- 1Key Laboratory of Flood and Drought Disaster Defense, The Ministry of Water Resources, Nanjing, China
- 2State Key Laboratory of Simulation and Regulation of Water Cycle in River Basin, China Institute of Water Resources and Hydropower Research, Beijing, China
- 3Yinshanbeilu Grassland Eco-Hydrology National Observation and Research Station, China Institute of Water Resources and Hydropower Research, Beijing, China
- 4College of Geomatics, Xi’an University of Science and Technology, Xi’an, China
- 5College of Hydrology and Water Resources, Hohai University, Nanjing, China
Extreme events normally have negative effects on crop growth. Many studies have reported findings on drought and flood events, while only sparse studies have focused on new types of extreme events, such as drought-flood abrupt alternation (DFAA). We attempted to gain an insight on the effects of DFAA over two-year field experiment on biomass, grain yield and quality, then simulated the yield loss to DFAA in history and future in summer maize planting area in the Northern Anhui Plain. Results show that DFAA significantly reduced root biomass and shoot biomass by 77.1% and 60.1% compared with that in the control systems. The negative effect lasted until mature stage. The grain yield loss was 14.1%–38.4% in different DFAA treatments. The numerical simulation reveals that the average annual yield loss due to DFAA has been increasing in the Northern Anhui Plain, with 21.19%–30.98% during 1964–2017, 14.10%–33.40% during 2020–2050. The spatial distribution of yield loss changed as well. This study increases our knowledge of the effects of DFAA on crop production and highlights the need to consider the targeted countermeasures.
1 Introduction
Crop production relates to welfare of global people (Piao et al., 2010; Zhao et al., 2017a). It has become a major issue to explore crop adaptation to changing environment, which guarantees food safety and socio-economic development (Zhao et al., 2017b; Magno Massuia de Almeida et al., 2022). In recent years, extreme events such as droughts and floods have occurred frequently and widely under intensive climate change (Han et al., 2021). The affected area of global extreme droughts since the 1970s has increased by about 1.5 times compared with that before the 1970s (Dai et al., 2004). There have occurred more than 2800 floods in the world in the past 100 years (Yang et al., 2013). According to the International Panel on Climate Change (IPCC) Working Group I Sixth Assessment Report, compound extreme events are expected to be more frequent and intensive (Zscheischler et al., 2018; Zscheischler et al., 2020; IPCC, 2021). Therefore, it is of great significance to study the crop growth characteristics under extreme climate.
Root system is an important bond between soil and aboveground crops, which was sensitive to water stress (Kano-Nakata et al., 2013; Suralta et al., 2018). Any changes in root would have a great impact on aboveground growth and eventually on yield. Numerous studies have investigated crop biomass and yield under soil moisture fluctuation, mainly under drought (Eziz et al., 2017; Griffiths et al., 2020; Liu et al., 2020; Jaman et al., 2022), flood (Glaz and Lingle, 2012; Chen et al., 2017; Meng et al., 2022), drying-rewetting (Wan et al., 2016; Farooq et al., 2020), etc. A certain degree of drought may stimulate root growth and increase the root-shoot ratio to better absorb water and nutrients, while severe drought would inhibit biomass accumulation (Uga et al., 2013; Kameoka et al., 2016; Chen et al., 2022). When subjected to flood, the root growth was restricted due to anaerobic environment while the shoot growth may be accelerated (Nishiuchi et al., 2012; Sauter, 2013). Periodic drying-rewetting cycles could have overcompensation effects on crop growth (Wang et al., 2022). However, only few studies have been conducted on drought-flood abrupt alternation (DFAA), which is a phenomenon of persistent drought followed by sudden heavy precipitation at a certain level (Wu et al., 2006). While the drying-rewetting does not require the water condition to reach drought and sudden flood at certain levels. In this study, DFAA refers to drought turning to flood. Whether drought and ‘abrupt flood’ exerts an additive effect on crop growth should be confirmed.
Maize (Zea mays L.), one of the most important crops, is the world’s second-largest growing area after wheat crop, its yield occupies 35% in all grain crops (Chung et al., 2018; Wang et al., 2021). Maize production is essential to global agriculture. Certain limits exist for its growth, such as, water stress (Liu et al., 2021; Li et al., 2022). The grain quality is affected by extreme events. The drought promoted crude protein formation (Ma et al., 2006). The crude starch and crude fat contents were less influenced by the drought (Shumway et al., 1992). The flood had negative impacts on crude protein content as water dilutes the nutrients (Singh et al., 2008). Hitherto, far less studies have been reported on maize growth under DFAA even less conducting infield, also the quantitative estimation in regional scale needs to be further studied. Hence, we conducted research in a site located in the Northern Anhui Plain, where is one of the largest grain bases in China, with DFAA events occurring every 3–4 years (Huang, 2015).
In this study, eight combinations of different DFAA levels and in different growth periods were applied to disentangle the impacts of DFAA on biomass and yield in field. In addition, the yield changes in summer maize planting area in the Northern Anhui Plain were estimated based on the obtained experimental data. We assumed that: i) the DFAA events reduced both the underground and aboveground biomass; ii) the DFAA events increased yield loss; and iii) the yield loss due to DFAA would increase in summer maize planting area in the Northern Anhui Plain.
2 Materials and methods
2.1 Experimental site
The field trial was conducted at Wudaogou Experimentation Research Station (33°09′N, 117°21′E), Bengbu, China during the growing season of summer maize in 2018 and 2019 (Supplementary Figure S1). The study site is located in the Northern Anhui Plain, where is a transitional zone between the northern subtropical climate and the warm temperate climate. The mean annual precipitation was 890 mm during 1963–2017, of over 60% in summer, which increases the risk for crops to suffer DFAA stresses.
The 2-year field study was conducted in 15 experimental plots (length × width = 5.5× 3.7 m for each plot). Of them, 12 experimental plots were sheltered under a ventilated shed to block the entry of external precipitation. An artificial rainfall device was set to simulate “abrupt flood” conditions. Surrounding each experimental plot, aluminum plastic composite panels with a depth of 1.2 m (0.2 m standing above ground level) were used as baffles to prevent external water and groundwater from other experimental plots. Each experimental plot had only one outlet (length × height = 10 × 10 cm), connected with a water collecting device made from acrylic. Pipes were buried for soil water content measurement (AIM-WiFi soil multi-parameters monitoring system). Experimental plots design is shown in Supplementary Figure S2 (Bi et al., 2022). The soil in the plots was undisturbed field soil of dark hydromorphic clay loam, which is the soil type with the largest area in the study area, accounting for 52.2% (Supplementary Figure S1). The dark-hydromorphic clay loam has a soil bulk density of about 1.4 g cm−3 and the pH of 6.7–7.9. It has the characteristics of poor structure, heavy texture, and low organic matter content, which tend to exacerbate the effects of drought and waterlogging.
2.2 Experimental design
As described in our previous studies (Bi et al., 2022), we proposed determination method of DFAA on considering both the meteorological and agricultural indicators at daily scale (Supplementary Section S1). Two types of treatments were arranged for this experiment: i) control treatment (CS, under the natural climatic conditions); and ii) four DFAA treatments, i.e., light drought-light flood (LL), light drought-moderate flood (LM), moderate drought-light flood (ML), and moderate drought-moderate flood (MM). The LL, LM, and ML treatments belong to light DFAA, which accounts for over 85% in all DFAA events in the Huaibei Plain. The four DFAA treatments are the most occurring types in the study area, accounting for over 90% in all DFAA events, which can represent almost all DFAA events. Each treatment had three replicates. Supplementary Table S2 plots the specific time points for each treatment. In this study, the drought level was evaluated based on continuous rainless days and soil moisture proposed by the Standard of Classification for Drought Severity (SL424–2008) and the Classification of Meteorological Drought (GB/T 20481-2006). The soil moisture was measured in triplicate in each experimental plot at 6:30 a.m. to 7:30 a.m. every day, with an AIM-WiFi soil multiparameter monitoring system (Beijing Aozuo Ecology Instrumentation Ltd., Beijing, China). The flood level is determined by the amount of effective precipitation within 5 days after drought. The onset of precipitation after drought within 24 h. The detailed information can be found in Supplementary Table S1.
All the tests were conducted in the growing season of summer maize (Deng-hai 618) from 25 June to 9 October, 2018, and 13 June to 27 September 2019. We conducted these four DFAA conditions during two summer maize growing stages, i.e., the seeding-jointing stage (sj) and the tasseling-grain filling stage (tg), which are the two most critical stages for crop growth and field production. The plant density of each experimental plot was 7.5 plant m−2. Before planting maize, we fertilized each experimental plot with 75 g m−2 of compound fertilizer with potassium sulfate and 30 g m−2 of urea. No more fertilizer was added during the experiment. The initial conditions (soil water content, P addition, and so on) were kept the same for all the experimental plots. Biomass, yield, and yield component were measured at specific time point. Each index measurement had three replicates.
2.3 Sampling and measurements
2.3.1 Biomass measurement
To measure the biomass characteristics, the above-ground biomass (shoot) and belowground biomass (root) were separately measured and calculated for each treatment. To measure the above-ground biomass, we harvested shoot for each plant. To collect the belowground biomass, we carefully excavated each plant following the direction of the root system. We washed the roots to remove any soil material. We did not distinguish between living roots and dead roots. All roots were treated as below-ground biomass. All biomass were oven-dried at 80°C until constant weight using an analytical balance. The biomass samples were collected at three specific time points, i.e., at the end of drought, 7 days after DFAA, and at harvest.
2.3.2 Gran yield and grain quality measurements
To measure the biomass characteristics, the above-ground biomass (shoot) and belowground biomass (root) were separately measured and calculated for each treatment. To measure the above-ground biomass, we harvested shoot for each plant. To collect the belowground biomass, we carefully excavated each plant following the direction of the root system. We washed the roots to remove any soil material. We did not distinguish between living roots and dead roots. All roots were treated as below-ground biomass. All biomass were oven-dried at 80°C until constant weight using an analytical balance. The biomass samples were collected at three specific time points, i.e., at the end of drought, 7 days after DFAA, and at harvest.
Ears were separated from the shoots and dried at 80°C until constant weight using an analytical balance. The number of ear rows and columns, ear length, total number of grains, and 100-seed weight were counted. The yield can be calculated as follows.
Where, GY is the yield of each experimental plot; EPN is the effective plant number of summer maize in each experimental plot; NG is the average total grains number in one plant; SW100 is the average 100-seed weight; Aplot is the surface area of the experimental plot.
The crude protein, crude starch, crude fiber, and crude fat content in the grains were analyzed. These four parameters were measured according to the methods proposed by Lu. (2000). The crude protein is determined based on the nitrogen concentration. The crude protein content was 6.25 times the nitrogen concentration for maize. The nitrogen concentration was measured by the elemental analyzer Shimadzu TOC-VCPH Organic Carbon Analyzer (Kyoto, Japan). The crude starch was measured by the CaCl2-HOAc extraction-polarimetry method. The crude fiber was measured by Acid Detergent Method provided by Van Soest. (1973), Van Soest. (1963). The crude fat was measured by the residual method.
2.4 Numerical simulation
To measure the biomass characteristics, the above-ground biomass (shoot) and belowground biomass (root) were separately measured and calculated for each treatment. To measure the above-ground biomass, we harvested shoot for each plant. To collect the belowground biomass, we carefully excavated each plant following the direction of the root system. We washed the roots to remove any soil material. We did not distinguish between living roots and dead roots. All roots were treated as below-ground biomass. All biomass were oven-dried at 80°C until constant weight using an analytical balance. The biomass samples were collected at three specific time points, i.e., at the end of drought, 7 days after DFAA, and at harvest.
To evaluate the impacts of DFAA events on crop yield, numerical simulation was applied to extended field experiment results to regional scale in the Northern Anhui Plain, which is one of the most important agricultural regions in China (Supplementary Figure S1). In total two types of scenarios were set, i.e., history scenarios, and future scenarios. The description for these scenarios were reported in our previous study (Bi et al., 2022), also can be found in Supplementary Section S2. The analysis was only based on the climatic change, not considering the distribution of human activities (additional fertilizer addition, control measures to DFAA, etc.), and the variations of cropping systems, irrigation regime, summer maize varieties, etc. The scenarios we set may not be highly consistent with actual situations, but can reflect the impacts of a single variable.
The average annual yield loss due to DFAA in history and future can be calculated as follows:
Where,
The average annual yield loss in hypothetical natural condition in history and in the future can be calculated as follows:
Where,
2.5 Statistical analysis
Data were shown in mean ± standard deviation. Variables were analyzed with one-way ANOVA. The significance of linear correlations between parameters was expressed as Pearson’s product moment correlation coefficient. A statistical difference was considered significant when p < 0.05. The figures were drawn in Origin version 9.0 (OriginLab Inc., Hampton, MA, United States), and ArcGIS10.2.2 software platform (ESRI, Redlands, CA, United states).
3 Results
3.1 Biomass changes under DFAA in the field experiment
In comparison with control treatment, DFAA significantly reduced the root dry weight (RDW) and shoot dry weight (SDW) at different time points during DFAA process, and the effects even continued until mature stage (Figure 1).
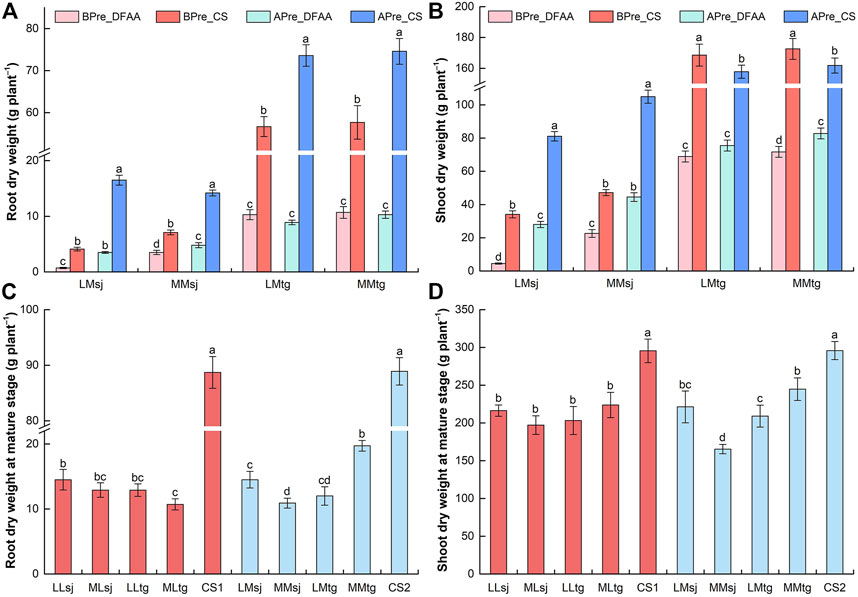
FIGURE 1. Changes of root dry weight, and shoot dry weight in different treatments. (A,C) changes of root dry weight during DFAA process, at the mature stage. (B,D) changes of shoot dry weight during drought-flood abrupt alternation (DFAA) process, at the mature stage. Error bars represent standard deviations (n = 3), different letters in the same row indicated significant difference among the treatments at 0.05. Note: LLsj, light drought-light flood in the seeding-jointing stage (SJS); MLsj, moderate drought-light flood in the SJS; LLtg, light drought-light flood in the tasseling-grain filling stage (TGS); MLtg, moderate drought-light flood in the TGS; LMsj, light drought-moderate flood in the SJS; MMsj, moderate drought-moderate flood in the SJS; LMtg, light drought-moderate flood in the TGS; MMtg, moderate drought-moderate flood in the TGS; CS1 and CS2, control system operated under natural climatic conditions; BPre_DFAA, after drought period of DFAA; APre_DFAA, after flooding period of DFAA; BPre_CS, APre_CS, changes in CS treatment at the same time point of BPre_DFAA, APre_DFAA.
During DFAA process, after drought, the RDW for LMsj, MMsj, LMtg, and MMtg treatments were 83.3%, 50.7%, 81.8%, and 81.5% lower than the control treatment, respectively (Figure 1A). The SDW for LMsj, MMsj, LMtg, and MMtg treatments were 86.8%, 52.1%, 59.1%, and 58.5% lower than the control treatment (Figure 1B). After flooding period of DFAA, compared with control treatment, the RDW and SDW for LMsj, MMsj, LMtg, and MMtg treatments were lower by 78.8% and 65.4%, 66.2%, and 57.6%, 88.0%, and 52.2%, 86.2%, and 48.8%, respectively (Figures 1A, B).
At the mature stage, the RDW for LLsj, MLsj, LLtg, MLtg, LMsj, MMsj, LMtg, and MMtg treatments were 83.7%, 85.5%, 85.5%, 87.9%, 83.7%, 87.7%, 86.5%, and 77.8% lower than CS treatment, respectively (Figure 1C). Compared with CS treatment, the SDW for LLsj, MLsj, LLtg, MLtg, LMsj, MMsj, LMtg, and MMtg treatments were lower by 26.8%, 33.3%, 31.2%, 24.3%, 25.2%, 44.1%, 29.3%, and 17.2% lower than CS treatment (Figure 1D).
Regression analysis showed a significant correlation between SDW and RDW in all treatments. For DFAA treatments, during DFAA process, r = 0.979, p < 0.05 (Figure 2A); at the mature stage, r = 0.606, p < 0.05 (Figure 2B). For the CS treatments, r = 0.853, p < 0.05 (Figure 2C). In addition, the root-shoot ratio (the ratio of RDW and SDW) under different treatments were calculated (Figure3A). The drought period of DFAA reduced root-shoot ratio by−0.03 (−26.3%), 0.004 (3%), 0.19 (55.5%), and 0.19 (55.4%) for LMsj, MMsj, LMtg, and MMtg treatments, respectively. After flooding period of DFAA, the root-shoot ratio in the LMsj, MMsj, LMtg, and MMtg treatments was reduced by 0.08 (38.6%), 0.03 (20.3%), 0.35 (75.0%), and 0.34 (73.0%) than that in the control treatments. Compared with the CS treatment, the root-shoot ratio at the mature stage reduced by 0.23 (77.7%), 0.23 (78.2%), 0.24 (78.9%), 0.25 (84.1%), 0.24 (78.2%), 0.23 (78.1%), 0.24 (80.9%), and 0.22 (73.2%) for LLsj, MLsj, LLtg, MLtg, LMsj, MMsj, LMtg, and MMtg treatments, respectively.
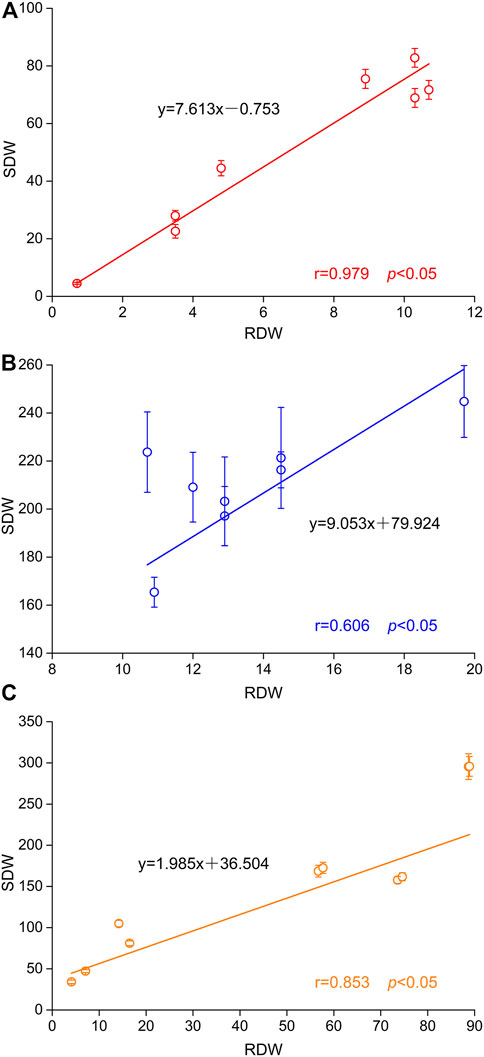
FIGURE 2. Relationships between root dry weight (RDW) and shoot dry weight (SDW). (A) during drought-flood abrupt alternation (DFAA) process in the DFAA treatments; (B) at the mature stage in the DFAA treatments; (C) in the control treatments. Error bars represent standard deviations (n = 3).
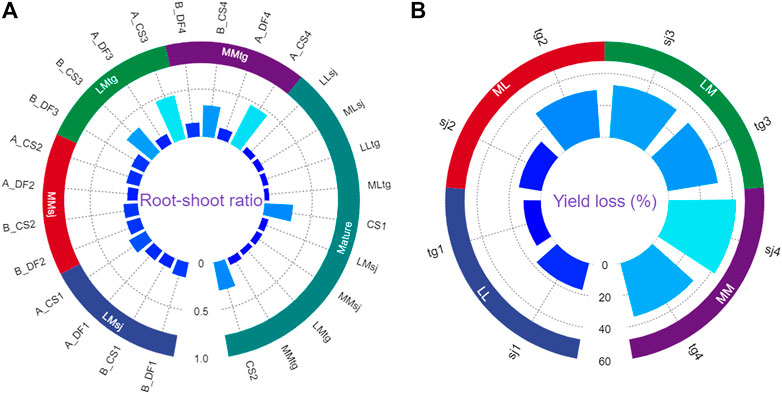
FIGURE 3. Root-shoot ratio in different treatment (A); grain yield loss of summer maize (B). Note: LLsj, MLsj, LLtg, MLtg, CS1, LMsj, MMsj, LMtg, MMtg, and CS2, same to the above explanations. B_DF, after drought period of drought-flood abrupt alternation (DFAA); A_DF, after flooding period of DFAA; B_CS, A_CS, changes in CS treatment at the same time point of B_DF, A_DF.
3.2 Grain changes under DFAA in the field experiment
3.2.1 Grain yield and yield components
The DFAA basically significantly decreased the grain yield of summer maize in 2018 and 2019 (p < 0.05), although their differences varied among treatments and years. In comparison with control treatment, the grain yield decreased by 1287.5 kg hm−1, 1084.6 kg hm−1, 812.7 kg hm−1, 2224.6 kg hm−1, 3888.9 kg hm−1, 4998.0 kg hm−1, 3759.0 kg hm−1, and 4089.5 kg hm−1, respectively (Figure 4A). Thus, the yield losses were 17.3%, 14.5%, 10.9%, 29.8%, 32.8%, 42.2%, 31.8%, 34.5% in the LLsj, MLsj, LLtg, MLtg, LMsj, MMsj, LMtg, and MMtg treatments (Figure 3B).
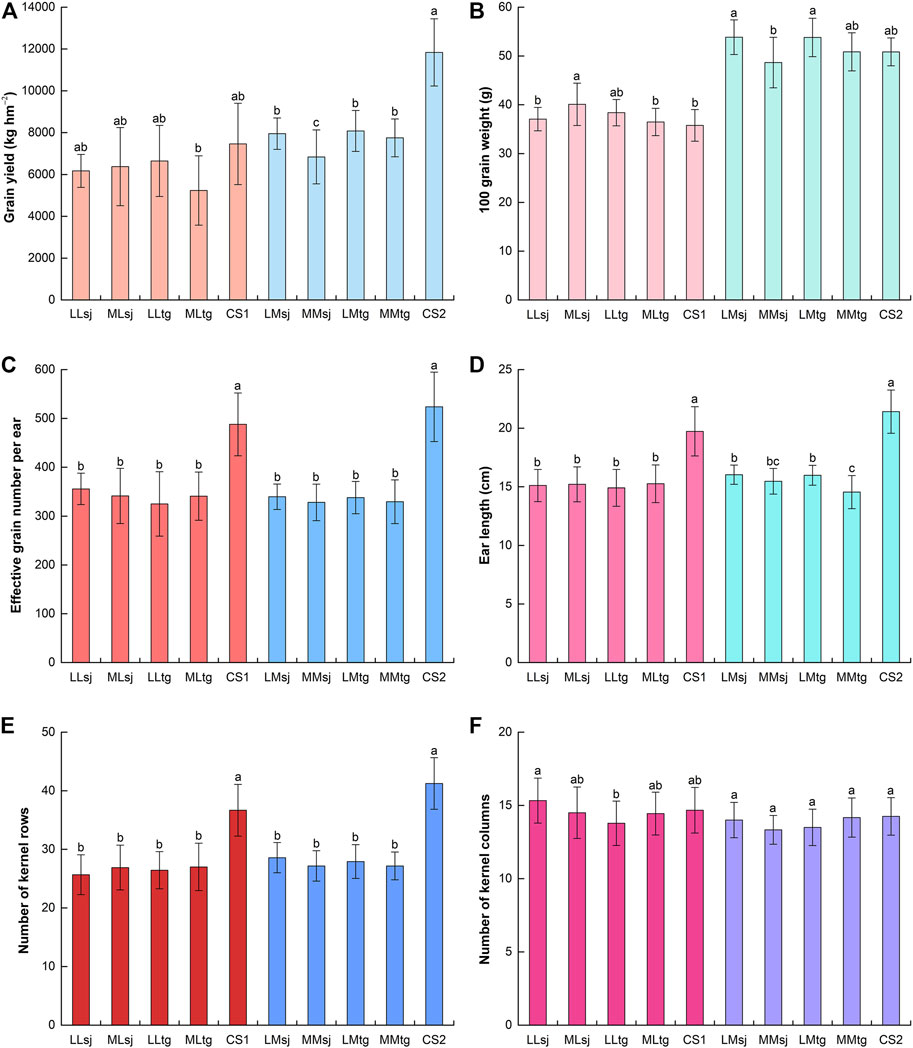
FIGURE 4. Grain yield and yield components in different treatments. (A) grain yield; (B) 100 grain weight; (C) effective grain number per ear; (D) ear length; (E) number of kernel rows; (F) number of kernel columns. Error bars represent standard deviations (n = 3), different letters in the same row indicated significant difference among the treatments at 0.05. Note: LLsj, MLsj, LLtg, MLtg, CS1, LMsj, MMsj, LMtg, MMtg, CS2, same to the above explanations.
There are certain yield components contributed to the grain yield loss due to DFAA. The 100 grain weight for DFAA treatments were heavier by−4.3%–12.1% than in the CS treatment (Figure 4B). The effective grain number per ear in the LLsj, MLsj, LLtg, MLtg, LMsj, MMsj, LMtg, and MMtg treatments were lower by 27.1%, 30.0%, 33.4%, 30.1%, 35.1%, 37.4%, 35.5%, and 37.1% than in the CS treatments (Figure 4C). The DFAA also significantly decreased the ear length (p < 0.05), by 26.4%–34.1% (Figure 4D). Compared with control treatment, the number of kernel rows and columns for LLsj, MLsj, LLtg, MLtg, LMsj, MMsj, LMtg, and MMtg treatments were lower by 30.0% and−4.5%, 26.7%, and 1.2%, 27.9%, and 6.1%, 26.4%, and 1.6%, 30.7%, and 1.8%, 34.1%, and 6.5%, 32.3%, and 5.3%, 34.1%, and 0.6%, respectively (Figures 4E, F).
3.2.2 Grain quality
The DFAA basically had no significant effects on grain quality (p > 0.05). Compared with CS treatment, the crude protein contents were lower by 1.5%,−6.1%, 6.5%,−0.1%, 19.3%, 0, 6.4%, and 2.6% in the LLsj, MLsj, LLtg, MLtg, LMsj, MMsj, LMtg, and MMtg treatments, respectively (Figure 5A). For the crude fat, the contents in the LLsj, MLsj, LLtg, MLtg, LMsj, MMsj, LMtg, and MMtg treatments were 7.4%, 3.8%,−6.8%, 0.6%,−5.7%, 0,−0.02%, and−11.0% lower than the CS treatment (Figure 5B). The crude starch contents in the LLsj, MLsj, LLtg, MLtg, LMsj, MMsj, LMtg, and MMtg treatments decreased by−2.0%,−0.4%, 2.3%,−1.8%, 2.8%,−1.9%,−0.4%, and 0.4%, respectively (Figure 5C). For crude fiber, the contents decreased by 7.1%,−3.5%, 4.0%,−8.5%,−13.7%, 0, 1.5%, and−7.1% (Figure 5D).
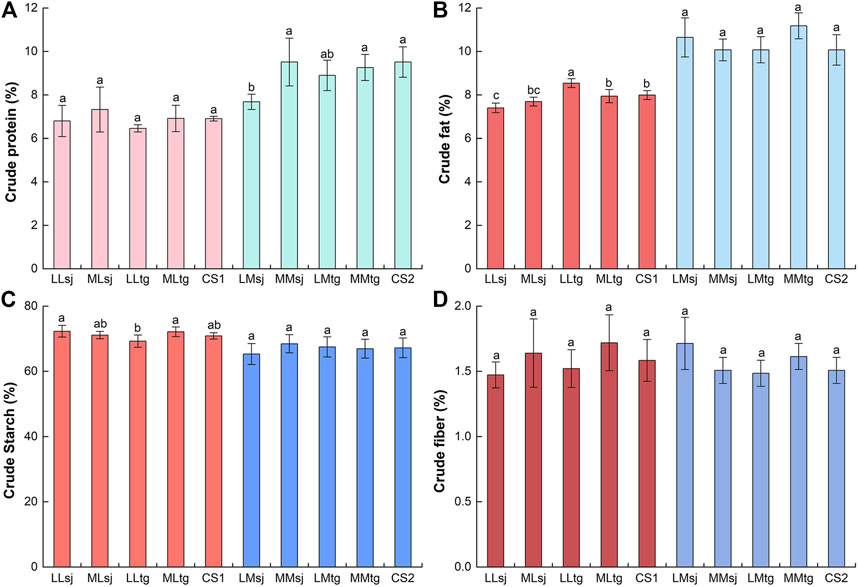
FIGURE 5. Grain quality in different treatments. (A), crude protein; (B), crude fat; (C), crude starch; (D), crude fiber. Error bars represent standard deviations (n = 3), different letters in the same row indicated significant difference among the treatments at 0.05. Note: LLsj, MLsj, LLtg, MLtg, CS1, LMsj, MMsj, LMtg, MMtg, and CS2, same to the above explanations.
3.3 Yield loss in summer maize planting area in the Northern Anhui Plain
According to the grain yield obtained in the field experiments, the yield losses were 14.1%, 22.2%, 32.3%, and 38.4% in the LL, ML, LM, and MM treatments, respectively. As almost no DFAA events in severe level, the yield loss was considered as 38.4% for the DFAA in severe level (same as that in moderate level) in this study.
For the history scenarios, during 1964–2017, the average annual yield loss of summer maize in the years occurring DFAA events was 21.19%–30.98% (Figure 6A). The average annual yield loss was 18.96%–34.35% during 1964–1993 (Figure 6C), and 19.14%–29.08% during 1994–2017 (Figure 6D). The spatial distribution of yield loss changed over time, with lowest center of yield loss shifted from the center (1964–1993) to the northeast (1994–2017).
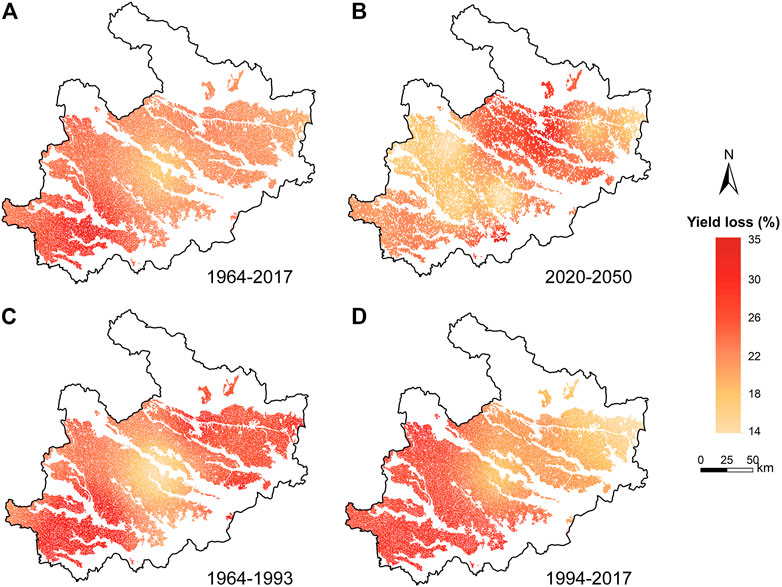
FIGURE 6. The average annual yield loss of summer maize in the years occurring DFAA events. (A), during 1964–2017; (B), during 1964–2017; (C), during 1964–1993; (D), during 1994–2017.
For the future scenario, during 2020–2050, the average annual yield loss of summer maize in the years occurring DFAA events was 14.10%–33.40% (Figure 6B). The highest average annual yield loss was in the central and some northern regions, and decrease to the southwest and north. The spatial distribution would change in the future compared with that in history, especially the highest center of yield loss, shifting from southwest to the central and northern parts.
4 Discussion
4.1 DFAA decreased biomass and increased yield loss in field experiments
The DFAA events significantly reduced both aboveground biomass and underground biomass, with mean decrease of 77.1% and 60.1%, respectively. Moreover, these effects lasted until mature stage, with mean decrease of 84.8% and 28.9% for RDW and SDW. Root systems are the critical organ for crop growth, which are the first to response to environmental changes and normally are the most affected (Norby and Jackson, 2000; Rao et al., 2021). Previous studies reported that crop roots respond via deep rooting and root branching when subjected to drought, which could improve their ability to absorb soil water and nutrient (Hazman and Kabil, 2022). When subjected to flooding, crop increase the leaves to acquire adequate photosynthesis, the excess soil water inhibits root growth (Sauter, 2013). Therefore, the response of biomass to different DFAA treatments had certain connection with drought levels and flood levels in DFAA events. Our study showed that the biomasses were higher in the DFAA with longer drought duration (with same flood level), which verified above findings. Our previous study revealed that the total root tips and total root surface area of root systems relatively increased with longer drought duration (Bi et al., 2020a), which could explain the relative reduction of root biomass in the DFAA treatments with longer drought duration. Also, the leaf area index presented an inapparent changing trend in the drought period of DFAA and a faster increasing trend during flooding under DFAA compared with CS treatment (Bi et al., 2020a), which explains the reduction effects of RDW was more severe than SDW in this study. In addition, the response of biomass was related to different growth periods of summer maize under DFAA. The root biomass reduction was higher when DFAA occurring in the tasseling-grain filling stage than in the seeding-jointing stage, of 14.6%. Several studies point out that the maize is very sensitive during the tasseling, silking, and pollination periods (Nemeskéri et al., 2019), thus the root death would increase more due to DFAA. The root systems are already formed in the TGS, their resilience is limited as well.
According to the correlation analysis of RDW and SDW, and the calculation results of root-shoot ratio, it indicates that a strong interdependent relationship exists between root biomass and shoot biomass. Similar findings have been reported in DFAA experiments on rice (Gao et al., 2019; Huang et al., 2019; Xiong et al., 2019). The probable mechanism could be explained as follows: a higher root biomass can guarantee supplying enough water, nutrients, and plant hormones to shoots, thus ensures high shoot biomass; in turn, a high shoot biomass could supply enough photosynthates to roots to guarantee roots growth (Liu et al., 2021). Compared with the CS treatments, the root-shoot ratio was larger at the drought period of DFAA during the seeding-jointing stage; while it was lower at the flooding period of DFAA during the seeding-jointing stage, and in the DFAA treatments occurring during the tasseling-grain filling stage. This is because of the synergistic effect of root increase under drought and the occurring time point (seeding-jointing stage).
In general, DFAA decreased both biomass and root-shoot ratio. It sheds light on that the effect of drought + “abrupt flood” on biomass especially on the root biomass were not additive but strong interaction, mainly negative compensatory effects. The sudden changing environment may cause a series of new traits due to re-adaptation.
The responses of gain yield to different DFAA treatments had apparent connection with drought and flood levels. The yield loss rate was higher with more severe drought (flood). Both the long-term drought and flood have negative impacts on the maize yield, while the reductions in grain yield caused by “abrupt flood” seemed to be greater than those caused by drought as the yield loss increased as: LL < ML < LM < MM treatments (Figure 5B), which is consistent with other study (Huang et al., 2019). The main causes are due to: i) soil moisture fluctuation. Several studies have shown that soil moisture changes contribute to changes in yield (Niones et al., 2012); ii) root systems. Soil water conditions influence root growth, further affect the transformation of water and nutrients to grain (Gao et al., 2021); and iii) morphological characteristics of ear. The effective grain number per ear, ear length, and number of kernel rows were significantly lower under DFAA than in the CS treatments (p < 0.05). Although the 100 grain weight has no obvious reductions under DFAA or even slight increases in several treatments, the grain yield loss had high correlation with reduced ear shape. The maize yield may decrease by around 18%–30%, 13%–50% and under single drought and single flood (Wang et al., 1999; Liu et al., 2013). The achieved yield was significantly lower than the attainable values by 27.9% under above-optimal water input (Li et al., 2019). Our experiments show that the maize yield decreased by 14%–38% under DFAA, which was between the yield loss of single drought and of single flood, implying that an interactive effect not additive effect existed between preceding drought and subsequent flooding. Other experimental data showed that rice yield loss was 2%–24%, 4%–48%, and 6%–67% for single drought, DFAA, and single flood, respectively (Huang et al., 2019). Thus, similar results were found on other crops, which confirmed our findings.
As we concluded, the potential causes for biomass reduction and yield loss are soil water fluctuations and nutrient transformation. Our previous studies yielded that phosphorus (P) absorbed by summer maize was significantly lower under DFAA (Bi et al., 2020b; Bi et al., 2022). This study analyzed the correlations among different indices, i.e., P absorbed by summer maize, biomass, yield, grain quality (Figure 7). The heatmap plots that the yield and biomass (RDW and SDW) had were highly positively correlated to P absorbed by summer maize, also highly positive correlation was found between yield and biomass (RDW and SDW), with correlation coefficients over 0.8. While the yield had weak correlation with grain quality (with absolute value lower than 0.4) under DFAA. The grain quality also had weak correlation with P absorbed by summer maize. These findings reveal that the DFAA decreased P absorbed by summer maize, further reduced biomass and yield, with no obvious effects on grain quality.
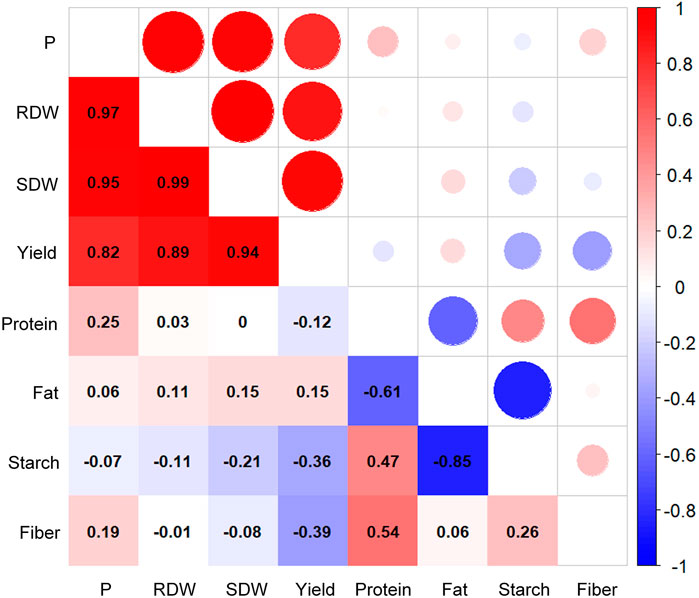
FIGURE 7. Heatmap of correlation between each pair of indices under drought flood abrupt alternation in summer maize farmland systems. The blue discs represent the negative correlation between the pair of indices. The red discs represent the positive correlation between the pair of indices. Note: P, soil absorption by summer maize; RDW, root dry weight; SDW, shoot dry weight; Yield, grain yield; Protein, grain crude protein; Fat, grain crude fat; Starch, grain crude starch; Fiber, grain crude fiber.
4.2 Increasing yield loss risk in the future
The numerical simulations based on field experiment data shed light on an increasing yield loss risk in the future due to DFAA in the summer maize planting area in the Huaibei Plain. The highest center of yield loss shifted as well. The highest average annual yield loss was in the central and some northern regions, and decrease to the southwest and north. The spatial distribution would change in the future compared with that in history, especially the highest center of yield loss, shifting from southwest to the central and northern parts. Our previous study showed that the counts of DFAA events in moderate and severe level increased in 2020–2050 (Bi et al., 2022), which was the main reason to the increasing yield loss as the yield reduction increased with higher DFAA level. The high-value center of yield loss shifted from the southwest (in history scenarios) to the center (in future scenario), which was basically consistent with the frequency of DFAA in severe level and moderate level. Therefore, the frequency of DFAA in high level had significant impacts on the distribution of yield loss. The yield loss increased during 1994–2017 than during 1964–1993, with the DFAA frequency increased as well. The increase of DFAA frequency has been reported (Huang, 2015). The relative reduction caused by drought and flood in maize’s whole growth stage was 22.4% and 28.9% in Northern Anhui Plain, which basically could corroborate our simulation results (Li et al., 2013).
Huaibei Plain is one of the most important crop production bases in China. While for the Northern Anhui Plain, situated in the Huaibei Plain, the crop planting area accounts for 97.7% of the total basin area (Supplementary Figure S1, 38,298.40 km2). The summer maize planting area in this region occupies 26.8% (Bi, 2020) of the crop planting area (10,263.97 km2). Thence, the yield of summer maize contributes critical impacts on local GDP. Several studies reported that the average summer maize yield was 8.25 t hm−1 (Xiong et al., 2021), with mean sale price of 3.0 yuan kg−1. We estimated the economic loss due to DFAA. During 1964–2017, the economic lost in every DFAA occurring year was 5.51–8.06 billion yuan (0.81–1.18 billion dollars). In the next 30 years, the economic lost in every DFAA occurring year was 3.67–8.68 billion yuan (0.54–1.27 billion dollars). The annual economic losses caused by global droughts and floods were about 39 billion US dollars from 1998 to 2017 (Wallemacq et al., 2018). The average annual economic losses reached 2.21 billion yuan in China from 1950–2017 (China Flood Control and Drought Relief Headquarters, 2017). The economic lost caused by DFAA seems to be more serious than that caused by droughts and floods. In a word, the yield loss due to DFAA significantly affects national food security local economic development. Adaptation measures to DFAA events should be timely studied.
5 Conclusion
The underlying objective of this work was to pinpoint, whether the DFAA affected the biomass, yield, and grain quality of summer maize. We conducted 2 years of field experiments, which provide critical parameter thresholds for regional numeric simulations. This study highlighted several significant results: i) DFAA significantly reduced both the underground and aboveground biomass (p < 0.05), with mean decrease of 77.1% and 60.1%, respectively. The negative effect lasted until mature stage, with mean reduction of 84.8% and 28.9% for RDW and SDW. High significant correlation was plotted between RDW and SDW (r = 0.606–0.979, p < 0.05). ii) The grain yield reduced under DFAA, by 14.1%, 22.2%, 32.3%, and 38.4% in the LL, ML, LM, and MM treatments, respectively. DFAA basically had no significant effects on grain quality, which also had weak correlation with grain yield. While the yield has strong positive correlation with biomass. iii) The average annual yield loss due to DFAA in summer maize planting area in the Huaibei Plain was 21.19%–30.98% during 1964–2017, 14.10%–33.40% during 2020–2050. The yield loss increased over time under DFAA, and the high-value center of annual yield loss shifted as well. These results verify our proposed hypothesis. This study reveals the DFAA effects on maize production in field and regional scale, which might guide the evaluation of climate change on maize production at large scales such as national and global scales. The findings could provide an empirical basis for agricultural protection under DFAA. As our field experiments and numerical simulations did not consider the distributions of control measures, further studies could explore the targeted regulation measures to DFAA by relevant field control experiments.
Data availability statement
The original contributions presented in the study are included in the article/Supplementary Material, further inquiries can be directed to the corresponding author.
Author contributions
WB, BW, and DY contributed to the conception and design of the study. HW confirmed and guided the study. WB, LJ, and SY carried out the experiments. WB performed the statistical analysis and wrote the first draft of the manuscript. DZ, CL, and XS guided writing sections of the manuscript. All authors contributed to manuscript revision, read, and approved the submitted version.
Funding
This work is supported by Open Fund of Key Laboratory of Flood and Drought Disaster Defense, the Ministry of Water Resources (No. KYFB202112071044), the National Key R&D Program of China (No. 2022YFC3080300), IWHR Research & Development Support Program (No. WH0145B022021), the Chinese National Natural Science Foundation (No. 52209043, 52022110), and the Open Fund of Yinshanbeilu Grassland Eco-Hydrology National Observation and Research Station (No.YSS202106).
Acknowledgments
Many thanks to Wudaogou Experimentation Research Station for their full support and help during this study.
Conflict of interest
The authors declare that the research was conducted in the absence of any commercial or financial relationships that could be construed as a potential conflict of interest.
Publisher’s note
All claims expressed in this article are solely those of the authors and do not necessarily represent those of their affiliated organizations, or those of the publisher, the editors and the reviewers. Any product that may be evaluated in this article, or claim that may be made by its manufacturer, is not guaranteed or endorsed by the publisher.
Supplementary material
The Supplementary Material for this article can be found online at: https://www.frontiersin.org/articles/10.3389/feart.2023.1086769/full#supplementary-material
References
Bi, W., Wang, M., Weng, B., Yan, D., Yang, Y., and Wang, J. (2020a). Effects of drought-flood abrupt alternation on the growth of summer maize. Atmos. (Basel). 11, 21. doi:10.3390/atmos11010021
Bi, W., Weng, B., Yan, D., Wang, M., Wang, H., Jing, L., et al. (2022). Soil phosphorus loss increases under drought-flood abrupt alternation in summer maize planting area. Agric. Water Manag. 262, 107426. doi:10.1016/j.agwat.2021.107426
Bi, W., Weng, B., Yan, D., Wang, M., Wang, H., Wang, J., et al. (2020b). Effects of drought-flood abrupt alternation on phosphorus in summer maize farmland systems. Geoderma 363, 114147. doi:10.1016/j.geoderma.2019.114147
Bi, W. X. (2020). Effect of drought-flood abrupt alternation on the transport and transformation of phosphorus in typical farmland systems. [dissertation/doctoral thesis]. Nanjing: Hohai University.
Chen, H., Liang, Z., Liu, Y., Liang, Q., and Xie, S. (2017). Integrated remote sensing imagery and two-dimensional hydraulic modeling approach for impact evaluation of flood on crop yields. J. Hydrol. 553, 262–275. doi:10.1016/j.jhydrol.2017.08.001
Chen, Q., Hu, T., Li, X., Song, C. P., Zhu, J. K., Chen, L., et al. (2022). Phosphorylation of SWEET sucrose transporters regulates plant root:shoot ratio under drought. Nat. Plants 8, 68–77. doi:10.1038/s41477-021-01040-7
China Flood Control and Drought Relief Headquarters (2017). Bulletin of flood and drought disaster in China. Beijing: China Water & Power Press.
Chung, I. M., Kim, J. K., Lee, K. J., Park, S. K., Lee, J. H., Son, N. Y., et al. (2018). Geographic authentication of Asian rice (Oryza sativa L.) using multi-elemental and stable isotopic data combined with multivariate analysis. Food Chem. 240, 840–849. doi:10.1016/j.foodchem.2017.08.023
Dai, A., Trenberth, K. E., and Qian, T. (2004). A global dataset of palmer drought severity index for 1870-2002: Relationship with soil moisture and effects of surface warming. J. Hydrometeorol. 5, 1117–1130. doi:10.1175/jhm-386.1
Eziz, A., Yan, Z., Tian, D., Han, W., Tang, Z., and Fang, J. (2017). Drought effect on plant biomass allocation: A meta-analysis. Ecol. Evol. 7, 11002–11010. doi:10.1002/ece3.3630
Farooq, N., Sarwar, G., Abbas, T., Bessely, L., Nadeem, M., Javaid, M., et al. (2020). Effect of drying-rewetting durations in combination with synthetic fertilizers and crop residues on soil fertility and maize production. Pak. J. Bot. 52, 2051–2058. doi:10.30848/pjb2020-6(37)
Gao, J., Lei, M., Yang, L., Wang, P., Tao, H., and Huang, S. (2021). Reduced row spacing improved yield by optimizing root distribution in maize. Eur. J. Agron. 127, 126291. doi:10.1016/j.eja.2021.126291
Gao, Y., Hu, T., Wang, Q., Yuan, H., and Yang, J. (2019). Effect of drought-flood abrupt alternation on rice yield and yield components. Crop Sci. 59, 280–292. doi:10.2135/cropsci2018.05.0319
Glaz, B., and Lingle, S. E. (2012). Flood duration and time of flood onset effects on recently planted sugarcane. Agron. J. 104, 575–583. doi:10.2134/agronj2011.0351
Griffiths, C. A., Reynolds, M. P., and Paul, M. J. (2020). Combining yield potential and drought resilience in a spring wheat diversity panel. Food Energy Secur 9, e241. doi:10.1002/fes3.241
Han, Z., Huang, S., Huang, Q., Wang, L., Leng, G., Liu, Y., et al. (2021). GRACE-based high-resolution propagation threshold from meteorological to groundwater drought. Agr. For. Meteorol. 307, 108476. doi:10.1016/j.agrformet.2021.108476
Hazman, M. Y., and Kabil, F. F. (2022). Maize root responses to drought stress depend on root class and axial position. J. Plant Res. 135, 105–120. doi:10.1007/s10265-021-01348-7
Huang, J., Hu, T., Yasir, M., Gao, Y., Chen, C., Zhu, R., et al. (2019). Root growth dynamics and yield responses of rice (oryza sativa L.) under drought—flood abrupt alternating conditions. Environ. Exp. Bot. 157, 11–25. doi:10.1016/j.envexpbot.2018.09.018
Huang, R. (2015). Research on evolution and countermeasures of droughts-floods abrupt alternation events in Huaihe River Basin. [dissertation/doctoral thesis]. Beijing, China: China Institute of Water Resources and Hydropower Research.
Jaman, M. S., Wu, H., Yu, Q., Tan, Q., Zhang, Y., Dam, Q. K., et al. (2022). Contrasting responses of plant above and belowground biomass carbon pools to extreme drought in six grasslands spanning an aridity gradient. Plant Soil 473, 167–180. doi:10.1007/s11104-021-05258-4
Kameoka, E., Suralta, R. R., Mitsuya, S., and Yamauchi, A. (2016). Developmental plasticity of rice root system grown under mild drought stress condition with shallow soil depth; comparison between nodal and lateral roots. Plant Prod. Sci. 19, 411–419. doi:10.1080/1343943X.2015.1128094
Kano-Nakata, M., Gowda, V. R. P., Henry, A., Serraj, R., Inukai, Y., Fujita, D., et al. (2013). Functional roles of the plasticity of root system development in biomass production and water uptake under rainfed lowland conditions. F. Crop. Res. 144, 288–296. doi:10.1016/j.fcr.2013.01.024
Li, D., Qi, H., and Ma, X. Q. (2013). The climate index and assessment about drought and flood in maize’s Key growth stage in Huaibei Plain in Anhui province. Chin. Agric. Sci. Bull. 29, 208–216.
Li, Y., Li, Z., Cui, S., Chang, S. X., Jia, C., and Zhang, Q. (2019). A global synthesis of the effect of water and nitrogen input on maize (Zea mays) yield, water productivity and nitrogen use efficiency. Agr. For. Meteorol. 268, 136–145. doi:10.1016/j.agrformet.2019.01.018
Li, Y., Ma, L., Wu, P., Zhao, X., Chen, X., and Gao, X. (2022). Impacts of interspecific interactions on crop growth and yield in wheat (Triticum aestivum L.)/Maize (Zea mays L.) strip intercropping under different water and nitrogen levels. Agronomy 12, 951. doi:10.3390/agronomy12040951
Liu, S., Wu, W., Yang, X., Yang, P., and Sun, J. (2020). Exploring drought dynamics and its impacts on maize yield in the Huang-Huai-Hai farming region of China. Clim. Change 163, 415–430. doi:10.1007/s10584-020-02880-6
Liu, X., Wang, H., Liu, C., Sun, B., Zheng, J., Bian, R., et al. (2021a). Biochar increases maize yield by promoting root growth in the rainfed region. Arch. Agron. Soil Sci. 67, 1411–1424. doi:10.1080/03650340.2020.1796981
Liu, Y., Zhang, L., Yin, X., Zou, X., and Chen, F. (2021b). Influence of climate change and mechanized harvesting on maize (Zea mays L.) planting and northern limits in northeast China. J. Sci. Food Agric. 101, 3889–3897. doi:10.1002/jsfa.11027
Liu, Z. G., Liu, Z. D., Xiao, J. F., Nan, J. Q., and Gong, W. J. (2013). Waterlogging at seedling and jointing stages inhibits growth and development, reduces yield in summer maize. Trans. Chin. Soc. Agric. Eng. 29, 44–52.
Lu, R. K. (2000). Soil agrochemical analysis methods. Beijing: China Agricultural Science and Technology Press.
Ma, X., Cui, Z., Chen, J., Xiao, C., Zhang, L., and Wang, Q. (2006). Effect of drought stress during seedling stage on the content of grain crude protein, and lysine of maize. J. Maize Sci. 14, 71–74. doi:10.3969/j.issn.1005-0906.2006.02.026
Magno Massuia de Almeida, L., Coulon, M., Avice, J. C., Morvan-Bertrand, A., Bessoule, J. J., Le Guédard, M., et al. (2022). Effects of two-generational heat stress exposure at the onset of seed maturation on seed yield and quality in Brassica napus L. Environ. Exp. Bot. 195, 104788. doi:10.1016/j.envexpbot.2022.104788
IPCC (2021). “Climate change 2021: The physical science basis,” in Contribution of working Group I to the Sixth assessment Report of the intergovernmental panel on climate change. Masson-Delmotte, V., Zhai, P., Pirani, A., Connors, S. L., Pean, C., and Berger, S. (New York: Cambridge University Press).
Meng, Y., Yu, S., Yu, Y., and Jiang, L. (2022). Flooding depth and duration concomitantly influence the growth traits and yield of rice. Irrig. Drain. 71, 94–107. doi:10.1002/ird.2632
Nemeskéri, E., Molnár, K., Rácz, C., Dobos, A., and Helyes, L. (2019). Effect of water supply on spectral traits and their relationship with the productivity of sweet corns. Agronomy 9, 63. doi:10.3390/agronomy9020063
Niones, J. M., Suralta, R. R., Inukai, Y., and Yamauchi, A. (2012). Field evaluation on functional roles of root plastic responses on dry matter production and grain yield of rice under cycles of transient soil moisture stresses using chromosome segment substitution lines. Plant Soil 359, 107–120. doi:10.1007/s11104-012-1178-7
Nishiuchi, S., Yamauchi, T., Takahashi, H., Kotula, L., and Nakazono, M. (2012). Mechanisms for coping with submergence and waterlogging in rice. Rice 5, 2. doi:10.1186/1939-8433-5-2
Norby, R. J., and Jackson, R. B. (2000). Root dynamics and global change: Seeking an ecosystem perspective. New Phytol. 147, 3–12. doi:10.1046/j.1469-8137.2000.00676.x
Piao, S., Ciais, P., Huang, Y., Shen, Z., Peng, S., Li, J., et al. (2010). The impacts of climate change on water resources and agriculture in China. Nature 467, 43–51. doi:10.1038/nature09364
Rao, I. M., Delhaize, E., and Chen, Z. C. (2021). Editorial: Root adaptations to multiple stress factors. Front. Plant Sci. 11, 626960. doi:10.3389/fpls.2020.626960
Sauter, M. (2013). Root responses to flooding. Curr. Opin. Plant Biol. 16, 282–286. doi:10.1016/j.pbi.2013.03.013
Shumway, C. R., Cothren, J. T., Serna-Saldivar, S. O., and Rooney, L. W. (1992). Planting date and moisture effects on yield, quality, and alkaline-processing characteristics of food-grade maize. Crop Sci. 32, 1265–1269. doi:10.2135/cropsci1992.0011183x003200050040x
Singh, S., Singh, G., Singh, P., and Singh, N. (2008). Effect of water stress at different stages of grain development on the characteristics of starch and protein of different wheat varieties. Food Chem. 108, 130–139. doi:10.1016/j.foodchem.2007.10.054
Suralta, R. R., Kano-Nakata, M., Niones, J. M., Inukai, Y., Kameoka, E., Tran, T. T., et al. (2018). Root plasticity for maintenance of productivity under abiotic stressed soil environments in rice: Progress and prospects. F. Crop. Res. 220, 57–66. doi:10.1016/j.fcr.2016.06.023
Uga, Y., Sugimoto, K., Ogawa, S., Rane, J., Ishitani, M., Hara, N., et al. (2013). Control of root system architecture by DEEPER ROOTING 1 increases rice yield under drought conditions. Nat. Genet. 45, 1097–1102. doi:10.1038/ng.2725
Van Soest, P. J. (1973). Collaborative study of acid-detergent fiber and lignin. J. Aoac Int. 56, 781–784. doi:10.1093/jaoac/56.4.781
Van Soest, P. J. (1963). Use of detergents in the analysis of fibrous feeds. II. A rapid method for the determination of fiber and lignin. J. Dairy Sci. 46, 829–835. doi:10.1093/jaoac/46.5.829
Wallemacq, P., UNISDR, , and CRED, (2018). Economic Losses, Poverty and Disasters 1998–2017. doi:10.13140/RG.2.2.35610.08643
Wan, Y., Xue, Q., Liu, L., and Zhao, L. (2016). The role of roots in the stability of landfill clay covers under the effect of dry–wet cycles. Environ. Earth Sci. 75, 71. doi:10.1007/s12665-015-4876-7
Wang, K., Xie, R., Xue, J., Sun, L., and Li, S. (2021). Comparison and analysis of maize grain commodity quality and mechanical harvest quality between China and the United States. Int. J. Agric. Biol. Eng. 14, 55–61. doi:10.25165/j.ijabe.20221501.6099
Wang, P. W., Dai, J. Y., and Wei, Y. (1999). The e ffects of drought stress on yield and quality of maize. J. Maize Sci. 7, 102–106.
Wang, T., Duan, Y., Liu, G., Shang, X., Liu, L., Zhang, K., et al. (2022). Tea plantation intercropping green manure enhances soil functional microbial abundance and multifunctionality resistance to drying-rewetting cycles. Sci. Total Environ. 810, 151282. doi:10.1016/j.scitotenv.2021.151282
Wu, Z., He, J., and Li, J. (2006). The summer drought-flood coexistence in the middle and lower reaches of the yangtze river and analysis of its air-sea background feathers in anomalous years. Chin. J. Atmos. Sci. 30, 570–577. doi:10.3878/j.issn.1006-9895.2006.04.03
Xiong, P., Guo, Z. C., Li, W., Zhang, Z. B., Wang, Y. K., Zhou, H., et al. (2021). Regional analysis of maize yield and physiochemical properties of shajiang black soil (vertusol) in Huaibei Plain. Soils 53, 391–397. doi:10.13758/j.cnki.tr.2021.02.024
Xiong, Q., Shen, T., Zhong, L., Zhu, C., Peng, X., He, X., et al. (2019). Comprehensive metabolomic, proteomic and physiological analyses of grain yield reduction in rice under abrupt drought–flood alternation stress. Physiol. Plant. 167, 564–584. doi:10.1111/ppl.12901
Yang, P., Hu, J., Yu, B., Song, W., and Li, J. (2013). Spatial and temporal characteristics of flood disaster in Asia-Pacific region. J. Shaanxi Normal Univ. Nat. Sci. Ed. 41, 74–81. doi:10.15983/j.cnki.jsnu.2013.01.007
Zhao, C., Liu, B., Piao, S., Wang, X., Lobell, D. B., Huang, Y., et al. (2017a). Temperature increase reduces global yields of major crops in four independent estimates. Proc. Natl. Acad. Sci. 114, 9326–9331. doi:10.1073/pnas.1701762114
Zhao, C., Piao, S., Wang, X., Huang, Y., Ciais, P., Elliott, J., et al. (2017b). Plausible rice yield losses under future climate warming. Nat. Plants 3, 16202. doi:10.1038/nplants.2016.202
Zscheischler, J., Martius, O., Westra, S., Bevacqua, E., Raymond, C., Horton, R. M., et al. (2020). A typology of compound weather and climate events. Nat. Rev. Earth Environ. 1, 333–347. doi:10.1038/s43017-020-0060-z
Keywords: extreme event, biomass, yield, grain quality, summer maize
Citation: Bi W, Weng B, Yan D, Zhang D, Liu C, Shi X, Jing L, Yan S and Wang H (2023) Response of summer maize growth to drought-flood abrupt alternation. Front. Earth Sci. 11:1086769. doi: 10.3389/feart.2023.1086769
Received: 01 November 2022; Accepted: 23 January 2023;
Published: 03 February 2023.
Edited by:
Jiefeng Wu, Nanjing University of Information Science and Technology, ChinaCopyright © 2023 Bi, Weng, Yan, Zhang, Liu, Shi, Jing, Yan and Wang. This is an open-access article distributed under the terms of the Creative Commons Attribution License (CC BY). The use, distribution or reproduction in other forums is permitted, provided the original author(s) and the copyright owner(s) are credited and that the original publication in this journal is cited, in accordance with accepted academic practice. No use, distribution or reproduction is permitted which does not comply with these terms.
*Correspondence: Baisha Weng, d2VuZ2JzQGl3aHIuY29t, d2JzX2l3aHJAMTI2LmNvbQ==