- 1Department of Earth and Planetary Sciences, Northwestern University, Evanston, IL, United States
- 2Institute for Policy Research, Northwestern University, Evanston, IL, United States
- 3United States Geological Survey, Pasadena, CA, United States
Earthquake scientists have traditionally relied on relatively small data sets recorded on small numbers of instruments. With advances in both instrumentation and computational resources, the big-data era, including an established norm of open data-sharing, allows seismologists to explore important issues using data volumes that would have been unimaginable in earlier decades. Alongside with these developments, the community has moved towards routine production of interpreted data products such as seismic moment tensor catalogs that have provided an additional boon to earthquake science. As these products have become increasingly familiar and useful, it is important to bear in mind that they are not data, but rather interpreted data products. As such, they differ from data in ways that can be important, but not always appreciated. Important - and sometimes surprising - issues can arise if methodology is not fully described, data from multiple sources are included, or data products are not versioned (time-stamped). The line between data and data products is sometimes blurred, leading to an underappreciation of issues that affect data products. This note illustrates examples from two widely used data products: moment tensor catalogs and Did You Feel It? (DYFI) macroseismic intensity values. These examples show that increasing a data product’s documentation, independence, and stability can make it even more useful. To ensure the reproducibility of studies using data products, time-stamped products should be preserved, for example as electronic supplements to published papers, or, ideally, a more permanent repository.
1 Introduction
Among the physical sciences, seismology has long been at the forefront with open data policies. This tradition began more than a century ago, driven by the need to use observations from many sites to locate and study earthquakes. Soon after the advent of modern seismometry in the late 19th century (Dewey and Byerly, 1969), arrival times were distributed in published network bulletins, although waveforms recorded on paper or film could not be shared easily. Global catalogs of earthquake data have evolved steadily over the years (Adams, 2002). The first major attempt to gather and publish seismically recorded arrival times was the Publications du Bureau Central de l’Association Internationale de Sismologie (ISA, Rosenthal, 1907), which began in 1904. The International Seismological Summary (ISS) began publication in 1918 and eventually became the Bulletin of the International Seismological Centre (ISC) in 1964. Not only arrival times but also polarities and amplitudes were disseminated, enabling the study of magnitudes and focal mechanisms. Today, high-quality digital seismic networks and analysis centers provide not only raw waveform data but also interpreted data products via the internet. In addition to traditional seismological data and data products, macroseismic data and data products are now increasingly collected in unprecedented volumes. The easy access to data and data products has fueled remarkable growth of the field over the past century. Not only can scientists work more efficiently, but the established practice of openness has encouraged the sharing of models as well as data of different types, and facilitated comparison and testing of results.
In this paper, we illustrate several issues that can arise with widely used data products. Although data products are typically produced with well-vetted methodologies, these products are not data, but rather interpretations. For most applications, the available data products are valuable and adequate. However, for some applications important issues can arise, if methodology is not fully described, data from multiple sources are included, or data products are not versioned (time-stamped). Here, we illustrate issues that have arisen in our studies using two widely used data products. Although these examples involve products by organizations within the United States (US), similar documentation, independence, and stability issues will be potentially important for other widely used seismological data products.
2 Documentation of data products
The increase in computational power and availability of large volumes of digital seismic data have made catalogs of both global and regional seismic moment tensors derived by various agencies widely available (e.g., Duputel et al., 2012; Ekström et al., 2012; Quinteros et al., 2021). These catalogs are a powerful tool in many applications, especially tectonic studies, because the results are sufficiently robust that the details of the inversion generally do not have a large influence on the moment tensor and hence slip or stress directions (Rösler et al., 2021). However, some applications depend on details of the moment tensors, and hence would benefit greatly from documentation of the specifics of the reported tensors and the inversion that produced them.
This issue is illustrated by recent studies comparing and combining moment tensors reported in various catalogs. Comparison is complicated in some cases by differing coordinate systems used in the description of the moment tensors. Most catalogs including the U.S. Geological Survey (U.S. Geological Survey, Earthquake Hazards Program, 2017) and Global Centroid Moment Tensor (Dziewonski et al., 1981; Ekström et al., 2012) catalogs use the up-south-east (USE) coordinate system convention with coordinates r (up), θ (south), and φ (east). However, others including that of the Southern California Earthquake Data Center use the north-east-down (NED) system. Combining solutions or comparing tensor components or quantitates derived from them thus can require transforming components:
Documentation is crucial because of the complexity of the moment tensor inversion process. The inversion models seismograms as a linear combination of Green’s functions weighted by the components of the moment tensor (Gilbert, 1971), which are determined by finding the best fit between observed and synthetic waveforms. The process involves a series of choices. The resulting moment tensor depends on assumptions about elastic and anelastic Earth structure (Šílený, 2004; Cesca et al., 2006; Rößler et al., 2007), the specifics of the inversion algorithm, the number and azimuthal coverage of seismic stations used (Cesca et al., 2006; Ford et al., 2010; Vera Rodriguez et al., 2011; Domingues et al., 2013), the seismic phases and frequencies inverted, and noise in the data (Šílený et al., 1996; Jechumtálová and Šílený, 2001).
Issues related to the moment tensor inversion are illustrated by Rösler et al.’s (2021) study assessing the uncertainty of moment tensor solutions by comparing solutions for the same earthquakes in the U.S. Geological Survey (USGS) and Global Centroid Moment Tensor (GCMT) catalogs. The comparison showed intriguing differences in the reported scalar moments. The USGS calculates the scalar moment as the Euclidian norm of the full deviatoric moment tensor, following Silver and Jordan (1982),
which includes the contribution of the non-double-couple component. In contrast, GCMT neglects the contribution of the NDC component and defines the scalar moment as the average of the two eigenvalues with largest absolute value, which yields the scalar moment of the best double couple,
where
GCMT generally reports larger scalar moments than USGS, with the difference decreasing with magnitude. This difference is larger than and of the opposite sign from that expected due to the different definitions of the scalar moment used in each catalog. Instead, the differences reflect differences in the moment tensor components. These differences are typically an order of magnitude larger than the reported errors, suggesting that estimated errors substantially underestimate the uncertainty. Moreover, the study found surprisingly large differences between the two catalogs in non-double-couple (NDC) components for individual events, reflecting processes other than slip on planar faults. In the absence of isotropic components in moment tensors reported in catalogs, NDC components are compensated linear vector dipoles (CLVDs). Although the differences likely result from methodological differences in the inversion, the methodology used is insufficiently documented for users to assess possible causes of the discrepancies. This would require information about the stations and components used, their weights given during the inversion, and the mathematical method used to obtain the best fit between synthetic and observed waveforms.
Rösler and Stein (2022) identified another difficulty that arises for earthquakes with magnitude M
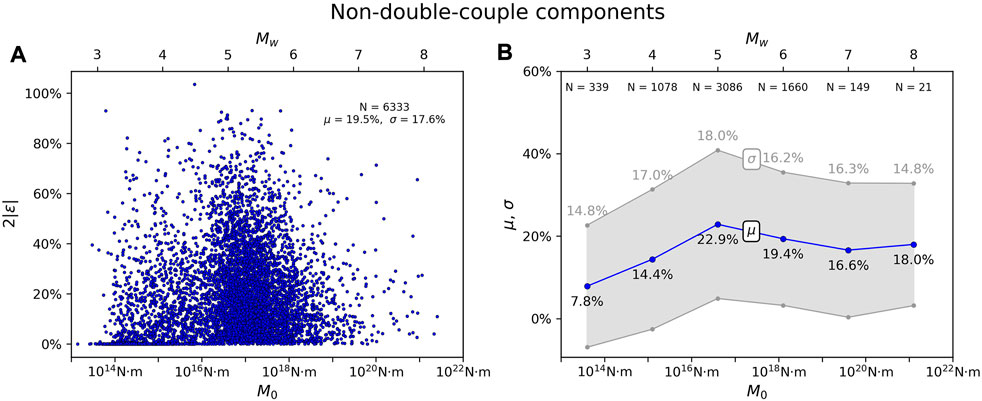
FIGURE 1. Distribution of non-double-couple components with magnitude in the USGS catalog. (A) The large number of earthquakes with no NDC components for M
3 Independence of data products
Comparison of the USGS and GCMT catalogs revealed a further complication, noted briefly by Rösler et al. (2021). Most moment tensor solutions were effectively identical for earthquakes with magnitude M
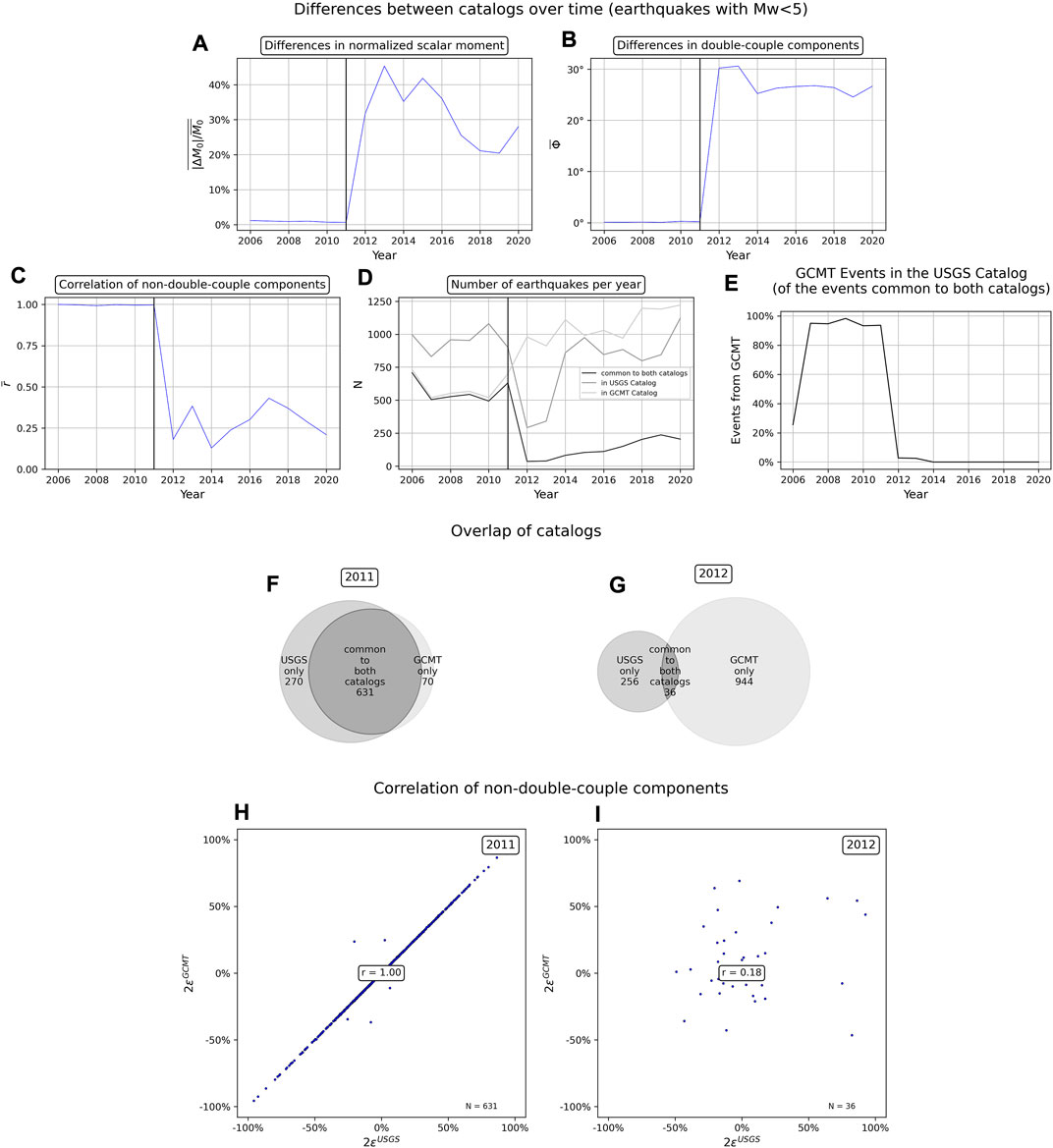
FIGURE 2. Differences in normalized scalar moment (A), in double-couple components (B), and correlation of non-double-couple components (C) between USGS and GCMT catalogs over time. (D) Number of earthquakes in each catalog over time and number of earthquakes common to both catalogs. (E) Number of events obtained from the GCMT catalog in the USGS catalog common to both catalogs over time. (F,G) Number of events common to both catalogs and number of events unique in both catalogs in 2011 and 2012. (H,I) Correlation of NDC components between catalogs in 2011 and 2012.
Independent determination of seismic moment tensors would result in a constant rate of overlapping events between catalogs. However, the number of earthquakes common to both catalogs decreases sharply after 2011 (Figures 2D,F,G), accompanied by a decrease in the number of earthquakes in the USGS catalog. The number of earthquakes in the GCMT catalog, on the other hand, continues to increase as expected considering the increase of seismic stations and hence better availability of seismic data. This suggests changes in the methodology used to produce the USGS catalog.
The USGS provides information about the provenance of the moment tensor solutions in their catalog when taken from other catalogs. Most events in the USGS catalog that are common to both catalogs were obtained from the GCMT before 2011 (Figure 2E), after which time this practice ceased. However, the origin of moment tensors seems not to be correctly indicated for many earthquakes in the USGS catalog. Only 25.6% of earthquakes common to both catalogs in 2006 are indicated to have been obtained from GCMT, whereas the coincidence between DC and NDC components indicates that nearly all moment tensors are identical between catalogs. Additionally, although more than 90% of the earthquakes common to both catalogs in the years 2007–2011 are indicated to have been obtained from GCMT, the differences between catalogs suggest that this percentage is closer to 100%. Apart, this information is not available through the FDSN Event Web Service, and can only be accessed for individual earthquakes in the catalog, or through an additional Python package.
Issues of independence and documentation, which arise when multiple moment tensor catalogs are combined, will become increasingly common as more agencies make such catalogs available. For example, Rösler and Stein (2022) combined moment tensors from three global and four regional catalogs into a dataset of NDC components of 12,856 earthquakes with 2.9
4 Stability of data products
The common blurring of the distinction between data and data products is further illustrated by the collection and interpretation of macroseismic data, defined as the effects of earthquake shaking on people and the built environment. The value of such information has long been recognized (Egen, 1828; Mallet, 1857; Ambraseys, 1971; Bakun and Wentworth, 1997; Gasperini et al., 2010; Sbarra et al., 2020). To investigate earthquakes or their effects, numerical intensity values are assigned based on the severity of shaking at each location, dating back to seminal work in the 19th century (Mallet, 1857). Starting with the introduction of the U.S. Geological Survey “Did You Feel It?” (DYFI) system in 1999 (Wald et al., 1999), intensity values have been determined using algorithms from responses to online questionnaires submitted by eyewitnesses (Wald et al., 1999; Bossu et al., 2015; Bossu et al., 2017; van Noten et al., 2017). The DYFI system has collected information about notable earthquakes that pre-date the introduction of the system (Quitoriano and Wald, 2020) and tens of thousands of reports even for moderate earthquakes. For example, over 1,000 and over 10,000 DYFI responses have been collected to date, respectively, for the 1971 Sylmar, California, and 1994 Northridge, California earthquakes.
Since its inception, the DYFI system has produced unprecedented volumes of intensity information for earthquakes in the United States (Quitoriano and Wald, 2020; and Data and Resources). The system now routinely collects thousands of reports even for moderate earthquakes in urban areas. Responses are analyzed with an algorithm to obtain community decimal intensity (CDI) values, designed to reproduce intensity values that would previously have been assigned subjectively using the modified Mercalli intensity (MMI) scale (e.g., Wood and Neumann, 1931). These data are not available to users due to U.S. Government rules that prohibit sharing of personally identifiable information, including home addresses, that has been collected by a government system. Users can download intensity values via the DYFI web site. Although DYFI values are often referred to as “intensity data,” the values available to users are interpretations - data products - rather than raw data. The term “data product” is used commonly in the geodetic community to describe processed products, such as site velocities derived from GPS data. Terminology has been less precise among the seismological community, as evidenced by the erroneous but widespread usage of “the term “DYFI intensity data”.”
As increasing volumes of DYFI information were collected, studies demonstrated a strong (indeed, “surprisingly good”) consistency between DYFI intensities and instrumental ground motion parameters such as peak ground acceleration (PGA) (Atkinson and Wald, 2007; Worden et al., 2012). DYFI intensities have thus proved useful beyond expectation to characterize earthquake effects, for myriad applications including ground motions investigations (e.g., Hough, 2012), earthquake early warning (e.g., Saunders et al., 2020), and earthquake response (Earle et al., 2009).
Spatially rich DYFI values have been useful to characterize ground motions from induced earthquakes. Following an increase in earthquake rates in parts of the central United States around 2009, a growing volume of literature (Horton, 2012; Ellsworth, 2013; Keranen et al., 2013) established a causal link between the increased seismicity rates and deep injection of wastewater (Healy et al., 1968). By 2013, seismic hazard associated with injection-induced earthquakes was an increasing concern (e.g., Ellsworth, 2013), motivating the U.S. Geological Survey to produce short-term hazard maps aimed at capturing the hazard from induced earthquakes in the central and eastern United States (Petersen et al., 2017; Petersen et al.,2018). Hough (2014), Hough (2015) analyzed DYFI values for moderate earthquakes in the central and eastern United States (CEUS) and concluded that, apart from the very near-field, shaking intensities from induced earthquakes are systematically lower than shaking from tectonic earthquakes of comparable magnitude. Hough (2015) suggested that induced earthquakes had lower stress drop values than tectonic earthquakes in the same area, giving rise to the lower intensities (Hanks and Johnston, 1992).
Subsequent analysis of instrumental data (e.g., Atkinson and Assatourians, 2017; Yoshimitsu et al., 2019) has generally supported the conclusion that stress drops of induced earthquakes in the CEUS are more comparable to stress drops of tectonic earthquakes in the western U.S. than to the high stress drops typically observed for tectonic events in the CEUS (Scholz et al., 1986). Although the results of Hough (2014) and Hough (2015) have generally been corroborated, those results were derived from aggregated DYFI intensities, whose values can potentially change if additional responses are received, or due to processing changes that may be undocumented. Although these changes may be small, they can be systematic and render earlier studies irreproducible. Such a case was brought to the third author’s attention, and motived her to consider stability issues in detail.
Changes in DYFI values due to processing or new responses are not documented, and their analysis is complicated by the fact that DYFI intensity catalogs are not versioned, so files that were used by earlier studies cannot be recreated retroactively. Apart from consideration of files downloaded earlier by researchers, changes can sometimes be seen in time-stamped systems files downloaded over time. We discuss two examples here.
First, changes to DYFI intensities can be illustrated by DYFI values for one of the most notable recent CEUS earthquakes, the 2011 M 5.8 Mineral, Virginia, earthquake, the largest tectonic CEUS earthquake in recent decades. Following (Hough 2014; Hough 2015), we focus on intensity values determined within postal ZIP codes, excluding information from Canada to avoid issues associated with aggregation of data. Over time, the DYFI system has increasingly moved towards aggregation of responses within 1- and 10-km geocoded cells, with user locations increasingly determined using georeferencing. These geocoded intensity values provide better spatial resolution than data aggregated by ZIP code. Although these changes potentially affect geocoded intensity values due to changes in how responses are aggregated, they are irrelevant for the ZIP-code-based values analyzed by Hough (2014) and Hough (2015) and discussed here. Because the spatial extent of ZIP codes is effectively static, focusing on DYFI values aggregated within ZIP codes provides an opportunity to explore how DYFI data can change due to factors other than spatial aggregation.
The earlier studies (Hough, 2014; Hough 2015) analyzed DYFI values downloaded from the USGS web site at different times, but no later than 31 December 2014. Figure 3 presents time-stamped DYFI maps for the earthquake, from 29 August 2011 and 27 June 2017. The panels show that total DYFI responses averaged within ZIP codes for the Mineral earthquake numbered 133,208 in 2011 and 144,178 in 2017. The number of ZIP codes plotted were 8,463 and 8,607, respectively. In general, DYFI responses are overwhelmingly received soon after an earthquake. Given the notoriety of the Mineral event, responses have continued to be submitted, in some cases in response to advertisement of the DYFI page on social media platforms. To visual inspection, intensity maps are effectively indistinguishable (Figure 3). Thus, temporal changes notwithstanding, large DYFI data sets appear to be robust to first order.
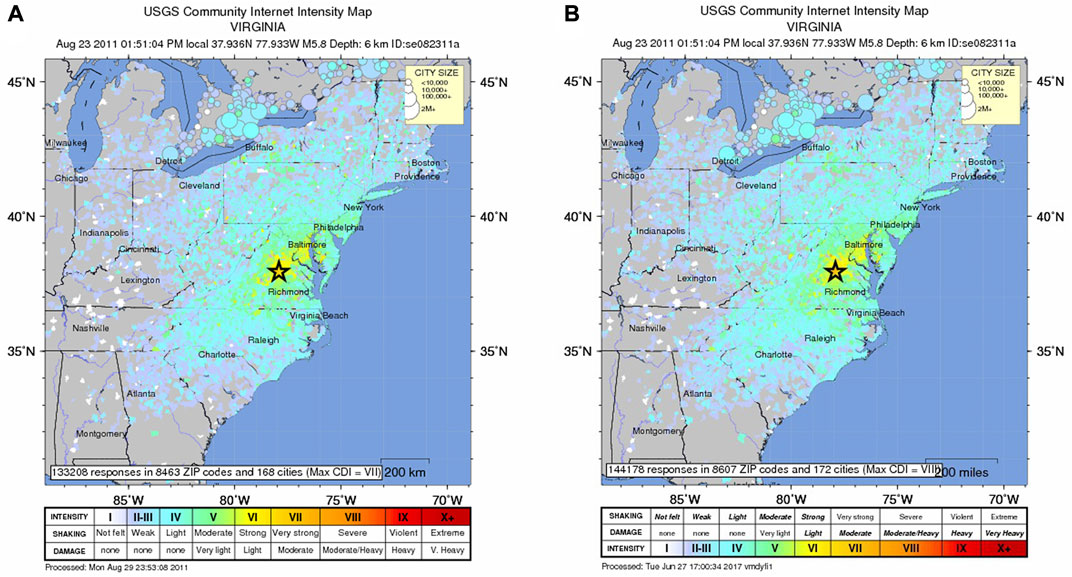
FIGURE 3. (A) Time-stamped map of Did You Feel It? Intensities for the 2011 Mineral, Virginia, earthquake, generated on 29 August 2011. (B) Time-stamped map generated on 27 June 2017. Although maps appear to be consistent to first order, close inspection reveals systematic differences. Differences are more clearly evident in plots showing intensities versus distance (Figure 4).
Systematic small changes in DYFI values may, however, be important for detailed analysis such as the comparisons presented by Hough (2014), Hough (2015). Figure 4 shows time-stamped system files downloaded on 29 August 2011 and 27 June 2017 with DYFI intensities plotted versus distance. Subtle but systematic changes in intensity values are plainly evident in these two panels. The intensity values predicted using the CEUS intensity prediction equation (IPE) developed by Atkinson and Wald (2007) are the same in both panels. Whereas pre-2015 bin-avearged DYFI intensities for the Mineral earthquake at distances between ∼20 and ∼200 km were consistent with the IPE (Figure 4A), values shown in Figure 4B are significantly below the curve over this key distance range. The scatter in individual intensity values is also noticeably different. Because intensity values have dropped systematically over the distance range over which large numbers of responses were received, the systematic change in values serves to negate or lessen the conclusions of Hough (2014), Hough (2015) regarding the comparison of these intensity values with those of the 2011 Prague, Oklahoma earthquake. Systematic differences in DYFI intensities for the Mineral, Virginia, earthquake will furthermore be potentially consequential for any study that uses this earthquake as a calibration event.
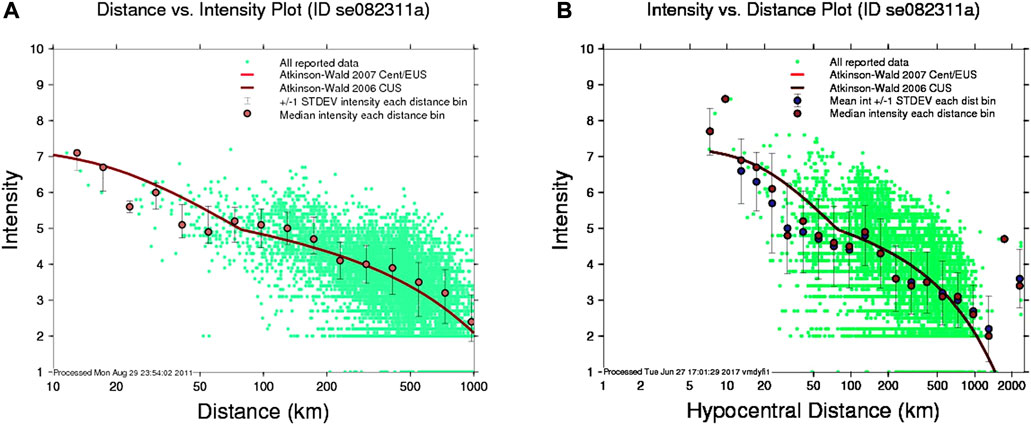
FIGURE 4. Comparison of time-stamped, system-generated Did You Feel It? Intensities versus hypocentral distance for the 2011 Mineral, Virginia, earthquake, downloaded on (A) 29 August 2011 and (B) 27 June 2017. Different plotting conventions were used to generate these plots, but both panels include the same reference intensity prediction equation (IPE; red line). Note that average intensities in (A) are highly consistent with the IPE for distances between 50 and ∼300 km, whereas average intensities in (B) systematically fall below the IPE.
As a second and more recent example, we consider DYFI files for the 19 September 2020, M 4.5 South El Monte, California, earthquake. This earthquake occurred shortly before midnight local time and was widely felt throughout the greater Los Angeles metropolitan area. This was a key event analyzed by Hough (2022), who showed that DYFI participation across the greater Los Angeles area correlated with average household income. As of 17 April, 2021, 22,546 responses had been received by the DYFI system, 5,781 of which were plotted in 591 ZIP codes. As of 30 August 2022, the DYFI system shows a similar map for this event: 21,404 responses, with 5,626 plotted in 590 ZIP codes. Although the total numbers do not differ much, a spot-check comparison of downloaded files reveals a decrease in the numbers of responses in various ZIP codes. In this case, changes appear to have been due to a filter designed to weed out possible duplicate responses, retroactively applied to the South El Monte event in March of 2021 (Vince Quitoriano, oral communication, 2022). Results presented by Hough (2022), which considered files downloaded before February, 2021, are thus now irreproducible.
We note again that DYFI intensities are interpretations of raw macroseismic data that are unavailable to users. DYFI intensity values are calculated from information that evolves over time, with unknown changes in processing. The community has, however, increasingly recognized the value of DYFI products for research, earthquake response, and early warning (e.g., Earle et al., 2009; Hough, 2012; Saunders et al., 2020). It might not be practical or useful to version DYFI values every time new responses are processed. However, to ensure reproducibility of results, values for an event could be versioned periodically. Were it possible to obtain versioned DYFI values retroactively, the effects of such changes could be analyzed systematically. Until then, the results presented here demonstrate that one cannot assume that analysis of earlier DYFI values will be reproducible with current intensity values.
5 Conclusion
Agencies and organizations that provide publicly available large sets of data and data products perform a difficult and valuable service to the seismological community. As advances in instrumentation and computational resources have heralded the modern big-data era, the community has benefited enormously from not only unprecedented volumes of data, but also interpretative data products. As the examples discussed here show, issues of documentation, independence, and stability can arise if methodology is not fully described, data from multiple sources are used, and data products are not versioned. Improving the documentation, independence, and stability of data products would make them even more useful, and help ensure that published results are reproducible. We recognize that addressing these issues will involve additional effort by, and commitment of resources to, the agencies that produce key data products. It is similarly incumbent on authors to preserve and make available the data products on which their conclusions are based, for example in electronic supplements to published manuscripts, or in an appropriate durable repository. Lastly, the issues discussed in this paper underscore a general challenge to the community regarding the need to preserve data products or other interpretive results (e.g., software model runs) that are not data, but can be too large to include in published papers.
Data and resources
The Global Centroid Moment Tensor (Global CMT) Project catalog (available at https://www.globalcmt.org, Dziewonski et al., 1981; Ekström et al., 2012) was last accessed February 2022. The moment tensors of the U.S. Geological Survey (USGS, U.S. Geological Survey, Earthquake Hazards Program, 2017) catalog were downloaded using the Python package ObsPy (Beyreuther et al., 2010) and its International Federation of Digital Seismograph Networks (FDSN) Event Web Service client (last accessed February 2022). Earthquakes with similar source time (60 s), location (difference of less than 1°), and magnitude (M ± 0.5) in both catalogs are considered the same event.
Current Did You Feel It? Data, and information about the earthquakes analyzed in this study, can be accessed via the USGS Comprehensive Earthquake Catalog site, which includes links to DYFI data on each event page: https://earthquake.usgs.gov/earthquakes/search/ (last accessed March 2021).
Data availability statement
All data in this study were freely available at the time they were downloaded.
Author contributions
BR wrote the sections about the documentation and independence of data products and the code to analyze moment tensor catalogs. SH contributed the section about the stability of data products and wrote the code to analyze macroseismic intensity observations. This paper is based on SS’s observation that both branches of seismology rely on data products and face similar challenges. SS also edited the manuscript.
Acknowledgments
The authors thank Roger Bilham for helpful discussions about the work presented in this manuscript and Adam T. Ringler and the two reviewers for constructive feedback about it. We further thank Vince Quitoriano for helpful assistance with DYFI files.
Conflict of interest
The authors declare that the research was conducted in the absence of any commercial or financial relationships that could be construed as a potential conflict of interest.
Publisher’s note
All claims expressed in this article are solely those of the authors and do not necessarily represent those of their affiliated organizations, or those of the publisher, the editors and the reviewers. Any product that may be evaluated in this article, or claim that may be made by its manufacturer, is not guaranteed or endorsed by the publisher.
References
Ambraseys, N. N. (1971). Value of historical records of earthquakes. Nature 232 (5310), 375–379. doi:10.1038/232375a0
Atkinson, G. M., and Assatourians, K. (2017). Are ground motion models derived from natural events applicable to the estimation of expected motions for induced earthquakes? Seismol. Res. Lett. 88 (2), 430–441. doi:10.1785/0220160153
Atkinson, G. M., and Wald, D. J. (2007). ”Did You Feel it?” intensity data: A surprisingly good measure of earthquake ground motion. Seismol. Res. Lett. 78 (3), 362–368. doi:10.1785/gssrl.78.3.362
Bakun, W. H., and Wentworth, C. M. (1997). Estimating earthquake location and magnitude from seismic intensity data. Bull. Seism. Soc. Am. 87 (6), 1502–1521.
Beyreuther, M., Barsch, R., Krischer, L., Megies, T., Behr, Y., and Wassermann, J. (2010). ObsPy: A Python toolbox for seismology. Seismol. Res. Lett. 81 (3), 530–533. doi:10.1785/gssrl.81.3.530
Bossu, R., Landès, M., Roussel, F., Steed, R., Mazet-Roux, G., Martin, S. S., et al. (2017). Thumbnail-based questionnaires for the rapid and efficient collection of macroseismic data from global earthquakes. Seismol. Res. Lett. 88 (1), 72–81. doi:10.1785/0220160120
Bossu, R., Laurin, M., Mazet-Roux, G., Roussel, F., and Steed, R. (2015). The importance of smartphones as public earthquake-information tools and tools for the rapid engagement with eyewitnesses: A case study of the 2015 Nepal earthquake sequence. Seismol. Res. Lett. 86 (6), 1587–1592. doi:10.1785/0220150147
Cesca, S., Buforn, E., and Dahm, T. (2006). Amplitude spectra moment tensor inversion of shallow earthquakes in Spain. Geophys. J. Int. 166 (2), 839–854. doi:10.1111/j.1365-246x.2006.03073.x
Dewey, J., and Byerly, P. (1969). The early history of seismometery (to 1900). Bull. Seism. Soc. Am. 59 (1), 183–227.
Domingues, A., Custodio, S., and Cesca, S. (2013). Waveform inversion of small-to-moderate earthquakes located offshore southwest Iberia. Geophys. J. Int. 1921, 248–259. doi:10.1093/gji/ggs010
Duputel, Z., Rivera, L., Kanamori, H., and Hayes, G. (2012). W phase source inversion for moderate to large earthquakes (1990-2010). Geophys. J. Int. 189 (2), 1125–1147. doi:10.1111/j.1365-246x.2012.05419.x
Dziewonski, A. M., Chou, T. A., and Woodhouse, J. H. (1981). Determination of earthquake source parameters from waveform data for studies of global and regional seismicity. J. Geophys. Res.: Solid Earth 86 (B4), 2825–2852.
Earle, P. S., Wald, D. J., Jaiswal, K. S., Allen, T. I., Hearne, M. G., Marano, K. D., et al. (2009). Prompt Assessment of Global Earthquakes for Response (PAGER) - A system for rapidly determining the impact of earthquakes worldwide. U.S. Geological Survey Open-File Report 2009 1131, 15.
Egen, P. N. C. (1828). Über das Erdbeben in den Rhein- und Niederlanden vom 23 Feb 1828. Ann. Phys. Chem. 13, 153–163. doi:10.1002/andp.18280890514
Ekström, G., Nettles, M., and Dziewonski, A. (2012). The global CMT project 2004-2010: Centroid-moment tensors for 13, 017 earthquakes. Phys. Earth Planet. Interiors 200-201, 1–2019. doi:10.1016/j.pepi.2012.04.002
Ellsworth, W. L. (2013). Injection-induced earthquakes. Science 341 (6142), 1225942. doi:10.1126/science.1225942
Ford, S. R., Dreger, D. S., and Walter, W. R. (2010). Network sensitivity solutions for regional moment-tensor inversions. Bull. Seismol. Soc. Am. 100 (5A), 1962–1970. doi:10.1785/0120090140
Gasperini, P., Vannucci, G., Tripone, D., and Boschi, E. (2010). The location and sizing of historical earthquakes using the attenuation of macroseismic intensity with distance. Bull. Seismol. Soc. Am. 100 (5A), 2035–2066. doi:10.1785/0120090330
Gilbert, F. (1971). Excitation of the normal modes of the Earth by earthquake sources. Geophys. J. Int. 22 (2), 223–226. doi:10.1111/j.1365-246x.1971.tb03593.x
Hanks, T. C., and Johnston, A. C. (1992). Common features of the excitation and propagation of strong ground motion for North American earthquakes. Bulletin of the Seismological Society of America 82 (1), 1–23.
Healy, J. H., Rubey, W. W., Griggs, D. T., and Raleigh, C. B. (1968). The Denver earthquakes. Science 161 (3848), 1301–1310. doi:10.1126/science.161.3848.1301
Horton, S. (2012). Disposal of hydrofracking waste fluid by injection into subsurface aquifers triggers earthquake swarm in central Arkansas with potential for damaging earthquake. Seismol. Res. Lett. 83 (2), 250–260. doi:10.1785/gssrl.83.2.250
Hough, S. E. (2022). Contributed reports of widely felt earthquakes in California, United States: If they felt it, did they report it? Front. Earth Sci. (Lausanne). 1111. doi:10.3389/feart.2021.770445
Hough, S. E. (2012). Initial assessment of the intensity distribution of the 2011 Mw 5.8 Mineral, Virginia, earthquake. Seismol. Res. Lett. 83 (4), 649–657. doi:10.1785/0220110140
Hough, S. E. (2014). Shaking from injection-induced earthquakes in the central and eastern United States. Bull. Seismol. Soc. Am. 104 (5), 2619–2626. doi:10.1785/0120140099
Hough, S. E. (2015). Shaking intensity from injection-induced versus tectonic earthquakes in the central-eastern United States. Lead. Edge 34 (6), 690–697. doi:10.1190/tle34060690.1
Jechumtálová, Z., and Šílený, J. (2001). Point-source parameters from noisy waveforms: Error estimate by Monte-Carlo simulation. Pure Appl. Geophys. 158 (9), 1639–1654. doi:10.1007/pl00001237
Kagan, Y. Y. (1991). 3-D rotation of double-couple earthquake sources. Geophys. J. Int. 106 (3), 709–716. doi:10.1111/j.1365-246x.1991.tb06343.x
Keranen, K. M., Savage, H. M., Abers, G. A., and Cochran, E. (2013). Potentially induced earthquakes in Oklahoma, USA: Links between wastewater injection and the 2011 Mw 5.7 earthquake sequence. Geology 41 (6), 699–702. doi:10.1130/g34045.1
Mallet, R. (1857). Report of the earthquake-expedition to naples, royal soc. Proc. London, United Kingdom: 10/12, Royal Society.
Petersen, M. D., Mueller, C. S., Moschetti, M., Hoover, S. M., Rukstales, K. S., McNamara, D. E., et al. (2018). 2018 one-year seismic-hazard forecast for the central and eastern United States from induced and natural earthquakes. Seismol. Res. Lett. 89 (3), 1049–1061. doi:10.1785/0220180005
Petersen, M. D., Mueller, C. S., Moschetti, M., Hoover, S. M., Shumway, A. M., McNamara, D. E., et al. (2017). 2017 one-year seismic-hazard forecast for the central and eastern United States from induced and natural earthquakes. Seismol. Res. Lett. 88 (3), 772–783. doi:10.1785/0220170005
Quinteros, J., Strollo, A., Evans, P. L., Hanka, W., Heinloo, A., Hemmleb, S., et al. (2021). The GEOFON program in 2020. Seismol. Res. Lett. 92 (3), 1610–1622. doi:10.1785/0220200415
Quitoriano, V., and Wald, D. J. (2020). USGS ”did You Feel it?” - science and lessons from 20 years of citizen science-based microseismology. Front. Earth Sci. (Lausanne). 120. doi:10.3389/feart.2020.00120
Rosenthal, E. (1907). Katalog der im Jahre 1904 registrierten seismischen Störungen. Strassburg, France: Veröffentlichungen des Zentralbureaus der Internationalen Seismologischen Assoziation. Serie B, Kataloge, 145. Available at: http://download.iaspei.org/publications/ISA/catalogues/katalog04.pdf.
Rösler, B., and Stein, S. (2022). Consistency of non-double-couple components of seismic moment tensors with earthquake magnitude and mechanism. Seismol. Res. Lett. 93 (3), 1510–1523. doi:10.1785/0220210188
Rösler, B., Stein, S., and Spencer, B. D. (2021). Uncertainties in seismic moment tensors inferred from differences between global catalogs. Seismol. Res. Lett. 92 (6), 3698–3711. doi:10.1785/0220210066
Rößler, D., Krüger, F., and Rümpker, G. (2007). Retrieval of moment tensors due to dislocation point sources in anisotropic media using standard techniques. Geophys. J. Int. 169 (1), 136–148. doi:10.1111/j.1365-246x.2006.03243.x
Saunders, J. K., Aagaard, B. T., Baltay, A. S., and Minson, S. E. (2020). Optimizing earthquake early warning alert distance strategies using the July 2019 Mw 6.4 and Mw 7.1 Ridgecrest, California, earthquakes. Bull. Seismol. Soc. Am. 110 (4), 1872–1886. doi:10.1785/0120200022
Sbarra, P., Tosi, P., De Rubeis, V., and Sorrentino, D. (2020). Quantification of earthquake diagnostic effects to assess low macroseismic intensities. Nat. Hazards 175, 1957–1973. doi:10.1007/s11069-020-04256-6
Scholz, C. H., Aviles, C. A., and Wesnousky, S. G. (1986). Scaling differences between large interplate and intraplate earthquakes. Bull. Seism. Soc. Am. 76 (1), 65–70.
Šílený, J., Campus, P., and Panza, G. F. (1996). Seismic moment tensor resolution by waveform inversion of a few local noisy records-I. Synthetic tests. Geophys. J. Int. 126 (3), 605–619. doi:10.1111/j.1365-246x.1996.tb04693.x
Šílený, J. (2004). Regional moment tensor uncertainty due to mismodeling of the crust. Tectonophysics 383 (2-4), 133–147. doi:10.1016/j.tecto.2003.12.007
Silver, P. G., and Jordan, T. H. (1982). Optimal estimation of scalar seismic moment. Geophys. J. R. Astronomical Soc. 70 (3), 755–787. doi:10.1111/j.1365-246x.1982.tb05982.x
U.S. Geological Survey, Earthquake Hazards Program (2017). Advanced national seismic system (ANSS) comprehensive catalog of earthquake events and products: Moment tensors. Reston, Virginia: U.S. Geological Survey, Earthquake Hazards Program. doi:10.5066/F7MS3QZH
Van Noten, K., Lecocq, T., Sira, C., Hinzen, K. G., and Camelbeeck, T. (2017). Path and site effects deduced from merged transfrontier internet macroseismic data of two recent <i&gt;M&lt;/i&gt;4 earthquakes in northwest Europe using a grid cell approach. Solid earth. 8, 453–477. doi:10.5194/se-8-453-2017
Vera Rodriguez, I., Gu, Y. J., and Sacchi, M. D. (2011). Resolution of seismic-moment tensor inversions from a single array of receivers. Bull. Seismol. Soc. Am. 101 (6), 2634–2642. doi:10.1785/0120110016
Wald, D. J., Quitoriano, V., Dengler, L., and Dewey, J. W. (1999). Utilization of the internet for rapid community intensity maps. Seismol. Res. Lett. 70 (6), 680–697. doi:10.1785/gssrl.70.6.680
Wood, H. O., and Neumann, F. (1931). Modified Mercalli intensity scale of 1931. Bull. Seismol. Soc. Am. 21 (4), 277–283. doi:10.1785/bssa0210040277
Worden, C. B., Gerstenberger, M. C., Rhoades, D. A., and Wald, D. J. (2012). Probabilistic relationships between ground-motion parameters and modified Mercalli intensity in California. Bull. Seismol. Soc. Am. 102, 204–221. doi:10.1785/0120110156
Keywords: seismological data products, moment tensor catalogs, moment tensor inversion, macroseismic intensities, reproducibility
Citation: Rösler B, Stein S and Hough SE (2022) On the documentation, independence, and stability of widely used seismological data products. Front. Earth Sci. 10:988098. doi: 10.3389/feart.2022.988098
Received: 06 July 2022; Accepted: 03 October 2022;
Published: 18 October 2022.
Edited by:
Nicola Alessandro Pino, National Institute of Geophysics and Volcanology (INGV), ItalyReviewed by:
Domenico Di Giacomo, International Seismological Centre (ISC), United KingdomJan Sileny, Institute of Geophysics (ASCR), Czechia
Copyright © 2022 Rösler, Stein and Hough. This is an open-access article distributed under the terms of the Creative Commons Attribution License (CC BY). The use, distribution or reproduction in other forums is permitted, provided the original author(s) and the copyright owner(s) are credited and that the original publication in this journal is cited, in accordance with accepted academic practice. No use, distribution or reproduction is permitted which does not comply with these terms.
*Correspondence: Boris RöslerLGJvcmlzQGVhcnRoLm5vcnRod2VzdGVybi5lZHU=