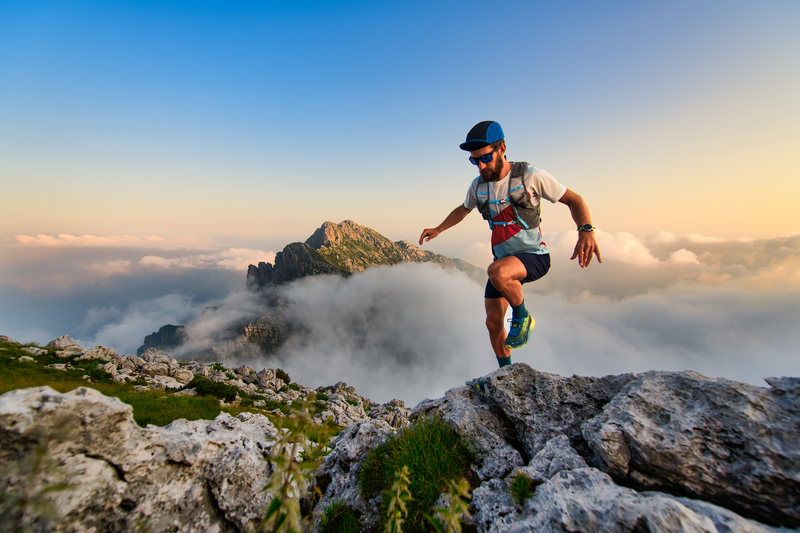
94% of researchers rate our articles as excellent or good
Learn more about the work of our research integrity team to safeguard the quality of each article we publish.
Find out more
ORIGINAL RESEARCH article
Front. Earth Sci. , 05 January 2023
Sec. Environmental Informatics and Remote Sensing
Volume 10 - 2022 | https://doi.org/10.3389/feart.2022.974442
This article is part of the Research Topic Meta-Scenario Computation for Social-Geographical Sustainability View all 60 articles
Ancient landslide has strong concealment and disturbance sensitivity due to its special geotechnical mechanical characteristics, and it is the potential hazard that cannot be ignored in human activities and major engineering planning. The quantitative assessment of ancient landslide reactivation risk has become more necessary for pre-disaster scientific warning. However, because the mechanisms of deformation and damage during the evolution of ancient landslides are quite complex, traditional landslide risk assessment methods only select the single-time scale and relatively stable environmental factors for analysis, lacking consideration of dynamic triggering factors such as rainfall. Focusing on the complexity, a quantitative enhanced assessment for ancient landslide reactivation risk considering cross-time scale joint response mechanism is proposed. First, on the basis of systematic analysis of the implicit genesis mechanism and explicit characterization, an evaluation system of the cross-time scale joint characteristics of ancient landslide reactivation is constructed. Then, XGBoost algorithm and SBAS-InSAR are used to establish the long-time scale developmental evolution mechanism model and the short-time scale dynamical trigger model, respectively. Subsequently, we propose a cross-time scale joint response mechanism. The information entropy weight method is applied to calculate the contribution degree of long-short time scale assessment models for ancient landslide reactivation based on the constraints of quantitative interval thresholds, and the assessment processes of different time scales are dynamically and quantitatively correlated. Finally, the updated optimization of the assessment of ancient landslide reactivation risk is achieved. In this research, experimental analysis was carried out for ancient landslide groups in a geological hazard-prone area in Fengjie County, Chongqing, a typical mountainous region of China. The results of the comparative analysis validate the superiority of the method in this paper. It helps to accurately assess the ancient landslide potential hazard in advance, providing scientific basis and technical support for the risk assessment of mountainous watershed geological hazards and major engineering projects.
Ancient landslide is a slope that has suffered one or more slides which has the deformation trend and potential reactivation risk (Wang S. B. et al., 2021). The reactivation risk of ancient landslides is the probability of slide and occurrence again, due to the influence of environmental and human triggering factors. (Luo et al., 2021; Huang et al., 2022; Xu et al., 2022). Related works indicate a certain path dependency of the dynamic evolution of ancient landslides in time series, which implies a high probability of landslides re-occurring in unstable areas where landslides have occurred before, and these will produce more severe disasters (Samia, 2018; Hu et al., 2022). Therefore, early ancient landslides are potential hazards for human engineering activities, and the precise analysis of the stability condition of ancient landslides and the quantitative assessment of the risk of re-landslides are of vital significance for the safety of people’s lives and properties and scientific disaster prevention and mitigation (Xu et al., 2019; Zhu et al., 2019).
Currently, many researches around landslide risk assessment have been mentioned and expected to be applied in reactivation risk assessment of ancient landslide. These studies can be classified into three categories according to the methodological focus: 1) the method of landslide risk assessment based on expert experience including fuzzy logic method (Zhu Q. et al., 2021), fuzzy comprehensive evaluation (Gu et al., 2012) and analytic hierarchy process (Das et al., 2022). Zhu T. T. et al. (2021) used fuzzy logic method to analyze landslides and obtained the results of landslide environmental factor characteristics and landslide susceptibility. However, such methods are susceptible to the influence by experts’ subjective experience, the accuracy of the analysis results is difficult to guarantee. With the rapid development of earth observation technology, many scholars have proposed 2) the method of landslide risk assessment based on data. The method mainly applies rich observation data such as GNSS, remote sensing and In-SAR to extract information on the spatial and temporal variation of landslides, and then quantitatively analyzes the landslide risk (Li et al., 2021; Zhu Q. et al., 2021; Bondur et al., 2022). In-SAR technology has the advantages of all-day, high precision and large range, which can obtain continuous surface deformation information of a certain area at the scale of centimeter or even smaller (Bondur et al., 2021). Wang N. Y. (2019) used the In-SAR technique to extract landslide deformation points and calculate deformation rates based on multi-period data as a way to classify the degree of landslide susceptibility. Ciampalini et al. (2016) and Yhokha et al. (2018) capture the signal of accelerated surface deformation and the phenomenon of local slow deformation in the early stage of landslide, and then analyze the distribution and development pattern of landslide hazards and conduct risk assessment (Huang et al., 2020; Yan et al., 2021). Rott and Nagler (2006) and Dong et al. (2018) used In-SAR data to analyze landslides and have demonstrated that the In-SAR technique is a reliable approach to improve landslide identification and monitoring. Especially the small baseline subset interferometric synthetic aperture radar (SBAS-InSAR) proposed by Italian scholar Berardino et al. (2002), the precision of its surface deformation monitoring can reach millimeter level, which has a significant superiority in landslide deformation monitoring research ((Lanari et al., 2007; Zhao et al., 2019; Yang, 2020; Zhang et al., 2022). It has been proved that such observation data can well reflect the real state of landslide, but how to further analyze the deformation trend is still a problem. For this problem, 3) the method of landslide risk assessment based on Machine Learning (ML) is mentioned. The use of logistic regression, random forest (Liu et al., 2018; Sun, 2019), artificial neural network (Polykretis and Chalkias, 2018; Sevgen et al., 2019; Hua et al., 2021), support vector machine (Kalantar et al., 2018; Yu et al., 2019), BP (back propagation) neural network and decision tree (Wang N. 2019) for integrated analysis of multiple landslide causal factors to improve the accuracy of landslide assessment analysis. The machine learning method can support the comprehensive analysis of multiple influencing factors, which can more effectively solve the problem of non-linear relationship expression. Moreover, it might achieve better generalization capabilities due to powerful study strategies (Tien Bui et al., 2016). In particular, XGBoost is a type of decision tree model that optimizes classification performance by combining multiple weakly predictive models into a high-accuracy ensemble, following the steepest gradient along a differentiable loss function (Friedman, 2001; Friedman, 2002). The XGBoost algorithm has been preferred by many researchers to fit models with well-documented speed and high predictability for the training dataset, which has already achieved superior results in classification and regression prediction in several fields (Li and Liu, 2019; Pham et al., 2021; Wang R. et al., 2021). Furthermore, some scholars have also adopted it for landslide susceptibility mapping and surface deformation monitoring (Zhao et al., 2018; Stanley et al., 2020). Zhang et al. (2020) noted that the accuracy of XGBoost in identifying landslide points was as high as 91.27%, well above the random forest algorithm, which indicates that the XGBoost algorithm has a high accuracy in landslide research. Chakraborty et al. (2019) used XGBoost to analyze the spatial distribution characteristics, genetic mechanisms and development environments of landslide hazard points in the study area, and demonstrated that it can support information mining of potential associations of landslide features (Chen and Guestrin, 2016), producing regular and generalizable results of landslide hazard environment characteristics.
However, the reactivation process of ancient landslides is very complex, it can be broadly divided into potential creeping and apparent activation process. In the creep process, the environmental conditions in which the ancient landslide is located remain relatively unchanged for a long time, as the basis for breeding hazards, its influence is impossible to ignore; when the ancient landslide is strongly triggered by rainfall or earthquake in short time, it will suddenly produce obvious deformation and reactivation. The existing methods fail to accurately and quantitatively assess the risk of ancient landslide reactivation due to a lack of comprehensive consideration of the environmental and triggering factors, which can easily lead to problems such as randomness, bias and blindness in the assessment results. Therefore, it is necessary to consider the effect of disaster-triggering factors in a short period of time on the basis of environment influence over a long time and then establish more stable and reliable risk assessment models.
Aiming at the above problems, this paper proposes a cross-time scale joint enhanced quantitative assessment method of ancient landslide reactivation risk. Firstly, a landslide factor system including long-time scale and short-time scale factor was established based on the reactivation process characteristics of ancient landslides. Then, XGBoost is used to analyze the long-scale factors and extract the deep environmental feature evolving for a long time, while SBAS-InSAR is used to extract the deformation feature of ancient landslide under the action of short time-scale factors. In particular, a cross-scale joint mechanism constrained by quantitative thresholds is proposed to fusion different scales features, and the results of the ancient landslides reactivation risk assessment using a single scale were updated. Finally, we analyzed the ancient landslides area in Fengjie, Chongqing, China, which can not only improve the assessment accuracy but also identify the potential risk. This method provides an effective way for prevention of ancient landslide reactivation. This paper is organized as follows. Principle of Mechanism Section presents the Principle of Mechanism of the study, including the cross-time scale joint quantitative enhanced assessment model, and its modeling and analyzing process. The study area and data for modeling on analytical framework are viewed in Materials Section, then presents the modeling process of study area in Modeling Process Section and Results and Analysis Section discusses the experimental results. Finally, the conclusions are presented in Conclusion Section.
Regarding to the difficulty of traditional methods to comprehensively consider the characteristics of the disaster-causing factors at different time scales. This paper innovatively proposes a quantitative enhanced assessment mechanism of ancient landslide reactivation risk jointly across time scales, which can jointly analyze landslide evolution characteristics at long-time scale and dynamical trigger characteristics at short-time scale. The assessment of complex ancient landslide reactivation risk based on establishing a dynamic quantitative correlation between the two scale processes, and finally achieving a risk assessment results consistent with the ancient landslide reactivation mechanism. The schematic principle flowchart of this method is shown in Figure 1, and the specific steps include:
1. First, this paper constructs a cross-time scale joint feature evaluation system for ancient landslide reactivation. Divides the causing factors into different single-scale categories according to the temporal characteristics of the factors based on the full consideration of the short-term dynamical triggering process and long-term developmental evolution of ancient landslide deformation.
2. Then, the adaptation analysis model was determined based on the evaluation factors of different time scales, and the quantitative risk assessment of the ancient landslide area was carried out in long-short scales separately. In which, the XGBoost is used to excavate the deep-seated disaster-generating characteristics and deformation damage mechanism of the long-time scale factors and to calculate the spatial-temporal probability of landslide re-occurrence. The SBAS-InSAR technique is used to invert the landslide surface deformation state and intensity based on short-time scale dynamical triggers.
3. Particularly, a cross-time scale joint response mechanism based on quantitative interval thresholds is then proposed to dynamically and quantitatively correlate the assessment processes of different time scales to achieve the updated optimization of the reactivation riskiness of ancient landslides. The information entropy weight method is used to calculate the contribution degree of long-short time scale assessment models for ancient landslide reactivation based on the constraints of quantitative interval thresholds, and the optimal weights are assigned separately and the assigned assessment models are nonlinearly superimposed jointly to obtain updated results on the riskiness of ancient landslide reactivation.
The landslide reactivation mechanism is particularly complex, and the effect of the reactivation characteristic factors on the deformation and damage of the internal structure of the ancient landslide varies with spacetime, showing a nonlinear and unsteady character. Moreover, the ancient landslide is dramatically influenced by external dynamic triggers such as heavy rainfall and sudden changes in reservoir water level. The triggers that induce secondary disasters at key nodes are kinetic and strong, resulting in a dynamic and random short-term activation process of residual landslide deformation.
In this study, the spatial-temporal characteristics of ancient landslide disaster-causing environments were generalized by considering the economic applicability of factor data and historical geography. Based on the principles of systematicity, representativeness, hierarchy and operability, the cross-time scale joint feature evaluation system of ancient landslide reactivation is constructed (Figure 2).
1 long-time scale feature evaluation factor sets: the feature factors of the disaster-generating environment with low correlation are identified, including deep geological factors (faults, stratum), topographic and geomorphological factors (slope, aspect, DEM, NDVI), human engineering factors (roads), and hydrological factors (river systems), which can be used for the study of ancient landslide susceptibility in long-time scales.
2 short-time scale feature evaluation factor sets: for the dynamic triggers that induce reactivation and their characteristics, rainfall and reservoir water level changes were identified as the influencing factors on short-time scales. Among them, rainfall, as a force majeure contingency, is the most important factor to induce landslide deformation in the short term.
Reactivation mechanisms and tendency are frequently correlated with historical disaster-causing environments strongly, leading to a trend for landslides to occur in areas where landslides have already occurred. And ancient landslides also show strongly reactivation characteristics under the combined effect of seasonal rainfall and periodic reservoir water level changes (Liao et al., 2016). Therefore, the assessment of the reactivation risk of ancient landslides requires the analysis of the response characteristics of the action of short-term dynamical triggers based on the full consideration of long-term regional environmental impacts. To address the above problems, this paper constructs a cross-time scale joint response mechanism based on quantitative interval thresholds. Combining the information entropy theory to correlate the assessment processes of different time scales dynamically and quantitatively, which can systematically couple the implicit mechanism and explicit characterization of ancient landslide development and evolution. In-depth analysis of the temporal correlation characteristics existing between long-term disaster-inducing conditions and sudden dynamical triggers, finally achieve the updated optimization of ancient landslide reactivation riskiness.
Step1: Regional rainfall is seasonal, sudden and diverse, and different intensity of rainfall will lead to different degrees of surface deformation and landslide displacement, which will in turn affect the evolutionary process of ancient landslide reactivation in a sudden manner. Therefore, we screen the interval threshold of high intensity of surface deformation subject to rainfall effects with a high landslide susceptibility level based on the wet season for the construction of the joint response mechanism across time scales. To take into account factors at different scales and stages, the joint constraints are expressed formally by wet season (WS), surface deformation (SD) and landslide susceptibility (LS) triads:
The quantitative interval thresholds set in Table 1 are adopted as the constraints for optimizing the entropy method model. (Where, ST = stability threshold, IST = instability threshold, NWS= non-wet season, sus. = susceptibility,
Step2: This paper quantifies the degree of importance of long-short time scale evaluation models for ancient landslide reactivation based on information entropy theory combined with the above joint constraints, as a weighted joint basis for single scale models. Of which, it is crucial to abstract the long-short time-scale assessment model into information factors and use the information entropy weight model to quantitatively calculate the contribution of both. The optimized entropy weight method model was regarded as the basis for updating the risk of ancient landslide reactivation.
The information entropy weight method is a means to determine objective weights, which measures the weights of influencing factors of different scales according to entropy value and the degree of discreteness between evaluation indicators. The entropy value is negatively correlated with the information entropy, and the information entropy can reflect the amount of information among the indicators, which is suitable for the study of complex relationships and correlation characteristics among factors of different scales (Yang and Qiao, 2009). The information entropy weight method has been widely used to determine the weight index of natural hazards, including comprehensive environmental evaluation of natural processes such as landslides and debris flows (Yang and Qiao, 2010; Pourghasemi et al., 2012). The information entropy weight method is calculated by the following formula:
where
Step3: The contribution of the single-scale model calculated by the optimized entropy weight method model is used as the weight, and the nonlinear weighted superposition of the long-short time scale evaluation results is carried out to achieve the cross-time scale joint assessment of the reactivation probability of ancient landslides.
The experimental area adopted in this study is the geographical range between 109°1′17″ and 109°45′58″ east longitude and 30°29′19″ and 31°22′23″ north latitude, which is located in the northern part of Fengjie County, Chongqing (Figure 3, China’s administrative zoning map is derived from the department of natural resources standard map service website (http://211.159.149.56/index.html), whose figure number is: GS (2020) 4632).
Fengjie County is a mountainous area in the eastern part of the Sichuan Basin, which is a geological disaster-prone area in the Three Gorges reservoir area of China. The terrain of Fengjie is undulating and the geological conditions are relatively fragile and it has abundant rainfall and high intensity of short-term storms. The entire area is severely affected by sudden changes in rainfall and reservoir levels throughout the year, leading to increasingly frequent landslide hazards caused. According to statistics, more than 1,000 landslides have occurred in the region since 1970, including 952 ancient landslides that were revived by the rainfall. Meanwhile, the relevant departments have deployed a large number of landslide monitoring instruments and obtained a large amount of data. Therefore, the conditions in the region are suitable for exploring the risk of ancient landslide reactivation at different time scales for research validation.
This paper collects the characteristic data on the disaster-causing factors of ancient landslides in Fengjie County, involving surface topography and geomorphology, deep geological structure, hydrology, and human engineering activities, for conducting risk assessment analysis of ancient landslide reactivation in the study area.
Data sources include:1) 30 m resolution DEM data 2) 2.5 m resolution slope and aspect data 3) 1:200,000 vector geological map for extracting information on stratum and faults 4) 1:5,000 national geo-monitoring data for extracting data on roads and river systems 5) 30 m resolution Landsat 8 satellite digital products for extracting normalized difference vegetation index (NDVI) 6) Monthly precipitation generated by processing China Surface Climate Information Daily Value Dataset V3.0 7) 952 ancient landslide points, contained in the 2018 historical landslide dataset, for analysis of the distribution status and patterns of historical landslide sites. Other primary data information is shown in Table 2.
Single Look Comple (SLC) SAR image data in Interferometric Wide (IW) mode from the Earth observation satellite Sentinel 1A are acquired in this study. 46 Sentinel-1A ascending orbital data covering the study area from January 2018 to December 2019 are selected for SBAS-InSAR processing to analyze the surface deformation characteristics ancient landslide at short time scales. In addition, Sentinel 1A has good baseline control and shorter revisiting times (12 days), which accumulates a large amount of high-precision radar image data in a relatively short period of time and can obtain time-domain continuous surface deformation characteristic information through its phase information (Huang et al., 2020). Sentinel 1A has been widely used in the fields of ground subsidence monitoring and landslide deformation monitoring. At the same time, the surface runoff and infiltration generated during the rainfall process are quite prone to the reactivation of larger-scale ancient landslide, so we specifically selected SAR image data containing before and after the wet season to extract information on the landslide surface deformation generated during this period.
Ancient landslide reactivation is generally the consequence of coupled deep-surface multi-factors. The deformation and damage mechanism during the long-term development and evolution of ancient landslides is relatively complex with numerous deformation influencing factors. This study combines the basic geographic conditions of the experimental area and the knowledge pattern of ancient landslides to summarize and analyze the response pattern of long-time scale feature evaluation factors to the regional disaster environment and the mechanism of ancient landslide development and evolution. By quantifying the influence of spatial-temporal effects of historical observation data on deep geological conditions (e.g. faults, geological rock formations, etc.) and topographic and geomorphological conditions (e.g. slope, aspect, NDVI, etc.) during the development of ancient landslides, we hierarchically quantify the magnitude of the probability of occurrence of the ancient landslide reactivation hazard. In this research, the XGBoost model was adopted to construct the long-time scale developmental evolution mechanism model for ancient landslide susceptibility evaluation.
In this research, landslide units and non-landslide units formed the sample dataset for the experiment, and the landslide units consisted of 952 historical landslide events. We finally chose a ratio of 1:10 to construct non-disaster negative sample points after several experiments (Sun et al., 2021). Moreover, in order to select truly “non-landslides” as closely as possible, the 500 m buffer zone of historical landslide points and major river system were avoided. Subsequently, the multi-source heterogeneous experimental data were pre-processed spatially, including data format and spatial reference unification. Since there were two types of continuous and discrete in the evaluation factors of ancient landslide reactivation features, they were quantified by classification or discretized by grading in order to unify the model inputs, respectively. The final processed sample data is a two-dimensional array of 10474×8 (10474 is the number of samples and 8 is the number of features) with element values of 0 or 1, where 1 is for landslide samples and 0 is for non-landslide samples.
XGBoost is a high-efficiency integrated learning algorithm based on decision trees. In this algorithm, the stepwise forward additive model is adopted to reduce the risk of overfitting by optimizing the structured loss function, and multiple preferred weak learners (decision trees) are combined to accomplish the learning task and thus achieve a superior classification model (Sahin, 2020; Can et al., 2021; Stanley et al., 2021). With the addition of the regularization term, the algorithm will select a simple and well-performing model. The regularization term at the right end of the loss function is designed to suppress overfitting of the weak learner in each iteration, but is not involved in the integration of the ultimate model. The objective function of XGBoost is as follows:
Where
where
where
In this work, the processed long-time scale feature evaluation factor data are used as the input of the XGBoost model, and 70% of the sample data are extracted for training the model and 30% are applied for prediction to verify the model accuracy using the random selection and 5-fold cross-validation methods. Then, on the basis of XGBoost algorithm, a long-time scale evolution mechanism model was established to quantitatively calculate the spatio-temporal probability of the reactivation of the ancient landslide development process. Eventually, the obtained ancient landslide susceptibility maps were reclassified into five classes using the quantile method: very low susceptibility, low susceptibility, moderate susceptibility, high susceptibility, and very high susceptibility (Figure 4).
Since the dynamic evolution of ancient landslide reactivation hazards are remarkably influenced by external dynamic-induced factors such as heavy rainfall and sudden changes in reservoir water level, the process of transforming ancient landslides from stable to unstable states is extremely short. However, the triggering factors do not simply obey the relevant laws of mathematical statistics in spacetime. When the ancient landslide has a tendency of peristaltic deformation in the long time series development and evolution, the cumulative displacement of the ground surface will show “stepwise” sudden changes in a short time series under the influence of external dynamics factors. Single-scale static long time series analysis is unable to simply obtain the correlation characteristics between dynamic triggers and landslide deformation. The amount of information on the spatial and temporal evolution of landslide hazards based only on data-driven landslide susceptibility analysis is relatively one-sided and lagging, and is prone to the problem of underfitting and overfitting of risk assessment analysis results, which directly affects the accuracy and reliability of ancient landslide reactivation risk assessment. Therefore, this study uses SBAS-InSAR technology to establish dynamic correlations of short-term kinetic trigger processes, and generalize and analyze the dynamic triggers (e.g., heavy rainfall, reservoir level changes) that induce the ancient landslide reactivation at key nodes of developmental evolution. To establish a short time-scale kinetic trigger model to cope with the shortage of short-term triggers such as suddenness and randomness. Finally, a short-time scale dynamical trigger model is established to overcome the shortage of short-term triggers such as suddenness and randomness.
SBAS-InSAR acquires the surface deformation time series by the least squares method and performs temporal fitting with singular value decomposition (SVD), which effectively improves the temporal resolution of monitoring. Further, the minimum-parametric least-squares value of the surface deformation rate between image sequences is sought (Berardino et al., 2002). The basic principle of SBAS-InSAR is as follows (Figure 5):
The M-scene SAR images of the same region are acquired in the time period from
The interferometric phase produced at the point (x, r) for the
where
Finally, the phase unwinding of the interferograms of N SAR images can obtain the deformation rates of different SAR images at the acquisition time.
In this study, the surface displacement and deformation velocity in the line of sight direction (
In this section, based on the above joint response mechanism, a cross-time scale joint enhanced quantitative assessment model for ancient landslide reactivation risk is developed. First, the single-scale quantitative assessment model was reclassified into five classes separately. In which, the interval threshold of surface deformation instability is |
A cross-time scale riskiness map of ancient landslide reactivation based on the joint response mechanism with constraints is derived. After setting the quantitative interval threshold, the optimized entropy weight method calculates the optimal weight to minimize the density of historical landslides in very low and low susceptibility areas and maximize the density of historical landslides in high susceptibility areas. Compared with the landslide susceptibility map, the ancient landslide reactivation risk map is consistent with the geospatial characteristics of historical landslide occurrence, fixing the overfitting and underfitting that occurred in the data processing. The refinement of landslide susceptibility using surface deformation data enables the assessment results to be more realistic, objective and reliable, making the evaluation results of ancient landslide reactivation risk more reasonable (Figure 8). Consequently, the optimized landslide reactivation risk map has absolute stability and relatively high precision.
FIGURE 8. Landslide maps at different scales. (A) Long-time scale landslide susceptibility map (B) Cross-time scale landslide risk map.
From the optimized riskiness map of ancient landslide reactivation, we found that the very-high-risk areas are mainly distributed in a band pattern on both sides of the Yangtze River and its major tributaries. This area has an extremely large number of ancient landslides, with 572 ancient landslides points, accounting for more than 60% of the ancient landslides in the study area. The ancient landslides occurring in the area are mainly influenced by the erosion of the Yangtze River and its tributaries by washing. The lithology is mainly soft rocks such as the Middle Triassic Badong Formation, and the joint action of stratum and geological structure has formed a penetrating soft zone, which provides favorable conditions for the occurrence of ancient landslide reactivation disasters. After the Three Gorges Reservoir was fully impounded, the long-term action of groundwater due to rainfall exacerbated the deep deformation of the reservoir bank slopes, leading to many apparent historical landslide events over a long time series. The high-risk area is mainly located on both sides of the river and on both sides of the very high-risk area, which is also distributed in a band pattern, with 278 landslide points. Other risk areas are distributed in patches throughout the study area, and there are fewer landslides developed in these areas. The joint cross-scale landslide riskiness maps generally exhibited a higher degree of riskiness than the long-scale landslide susceptibility maps, which was caused by fusing SBAS-InSAR surface deformation monitoring results (Figure 9). As a result, this technique can effectively reduce the possibility of false negatives in long-scale models and extremely improve the precision of risk assessment.
FIGURE 9. Comparative maps of typical areas at different scales. (A) Long-time scale landslide susceptibility map (B) Cross-time scale landslide risk map.
Furthermore, in this section, the spatial superposition analysis of the optimized updated results of the ancient landslides reactivation risk and the distribution of historical landslide disaster points is carried out. Also making a comprehensive statistic of the area ratio, landslide ratio and landslide frequency ratio of zoning. From the statistical results (Table 4), it can be seen that the landslide ratio and landslide frequency ratio increase with the increase of zoning class. Among them, 92 and 98% of the historical landslide hazard points fall in the medium-risk area and above of the landslide susceptibility map and the cross-time scale landslide riskiness map, respectively. More of the occurred ancient landslide events fall in the medium-high risk area, indicating that the overall accuracy of the optimized and updated landslide riskiness map is greatly improved. Of these, 18.18% of the very high susceptible zone area covers 56.99% of the historical landslide hazard sites, and 22.46% of the very high-risk zone area covers 60.15% of the historical landslide hazard sites, with an increase in both the area of the zone and the number of ancient landslides that have occurred. The frequency ratio of landslides in the low-susceptibility area and the low-risk area are both less than 1. The updated landslide risk map only accounts for 1.79% of the ancient landslide hazard points and the density of ancient landslides is extremely low, indicating that ancient landslide hazard points are concentrated in the optimized medium-high risk zone. The results of landslide risk assessment in this experimental study area can better and realistically reflect the spatial distribution of the occurrence of ancient landslides, and fully verify the reliability and validity of the cross-time scale joint response mechanism.
TABLE 4. Statistical results of landslide susceptibility zoning and risk zoning based on cross-time scale joint response mechanism.
However, the single-scale landslide susceptibility map has steep landslide frequency ratio curves in the high and very-high susceptibility areas with large leap order standard deviations, and the smoothness and reasonableness of the zoning results are insufficient. The ancient landslide reactivation risk map fixes the overly varying landslide frequency ratio of the susceptibility map, making the data more smooth and more stable and with higher preciseness (Figure 10). The experimental results indicated that the joint results of the SBAS-InSAR technology-based ground deformation evaluation model and the XGBoost model-based landslide susceptibility evaluation model obtained based on the cross-time scale joint response mechanism could indeed update and improve the sensitivity of ground deformation and optimize the final risk assessment results, making the evaluation results of the model more reasonable and convincing.
FIGURE 10. Statistical chart of area ratio, landslide ratio and landslide frequency ratio. Where, SLR = susceptibility landslide ratio, RLR = risk landslide ratio, SLFR = susceptibility landslide frequency ratio, RLFR = risk landslide frequency ratio.
The experimental results showed that the average surface deformation and surface deformation velocity in the study area varied significantly in the short time series after the beginning of wet season (Figure 11). Before the wet season (Feb 2018–April 2018), the surface deformation changes were relatively stable, the deformation displacement of the slope was mainly within the range of −14.1–18.4 mm, and the minor deformation means lower probability of ancient landslide reactivation. However, by plotting of monthly precipitation and surface deformation against time for the study area in 2018 (Figure 12), we can clearly observe that: The monthly precipitation in May reached its peak throughout the year. Starting from the intense wet season (May 2018–September 2018), with the increase of accumulated rainfall, the geological environment was greatly affected by the change of water level, which led to an ever-increasing amount of both surface uplift and subsidence, and the magnitude of displacement increased with rainfall intensity. Particularly, the surface deformation increased to the range of −56.7–56 mm in September, and the accumulated deformation displacement added to the maximum. As a result, the risk of ancient landslide reactivation has also been increased throughout the region due to a sharp and extremely rapid increase in surface deformation triggered by major external triggering factors such as rainfall, resulting in a particularly large area of high and very-high risk areas.
FIGURE 12. Monthly precipitation and surface deformation in the study area plotted against time in 2018.
Comparing the updated optimized cross-time scale model and short-time scale model in temporal and spatial dimensions, the following summary can be drawn: 1) Temporally, during the non-wet season, the surface deformation changes less and most areas should show non-sensitive characteristics. However, during the wet season, when cumulative rainfall increases from May to September, the surface deformation increases sharply and areas of increased risk are widely distributed over the study area, with a consequent enhancement in the probability of ancient landslide reactivation. 2) Spatially, the areas of surface deformation are mainly distributed around rivers and special geological formations. Especially after the rainfall, the sudden change of reservoir water level may also be an important influencing factor for the ancient landslide reactivation. As the reservoir water level rises, the rapid deformation of the reservoir bank landslide under the action of pore water pressure, and the influence range of reservoir water fluctuation increases accordingly, leading to the rising risk of reactivation of ancient landslide. At this point, the contribution of the long-time scale factor water system is relatively larger. The very-high risk area of the ancient landslide reactivation requires focused attention from decision makers.
In this study, the XGBoost algorithm is used to excavate the deformation damage mechanism during the long-term evolution of the ancient landslide, and the surface deformation inversion by SBAS-InSAR technique is used to clear the potential sensitivity of some area in landslide. Compared with the conventional model, the enhanced assessment based on cross-time scale joint response mechanism highlights the risk classes improved by the surface deformation velocity, and the classification of landslide riskiness is more accurate, realistic and reliable. It is specifically reflected in the follows:
1) From the perspective of extracting the significant hazard, the false negative of each landslide susceptibility class is reduced by more than 20% based on cross-time scale joint response mechanism. It indicated that t the cross-time scale joint response mechanism can effectively update the risk level and reduce misreporting and omission, which has outstanding advantages for avoiding false negatives.
2) From the perspective of extracting the potential hazard, the results of landslide risk assessment based on cross-time scale joint response mechanism show that about 18.18% of the area is updated to a high risk level. It indicated that the cross-time scale joint response mechanism can capture the signal of accelerated ground deformation before the ancient landslide reactivation, improve the risk level of landslides characterized by ground motion.
3) From the perspective of model scalability and migratability, the reactivation of ancient landslides is not only significantly associated with rainfall, but also with short-scale factors such as earthquakes and reservoir rise-fall. Combining this information based on cross-time scale joint response mechanism can make the assessment results more accurate in different environments.
Consequently, we believe that the cross-time scale joint enhanced quantitative assessment method of ancient landslide reactivation risk may be a more effective method to assess the potential riskiness of secondary landslides, and can be applied to landslide mapping and quantitative risk management on a regional scale, which is of great value for effective land use management and project planning.
The original contributions presented in the study are included in the article/Supplementary material, further inquiries can be directed to the corresponding author.
Author Contributions: Conceptualization, XX; methodology, ZY, TW, and CE.; software, ZY, and CE; validation, TW and LT; formal analysis, XX, CE, and XJ; investigation, TW and XX; resources, XX; data curation, XX; writing—original draft preparation, ZY, TW, and XX; writing—review and editing, XX and CE; visualization, TW and ZY; supervision, XX; project administration, XX; funding acquisition, XX. All authors have read and agreed to the published version of the manuscript.
Supported by The Shenyang Young and Middle-aged Scientific and Technological Talents Program (RC210502); The Weifang Science and Technology Project (2021ZJ1134); The Support of Science and Technology for the Sichuan-Tibet Railway Special Project (XZ202101ZD0001G) and the LZJTU EP 201806.
We are grateful to Qing Zhu (Southwest Jiaotong University) and Tao Liu (Lanzhou Jiaotong University) for their guidance on this study. Zhu provided valuable professional advice both at the start of the study and during the refinement process.
XJ is employed by the company Jiangshan Weiye Surveying and Mapping Co., Ltd.
The remaining authors declare that the research was conducted in the absence of any commercial or financial relationships that could be construed as a potential conflict of interest.
All claims expressed in this article are solely those of the authors and do not necessarily represent those of their affiliated organizations, or those of the publisher, the editors and the reviewers. Any product that may be evaluated in this article, or claim that may be made by its manufacturer, is not guaranteed or endorsed by the publisher.
Berardino, P., Fornaro, G., Lanari, R., and Sansosti, E. (2002). A new algorithm for surface deformation monitoring based on small baseline differential SAR interferograms. IEEE Trans. Geosci. Remote Sens. 40 (11), 2375–2383. doi:10.1109/tgrs.2002.803792
Bondur, V., Chimitdorzhiev, T., Dmitriev, A., and Dagurov, P. (2021). Fusion of SAR interferometry and polarimetry methods for landslide reactivation study, the bureya river (Russia) event case study. Remote Sens. (Basel). 13 (24), 5136. doi:10.3390/rs13245136
Bondur, V. G., Chimitdorzhiev, T. N., Dmitriev, A. V., and Dagurov, P. N. (2022). Assessment of the Bureya River landslide reactivation using the persistent scatterer interferometry. Dokl. Earth Sci. 502 (1), 31–36.
Can, R., Kocaman, S., and Gokceoglu, C. (2021). A comprehensive assessment of XGBoost algorithm for landslide susceptibility mapping in the upper basin of Ataturk dam, Turkey. Appl. Sci. (Basel). 11 (11), 4993. doi:10.3390/app11114993
Chakraborty, T., Alam, M. S., and Islam, M. D. (2019). “Landslide susceptibility mapping using XGBoost model in Chittagong District, Bangladesh,” in Proceedings on International Conference on Disaster Risk Management, Dhaka, Bangladesh, 431–434.
Chen, T., and Guestrin, C. (2016). “XGBoost,” in Proceedings of the 22nd ACM SIGKDD International Conference on Knowledge Discovery and Data Mining - KDD ’16, New York, USA, 785–794. doi:10.1145/2939672.2939785
Ciampalini, A., Raspini, F., Lagomarsino, D., and Catani, F. (2016). Landslide susceptibility map refinement using PSInSAR dataLandslide susceptibility map refinement using PSInSAR data. Remote Sens. Environ. 184, 302–315. doi:10.1016/j.rse.2016.07.018
Das, S., Sarkar, S., and Kanungo, D. P. (2022). GIS-based landslide susceptibility zonation mapping using the analytic hierarchy process (AHP) method in parts of Kalimpong Region of Darjeeling Himalaya. Environ. Monit. Assess. 194 (3), 234–328. doi:10.1007/s10661-022-09851-7
Dong, J., Zhang, L., Li, M., Yu, Y., Liao, M., Gong, J., et al. (2018). Measuring precursory movements of the recent Xinmo landslide in Mao County, China with Sentinel-1 and ALOS-2 PALSAR-2 datasets. Landslides 15 (1), 135–144. doi:10.1007/s10346-017-0914-8
Friedman, J. H. (2001). Greedy function approximation: A gradient boosting machine. Ann. Stat. 29, 1189–1232. doi:10.1214/aos/1013203451
Friedman, J. H. (2002). Stochastic gradient boosting. Comput. Stat. Data Anal. 38 (4), 367–378. doi:10.1016/s0167-9473(01)00065-2
Gu, C. Z., Yang, H. F., Hu, X. F., Zeng, J. X., and Luo, G. (2012). Analysis of energy conversion of earthquake-induced landslide based on fuzzy synthetic evaluation. Rock Soil Mech. 33 (S1), 297–302.
Hu, S., Qiu, H. J., Wang, N. L., Wang, X. G., Ma, S. Y., Yang, D. D., et al. (2022). Movement process, geomorphological changes, and influencing factors of a reactivated loess landslide on the right bank of the middle of the Yellow River, China. Landslides 19 (6), 1265–1295. doi:10.1007/s10346-022-01856-0
Hua, Y., Wang, X., Li, Y., Xu, P., and Xia, W. (2021). Dynamic development of landslide susceptibility based on slope unit and deep neural networks. Landslides 18 (1), 281–302. doi:10.1007/s10346-020-01444-0
Huang, J. X., Xie, M. W., and Atkinson, P. M. (2020). Dynamic susceptibility mapping of slow-moving landslides using PSInSAR. Int. J. Remote Sens. 41 (19), 7509–7529. doi:10.1080/01431161.2020.1760398
Huang, X., Wang, L., Ye, R., Yi, W., Huang, H., Guo, F., et al. (2022). Study on deformation characteristics and mechanism of reactivated ancient landslides induced by engineering excavation and rainfall in Three Gorges Reservoir area. Nat. Hazards (Dordr). 110 (3), 1621–1647. doi:10.1007/s11069-021-05005-z
Kalantar, B., Pradhan, B., Naghibi, S. A., Motevalli, A., and Mansor, S. (2018). Assessment of the effects of training data selection on the landslide susceptibility mapping: A comparison between support vector machine (SVM), logistic regression (LR) and artificial neural networks (ANN). Geomat. Nat. Hazards Risk 9 (1), 49–69. doi:10.1080/19475705.2017.1407368
Lanari, R., Casu, F., Manzo, M., and Lundgren, P. (2007). Application of the SBAS-DInSAR technique to fault creep: A case study of the hayward fault, California. Remote Sens. Environ. 109 (1), 20–28. doi:10.1016/j.rse.2006.12.003
Li, X. E., Zhou, L., Su, F. Z., and Wu, W. Z. (2021). Application of InSAR technology in landslide hazard: Progress and prospects. Natl. Remote Sens. Bull. 25 (02), 614–629.
Li, Z. S., and Liu, Z. G. (2019). Feature selection algorithm based on XGBoost. J. Commun. 40 (10), 101–108.
Liao, H. B., Yin, K. L., and Wang, G. H. (2016). Dynamic mechanism of intermittent reactivation of deep-seated reservoir ancient landslide. Rock Soil Mech. 37 (09), 2645–2653. doi:10.16285/j.rsm.2016.09.029
Liu, J., Li, S. L., and Chen, T. (2018). Landslide susceptibility assesment based on optimized random forest model. Geomatics Inf. Sci. Wuhan Univ. 43 (7), 1085–1091.
Luo, G., Ren, G., Bao, X., Yang, X., and Liu, T. (2021). Stability analysis of the shiliushubao landslide based on deformation characteristics and external trigger factors in the three Gorges reservoir. Adv. Civ. Eng. 2021, 1–12. doi:10.1155/2021/2714918
Pham, Q. B., Achour, Y., Ali, S. A., Parvin, F., Vojtek, M., Vojteková, J., et al. (2021). A comparison among fuzzy multi-criteria decision making, bivariate, multivariate and machine learning models in landslide susceptibility mapping. Geomat. Nat. Hazards Risk 12 (1), 1741–1777. doi:10.1080/19475705.2021.1944330
Polykretis, C., and Chalkias, C. (2018). Comparison and evaluation of landslide susceptibility maps obtained from weight of evidence, logistic regression, and artificial neural network models. Nat. Hazards 93 (1), 249–274. doi:10.1007/s11069-018-3299-7
Pourghasemi, H. R., Mohammady, M., and Pradhan, B. (2012). Landslide susceptibility mapping using index of entropy and conditional probability models in GIS: Safarood Basin, Iran. Catena 97, 71–84. doi:10.1016/j.catena.2012.05.005
Rott, H., and Nagler, T. (2006). The contribution of radar interferometry to the assessment of landslide hazards. Adv. Space Res. 37 (4), 710–719. doi:10.1016/j.asr.2005.06.059
Sahin, E. K. (2020). Assessing the predictive capability of ensemble tree methods for landslide susceptibility mapping using XGBoost, gradient boosting machine, and random forest. SN Appl. Sci. 2 (7), 1308–1317. doi:10.1007/s42452-020-3060-1
Samia, J. (2018). Landslide path dependency and its impact on space-time modelling of landslide susceptibility, PhD Thesis. Wageningen University.
Sevgen, E., Kocaman, S., Nefeslioglu, H. A., and Gokceoglu, C. (2019). A novel performance assessment approach using photogrammetric techniques for landslide susceptibility mapping with logistic regression, ANN and random forest. Sensors 19 (18), 3940. doi:10.3390/s19183940
Stanley, T. A., Kirschbaum, D. B., Benz, G., Emberson, R. A., Amatya, P. M., Medwedeff, W., et al. (2021). Data-driven landslide nowcasting at the global scale. Front. Earth Sci. 378. doi:10.3389/feart.2021.640043
Stanley, T. A., Kirschbaum, D. B., Sobieszczyk, S., Jasinski, M. F., Borak, J. S., and Slaughter, S. L. (2020). Building a landslide hazard indicator with machine learning and land surface models. Environ. Model. Softw. 129, 104692. doi:10.1016/j.envsoft.2020.104692
Sun, D. L. (2019). Mapping landslide susceptibility based on machine learning and forecast warning of landslide induced by rainfall, PhD Thesis. East China Normal University.
Sun, D., Wen, H., Xu, J., Zhang, Y., Wang, D., and Zhang, J. (2021). Improving geospatial agreement by hybrid optimization in logistic regression-based landslide susceptibility modelling. Front. Earth Sci. 686. doi:10.3389/feart.2021.713803
Tien Bui, D., Tuan, T. A., Klempe, H., Pradhan, B., and Revhaug, I. (2016). Spatial prediction models for shallow landslide hazards: A comparative assessment of the efficacy of support vector machines, artificial neural networks, kernel logistic regression, and logistic model tree. Landslides 13 (2), 361–378. doi:10.1007/s10346-015-0557-6
Wan, D., Ding, Y., Lu, Y., Jiang, X., and Xiong, G. (2021). Study on the mechanism of Xiajiang Hydraulic Project landslide reactivation based on long-term in situ monitoring techniques. IOP Conf. Ser. Earth Environ. Sci. 861 (4), 042046. doi:10.1088/1755-1315/861/4/042046
Wang, N. (2019). Study on early warning of rainfall-induced landslide in bazhou district, Master’s Thesis. University of Electronic Science and Technology of China.
Wang, N. Y. (2019). Study on evaluation of landslide susceptibility in the middle reaches of Bailongjiang Basin, Master’s Thesis. Nanjing Normal University.
Wang, R., Zhang, K., Ning, Y., Xu, W., Wang, W., and Qin, J. (2021). Reactivation of ancient landslide deposits: Geological characteristics and deformation mechanism. Adv. Civ. Eng. 2021, 1–12. doi:10.1155/2021/6614180
Wang, S. B., Zhuang, J. Q., Zheng, J., Fan, H. Y., Kong, J. X., and Zhan, J. W. (2021). Application of Bayesian hyperparameter optimized random forest and XGBoost model for landslide susceptibility mapping. Front. Earth Sci. 617. doi:10.3389/feart.2021.712240
Wang, W. H. (2021). Landslide hazard identification based on SBAS-InSAR and machine learning -Taking Lanzhou City as an example, Master’s Thesis. Lanzhou Jiaotong University. doi:10.27205/d.cnki.gltec.2021.001122
Xu, Q., Dong, X. J., and Li, W. L. (2019). Integrated space-air-ground early detection, monitoring and warning system for potential catastrophic geohazards. Geomatics Inf. Sci. Wuhan Univ. 44 (07), 957–966. doi:10.13203/j.whugis20190088
Xu, Q., Lu, H. Y., Li, W. L., Dong, X. J., and Guo, C. (2022). Types of potential landslide and corresponding identification technologies. Geomatics Inf. Sci. Wuhan Univ. 47 (03), 377–387. doi:10.13203/j.whugis20210618
Yan, Y. Q., Guo, C. B., Zhang, Y. S., Zhang, X. J., Zheng, Y. Z., Li, X., et al. (2021). Study of the deformation characteristics of the Xiongba ancient landslide based on SBAS-InSAR method, Tibet, China. Acta Geol. Sin. 95 (11), 3556–3570. doi:10.19762/j.cnki.dizhixuebao.2021195
Yang, G. (2020). Optimized regional landslide susceptibility evaluation in Maoxian based on SBAS-InSAR technique, Master’s Thesis. Jilin University. doi:10.27162/d.cnki.gjlin.2020.005732
Yang, Z., and Qiao, J. (2009). “Entropy-based hazard degree assessment for typical landslides in the Three Gorges Area, China,” in Landslide dis. Mitigat. Three gor. Res, 519–529.
Yang, Z., and Qiao, J. (2010). “Regional landslide zonation based on entropy method in Three Gorges area, China,” in 2010 Seventh International Conference on Fuzzy Systems and Knowledge Discovery, 1336–1339.
Yhokha, A., Goswami, P. K., Chang, C. P., Yen, J. Y., Ching, K. E., and Aruche, K. M. (2018). Application of persistent scatterer interferometry (PSI) in monitoring slope movements in Nainital, Uttarakhand Lesser Himalaya, India. J. Earth Syst. Sci. 127 (1), 6–13. doi:10.1007/s12040-017-0907-y
Yu, L. B., Cao, Y., Zhou, C., Wang, Y., and Huo, Z. T. (2019). Landslide susceptibility mapping combining information gain ratio and support vector machines: A case study from wushan segment in the three Gorges reservoir area, China. Appl. Sci. (Basel). 9 (22), 4756. doi:10.3390/app9224756
Zhang, F. H., Zhu, Y. Y., Zhao, X. Z., Zhang, Y., Shi, L. H., and Liu, X. D. (2020). Spatial distribution and identification of hidden danger points of landslides based on geographical factors. Geomatics Inf. Sci. Wuhan Univ. 45 (08), 1233–1244. doi:10.13203/j.whugis20200126
Zhang, J., Gao, B., Huang, H., Chen, L., Li, Y., and Yang, D. (2022). SBAS-InSAR-based landslide susceptibility mapping along the north Lancang River, Tibetan Plateau. Front. Earth Sci. (Lausanne). 1169. doi:10.3389/feart.2022.901889
Zhao, F., Meng, X., Zhang, Y., Chen, G., Su, X., and Yue, D. (2019). Landslide susceptibility mapping of karakorum highway combined with the application of SBAS-InSAR technology. Sensors 19 (12), 2685. doi:10.3390/s19122685
Zhao, J., Liu, Y., and Hu, M. (2018). Optimisation algorithm for decision trees and the prediction of horizon displacement of landslides monitoring. J. Eng. 2018, 1698–1703. doi:10.1049/joe.2018.8305
Zhu, Q., Chen, L., Hu, H., Pirasteh, S., Li, H., and Xie, X. (2020). Unsupervised feature learning to improve transferability of landslide susceptibility representations. IEEE J. Sel. Top. Appl. Earth Obs. Remote Sens. 13, 3917–3930. doi:10.1109/jstars.2020.3006192
Zhu, Q., Zeng, H. W., Ding, Y. L., Xie, X., Liu, F., Zhang, L. G., et al. (2019). A review of major potential landslide hazards analysis. Acta Geod. Cartogr. Sinica 48 (12), 1551–1561.
Zhu, Q., Zhu, M. D., Ding, Y. L., Zeng, H. W., Wang, W., and Liu, F. (2021). Fuzzy logic approach for regional landslide susceptibility analysis constrained by spatial characteristics of environmental factors. Geomatics Inf. Sci. Wuhan Univ. 46 (10), 1431–1440.
Keywords: ancient landslide, reactivation risk, quantitative assessment, cross-time scale joint response mechanism, SBAS-InSAR, China
Citation: Yang Z, Wu T, E C, Xie X, Tan L and Jiang X (2023) A quantitative enhanced assessment for ancient landslide reactivation risk considering cross-time scale joint response mechanism. Front. Earth Sci. 10:974442. doi: 10.3389/feart.2022.974442
Received: 21 June 2022; Accepted: 12 September 2022;
Published: 05 January 2023.
Edited by:
Jianhong Xia, Curtin University, AustraliaCopyright © 2023 Yang, Wu, E, Xie, Tan and Jiang. This is an open-access article distributed under the terms of the Creative Commons Attribution License (CC BY). The use, distribution or reproduction in other forums is permitted, provided the original author(s) and the copyright owner(s) are credited and that the original publication in this journal is cited, in accordance with accepted academic practice. No use, distribution or reproduction is permitted which does not comply with these terms.
*Correspondence: Xiao Xie, eGlleGlhb0BpYWUuYWMuY24=
Disclaimer: All claims expressed in this article are solely those of the authors and do not necessarily represent those of their affiliated organizations, or those of the publisher, the editors and the reviewers. Any product that may be evaluated in this article or claim that may be made by its manufacturer is not guaranteed or endorsed by the publisher.
Research integrity at Frontiers
Learn more about the work of our research integrity team to safeguard the quality of each article we publish.