered point cloud acquired during the smallest speed of a vessel.
However, knowing the position of specific marine litter within the test area, through UAV DOP and ROV models enabled the detection of the sheet metal object in the unfiltered point cloud. However, its detection was highly uncertain, considering that it depends on the position under which the point cloud was viewed/rotated, and even then, it cannot be determined with absolute certainty. Only if the point cloud was viewed in a certain position, namely at the place where according to the DOF the sheet metal should be, a certain bulge in the point cloud can be observed (Figure 11).
Although the MBES survey plan was well-defined and all surveyed area was covered, almost no marine litter was detected in selected area. On all dense point clouds derived from interval surveys, only the sheet metal object was observed within the defined test area, and not with 100% certainty. The reason for this was the specifications of the MBES, which determine the minimum mapping unit and, thus the minimum size of the object to be detected (Hughes-Clarke et al., 1999).
a) Ping rate - the number of acoustic signals depends on the water depth and can be up to 60 signals per second in shallow waters. During the interval recording in the test area, the ping rate was only 12. A high number of short acoustic signals means that much more data is collected.
b) Pulse length and bandwidth - the shorter the pulse length, the higher the vertical recording resolution. Recording with short pulse lengths is possible with high-performance MBES with excellent electronics that produce little or no noise. The bandwidth used in this survey was 40 kHz and is controlled by the length of the transmitted sound signals (pulse width). The shorter the length of the transmitted sound signals, the higher the bandwidth and resolution (Le Bouffant et al., 2013).
c) Operating frequency - depending on the depth of the survey area it is important to use the optimal frequency to obtain accurate and high-quality data. For example, low-frequency recording is characteristic for deeper areas (e.g., wavelengths with a frequency of 90 or 100 kHz are optimal for recording areas with a depth of 700 m, or even deeper), while high frequencies are optimal for recording shallower areas or for recording seafloor details, such as marine litter. In this case, WASSP S3 was used, which has a frequency of 160 kHz. This MBES system does not support changing or increasing the recording frequency.
d) Vessel speed - depending on the specifications of the sensor and the area covered, the maximum allowable speed is set to ensure complete coverage of the area covered. Almost all marine litter could not be detected even at the lowest speed of the vessel (1.5 knots). By indirect analysis, but not with 100% certainty, only the sheet metal in the center of the test area was detected from the dense point clouds. So, in this case, the sailing speed cannot be the reason for the lack of marine litter detection.
e) Halocline - the test area is part of the Krka River estuary, which should be considered when interpreting the obtained results. Namely, the mixing of fresh and saltwater can lead to a change in the speed of sound in the water. Accordingly, the output results showing no marine debris on the seabed may be the result of this, although this reason is hardly possible.
The accumulation of marine litter on the coasts and on the seafloor is a growing problem in the world’s seas and oceans (Andriolo et al., 2020). The Adriatic Sea is no exception (MINGOR, 2020; Funduk et al., 2021) where heterogeneous waste generated from different sources will increasingly accumulate on the shores (Funduk et al., 2021). By testing the selected technologies (MBES, ROV, UAV) appropriate combined (integrated) approach for the detection of marine litter have been suggested. The proposed methodological framework integrates remote sensing and in situ observations. The following are guidelines:
1) Pre-selection of locations for marine litter inspection using remote sensing data and/or GIS-MCDA (Geographic Information System - MultiCriteria Decision Analysis) - when detecting sites on a large area (smaller scale) that are most susceptible to the occurrence of coastal, floating and seafloor marine litter, application of the remote sensing data and/or GIS-MCDA process can be used. For example, some studies (Ioakeimidis et al., 2015) show a relationship between the occurrence of marine litter and the following parameters: distance from land, proximity to fishing routes, distance to cities, tourist activities, population density, sea depth, sea currents. This parameters can be used as input criteria in deriving a susceptibility model of marine litter occurrence. The application of high-resolution commercial multispectral satellite images can be very useful in narrowing the area of interest on which more detailed marine litter mapping will be done. A great review on the use of optical remote sensing data in marine litter detection was given by Topouzelis et al. (2021).
2) Initial visual inspection of coastal, floating and seafloor marine litter using a UAV - after the pre-selection of locations for marine litter inspection UAVs can be used for the initial inspection of marine litter. Namely, UAVs have been proven efficient tools for environmental monitoring because they enable real-time high-resolution monitoring at a relatively low cost (Andriolo et al., 2020). In recent papers applicability of UAVs in mapping stranded marine litter (>2.5) on DOPs has to be proven (Merlino et al., 2020). The advantages of using UAVs in the initial detection of coastal, floating and seafloor marine litter are the coverage of large, hard-to-reach areas, lower human costs, concerning marine litter it can overcome logistical constraints (Andriolo et al., 2020). With the aim of increasing the chance of marine litter detection on the coast and seafloor, it is desirable to perform UAV surveys in ideal weather conditions that would favor bigger transparency of the sea. The amount of sunshine must be high, the cloudiness and turbulence of the sea surface must be low.
3) Application of optimal MBES in marine litter detection - in order to accurately detect the marine litter on the seafloor of a specific size (micro, meso, macro) it is necessary to carefully analyze the specifications of the used MBES system (Chapter 5.1), i.e. the user-defined parameters during MBES imaging. If it is necessary to detect smaller forms of marine debris on the seafloor, the MBES acquisition frequency must be higher, the number of acoustic signals (ping rate) must be higher, and the bandwidth must be smaller.
4) Visual inspection and morphometric analysis of marine litter using ROV - in the detection of marine litter on the seafloor in shallow waters, the most used method is the diving method, which, although widely applicable and validated, can be time-consuming, requires high costs, expertise, and the deployment of a larger team (Escobar-Sánchez et al., 2022). For this reason, ROV can be very useful, although it is important to be aware of the lack of application of ROV in visual inspection, which leads to a possible underestimation of the amount of litter on the seafloor since buried elements of marine waste cannot be detected. In addition, poor visibility may limit its use in classifying objects. The use of various underwater positioning systems, such as LBL (Long BaseLine), USBL (Ultra-Short Baseline), SBL (Short Baseline), and GPS “smart” buoys, would greatly facilitate the process of acquisition of underwater photographs, while the presence of local coordinate system or appropriate scales would allow accurate measurement of morphometric parameters (Drap, 2012; Kwasnitschka et al., 2013; Ioakeimidis et al., 2015). The following are guidelines for conducting underwater photogrammetry, aimed at capturing and determining the morphometry of various objects.
(a) additional ROV equipment - the quality and applicability of the reconstructed 3D models on the seafloor depend primarily on the available equipment and user expertise. If possible, users should have the following additional equipment: a) additional lighting; this helps to highlight the true colors of the captured objects and reduces the green and blue wavelengths that typically dominate most underwater photographs. A great amount of light allows a higher shutter speed value to be set, which allows a sharp photo to be taken (freezing motion); (b) a system for underwater positioning that allows the position of the ROV to be known in real-time.
(b) quality of input data - if possible, underwater photography should be conducted in ideal weather conditions (calm weather, no waves, and diffused light). This will allow for an increase in shooting distance. An important factor is the use of high-resolution sensors with low noise. Shooting in bright light is not recommended in shallow areas as it creates wave patterns on the model. A lens should have a very large depth of field or a fixed focus that is compensated with a small relative aperture. Three basic conductions must be met: the photos must be in focus (sharp), have good exposure, and have sufficient resolution. One should always be guided by the basic rule of photogrammetry: taking more photos is better than insufficient overlapping images or an incomplete data set.
(c) measuring scales - measuring scales should be in a scene if the practicality of the application allows it. The scales should be perpendicular to each other to reduce relative length errors and possible one-sided errors. For the purposes of assessing the accuracy of the reconstructed model, it is possible to set up additional measuring scales. Scales should be uniformly distributed throughout the recording scene.
(d) underwater orientation or control points - like conducting UAV photogrammetry, it is desirable to place underwater control points within the imaging area. Although not mandatory, it is possible to use a photogrammetric program to georeference the model (if XYZ coordinates are determined) or to connect different reconstructed models
5) Raising awareness of marine environmental protection using ROV - the use of ROV for promotional purposes, even its only basic package when no detailed morphometric analysis of marine litter is done, helps to raise awareness of the negative impacts on the marine environment (Ioakeimidis et al., 2015). This is particularly effective for younger generations (Lallensack, 2018).
The study investigated the applicability of the ROV Chasing M2 and the MBES WASSP S3 in mapping, i.e., detecting and quantifying marine litter in the wider area of the St. Anthony’s Channel (Šibenik, Croatia). According to the derived results, research hypotheses were confirmed or rejected.
The first hypothesis (H1) was accepted. Through the analysis of DBMs and the unfiltered point cloud obtained at the lowest recording speeds, the minimum mapping unit, i.e., the smallest object of marine litter that WASSP S3 can detect at a depth of up to 10 m, was determined. Its surface is 100 cm * 100 cm, with a height of about 40 cm.
The second hypothesis (H2) was confirmed. Interval MBES measurements have established that WASSP S3 has a measurement accuracy in the centimeter range (<10 cm) at various sailing speeds. The average value of SD, where point clouds from the data set with the highest sailing speed (4.5 knots) (C, D) were used as a reference model, is 7 cm.
In addition, it was found that the ROV Chasing M2 can be effectively used for the initial detection of debris in shallow waters. However, when the ROV’s underwater navigation system and additional measurement scales were not used, the ROV has a limited ability to quantify marine litter. This was determined by comparing the morphometric parameters of the tire, derived from the 3D model produced with a hand-held 3D scanner. The present results have helped to provide guidelines for the use of geospatial technologies in the detection of marine litter on the seafloor.
The raw data supporting the conclusion of this article will be made available by the authors, without undue reservation.
AŠ, IM, and SK contributed to the conception and design of the study. AŠ, NC, ML, and IM conducted an MBES survey. IM, FD, and LP conducted UAV photogrammetry and process the data. AŠ, IM, NC, SK, and TB conducted an ROV survey and process the data. IM, SK, FD, and LP conducted 3D scanning and process the data. TB was the official diver necessary for a survey. SK wrote the first draft of the manuscript. AŠ, IM, and SK did paper preparation and editing. All authors contributed to manuscript revision, read, and approved the submitted version.
This research is founded through the Croatian Science Foundation project UIP-2017-05-2694 and supported by Center for geospatial technology.
The authors declare that the research was conducted in the absence of any commercial or financial relationships that could be construed as a potential conflict of interest.
All claims expressed in this article are solely those of the authors and do not necessarily represent those of their affiliated organizations, or those of the publisher, the editors and the reviewers. Any product that may be evaluated in this article, or claim that may be made by its manufacturer, is not guaranteed or endorsed by the publisher.
Andriolo, U., Gonçalves, G., Sobral, P., Fontán-Bouzas, Á., and Bessa, F. (2020). Beach-dune morphodynamics and marine macro-litter abundance: An integrated approach with Unmanned Aerial System. Sci. Total Environ. 749, 141474. doi:10.1016/j.scitotenv.2020.141474
Avio, C. G., Gorbi, S., and Regoli, F. (2017). Plastics and microplastics in the oceans: From emerging pollutants to emerged threat. Mar. Environ. Res. 128, 2–11. doi:10.1016/j.marenvres.2016.05.012
Barnhart, T. B., and Crosby, B. T. (2013). Comparing two methods of surface change detection on an evolving thermokarst using high-temporal-frequency terrestrial laser scanning, Selawik River, Alaska. Remote Sens. 5 (6), 2813–2837. doi:10.3390/rs5062813
Bauer, L., Kendall, M., and Jeffrey, C. (2008). Incidence of marine debris and its relationships with benthic features in gray’s reef national marine sanctuary, southeast USA. Mar. Pollut. Bull. 56, 402–413. doi:10.1016/j.marpolbul.2007.11.001
Brown, C. J., and Blondel, P. (2009). Developments in the application of multibeam sonar backscatter for seafloor habitat mapping. Appl. Acoust. 70 (10), 1242–1247. doi:10.1016/j.apacoust.2008.08.004
Cincinelli, A., Martellini, T., Guerranti, C., Scopetani, C., Chelazzi, D., and Giarrizzo, T. (2018). A potpourri of microplastics in the sea surface and water column of the Mediterranean Sea. TrAC Trends Anal. Chem. 110, 321–326. doi:10.1016/j.trac.2018.10.026
Coleman, J. B., Yao, X., Jordan, T. R., and Madden, M. (2011). Holes in the ocean: Filling voids in bathymetric lidar data. Comput. geosciences 37 (4), 474–484. doi:10.1016/j.cageo.2010.11.008
Consoli, P., Falautano, M., Sinopoli, M., Perzia, P., Canese, S., Esposito, V., et al. (2018). Composition and abundance of benthic marine litter in a coastal area of the central Mediterranean Sea. Mar. Pollut. Bull. 136, 243–247. doi:10.1016/j.marpolbul.2018.09.033
Escobar-Sánchez, G., Markfort, G., Berghald, M., Ritzenhofen, L., and Schernewski, G. (2022). Aerial and underwater drones for marine litter monitoring in shallow coastal waters: Factors influencing item detection and cost-efficiency. Environ. Monit. Assess. 194 (12), 863. doi:10.1007/s10661-022-10519-5
Funduk, M., Tutman, P., Farkaš, A., Tišma, S., and Boromisa, A. M. (2021). Marine litter in Croatian adriatic: Sources, quantities and stakeholders′ perspectives. Sustainability 13 (9), 4691. doi:10.3390/su13094691
Galgani, L., Beiras, R., Galgani, F., Panti, C., and Borja, A. (2019). Editorial: Impacts of marine litter. Front. Mar. Sci. 6, 208. doi:10.3389/fmars.2019.00208
Gerigny, O., Brun, M., Fabri, M. C., Tomasino, C., Le Moigne, M., Jadaud, A., et al. (2019). Seafloor litter from the continental shelf and canyons in French Mediterranean Water: Distribution, typologies and trends. Mar. Pollut. Bull. 146, 653–666. doi:10.1016/j.marpolbul.2019.07.030
Haarr, M. L., Falk-Andersson, J., and Fabres, J. (2022). Global marine litter research 2015–2020: Geographical and methodological trends. Sci. Total Environ., 820, 153162. doi:10.1016/j.scitotenv.2022.153162
Hughes Clarke, J. E., Mayer, L. A., Shaw, J., Parrott, R., Lamplugh, M., and Bradford, J. (1999). “Data handling methods and target detection results for multibeam and sidescan data collected as part of the search for SwissAir Flight 111,” in Proceedings of the shallow water survey conference (SWS) (Durham, NH, USA.
Hughes Clarke, J. E. (2018). “Multibeam echosounders,” in Submarine geomorphology. Editors A. Micallef, S. Krastel, and A. Savini (Heidelberg, Germany: Springer), 25–41. doi:10.1007/978-3-319-57852-1_3
Huvenne, V. A., Robert, K., Marsh, L., Iacono, C. L., Le Bas, T., and Wynn, R. B. (2018). “Rovs and auvs,” in Submarine geomorphology. Editors A. Micallef, S. Krastel, and A. Savini (Heidelberg, Germany: Springer), 93–108.
Ioakeimidis, C., Papatheodorou, G., Fermeli, G., Streftaris, N., and Papathanassiou, E. (2015). Use of ROV for assessing marine litter on the seafloor of saronikos gulf (Greece): A way to fill data gaps and deliver environmental education. SpringerPlus 4 (1), 463. doi:10.1186/s40064-015-1248-4
Jeftić, Lj., Sheavly, S., and Adled, E. (2009). Marine litter: A global challenge. Nairobi, Kenya: UNEP, 232.
Jin, S., Sun, W., Bao, J., Liu, M., and Cui, Y. (2015). “Sound velocity profile (SVP) inversion trough correcting the terrain distortion,” in International hydrographic (Dalian Naval Academy, China: Department of Hydrography and Cartography).
Kalacska, M., Lucanus, O., Sousa, L., Vieira, T., and Arroyo-Mora, J. P. (2018). Freshwater fish habitat complexity mapping using above and underwater structure-from-motion photogrammetry. Remote Sens. 10 (12), 1912. doi:10.3390/rs10121912
Kostylev, V. E., Todd, B. J., Fader, G. B., Courtney, R. C., Cameron, G. D., and Pickrill, R. A. (2001). Benthic habitat mapping on the Scotian Shelf based on multibeam bathymetry, surficial geology and sea floor photographs. Mar. Ecol. Prog. Ser. 219, 121–137. doi:10.3354/meps219121
Kühn, S., Bravo Rebolledo, E. L., and van Franeker, J. A. (2015). Deleterious effects of litter on marine life. Mar. Anthropog. Litter, 17, 75–116. doi:10.1007/978-3-319-16510-3_4
Kwasnitschka, T., Hansteen, T. H., Devey, C. W., and Kutterolf, S. (2013). Doing fieldwork on the seafloor: Photogrammetric techniques to yield 3D visual models from ROV video. Comput. Geosciences 52, 218–226. doi:10.1016/j.cageo.2012.10.008
Kwokal, Ž., and Štefanović, B. (2009). Plutajući otpad iz mora zanemarivanje ne znači nepostojanje, Adriatic Boat Show 2009. Šibenik, Hrvatska: 17–21.
Lacharité, M., Brown, C. J., and Gazzola, V. (2018). Multisource multibeam backscatter data: Developing a strategy for the production of benthic habitat maps using semi-automated seafloor classification methods. Mar. Geophys. Res. 39 (1), 307–322. doi:10.1007/s11001-017-9331-6
Lallensack, R. (2018). This 12-year-old girl built a robot that can find microplastics in the ocean. available at: https://www.smithsonianmag.com/innovation/12-year-old-girlbuilt-robot-can-find-microplastics-ocean-180970607/.
Le Bouffant, N., Berger, L., and Lurton, X. (June, 2013). “Simple method for multiple soundings extraction for wide beamwidth echosounders,” in UAM 2013-1st international conference and exhibition on Underwater Acoustics (UA2013), (Corfu island, Greece.
Lim, A., Löhr, A., Savelli, H., Beunen, R., Kalz, M., Ragas, A., et al. (2017). Solutions for global marine litter pollution. Curr. Opin. Environ. Sustain. 28, 90–99, doi:10.1016/j.cosust.2017.08.009
Lusher, A. (2015). Microplastics in the marine environment: Distribution, interactions and effects. Mar. Anthropog. Litter, 245–307. doi:10.1007/978-3-319-16510-3_10
Madricardo, F., Foglini, F., Campiani, E., Grande, V., Catenacci, E., Petrizzo, A., et al. (2019). Assessing the human footprint on the sea-floor of coastal systems: The case of the venice lagoon, Italy. Italy. Sci. Rep. 9 (1), 6615. doi:10.1038/s41598-019-43027-7
Madricardo, F., Ghezzo, M., Nesto, N., Mc Kiver, W. J., Faussone, G. C., Fiorin, R., et al. (2020). How to deal with seafloor marine litter: An overview of the state-of-the-art and future perspectives. Front. Mar. Sci. 7, 505134. doi:10.3389/fmars.2020.505134
Maes, T., Barry, J., Leslie, H. A., Vethaak, A. D., Nicolaus, E. E. M., Law, R. J., et al. (2018). Below the surface: Twenty-five years of seafloor litter monitoring in coastal seas of north west europe (1992–2017). Sci. Total Environ. 630, 790–798. doi:10.1016/j.scitotenv.2018.02.245
Mayer, L. A., Raymond, R., Glang, G., Richardson, M. D., Traykovski, P., and Trembanis, A. C. (2007). High-resolution mapping of mines and ripples at the Martha’s Vineyard coastal observatory. IEEE J. Ocean. Eng. 32, 133–149. doi:10.1109/joe.2007.890953
Merlino, S., Paterni, M., Berton, A., and Massetti, L. (2020). Unmanned aerial vehicles for debris survey in coastal areas: Long-term monitoring programme to study spatial and temporal accumulation of the dynamics of beached marine litter. Remote Sens. 12, 1260. doi:10.3390/rs12081260
Mingor, (2020). Mjera 3.3.1. Izraditi nacionalni plan upravljanja morskim otpadom iz Programa mjera zaštite i upravljanja morskim okolišem i obalnim područjem Republike Hrvatske (NN 97/17) Plan gospodarenja morskim otpadom. Zagreb, Croatia: Ministarstvo gospodarstva i održivog razvoja.
Oliveira, F., Monteiro, P., Bentes, L., Henriques, N. S., Aguilar, R., and Gonçalves, J. M. S. (2015). Marine litter in the upper são vicente submarine canyon (SW Portugal): Abundance, distribution, composition, and fauna interactions. Mar. Pollut. Bull. 97, 401–407. doi:10.1016/j.marpolbul.2015.05.060
Pham, C. K., Ramirez-Llodra, E., Alt, C. H. S., Amaro, T., Bergmann, M., Canals, M., et al. (2014). Marine litter distribution and density in European seas, from the shelves to deep basins. PLoS ONE 9 (4), 95839. doi:10.1371/journal.pone.0095839
Pierdomenico, M., Casalbore, D., and Chiocci, F. L. (2019). Massive benthic litter funnelled to deep sea by flash-flood generated hyperpycnal flows. Sci. Rep. 9, 5330. doi:10.1038/s41598-019-41816-8
Price, D. M., Robert, K., Callaway, A., Hall, R. A., and Huvenne, V. A. (2019). Using 3D photogrammetry from ROV video to quantify cold-water coral reef structural complexity and investigate its influence on biodiversity and community assemblage. Coral Reefs 38 (5), 1007–1021. doi:10.1007/s00338-019-01827-3
Šiljeg, A., Barada, M., and Marić, I. (2018). Digitalno modeliranje reljefa, sveučilišni priručnik. Zagreb, Croatia: Sveučilište u Zadru, Alfa d.d.
Šiljeg, A., Jurišić, M., and Marić, I. (2016). Bathymetric measures of the lakes skradinski buk. Geod. List. 70 (3), 231–252.
Šiljeg, A., Marić, I., Domazetović, F., Cukrov, N., Lovrić, M., and Panđa, L. (2022). Bathymetric survey of the St. Anthony channel (Croatia) using multibeam echosounders (MBES)—a new methodological semi-automatic approach of point cloud post-processing. J. Mar. Sci. Eng. 10 (1), 101. doi:10.3390/jmse10010101
Simons, D. G., and Snellen, M. (2009). A Bayesian approach to seafloor classification using multi-beam echo-sounder backscatter data. Appl. Acoust. 70 (10), 1258–1268. doi:10.1016/j.apacoust.2008.07.013
Spedicato, M. T., Zupa, W., Carbonara, P., Fiorentino, F., Follesa, M. C., Galgani, F., et al. (2019). Spatial distribution of marine macro-litter on the seafloor in the northern Mediterranean Sea: The MEDITS initiative. Sci. Mar. 83, 257–270. doi:10.3989/scimar.04987.14A
Topouzelis, K., Papageorgiou, D., Suaria, G., and Aliani, S. (2021). Floating marine litter detection algorithms and techniques using optical remote sensing data: A review. Mar. Pollut. Bull., 170, 112675.d: doi:10.1016/j.marpolbul.2021.112675
Tubau, X., Canals, M., Lastras, G., Rayo, X., Rivera, J., and Amblas, D. (2015). Marine litter on the floor of deep submarine canyons of the Northwestern Mediterranean Sea: The role of hydrodynamic processes. Prog. Oceanogr. 134, 379–403. doi:10.1016/j.pocean.2015.03.013
Url 1, . Jadransko more https://enciklopedija.hr/natuknica.aspx?ID=28478.
Url 2, . Fast 3D scanner for professionals https://www.artec3d.com/portable-3d-scanners/artec-eva/#specifications.
Url 3, Inertial Navigation package with Hemisphere V123 Satellite Compass, WASSP WSP-038 INU and Junction Box w/5M cables https://www.furunousa.com/en/products/wsp002-inu.
Vlachogianni, T., Fortibuoni, T., Ronchi, F., Zeri, C., Mazziotti, C., Tutman, P., et al. (2018). Marine litter on the beaches of the Adriatic and Ionian Seas: An assessment of their abundance, composition and sources. Mar. Pollut. Bull. 131, 745–756. doi:10.1016/j.marpolbul.2018.05.006
Keywords: marine litter, multi-beam echo sounder (MBES), remotely operated underwater vehicle (ROV), precision assessment, photogrammetry
Citation: Šiljeg A, Marić I, Krekman S, Cukrov N, Lovrić M, Domazetović F, Panđa L and Bulat T (2023) Mapping of marine litter on the seafloor using WASSP S3 multibeam echo sounder and Chasing M2 ROV. Front. Earth Sci. 11:1133751. doi: 10.3389/feart.2023.1133751
Received: 29 December 2022; Accepted: 12 April 2023;
Published: 24 April 2023.
Edited by:
Ali Khenchaf, UMR6285 Laboratoire des Sciences et Techniques de l’Information, de la Communication et de la Connaissance (LAB-STICC), FranceReviewed by:
Rozaimi Che Hasan, University of Technology Malaysia, MalaysiaCopyright © 2023 Šiljeg, Marić, Krekman, Cukrov, Lovrić, Domazetović, Panđa and Bulat. This is an open-access article distributed under the terms of the Creative Commons Attribution License (CC BY). The use, distribution or reproduction in other forums is permitted, provided the original author(s) and the copyright owner(s) are credited and that the original publication in this journal is cited, in accordance with accepted academic practice. No use, distribution or reproduction is permitted which does not comply with these terms.
*Correspondence: Ivan Marić, aW1hcmljMUB1bml6ZC5ocg==
Disclaimer: All claims expressed in this article are solely those of the authors and do not necessarily represent those of their affiliated organizations, or those of the publisher, the editors and the reviewers. Any product that may be evaluated in this article or claim that may be made by its manufacturer is not guaranteed or endorsed by the publisher.
Research integrity at Frontiers
Learn more about the work of our research integrity team to safeguard the quality of each article we publish.