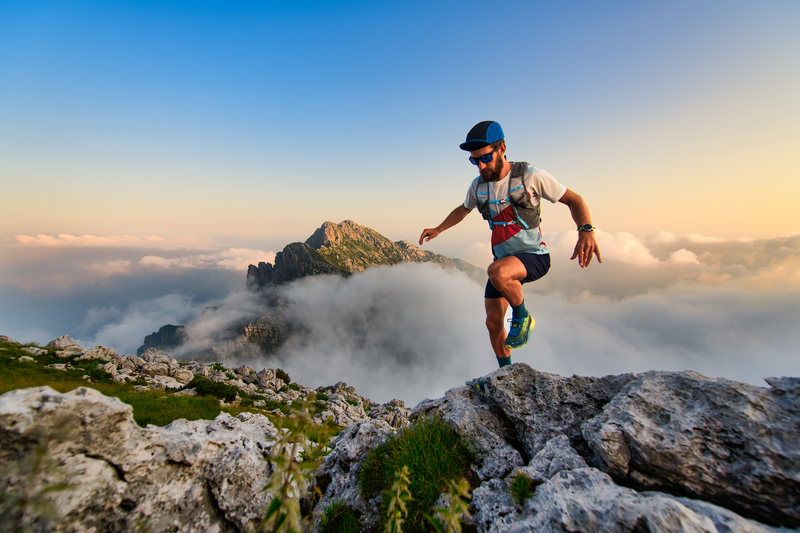
94% of researchers rate our articles as excellent or good
Learn more about the work of our research integrity team to safeguard the quality of each article we publish.
Find out more
ORIGINAL RESEARCH article
Front. Earth Sci. , 04 April 2023
Sec. Volcanology
Volume 11 - 2023 | https://doi.org/10.3389/feart.2023.1088992
This article is part of the Research Topic Remote Sensing of Volcanic Gas Emissions from the Ground, Air, and Space View all 18 articles
Since its introduction to volcanology in the mid-2000 s, the SO2 camera has become an important instrument for the acquisition of accurate and high time-resolution SO2 emission rates, aiding in hazard assessment and volcanological research. However, with the exception of a few locations (Stromboli, Etna, Kīlauea), hitherto the majority of measurements have been made on discrete field campaigns, which provide only brief snapshots into a volcano’s activity. Here, we present the development of a new, low-cost, low-power SO2 camera for permanent deployment on volcanoes, facilitating long-term, quasi-continuous (daylight hours only) measurements. We then discuss preliminary datasets from Lascar and Kīlauea volcanoes, where instruments are now in continuous operation. Further proliferation of such instrumentation has the potential to greatly improve our understanding of the transient nature of volcanic activity, as well as aiding volcano monitoring/eruption forecasting.
Volcanic emissions are the manifestation of subsurface magmatic activity, therefore providing an insight into the state of a volcanic system and potentially providing a means of hazard forecasting through their measurement (e.g., Aiuppa et al., 2009; De Moor et al., 2016; Kunrat et al., 2022). Sulphur Dioxide (SO2) is the most common gas species detected at open-vent volcanoes with remote sensing instrumentation, owing to its relative ease of detection that stems from two principal factors: Its relatively low background atmospheric concentration and its strong distinctive absorption bands at ultraviolet (UV) and infrared (IR) wavelengths (Platt and Stutz, 2008).
Since their development in the mid-2000s, ultraviolet (UV) SO2 cameras (Mori and Burton, 2006; Bluth et al., 2007) have become extremely valuable tools for measuring volcanic emissions, due to their provision of high temporal (at times >1 Hz) and high spatial resolution (providing two-dimensional, 2D, plume images) datasets. However, unlike the similarly popular differential optical absorption spectroscopy (DOAS) instruments, which have been installed as permanent/continuously operating instruments on a number of open-vent volcanoes worldwide (Burton et al., 2009; Galle et al., 2010; Arellano et al., 2021), SO2 camera technology is yet to proliferate in this regard; to the best of our knowledge, previous permanent systems have been installed on only Stromboli (Burton et al., 2015), Etna (D’Aleo et al., 2016; Delle Donne et al., 2017), and Kīlauea (Kern et al., 2015), with the latter having to be removed from the field during the 2018 caldera collapse. Further installations may have been prevented, in part, by the cost of such equipment, which typically utilizes scientific grade UV cameras (≈1000s–10000 s USD per camera; 2 cameras per SO2 camera system).
Discrete field campaigns with SO2 cameras have regularly been conducted, as they are often easier and cheaper to undertake; however, recent research has emphasized the importance of gathering long-term time series, since a volcano can exhibit significant changes in activity over a wide range of time scales (e.g., Pering et al., 2019). Such datasets form the foundation of volcano monitoring, which often relies on identifying the departure of a volcano’s activity away from some baseline (Phillipson et al., 2013), and also allow more detailed/reliable volcanological research. Indeed, the permanent SO2 cameras currently in operation have already provided important new insights into volcanic activity, as well as instrument performance. On Etna, D’Aleo et al. (2016) captured shifts in activity from one vent to another over the course of an eruptive period, inferring shallow interconnections between the vents of the volcano. Delle Donne et al. (2019) observed a mild but detectable increase in SO2 emission rate prior to the onset of paroxysmal activity on Etna in May 2016. At Stromboli, Burton et al. (2015) found reasonable agreement of camera data with a scanning DOAS network, whilst also highlighting that the high temporal resolution allows quantification of explosive events which are too brief to be captured by the scanning DOAS network. Delle Donne et al. (2017) then found that the frequency of explosion/puffing activity at Stromboli increased significantly prior to the onset of Stromboli’s August-November 2014 effusive activity. More recently on Stromboli, Delle Donne et al. (2022) found that explosive degassing accounts for ≈10% of the total SO2 emission budget, over a year-long observation period. They further showed that explosive SO2 flux correlates well with both very-long-period (VLP) seismicity and passive SO2 flux, inferring some commonality in the source processes involved in passive and explosive activity. In a somewhat different application, Elias et al. (2018) acquired highly-accurate SO2 emission rates by combining UV camera-derived plume velocities with SO2 measurements made by an array of upward-looking UV spectrometers. Their approach integrates the benefit of sophisticated DOAS retrievals of SO2 column densities with the benefit of image-based plume speed estimation.
The work herein aims to contribute to the aforementioned growing need for long-term, quasi-continuous SO2 emission rate data from active volcanoes. We present a new SO2 camera which has significant potential to broaden the use of permanent SO2 cameras in volcanology, owing largely to its relatively low-cost and low-power design, as well as its associated open-source code for data processing. The instrument is based on previously developed Raspberry Pi-based UV camera technology that exploits low-cost visible imaging cameras, modifying them to improve their UV sensitivity (Wilkes et al., 2016; Wilkes et al., 2017). We discuss installations of the system on 2 volcanoes, Lascar (Chile) and Kīlauea (Hawaii, United States), presenting preliminary datasets from both. We note that the instrument has also been installed on two other volcanoes (El Reventador, Ecuador; Lastarria, Chile); however, we do not yet have data from these instruments so they are not discussed further herein.
The SO2 camera works by exploiting the significant absorption of ultraviolet (UV) light by SO2. Images are acquired at two distinct wavelengths (bands), using bandpass filters (≈10 nm full width at half maximum; FWHM) inserted into the camera optics. The on-band is located in a spectral region where SO2 absorption is significant (≈310 nm), whilst the off-band is located in a nearby region where SO2 absorption has diminished to be negligible in comparison (≈330 nm). Using the Beer-Lambert law of absorption, plume pixels in an image can be compared to the background sky radiance to calculate pixel optical depths in each band:
where
SO2 optical depth can be calibrated to SO2 column densities using either gas cells of known SO2 amounts or a co-aligned spectrometer retrieving contemporaneous SO2 column densities from a small region within the field of view of the camera (Lübcke et al., 2013). For permanent SO2 camera installations, the latter method is universally employed, since gas cell calibration would require the incorporation of moving parts into the system, such as a filter wheel, to cycle through cell calibrations a number of times each day. Moving parts are likely to result in failing components over the lifetime of a camera system, as well as adding more complexity to the initial design and construction. Furthermore, contemporaneous spectroscopic measurements can allow for more sophisticated retrievals, including correction for light dilution and in-plume scattering (Kern et al., 2013; Varnam et al., 2021).
An emission rate is retrieved by multiplying a plume cross-sectional mass (often referred to as integrated column amount; ICA) by the plume speed. Plume speed is primarily calculated by one of two methods: 1) cross-correlating time series from two parallel ICAs, one set downwind of the other, and therefore calculating the lag in plume motion over the series; 2) computer vision algorithms, principally optical flow, which estimate the motion of all features in an image from one frame to the next (e.g., Peters et al., 2015). The latter provides both a higher spatial (theoretically down to pixel-level) and temporal resolution (frame-by-frame) to plume speed estimation, relative to cross-correlation. An alternative cross-correlation technique, which can also provide frame-by-frame temporal resolution, uses a single plume transect line, this time parallel to plume motion, and cross-correlates this spatial profile in successive images to estimate velocity (Nadeau, 2011; Nadeau et al., 2011). However, this is less commonly found in the literature to date and, thus, is not currently available in our processing software.
The instrument makes use of sensors from Raspberry Pi cameras, which have been modified to enhance UV sensitivity by removal of their Bayer filter (Wilkes et al., 2016). This approach provides a significant cost reduction relative to the use of scientific-grade commercial UV cameras—100 s USD versus 1,000–10000 s USD for this component of the instrument (Wilkes et al., 2017). Following sensor modification, a UV transmissive optical system, designed in Zemax ray tracing software, was constructed and mounted to the sensor. A triplet design was used to improve upon the previous lower-cost plano-convex singlet (Wilkes et al., 2016; Wilkes et al., 2017), which suffered somewhat from spherical aberrations at the edges of images and had a reasonably low light throughput. Unlike our previous work, which constructed optics holders from three-dimensional (3D) prints, here the triplet mount was constructed in machined black anodised aluminium, for improved precision and longevity. The resulting instrument field of view (FOV) is 28˚ x 21˚. The UV bandpass filters were mounted behind the triplet, to mitigate the effects of changing wavelength transmission that occurs with changing light incidence angle on the filter (Kern et al., 2010b).
The remainder of instrument design is similar to that of Kern et al. (2015), including a network switch for internal and external communications and a co-aligned spectrometer for calibration of the camera optical depths (Figure 1). The most notable difference, other than the different UV cameras used (which has a considerable influence on the overall cost of the instrument), is our omission of a thermoelectric cooler (TEC) for temperature control of the spectrometer. Whilst previous versions of the Ocean Optics (now Ocean Insight) spectrometers have shown notable temperature instabilities (pixel-wavelength calibration shift and change in instrument line shape with changing temperature), the relatively new Flame spectrometer series (c. 2015) has a much-improved thermal stability, both as quoted by the manufacturer and under external testing (see Supplementary Material S1). We therefore omitted the TEC, thereby significantly decreasing the power consumption of the instrument (the TEC consumed 3 W in Kern et al., 2015), as well as saving costs and reducing instrument complexity.
FIGURE 1. (A) Layout of permanent SO2 camera. Most parts are mounted to the case using custom-designed three-dimensional prints. Whilst this system is housed inside a Peli Case 1,400 (306 × 234 × 130 mm), other systems have used the Peli Case 1,450 (380 × 266 × 153 mm) to provide extra space for mounting the system. (B) Front view of instrument optical systems.
Power consumption of the system is relatively low, since the camera utilises a low-power CMOS sensor. All components of the instrument run from 5 V; however, the instrument contains a 12 to 5 V voltage regulator inside, such that the instrument accepts standard 12 V power supplies such as car batteries. Overall power consumption is summarised in Table 1. In full operation the instrument consumes a maximum of 12 W; however, in most cases, operation will occur during only a small fraction of a day, especially since the instrument cannot acquire at night due to the lack of UV radiation. Controlled by the scheduling on the WittyPi HAT, the Raspberry Pi components of the instrument can be powered down when not in operation, leaving only the network switch still running; this consumes 1 W, therefore a time-averaged power consumption (assuming 6 h of operation), may be as little as ≈3.75 W. This is approximately half the power of the system presented in Kern et al. (2015), which consumes ≈25 W in operation and ≈2 W in sleep mode. In locations where light conditions for solar power are poor, such as at high latitudes or regularly cloudy areas, this difference will be particularly beneficial. Lower power consumption should also mean that the instrument can be run on fewer and/or smaller solar panels and batteries, again further reducing the overall cost of the installation.
Overall, the cost of parts for a one-off instrument (bulk purchasing would reduce part costs) is ≈5000 USD, which is primarily controlled by the cost of the UV spectrometer (≈3500 USD for spectrometer and associated optics). Note, these costs do not include data transmission or powering options, which will vary from installation to installation, depending on what existing infrastructure a group may have or the availability of technology in specific countries/regions. Whilst this cost is a notable increase on our discrete field campaign instrument cost (≈500 USD), it remains considerably cheaper than the current alternatives, which employ scientific-grade UV cameras. For example, the instrument presented by Kern et al. (2015) had a part cost (not adjusted for inflation) of ≈18,000 USD (C. Kern, personal communication), not including software licenses which were also 1000 s USD; our use of open-source software also avoids this additional cost.
Data acquisition is controlled by software written in Python 3, which is freely available at https://github.com/twVolc/PyCamPermanent. With the aim of making instrument control and processing of SO2 camera data as accessible as possible, the code provides a graphical user interface (GUI) such that users should not need significant coding knowledge to work with the instrument. The principal aim is to ensure that robust and high-quality data capture and processing is as straightforward as possible for users.
The package contains a number of scripts that control the two cameras and spectrometer through a master script (“pycam_masterpi.py”), which also handles external communication (e.g., for adjusting settings or manual acquisition control). Once the instrument has been setup to acquire automatically, it can then run in a headless state, where connection to the GUI or an external computer is not required.
Automated image capture requires the automatic adjustment of camera and spectrometer shutter speed/integration time to avoid pixel saturation whilst ensuring sufficient light levels to obtain a good signal-to-noise ratio. To this end, image analysis performed on the instrument assesses the level of pixel saturation (relative to the maximum digital number measurable by the sensor—Defined by the sensor bit-depth) in every image/spectrum. User-defined parameters then allow the instrument to determine whether an increase or decrease in shutter speed/integration time is required for each image/spectrum. For images, the user predefines lower (a) and upper (ß) limits for maximum pixel saturation (as a fraction), the number of pixels to average for this analysis (X), the number of image rows to use for the analysis, and whether to extract these rows from top-down or bottom-up. The option of only analysing a set number of rows allows us to only interrogate sky pixels in the analysis, omitting irrelevant pixels on the volcano flank. This is particularly important for snow-covered volcanoes, where snow-covered pixels appear very bright and would lead to an unwanted decrease in shutter speed if they were included in the analysis—It is important to optimise sky pixel intensity rather than the image as a whole. After this region of interest (ROI) has been extracted from the image, the brightest X pixels are found and the average digital number (DN) computed. Note, we choose to average a number of pixels rather than analysing only the brightest pixel, since erroneous “hot pixels” could interfere with the analysis. The saturation level of this average DN relative to the bit-depth DN is then computed. If this value is below α, the shutter speed of the next image will be increased; if the value is above β, the shutter speed of the next image will be decreased; for levels within the range α to β, the shutter speed will remain the same. For spectrometer integration time, the principle is the same; however, the user predefines a spectral window in which the saturation analysis takes place, rather than a spatial ROI. Typically this window may be 310–340 nm, ensuring that pixels do not become saturated in the spectrometer fitting window.
The instrument holds a 1 TB SSD external storage device. This is the principal location for data storage. To ensure a backup, the data are also saved on the Raspberry Pi micro-SD card (128 GB), which can hold at least 100 GB of data (since it also holds the operating system of the Raspberry Pi). Images have a file size of 617 KB and spectra are 33 KB; of course, data accumulation depends on the acquisition rate and acquisition length per day. As a typical example, acquiring at a rate of 0.2 Hz for 6 h a day will generate ≈165 GB per month (2 TB per year); the storage will therefore be entirely full in 6 months, whilst back-up storage lasts less than 1 month. If frequent trips to download data are not possible, and data back-up is critical, an extra SSD external storage device could be added with relative ease; alternatively, larger SSDs are becoming more readily available. Data compression could also be explored in the future, to improve storage requirements. Where possible, telemetry of data would be desirable to allow near-real-time emission rate estimates and prevent the necessity for frequent trips to the instrument.
Data processing can also be performed using the freely available PyCam software (https://github.com/twVolc/PyCamPermanent). Alongside in-house developed code, much of the processing makes use of the pyplis toolbox (Gliß et al., 2017), whilst spectrometer SO2 retrievals are performed with iFit (Esse et al., 2020); light dilution correction of the spectrometer retrievals is also made possible using code associated with (Varnam et al., 2020). Information on the processing routines available can be found in those articles, and it is not within the scope of this paper to provide a detailed review of these routines. Using example datasets from Lascar and Kīlauea, Section 4 provides more details on some of the data processing algorithms available.
We note that absolute automation of SO2 camera data processing remains troublesome, often requiring an expert user’s retrospective interaction to ensure that processing routines have been optimally applied and to interrogate data quality. Others have achieved at least reasonable levels of automation (e.g., Kern et al., 2015; Delle Donne et al., 2017; Delle Donne et al., 2019), for instance, with the incorporation of a visibility index to omit data with poor visibility (Delle Donne et al., 2017) and a closed-path semi-circular integration line to capture gas emissions for all plume orientations (Kern et al., 2015). Of particular note, Delle Donne et al. (2019) investigated the difference between manual and automatic processing procedures, finding a reasonable agreement between their automatic processing routine and manual expert analysis (r2 ≈ 0.75); however, there clearly remains a notable disparity between the two, and this MATLAB-based (requiring a license) automation software is not open-source. In this respect, our work herein does not present any further steps in automation routines; however, it does provide open-source, user-friendly (with a graphical user interface) software which can promote the accessibility of SO2 cameras to the wider volcanology community.
Data herein were processed with some manual interaction, for example, with the plume cross-sections optimized for the plume direction in each time series. Our software does, however, allow the use of multiple cross-sections, which would allow a quasi-semi-circular integration line, as in Kern et al. (2015), ensuring the capture of gas emissions for all plume orientations; future software development will include the option to use true semi-circular lines. Background sky intensities also require manual derivation at times, although automated procedures, which harness the pyplis functionality, are available within our GUI. In general, we have found that at times of non-perfect conditions (e.g., heterogeneous cloud cover), it was optimal to manually define the background sky region for an image sequence. Other automated background sky retrieval algorithms have been proposed (Osorio et al., 2017) and may offer improved performance when moving towards a fully automated processing procedure; again, this could be the focus of future software development.
Lascar (5,592 m; 23.37˚S, 67.73˚W) is a stratovolcano composed of 6 overlapping summit craters. It is predominantly andesitic-dacitic in composition. In 1993 a VEI 4 eruption created a column reaching 20–22 km altitude and resulted in ash fall as far away as Buenos Aires (1,500 km SE of the volcano) (Global Volcanism Program, 1993). Current activity is primarily confined to fumarolic activity within the active crater, although during the camera installation a few small explosions were possibly heard, albeit with no clear visible associated phenomena.
The camera system was installed on Lascar on 18th May 2022, approximately 4,300 m from the crater (Figure 2A). The camera location provides a near-orthogonal viewing direction relative to the prevailing westerly winds. However, the plume has a tendency to swirl and stagnate within Lascar’s crater before drifting eastwards. We also note that the front of the crater can at times obscure full view of the gas, which may lead to underestimations in emission rates at times when the plume does not loft above the crater. The installation is displayed in Figures 3A, B.
FIGURE 2. Digital elevation models (DEMs) of site locations with cameras shown as blue dots and their field of views extending from them, along with an example image from the on-band camera in each case. Red symbols indicate gas source locations. Typical plume motions are shown by yellow-headed arrows, but can vary significantly throughout the year. (A) Lascar (DEM from SRTM data); (B) Kīlauea (DEM from LiDAR data taken from Mosbrucker et al., 2020).
FIGURE 3. (A, B) Instrument installation on Lascar volcano, Chile (Photo credit: T. Wilkes). (C, D) Instrument installation on Kīlauea volcano, Hawaii, United States (Photo credit: T. Pering).
Kīlauea (1,247 m; 19.41° N, 155.28° W) is a shield volcano on the Island of Hawaiʻi. Starting in May 2018, a series of fissure eruptions in the lower East Rift Zone accompanied drainage from the Halemaʻumaʻu lava lake, which resulted in collapse of the summit caldera (Neal et al., 2019). During this period, SO2 emission rates were estimated to have reached at least 200 kt/day (2,300 kg/s) (Kern et al., 2020). These extreme conditions were modelled to have significantly impacted air quality hundreds of kilometres downwind of the volcano (Kern et al., 2020), whilst in the local vicinity lava flows and seismicity caused substantial damage and destruction of infrastructure (Neal et al., 2019).
The system was installed on Kīlauea on 21st July 2022, approximately 2,700 m from the crater (Figure 2B). The north-easterly trade winds are relatively consistent, resulting in right to left gas motion through the image, which is almost exactly orthogonal to the viewing direction of the camera. This installation was augmented with data telemetry (up to 20 MB/s) from the instrument to a local observatory, allowing near-real-time processing of the data. This will enable rapid integration of SO2 emission rates into hazard assessments at the volcano. The installation, along with radio antenna, is displayed in Figures 3C, D.
We here first present an in-depth analysis of a sample dataset retrieved shortly after camera installation on Lascar volcano, over the period 16:05:05–17:05:00 UTC on 20th May 2022. This is followed by a longer-term time series spanning 20th-21st May. Spectrometer FOV calibration is shown in Figure 4, along with the subsequent camera optical depth (τSO2) calibration. The FOV location is found through iterative calculation of the correlation coefficient between the spectrometer column density time series (Figure 4B) and τSO2 time series of each pixel individually (Lübcke et al., 2013; Gliß et al., 2017); Figure 4A displays the resulting correlation image and location of the spectrometer’s FOV within the image. Once this is known, the spectrometer FOV can be fixed for subsequent datasets if desired. A regression between the spectrometer column densities and τSO2 within the spectrometer’s FOV can then be formed (Figure 4C), therefore allowing calibration of all pixel τSO2 values. We note that the spectrometer’s FOV is positioned just above the crater, such that it should be located within gas for most plume motions; however, there may still be periods of time where it is not located within the gas plume. In such periods it may be necessary to revert back to older calibrations, either earlier in the day or from previous days. Over time, we will build a catalog of instrument calibrations, which may then allow more robust selection of calibrations during periods where the plume is not in the spectrometer’s FOV, for instance by identifying similar illumination conditions where a calibration was possible. A time series of the spectrometer SO2 slant column density (SCD) retrievals (Figure 4C) also highlights that the spectrometer FOV is positioned such that it typically captures a wide range of SCDs in relatively short periods (<30 min). This ensures that camera optical depth calibration is unlikely to require significant extrapolation to larger values, which would result in greater measurement uncertainties. In this case the relationship between optical depth and SCDs was assumed linear, which is often reasonable for low SCDs. However, Kern et al. (2013) found that for high SO2 burdens optical depths deviate significantly from the linear Beer-Lambert approximation, in part due to complex radiative transfer caused by in-plume aerosols and light dilution. The pyplis backend allows for non-linear regressions to be used in fitting camera optical depth to SCD, which can be changed within the pycam GUI.
FIGURE 4. (A) Pearson correlation coefficients (colour scale) of spectrometer SO2 retrievals with camera optical depths (τSO2), enabling determination of where the spectrometer field of view (FOV) is located within the camera image. In this case, the highest correlation coefficient is centered at pixel coordinate (270, 215). (B) Spectrometer-retrieved SO2 slant column density retrieval time series (in UTC), highlighting that the FOV location is well-positioned to measure a wide range of column densities through time, therefore allowing a robust calibration of the camera optical depths. (C) Spectrometer SO2 slant column density (SSO2) retrievals versus camera optical depths measured in the spectrometer’s FOV (as determined in A). The fitted calibration line is used to calibrate all pixel optical depths in the images.
Figure 5 shows a typical optical depth image from the instrument, also displaying the ICA line used to determine SO2 emission rates for the time series discussed here. Emission rates can be retrieved using 4 distinct plume speed estimation algorithms; these are outlined in detail by Gliß et al. (2017), Gliß et al. (2018) and briefly summarised in Table 2. The cross-correlation technique introduced in Section 2.1 is represented by flow_glob, whilst the 3 other algorithms (flow_raw, flow_histo, flow_hybrid) utilise optical flow vectors with varying degrees of post-analysis. Figure 6 displays these effective velocities and the associated emission rate estimations; for clarity, we omit flow_hybrid from the plot, but these effective velocities, and thus emission rates, were extremely similar to the flow_histo algorithm. We note that, in this case and in our experience more generally, the optical flow algorithms typically estimate lower plume speeds than cross-correlation (flow_glob). This may be a result of cross-correlation preferentially fitting to larger pulsing events of gas (driven either by volcanic or meteorological factors), which have the most distinctive signature–notably standing out from general noise in a degassing time series. This is supported by the higher optical flow speeds often coinciding with the larger mass loading along the ICA lines (peaks in the cross-correlation emission rate series); at these points the optical flow effective velocity is very similar to the cross-correlation plume speed (≈7.3 m/s in this case). In general, we therefore suggest the use of optical flow algorithms leads to more reliable emission rate estimates (Peters et al., 2015), especially the flow_histo (or flow_hybrid) algorithm (Gliß et al., 2018), which retrieves a local average flow velocity and can potentially mitigate unreliable retrievals from the raw Farnebäck algorithm. We note, however, that there are relatively frequent periods where the flow_histo algorithm fails, resulting in no emission rate for these points (e.g., see Figure 6 or the “flow_histo LD corrected” sheet in Lascar spreadsheet of supplementary materials). To account for such gaps, either interpolating between recent plume speeds, or reverting to the flow_raw or cross-correlation algorithm, are solutions. More detailed discussions of plume speed algorithms and associated uncertainties can be found elsewhere (Peters et al., 2015; Gliß et al., 2017; Klein et al., 2017; Elias et al., 2018; Gliß et al., 2018).
FIGURE 5. (A) Optical depth image of Lascar volcano from an acquisition at 14:00:35 UTC (10:00:35 CLT) on 25th May 2022. The black rectangle indicates the region of clear sky where the intensity I0 was estimated. (B) Optical depth values across integration lines shown in (A). The dark blue line was used for emission rate retrievals whilst the light blue was the secondary line used for cross-correlation plume speed estimation.
TABLE 2. Plume speed estimation algorithms, based on Gliß et al. (2017).
FIGURE 6. Emission rate (Φ) time series and associated effective velocity estimation (Veff) using 3 different plume speed estimation algorithms. The single cross-correlation velocity is faster than optical flow velocities for the majority of the series, but peak optical flow velocities match quite well with this cross-correlation speed. We suggest flow_histo (bold orange) is likely to provide the most reliable data in general; however, at times this algorithm is unable to compute a reliable speed, therefore some parts of this time series are absent. Data from Lascar volcano on 20th May 2022; times are in UTC.
For the series shown in Figure 6, cross-correlation emission rates ranged between 1.3 and 6.0 kg/s, with a mean of 2.8 ± 0.7 (±1 SD) kg/s, whilst flow_histo generated a mean emission rate of 2.0 ± 0.7 kg/s, with the series ranging between 0.4 and 4.9 kg/s. The emission rate time series shows clear pulsing behaviour at Lascar, although it is difficult to definitively attribute this to volcanic activity, since the gas typically accumulates somewhat in the crater before exiting to where it can be observed by remote sensing equipment. It is therefore possible that wind turbulence at the summit is influencing the retrieved time series.
The above results were generated neglecting a light dilution (LD) correction, the phenomenon where light is scattered into the FOV of the instrument between the plume and the instrument; such light has not passed through the plume, so does not contain absorption features of SO2, but contributes to the radiance at the detector. This can result in a significant underestimation of SO2 emission rates (Mori et al., 2006; Kern et al., 2010a). Campion et al. (2015) proposed an image-based correction light dilution for camera optical depths, based on the changing measured intensity of the volcanic flank as it gets closer to the observer. We implement this correction using the pyplis API, finding that even in the dry Atacama air at >4,500 m altitude, there is still significant light dilution in the images (Figure 7). However, using this correction alone is only applicable to gas cell calibrated systems. Spectrometer-calibrated instruments require that the column densities measured by the spectrometer have also been corrected for associated light dilution effects; in fact, the spectrometer correction is much more critical than camera optical depth calibration, since the final calibration links column densities to somewhat arbitrary optical depths.
FIGURE 7. Image-based light dilution processing. (A) On-band image extinction coefficient calculation. (B) Off-band image atmospheric extinction coefficient calculation. (C) Digital elevation model of the camera viewing geometry on Lascar volcano; camera field of view is displayed in green. Magenta and red lines indicate the location of the extracted lines in the image in relation to the topography. Data points from these lines are outlined in A and B by their respective colours.
To correct the spectrometer-retrieved column densities we used the dual-band method proposed by Varnam et al. (2020). However, we found that the correction gave quite stochastic light-dilution factors that ranged from anywhere between 0 and 1. Correcting each spectrum individually therefore introduced large errors which resulted in a poor correlation between the spectrometer column densities and the associated optical depths in the camera images. The high correlation presented in Figure 4 suggests that the column density trend is accurate, even if absolute values are affected by light dilution. Below, we therefore present a method for stabilising the light dilution correction. Following Varnam et al. (2020), we first calculated the light dilution factor (LDF) and the associated corrected column density for each spectrum in the time series. We suggest the light dilution correction algorithm is likely to perform better on spectra with larger column densities, since the dual-band technique should see more disparity between the two bands than if fitting to low column densities. We therefore then discarded all spectra measuring column densities below 1E18 molecules cm−2. We then took the median LDF of these retrievals, in this case 0.25. Looking at the full time series again, we extracted all data points within ±20% of the median LDF (0.20 ≤ LDF ≤ 0.30). From this subset, a relationship between corrected and uncorrected column densities can be found, which is relatively linear within the range of values found here. This relationship was thus used to correct the initial column density time series. The correction increased the column densities by between ≈30% and ≈70%, depending on the initial uncorrected column density. This correction maintained the high >0.8 correlation between the spectrometer column densities and the associated optical depths within the spectrometer’s FOV in the image series. We note, however, that this correction may be more complicated to implement in near-real-time when data are required rapidly for volcano monitoring purposes. Perhaps importantly, the general trends in the emission rates without a LD correction (Figure 6) are very similar to those with a LD correction (Figure 8), since the correction is relatively stable through time. This similartity suggests that emission rate time series without this correction could still have utility for rapid monitoring and response in real-time; the more accurate LD-corrected time series can then be generated post hoc.
FIGURE 8. (A) Emission rate (Φ) time series as in Figure 6 but corrected for light dilution. Blue shaded region shows the difference between the uncorrected and corrected emission rates for the flow_glob series. (B) A longer-term series spanning 20th-21st May based on flow_glob plume speeds (blue) with a 10-min moving average (red). Plume speed (veff) is also displayed and is relatively consistent across the 2 days, unlike the emission rate which is significantly higher on the 21st.
The resulting light dilution-corrected emission rate time series increased somewhat (Figure 8A), with a mean cross-correlation emission rate of 3.8 ± 1.3 kg/s, whilst the flow_histo plume speed produced a mean emission rate of 2.8 ± 1.2 kg/s. From these mean values, the uncorrected retrievals therefore underestimate emission rates by 26% and 29% for flow_glob and flow_histo, respectively. These values are in the middle of the range of the examples presented by Campion et al. (2015); however, with the high altitude and dry conditions at Lascar, the level of light dilution is slightly higher than we had anticipated, highlighting that light dilution is likely to be a significant source of error for any measurements made at greater than 1–2 km from a volcano.
The longer-term series (Figure 8B), based on the same processing procedure outlined above (including light dilution correction), highlights the instrument’s ability to perform shut-down/start-up sequences and adjust to changing light conditions throughout the day. Here, it is evident that the emission rates from the 2 days are significantly different. Whilst this could represent a true change in volcanic degassing, we cannot rule out the possibility that emission rates on 20th were incomplete measurements, with part of the plume being obscured by the crater as it drifted to the east. We note that plume speeds (veff) across the 2 days are relatively stable. The mean emission rate across the 2 days is 5.2 ± 3.1 kg/s, whilst the emission rate for only the 21st is 6.7 ± 3.1 kg/s. The emission rate is relatively variable, reaching a maximum of 17.5 kg/s; as mentioned above, this could be volcanogenic in nature but may also be associated with gas accumulation in the crater and subsequent large ejections driven by metrological conditions (e.g., wind turbulence).
Recent SO2 camera measurements, in January 2019, are in good agreement with our measurements (4.7 ± 1.4 kg/s), suggesting relative stability of the volcanic system over the last few years (Layana, 2022). However, Layana (2022) also found that, during a period where satellite-derived thermal anomalies at the volcano were absent, SO2 emissions (measured in March and June 2018) were significantly lower—close to or below the detection limit of their SO2 camera. This highlights the importance of more continuous monitoring, which can identify changes in activity over a broad range of timescales (from the order of minutes to sub-annual/annual and beyond); Thus, again we emphasize that discrete field campaigns cannot provide a complete picture of a volcano’s state. Our measurements are somewhat higher than NOVAC scanner data between 2013–2016, which retrieved a mean of 2.6 ± 1.4 kg/s (Bucarey et al., 2020; Arellano et al., 2021). This may be a result of changing subsurface conditions in recent years, or a product of the longer-term nature of the NOVAC time series incorporating days of lower activity too. Discrete campaigns have also measured a broad range of emission rates on Lascar: 6.4 ± 2.5 kg/s in December 2012 (Tamburello et al., 2014); 27.8 kg/s in January 2003 (Mather et al., 2004). The general trend appears to be a steady decrease in SO2 degassing (≈2003–2016), with perhaps a new increase in emissions in the last few years; this is something which the new installation will be able to elucidate further in the coming months/years.
We present here data acquired from Kīlauea volcano on 25th July 2022. Figure 9 displays a representative optical depth image acquired by the camera. Within the time period of this camera dataset, 9 traverse-mode DOAS measurements were also made from a vehicle transecting the plume ≈1.5 km downwind of the Halemaʻumaʻu Crater. Wind speeds for emission rate calculations of these traverses were retrieved from a weather station located at Sand Hill, about 2 km west of the active vent. These were then scaled by a factor of 1.2 to account for systematic underestimation of the wind speeds aloft by our ground-based measurements (Elias et al., 2018). Spectral and spatial analysis of the traverse data was performed according to standard DOAS analysis procedures (Platt and Stutz, 2008) using the DOASIS software (Kraus, 2006) in combination with a custom MATLAB code (“mDOAS”) specifically developed for spatial analysis of Mobile DOAS measurements.
FIGURE 9. (A) Optical depth image of Kīlauea volcano from an acquisition at 20:21:35 UTC (10:21:35 HST) on 25th May 2022. The black rectangle indicates the region of clear sky where the intensity I0 was estimated. (B) Optical depth values across integration lines shown in (A). The dark blue line was used for emission rate retrievals whilst the light blue was the secondary line used for cross-correlation plume speed estimation.
Using the same method as in Section 4.1, we corrected the iFit spectrometer retrievals for light dilution. In this case, the image-based correction was not possible, due to the viewing geometry of the camera not providing enough volcanic flank. However, we propose that a first order light dilution correction should be possible simply by correcting the spectrometer retrievals, if we assume that image optical depths are uniformly affected by light dilution and that light dilution magnitude does not change significantly within a calibration window (typically 30–60 min). These assumptions are not strictly correct, however, since the correlation between image optical depth and column density (e.g., as in Figure 4B) remains strong following the spectrometer light dilution correction, correcting only the spectrometer retrievals may be adequate in many cases.
The traverse and camera datasets are displayed in Figure 10. Due to the significant differences in sampling frequency, and measurement location, exact comparison between the values is somewhat complicated and includes caveats; nevertheless, a basic comparison can still be useful. The 8 early DOAS traverses found an average emission rate of 18.7 ± 8.0 (±1 SD) kg/s, with a minimum measurement of 6.2 kg/s and maximum of 27.6 kg/s. Whilst there is a disparity, these measurements are in reasonably good agreement with the camera’s contemporaneous first acquisition period (≈20:00–21:30 UTC), which has a mean emission rate of 12.7 ± 5 kg/s and a maximum of 28.0 kg/s. For the period 11:00–01:00 UTC (25/26th July), the difference in emission rates is more notable, with the single traverse measurement of 23.5 kg/s, whilst the mean camera emission rate was just 7.5 ± 3.0 kg/s.
FIGURE 10. Permanent camera SO2 emission rate (Φ) time series at Kīlauea on 25th and 26th July 2022 (UTC; all measurements were 25th July HST), with contemporaneous DOAS traverse measurements (red dots). Camera retrievals were made with the cross-correlation (flow_glob) plume speed estimation algorithm. The magnitude of the light dilution correction (applied through the instrument’s spectrometer iFit retrievals) is displayed by the shaded blue region. Agreement between the camera and traverse measurements is reasonably good for the 25th measurements, whilst on the 26th the SO2 camera retrieves significantly lower emissions rates, albeit with only one traverse measurement for comparison in this sequence. Note that the traverse measurements were performed ≈1.5 km downwind of the source, therefore with a plume speed of ≈8–10 m/s we may expect a lag in measurements of ≈150–190 s.
It is not clear what has caused this large disparity in the second period (albeit comparing with only one contemporaneous traverse measurement), although some possible influences may be: differences in wind speed estimates (and the sources of these estimates); In-plume aerosol scattering of light; incomplete capture of the full gas plume by the camera, due to its viewing geometry and the crater geometry; non-orthogonal plume motion relative to camera viewing direction (e.g., Klein et al., 2017). Regarding the latter, using the plume centers based on traverse measurements, we can calculate the difference between the plume’s motion and the camera’s focal plane azimuth. For the first acquisition period the difference ranged between 13˚ and 25˚, whilst for the second period this difference was 17˚. Although this can lead to an underestimation in emission rates, since the second period’s angular deviation falls within the bounds of the first period, it is unlikely that this is the principal cause for the larger emission rate disparities in the second period. Alternatively, incomplete capture of the gas plume by the SO2 camera could be a notable source of error for the camera measurements. The low lying crater geometry means that at times the plume can hug the ground, therefore making it difficult to fully capture emissions close to the source with the SO2 camera. This would be particularly notable in stronger wind conditions, which would reduce the degree of plume lofting as it leaves the crater. It is difficult to quantify this error, but it certainly could contribute to a significant underestimation in emission rates. Using optical flow motion vectors, it should be possible to identify periods where plume motion is less buoyant (more horizontal motion) and flag such periods as potentially having unreliable emission rate estimates; longer-term datasets will allow deeper investigation into this.
Disparities in plume speeds, and associated emission rates, are somewhat more quantifiable; Although we note that direct comparisons may be troublesome, since the SO2 camera images and weather stations are not collocated. Furthermore, neither technique is a direct measurement of the plume’s velocity at its altitude above the traverse measurements. Details of the methodology employed for this comparison are outline in Supplementary Material S2. During the final traverse (23:56 UTC), in the second camera acquisition period, corrected image-based horizontal plume speeds had a mean of 6.0 m/s for optical flow analysis, whilst cross-correlation measured 9.0 m/s. As discussed in Section 4.1, the discrepancy is likely due to cross-correlation fitting to large pulses of gas exiting the crater, which are likely relate to larger gusts of wind and/or changes in volcanic degassing vigor. Indeed, much like in Figure 6, the optical flow speeds reach similar (and slightly larger) values at times; however, for the specific 10-min period of the traverse (adjusted for time for the plume to drift from crater to traverse location), the values are notably lower. The Sand Hill weather station measurement at this time resulted in a scaled plume speed of 10 m/s. Using our plume measurements to rescale the traverse emission rates, we find emission rates of 14.1 and 21.2 kg/s for optical flow and cross-correlation, respectively. Whilst these values remain higher than the SO2 camera average for this period, the camera data reach 12.9 kg/s at 23:53:15 UTC and 13.8 kg/s at 23:54:30 UTC; the center of the traverse was reached at 23:56:59 UTC and is located ≈2–3 min downwind (1.5 km from crater). For comparison, the difference between camera-derived and weather station-derived plume speeds for the first acquisition period (20:00–21:30 UTC) is markedly smaller; camera-derived speeds averaged 6.9 and 9.9 m/s, for optical flow and cross-correlation, respectively, whilst the weather station-derived speed was 9 m/s throughout.
Although it seems to be a contributing factor, it is very unlikely that the above plume speed difference is the only source of disparity between the traverse and SO2 camera measurements. Whilst the academic literature currently lacks long-term comparisons between SO2 camera measurements and traverse-/scanning-mode DOAS measurements, some previous work has found good agreement between DOAS traverses and UV camera data in discrete campaigns (Varnam et al., 2021). Conversely, however, de Moor et al. (2017) highlighted that their permanent scanning DOAS system systematically measured lower emission rates relative to contemporaneous traverse DOAS measurements. They suggest that one possible cause is having a sub-optimal scanning position that fails to capture the full plume in a large number of scans, due to variable wind direction blowing the plume away from fixed-location scanners. We note that similar issues, associated with camera location/viewing geometry, are particularly pertinent for permanent installations; during discrete field campaigns imaging location can be chosen on a daily (or sub-daily) basis depending on plume conditions. On many volcanoes it can be difficult to find a suitable viewing geometry to ensure that, given any wind direction and degassing strength, the camera will always be able to capture complete and robust emission rates. Indeed, in many cases it may be advisable to install multiple systems around a target, providing various vantage points that could facilitate more robust retrievals under a range of meteorological and volcanic conditions (e.g., Delle Donne et al., 2022). Scanning DOAS networks often do exactly this on the most comprehensively monitored volcanoes (Burton et al., 2009; Galle et al., 2010). The relatively low cost of our new permanent camera design would facilitate such ambitions. Additionally, we also suggest that longer-term comparisons between SO2 cameras and scanning-/traverse-mode DOAS measurements, as well as satellite retrievals (e.g., TROPOMI), are critical to understanding the validity of each measurement technique under a wide range of conditions.
We have presented the design of a new permanent UV camera system for volcanic SO2 emission rate measurements, followed by installation of the instrument on 2 active volcanoes (Lascar, Chile; Kīlauea, Hawaii, United States). The instruments make use of a suite of previously developed open-source Python packages to supplement custom software, ensuring robust/standardised and comprehensive data analysis. We have highlighted how the relatively low cost and power consumption of these instruments makes them suitable for wide-scale deployment on volcanoes in a range of different environments around the globe. Although we note that longer-term testing of instrument performance is still required, such instruments have the potential to provide valuable datasets for comparison with alternative ground-based remote sensing instruments (i.e., DOAS scanning networks and traverse measurements) as well as ground-truthing satellite retrievals.
We provide example datasets from Lascar (Chile) and Kīlauea (Hawaii, United States). At Lascar, mean emission rates of 5.2 ± 3.1 kg/s agree well with recent (2019) measurements but are somewhat higher than earlier (2013-2016) longer term NOVAC time series (2.6 ± 1.4 kg/s). On Kīlauea we presented a comparison with contemporaneous traverse-mode DOAS measurements, which showed a reasonable agreement, albeit with the traverse emission rates (18.7 ± 8.0 kg/s) typically being higher than the camera-derived rates (12.7 ± 5 kg/s for the first series). A later measurement series gave lower camera-derived emission rates that were typically half the value of a traverse measurement in this window. We found that differences in wind speed estimates likely contributed significantly to the disparity; however, it is likely that other effects contributed too (e.g., in-plume scattering of light, incomplete capture of the entire plume by camera measurements).
We particularly emphasise the importance of permanent/continuous monitoring equipment in volcanic gas measurements, since volcanic activity is notably transient and variable in nature over a wide span of timescales. Whilst of course having significant value to the scientific community, the danger of discrete field campaigns is in interpreting such measurements as representative of that volcano outside of the measurement window. In some branches of volcanology (e.g., seismology), continuous monitoring is already somewhat commonplace; however, although there are a number of installations for permanent gas monitoring (e.g., most notably NOVAC), campaign-style measurements are still routine in a number of places across the globe. This is likely influenced by the cost and complexity of permanent systems. The instrument presented herein has the potential to aid the transition to more continuous geochemical monitoring of hazardous volcanoes across the globe, which in turn stands to improve our understanding of hazardous volcanic events and inform eruption forecasts (Kern et al., 2022).
The original contributions presented in the study are included in the article/Supplementary Material, further inquiries can be directed to the corresponding author.
TW wrote the manuscript, developed the instrument and installed the instrument on Lascar. TP contributed to the manuscript, contributed to instrument development and installed the instrument on Kīlauea. FA contributed to instrument development. SL installed the instrument on Lascar and contributed to instrument development. PN and CK installed the instrument on Kīlauea; CK also contributed to instrument development. AM contributed to instrument development. MA contributed to field work in Chile. CZ contributed to instrument development.
TW acknowledges the support of a Leverhulme Early Career Fellowship (ECF-2020-107). We acknowledge the University of Sheffield Institutional Open Access Fund for covering the publication fees of this manuscript. For the purpose of open access, the author has applied a Creative Commons Attribution (CC BY) licence to any Author Accepted Manuscript version arising. This work has been partially funded by FONDECYT Regular project 1211220 and Antofagasta Regional Government, FIC-R project, code BIP N°30488832-0. SL is funded by Millennium Institute on Volcanic Risk Research - Ckelar Volcanoes, Millennium Scientific Initiative, code ICN2021_038. MA is funded by FONDECYT Regular project 1211220 and Master fellowship from Postgraduate Direction, Universidad Católica del Norte, Chile.
We would like to thank two reviewers whose comments have greatly improved the manuscript. TW would like to thank Jonas Gliß, Benjamin Esse and Mathew Varnam for their development of, and subsequent support with, their valuable Python packages. We also acknowledge the support of Silvana Hidalgo and colleagues at IGEPN for their work on the installation of an instrument on El Reventador, Ecuador. Any use of trade, firm, or product names is for descriptive purposes only and does not imply endorsement by the U.S. Government.
The authors declare that the research was conducted in the absence of any commercial or financial relationships that could be construed as a potential conflict of interest.
All claims expressed in this article are solely those of the authors and do not necessarily represent those of their affiliated organizations, or those of the publisher, the editors and the reviewers. Any product that may be evaluated in this article, or claim that may be made by its manufacturer, is not guaranteed or endorsed by the publisher.
The Supplementary Material for this article can be found online at: https://www.frontiersin.org/articles/10.3389/feart.2023.1088992/full#supplementary-material
Aiuppa, A., Federico, C., Giudice, G., Giuffrida, G., Guida, R., Gurrieri, S., et al. (2009). The 2007 eruption of Stromboli volcano: Insights from real-time measurement of the volcanic gas plume CO2/SO2 ratio. J. Volcanol. Geotherm. Res. 182, 221–230. doi:10.1016/j.jvolgeores.2008.09.013
Arellano, S., Galle, B., Apaza, F., Avard, G., Barrington, C., Bobrowski, N., et al. (2021). Synoptic analysis of a decade of daily measurements of SO&lt;sub&gt;2&lt;/sub&gt; emission in the troposphere from volcanoes of the global ground-based Network for Observation of Volcanic and Atmospheric Change. Earth Syst. Sci. Data 13, 1167–1188. doi:10.5194/essd-13-1167-2021
Bluth, G. J. S., Shannon, J. M., Watson, I. M., Prata, A. J., and Realmuto, V. J. (2007). Development of an ultra-violet digital camera for volcanic SO2 imaging. J. Volcanol. Geotherm. Res. 161, 47–56. doi:10.1016/j.jvolgeores.2006.11.004
Bucarey, C., Arellano, S., Velasquez, G., and Galle, B. (2020). SO2 flux of Lascar volcano from the NOVAC data-base. doi:10.17196/novac.lascar.001
Burton, M. R., Caltabiano, T., Murè, F., Salerno, G. G., and Randazzo, D. (2009). SO2 flux from Stromboli during the 2007 eruption: Results from the FLAME network and traverse measurements. J. Volcanol. Geotherm. Res. 182, 214–220. doi:10.1016/j.jvolgeores.2008.11.025
Burton, M. R., Salerno, G. G., D’Auria, L., Caltabiano, T., Murè, F., and Maugeri, R. (2015). SO2 flux monitoring at Stromboli with the new permanent ingv SO2 camera system: A comparison with the flame network and seismological data. J. Volcanol. Geotherm. Res. 300, 95–102. doi:10.1016/j.jvolgeores.2015.02.006
Campion, R., Delgado Granados, H., Mori, T., Delgado-Granados, H., and Mori, T. (2015). Image-based correction of the light dilution effect for SO2 camera measurements. J. Volcanol. Geotherm. Res. 300, 48–57. doi:10.1016/j.jvolgeores.2015.01.004
D’Aleo, R., Bitetto, M., Delle Donne, D., Tamburello, G., Battaglia, A., Coltelli, M., et al. (2016). Spatially resolved SO 2 flux emissions from Mt Etna. Geophys. Res. Lett. 43, 7511–7519. doi:10.1002/2016GL069938
De Moor, J. M., Aiuppa, A., Avard, G., Wehrmann, H., Dunbar, N., Muller, C., et al. (2016). Turmoil at Turrialba Volcano (Costa Rica): Degassing and eruptive processes inferred from high-frequency gas monitoring. J. Geophys. Res. Solid Earth 121, 5761–5775. doi:10.1002/2016JB013150
de Moor, J. M., Kern, C., Avard, G., Muller, C., Aiuppa, A., Saballos, A., et al. (2017). A new sulfur and carbon degassing inventory for the southern central American volcanic arc: The importance of accurate time-series data sets and possible tectonic processes responsible for temporal variations in arc-scale volatile emissions. Geochem. Geophys. Geosystems 18, 4437–4468. doi:10.1002/2017GC007141
Delle Donne, D., Tamburello, G., Aiuppa, A., Bitetto, M., Lacanna, G., D’Aleo, R., et al. (2017). Exploring the explosive-effusive transition using permanent ultraviolet cameras. J. Geophys. Res. Solid Earth 122, 4377–4394. doi:10.1002/2017JB014027
Delle Donne, D., Aiuppa, A., Bitetto, M., D’Aleo, R., Coltelli, M., Coppola, D., et al. (2019). Changes in SO2 Flux regime at mt. etna captured by automatically processed ultraviolet camera data. Remote Sens. 11, 1201. doi:10.3390/rs11101201
Delle Donne, D., Coco, E. L., Bitetto, M., Paolo, F., La Monica, F. P., Lacanna, G., et al. (2022). Spatio-temporal changes in degassing behavior at Stromboli volcano derived from two co-exposed SO2 camera stations. Front. Earth Sci. 10, 1–17. doi:10.3389/feart.2022.972071
Elias, T., Kern, C., Horton, K. A., Sutton, A. J., and Garbeil, H. (2018). Measuring SO2 emission rates at Kīlauea volcano, Hawaii, using an array of upward-looking UV spectrometers, 2014–2017. Front. Earth Sci. 6, 1–20. doi:10.3389/feart.2018.00214
Esse, B., Burton, M. R., Varnam, M., Kazahaya, R., and Salerno, G. (2020). iFit: A simple method for measuring volcanic SO2 without a measured Fraunhofer reference spectrum. J. Volcanol. Geotherm. Res. 402, 107000. doi:10.1016/j.jvolgeores.2020.107000
Galle, B., Johansson, M., Rivera, C., Zhang, Y., Kihlman, M., Kern, C., et al. (2010). Network for observation of volcanic and atmospheric change (NOVAC)—a global network for volcanic gas monitoring: Network layout and instrument description. J. Geophys. Res. 115, D05304. doi:10.1029/2009JD011823
Gliß, J., Stebel, K., Kylling, A., Dinger, A., Sihler, H., and Sudbø, A. (2017). Pyplis–A Python software toolbox for the analysis of SO2 camera images for emission rate retrievals from point sources. Geosciences 7, 134. doi:10.3390/geosciences7040134
Gliß, J., Stebel, K., Kylling, A., and Sudbø, A. (2018). Improved optical flow velocity analysis in SO&lt;sub&gt;2&lt;/sub&gt; camera images of volcanic plumes – implications for emission-rate retrievals investigated at Mt Etna, Italy and Guallatiri, Chile. Atmos. Meas. Tech. 11, 781–801. doi:10.5194/amt-11-781-2018
Kern, C., Deutschmann, T., Vogel, L., Wöhrbach, M., Wagner, T., and Platt, U. (2010a). Radiative transfer corrections for accurate spectroscopic measurements of volcanic gas emissions. Bull. Volcanol. 72, 233–247. doi:10.1007/s00445-009-0313-7
Kern, C., Kick, F., Vogel, L., Platt, U., and Wohrbach, M. (2010b). Theoretical description of functionality, applications, and limitations of SO&lt;sub&gt;2&lt;/sub&gt; cameras for the remote sensing of volcanic plumes. Atmos. Meas. Tech. 3, 733–749. doi:10.5194/amt-3-733-2010
Kern, C., Werner, C., Elias, T., Sutton, a. J., and Lübcke, P. (2013). Applying UV cameras for SO2 detection to distant or optically thick volcanic plumes. J. Volcanol. Geotherm. Res. 262, 80–89. doi:10.1016/j.jvolgeores.2013.06.009
Kern, C., Sutton, J., Elias, T., Lee, L., Kamibayashi, K., Antolik, L., et al. (2015). An automated SO2 camera system for continuous, real-time monitoring of gas emissions from Kilauea Volcano’s summit Overlook Crater. J. Volcanol. Geotherm. Res. 300, 81–94. doi:10.1016/j.jvolgeores.2014.12.004
Kern, C., Lerner, A. H., Elias, T., Nadeau, P. A., Holland, L., Kelly, P. J., et al. (2020). Quantifying gas emissions associated with the 2018 rift eruption of Kīlauea Volcano using ground-based DOAS measurements. Bull. Volcanol. 82, 55. in press. doi:10.1007/s00445-020-01390-8
Kern, C., Aiuppa, A., and Moor, J. M. D. (2022). A golden era for volcanic gas geochemistry? Bull. Volcanol. 84, 43. doi:10.1007/s00445-022-01556-6
Klein, A., Lübcke, P., Bobrowski, N., Kuhn, J., and Platt, U. (2017). Plume propagation direction determination with SO&lt;sub&gt;2&lt;/sub&gt; cameras. Atmos. Meas. Tech. 10, 979–987. doi:10.5194/amt-10-979-2017
Kraus, S. G. (2006). Doasis: A framework design for DOAS. PhD thesis. Heidelberg: University of Heidelberg, 143. ISBN 978-3-8322-5452-0.
Kunrat, S., Kern, C., Alfianti, H., and Lerner, A. H. (2022). Forecasting explosions at Sinabung Volcano, Indonesia, based on SO2 emission rates. Front. Earth Sci. 10, 976928. doi:10.3389/feart.2022.976928
Layana, S. (2022). Procesos que controlan el balance térmico-magmático en volcanes activos de la zona Volcánica Central (ZVC). PhD thesis. Antofagasta: Universidad Católica del Norte.
Lübcke, P., Bobrowski, N., Illing, S., Kern, C., Alvarez Nieves, J. M., Vogel, L., et al. (2013). On the absolute calibration of SO&lt;sub&gt;2&lt;/sub&gt; cameras. Atmos. Meas. Tech. 6, 677–696. doi:10.5194/amt-6-677-2013
Mather, T. A., Tsanev, V. I., Pyle, D. M., Mcgonigle, A. J. S., Oppenheimer, C., and Allen, A. G. (2004). Characterization and evolution of tropospheric plumes from Lascar and Villarrica volcanoes, Chile. J. Geophys. Res. 109, D21303. doi:10.1029/2004JD004934
Mori, T., and Burton, M. R. (2006). The SO2 camera: A simple, fast and cheap method for ground-based imaging of SO2 in volcanic plumes. Geophys Res. Lett. 33, L24804. doi:10.1029/2006GL027916
Mori, T., Mori, T., Kazahaya, K., Ohwada, M., Hirabayashi, J., and Yoshikawa, S. (2006). Effect of UV scattering on SO2 emission rate measurements. Geophys. Res. Lett. 33, L17315–L17317. doi:10.1029/2006GL026285
Mosbrucker, A. R., Zoeller, M. H., and Ramsey, D. W. (2020). Digital elevation model of Kīlauea Volcano, Hawaiʻi, based on July 2019 airborne lidar surveys. U.S. Geological Survey data release. doi:10.5066/P9F1ZU8O
Nadeau, P. A., Palma, J. L., and Waite, G. P. (2011). Linking volcanic tremor, degassing, and eruption dynamics via SO2 imaging. Geophys. Res. Lett. 38, L01304. doi:10.1029/2010GL045820
Nadeau, P. A. (2011). Ultraviolet digital imaging of volcanic plumes: Implementation and application to magmatic processes at basaltic volcanoes. PhD thesis. Houghton, Michigan: Michigan Technological University.
Neal, C. A., Brantley, S. R., Antolik, L., Babb, J. L., Burgess, M., Calles, K., et al. (2019). The 2018 rift eruption and summit collapse of Kilauea Volcano. Science 363, 367–374. doi:10.1126/science.aav7046
Osorio, M., Casaballe, N., Belsterli, G., Barreto, M., Gómez, Á., Ferrari, J., et al. (2017). Plume segmentation from UV camera images for SO2 emission rate quantification on cloud days. Remote Sens. 9, 517. doi:10.3390/rs9060517
Pering, T. D., Ilanko, T., and Liu, E. J. (2019). Periodicity in volcanic gas plumes: A review and analysis. Geosci 9, 394. doi:10.3390/geosciences9090394
Peters, N., Hoffmann, A., Barnie, T., Herzog, M., and Oppenheimer, C. (2015). Use of motion estimation algorithms for improved flux measurements using SO2 cameras. J. Volcanol. Geotherm. Res. 300, 58–69. doi:10.1016/j.jvolgeores.2014.08.031
Phillipson, G., Sobradelo, R., and Gottsmann, J. (2013). Global volcanic unrest in the 21st century: An analysis of the first decade. J. Volcanol. Geotherm. Res. 264, 183–196. doi:10.1016/j.jvolgeores.2013.08.004
Platt, U., and Stutz, J. (2008). in Differential optical absorption spectroscopy (DOAS). Editor M. W. Sigrist (Berlin, Heidelberg: Springer Berlin Heidelberg). doi:10.1007/978-3-540-75776-4
Tamburello, G., Hansteen, T. H., Bredemeyer, S., Aiuppa, A., and Tassi, F. (2014). Gas emissions from five volcanoes in northern Chile and implications for the volatiles budget of the Central Volcanic Zone. Geophys. Res. Lett. 41, 4961–4969. doi:10.1002/2014GL060653
Varnam, M., Burton, M. R., Esse, B., Kazahaya, R., Salerno, G., Caltabiano, T., et al. (2020). Quantifying light dilution in ultraviolet spectroscopic measurements of volcanic SO2 using dual-band modeling. Front. Earth Sci. 8, 1–11. doi:10.3389/feart.2020.528753
Varnam, M., Burton, M. R., Esse, B., Salerno, G., Kazahaya, R., and Ibarra, M. (2021). Two independent light dilution corrections for the SO2 camera retrieve comparable emission rates at masaya. Remote Sens. 13, 935. doi:10.3390/rs13050935
Global Volcanism Program (1993). “Report on lascar (Chile),”. Bulletin of the global volcanism network. Editor E. Venzke (Smithsonian Institution), 18, 4. doi:10.5479/si.GVP.BGVN199304-355100
Wilkes, T. C., McGonigle, A. J. S., Pering, T. D., Taggart, A., White, B., Bryant, R., et al. (2016). Ultraviolet imaging with low cost smartphone sensors: Development and application of a raspberry pi-based UV camera. Sensors 16, 1649. doi:10.3390/s16101649
Keywords: volcanology, sulphur dioxide, ultraviolet camera, remote sensing, raspberry pi
Citation: Wilkes TC, Pering TD, Aguilera F, Layana S, Nadeau P, Kern C, McGonigle AJS, Aguilera M and Zhu C (2023) A new permanent, low-cost, low-power SO2 camera for continuous measurement of volcanic emissions. Front. Earth Sci. 11:1088992. doi: 10.3389/feart.2023.1088992
Received: 03 November 2022; Accepted: 28 February 2023;
Published: 04 April 2023.
Edited by:
Georg Rümpker, Goethe University Frankfurt, GermanyReviewed by:
Philipson Bani, UMR6524 Laboratoire Magmas et Volcans (LMV), FranceCopyright © 2023 Wilkes, Pering, Aguilera, Layana, Nadeau, Kern, McGonigle, Aguilera and Zhu. This is an open-access article distributed under the terms of the Creative Commons Attribution License (CC BY). The use, distribution or reproduction in other forums is permitted, provided the original author(s) and the copyright owner(s) are credited and that the original publication in this journal is cited, in accordance with accepted academic practice. No use, distribution or reproduction is permitted which does not comply with these terms.
*Correspondence: Thomas Charles Wilkes, dGN3aWxrZXMxQHNoZWZmaWVsZC5hYy51aw==
Disclaimer: All claims expressed in this article are solely those of the authors and do not necessarily represent those of their affiliated organizations, or those of the publisher, the editors and the reviewers. Any product that may be evaluated in this article or claim that may be made by its manufacturer is not guaranteed or endorsed by the publisher.
Research integrity at Frontiers
Learn more about the work of our research integrity team to safeguard the quality of each article we publish.