- Development Research Center of the Ministry of Water Resources of P.R. China, Beijing, China
How to formulate scientific and effective water-saving policies is of great significance for the sustainable water consumption. As a country with the largest water consumption in the world, China has been committed to formulating scientific and effective water-saving policies. However, due to geospatial variations of water consumption in different regions, how to formulate targeted and efficient water-saving policies which suit for different local characteristics has always been a key issue to be solved. In a quest to understand the water consumption patterns, this study first adopted a dual evaluation index system combining Gini coefficient and Global Moran’s Index to classify different types of water consumption. Taking 110 cities in the Yangtze River Economic Belt as research objects, the water consumption of 110 cities was divided into nine types. According to each type, a targeted water-saving policy is given. This study provides important theoretical support for the efficient management of water resources in the Yangtze River Economic Belt, and provides reference for water resources management in other regions at the same time.
Introduction
Research background
China is a country with severe drought and water shortage. China’s per capita water resources is only one quarter of the world average. It has been listed by the United Nations as one of the 13 countries with the most water stress. However, China is also a country with the largest water consumption in the world, accounting for about 13% of the world’s annual consumption (UN-water, 2018). China’s water resources situation is severe, therefore effective water resources management is of great significance to China’s sustainable development.
In response to the current problems of over-exploitation of water resources, China strictly controls the excessive growth of total water consumption. To address the current problems of over-exploitation of water resources, China proposed to limit its total water consumption to 700 billion cubic meters by 2030 (CPGPRC (The Central People’s Government of the People’s Republic of China), 2010). The situation of water resource consumption reduction is severe. It is important to carry out refined management of water resources and formulate appropriate policies to efficiently and effectively reduce water consumption.
The Yangtze River Economic Belt is one of China’s most developed regions with an area of about 2.05 million square kilometers. Its population and GDP are more than 40% of the total (EPMC (Environmental Protection Ministry of China), 2017). Working out effective policies to reduce water consumption in the Yangtze River Economic Belt is critical to China achieving its stated goal of controlling total water consumption to 700 billion cubic meters per year by 2030. In addition, the Yangtze River Economic Belt is an important water-out area in China’s South-to-North Water Diversion Project. The research on the patterns of water resource consumption in the Yangtze River Economic Belt is conducive to the formulation and implementation of more effective water-saving policies in this region, which is of great significance for ensuring China’s water security and improving the spatial equilibrium of water resource in China.
Literature review and research gap
In academia, water resources management has always been a research hotspot, and has received widespread attention from scholars worldwide (Bian et al., 2022a). According to the existing literature, research on water resources management mainly focuses on the following four aspects: 1) water resources development and protection, 2) optimal allocation of water resources, 3) watershed non-point source pollution control, and 4) water carrying capacity. Comparatively, there is relatively little research on water consumption. This is because research on water consumption requires a large amount of data on a small scale, which is often difficult to obtain. However, research on water consumption is very important and can provide important support for the development of more targeted water resources management policies.
When it comes to water consumption, there is a large degree of confusion between these concepts: water consumption, water use, water withdrawal and water demand. One has to be careful when using these concepts (Bian et al., 2022b). The contents of these concepts are slightly different. Water use refers to the actual amount of water resources used at a certain period of time or in a region or for a specific production activity. Water withdrawal generally refers to the amount of water extracted from a water source within a certain period of time or in an area for a specific production activity. Water demand refers to the amount of water resources required for a specific time or in a region for a specific production activity (Gleick, 2003).
Water consumption has two separated meanings: 1) The relatively narrow understanding, which refers to the amount of water resources that evaporate and infiltrate in an irreparable loss. 2) The widely understood concept of water consumption which refers to the actual water usage at a certain period of time for a specific production activity. The concept of water consumption used in this study refers to the later one.
In order to find out the factors that affect water consumption, some researches in recent years have focused on the driving factors of water consumption. Jaramillo and Destouni (2015) studied the relationship between the extensive use of dam construction and irrigation facilities and the consumption of water resources based on the hydrological and climatic observations of 100 large hydrological basins around the world. Compared with the years from 1901 to 1954, the per capita consumption of fresh water resources increased significantly from 1955 to 2008. Long et al. (2019) used the logarithmic mean Divisia index to decompose the driving factors of water consumption in China’s provinces from 2000 to 2015. The results are as follows: 1) In the agricultural field, technological progress and population mobility are the main reasons for the decline in water consumption. 2) In the industrial sector, advances in industrial technology have contributed to the decline in water consumption. 3) In the residential sector, technological advances in household appliances have contributed to the decline in water consumption. At the same time, per capita income is a major factor that affects water consumption. Studies have also shown that water consumption is strongly related to the level of economic development (Fercovic et al., 2019). Sauri (2019) has studied the driving factors of the significant decline in water consumption in some major Spanish cities between 2003 and 2016. The study believes that the driving factors that caused the decline in water consumption are multifaceted. The study also discussed the different degrees of economic, technological, and behavioral aspects driving the decline in water consumption.
Another research area of water consumption is water use efficiency. For example, Hu et al. (2006) applied data envelopment analysis to study the efficiency of water resources use in China from 1997 to 2002, taking the provincial level as the research scale. They found that water efficiency in central areas of China is the worst. In central China, more efficient production processes and advanced technologies are needed to improve water efficiency. Deng et al. (2016) evaluated water efficiency of 31 provinces in China from 2004 to 2013 by using slack based measure-data development analysis. Wang et al. (2015) studied the relationship between the water efficiency of China’s regional industrial systems and the emission reduction costs of related pollutants based on the relaxation variable method.
Other studies have focused on two parts. On one hand, water consumption research is concentrated in some special industries, such as water consumption in aquaculture (Lebel et al., 2019), rice planting (Silalertruksa et al., 2017), and power generation (Chang et al., 2015). On the other hand, water consumption research focuses on water consumption and other related fields, such as virtual water (Zhang et al., 2019), water use efficiency (Freire-González, 2019), sewage treatment (Risch et al., 2014), water footprints (Hoekstra and Chapagain, 2006; Boulay et al., 2018), spatial imbalance (Cole et al., 2018), and other studies.
However, research on water consumption is very limited. The only few studies on water consumption focused on households and studied the water consumption in various parts of the household (such as showers, toilets, washing machines, and garden irrigation). Keshavarzi et al. (2006) collected water consumption from 653 households in 33 villages from 1999 to 2004 through a questionnaire survey. They found that household water consumption was significantly related to the number of households and the age of the head of household. The study also found that in rural households, water consumption is related to the area of the garden and the greenhouse, and the frequency of watering. Jethoo and Poonia (2011) used a questionnaire method to investigate the relationship between household water consumption and household income in Jaipur City, India. The study found that these two aspects are highly correlated. Willis et al. (2013) used a combination of both actual monitoring and questionnaires to study the different performances of water consumption in households located in different socioeconomic states and those with different number of users of facilities such as the kitchen, toilet, washing machine, shower etc., and gave out a number of water conservation recommendations.
Even though, research on water consumption is very limited, based on the analysis of the existing literature above, we find that research on water consumption has attracted more attention in recent years. Furthermore, research on water consumption is mostly focused on the study of driving factors, water efficiency, water consumption in some special industries, water consumption and other research in related fields, and very little research on the patterns of water consumption. From the view of research scale and data acquisition methods, the research on water consumption driving factors and water efficiency are mainly concentrated on the provincial scale or industry scale. The data is generally obtained through national statistical yearbooks, while for research on water consumption patterns concentrated on the household scale, the data is obtained by questionnaires or combined with actual monitoring.
However, research on water consumption at the provincial and industry scales is generally difficult to formulate water resources management policies for different regions due to the large research scale. The research on water consumption on a household scale does not take into account other aspects such as agricultural water use, industrial water use, and eco-environment water use. It also has no reference value when formulating water management policies for different regions. Therefore, because studying the consumption of water resources at the city scale takes into account all the water consumption channels in the city, it can provide a reliable basis for effective water resources management, help to formulate targeted policies, and be more targeted, thus can reduce water consumption.
Because the characteristics of water consumption in different cities vary, it is very necessary to figure out the key differences in water consumption in different cities and clarify the water consumption patterns, so that more effective localized policies can be formulated and implemented. When studying water consumption patterns in different cities, it is necessary to take the differences in water consumption in different areas of the city into account, in order to formulate water resources management policies for the main water consumption areas. And in order to formulate water resources management policies with different regional characteristic, it is also necessary to take full account of the spatial distribution characteristics of regional water consumption. In other words, both the quantitative and spatial differences in water consumption in different areas of the city should be fully considered.
The main work and innovations
This study evaluates the water consumption patterns of 110 cities in the Yangtze River Economic Belt and compares the difference in the amount of water resources as well as the spatial variations in water use within the cities. We used Gini coefficient to characterize the amount of water resources in different areas of the city and Global Moran’s I (index) to characterize the regional differences in water consumption in different regions of the city. Based on the calculation results of Gini coefficient and Global Moran’s I, the water consumption patterns of 110 cities in the Yangtze River Economic Belt are divided. For different types, more specific water resource management recommendations are given, which will provide important support for minimizing water consumption in the Yangtze River Economic Belt. At the same time, this study can provide reference for water resources management and control in other regions.
Materials and methods
Study area
The research area in this study is the Yangtze River Economic Belt. The Yangtze River Economic Belt is one of the most developed regions in China. It is a strategic water source for China’s water resources allocation, an important strategic base for clean energy, and a natural treasure house of rare aquatic life (EPMC (Environmental Protection Ministry of China), 2017). It has a very important strategic position in environmental protection. The Yangtze River Economic Belt covers 11 provincial-level administrative regions including Shanghai, Jiangsu, Zhejiang, Anhui, Jiangxi, Hubei, Hunan, Chongqing, Sichuan, Yunnan and Guizhou, including the Yangtze River Delta urban agglomeration, urban agglomeration in the middle reaches of the Yangtze River, and the Chengdu-Chongqing urban agglomeration. The eastern region of the Yangtze River Economic Belt includes Shanghai, Jiangsu, and Zhejiang. It is the core component of the Yangtze River Delta and the most economically developed region in the Yangtze River Economic Belt. The central region includes Hubei, Hunan, Jiangxi, and Anhui. The region has abundant natural resources and convenient transportation. The central region has obvious advantages in grain production, strong industrial foundation, and good conditions for accelerating economic and social development. Western region includes Chongqing, Sichuan, Yunnan, Guizhou, and is rich in hydropower, minerals, and biological resource. The Yangtze River Economic Belt plays an extremely important role in China’s economic and social development and ecological environment protection.
The Yangtze River Economic Belt covers 11 provincial-level administrative regions including 130 prefecture level cities. Considering the accuracy of Global Moran’s I, a city needs to have at least 4 second-tier cities. In this study, we chose 110 of 130 cities in the Yangtze River Economic Belt as research objects as presented in Table 1.
Data collection
Data on water resources planning and management normally comes from Statistical Yearbooks or Water Resources Bulletins. However, the data in the Statistical Yearbook and Water Resources Bulletin is on city scale, but in order to formulate more targeted and localized policies, smaller level, like sub-cities level data has been required. But this data is usually not available in Statistical Yearbooks and Water Resources Bulletins. Data in the study is the water consumption data of all sub-cities of the 110 cities in the Yangtze River Economic Belt in 2015. This data has been obtained by an investigation among the water resources planning and management department of the local government of these 110 cities. After we obtained the water consumption data of all the sub-cities of the 110 cities in the Yangtze River Economic Belt in 2015, we obtained water consumption data of the 110 cities at the same time. And then, a comparison between water consumption data of the 110 cities in 2015 that we obtained and water consumption data of these 110 cities that in the Water Resources Bulletin have been carried out. If they are not equal, we reinvestigated. The amount of water collected included agricultural water, industrial water, domestic water, and ecological water.
Patterns classification of water consumption by adopting two statistics
In order to achieve better management of water resources, the common way is to evaluate water carrying capacity or water efficiency. The core of water resources carrying capacity evaluation is to find the population or economic scale of the largest amount of water that can be carried by the water resources in a certain area. The core of water efficiency is to find the way to obtain the maximum output with the least water resources input. When evaluate water resources carrying capacity, both the consumption and the supply of water resources have been taken into consideration comprehensively. While assess water efficiency, the relationship between the consumption of water resources and the output (generally economic benefits) has been comprehensively considered.
Both water carry capacity and water efficiency provide a solid foundation and theoretical basis for water resources management. The research focus on water resources carrying capacity is to pay attention to whether the overall state of the city exceeds the threshold that the regional water resources can bear. Although the unevenness of the spatial distribution of water resources has been considered by water carrying capacity evaluation, the quantitative dimensions have not been researched. Water efficiency focuses on the relationship between water consumption and the output (generally refers to economic benefits). It takes the quantitative unevenness of water consumption into account without considering the spatial imbalance of water consumption. Neither water carrying capacity nor water efficiency has taken full account of water consumption patterns. However, water consumption patterns in different regions are not the same. Only by fully researching water consumption patterns in different regions, can more effective comprehensive water resources management and control strategies be formulated.
Therefore, we adopt the method proposed by Liu et al. (2019) when studying the carbon dioxide emission patterns. Two indicators have been selected in their research. The two indicators are Gini coefficient and Global Moran’s I. Gini coefficient is used to represent the unevenness in quantity; Global Moran’s I is used to represent the special unevenness. Based on these two indicators, it is possible to study the water consumption patterns of 110 cities in the Yangtze River Economic Belt. By classifying different urban water consumption patterns, targeted measures are given for each category.
Gini coefficient
Gini coefficient is a term of economics, which refers to a common indicator used internationally to measure the income gap of residents in a country or region. The maximum Gini coefficient is “1” and the minimum is equal to “0”. In economics: the closer Gini coefficient is to 0, the more equal the wealth distribution is; when Gini coefficient is larger, the gap between the rich and the poor is larger. Gini coefficient has been introduced in other research fields, such as carbon dioxide emissions, water resources and other fields, to study the quantitative distribution characteristics. The larger Gini coefficient, the more uneven the distribution, and vice versa. We also use water consumption of sub-cities to fit the Lorentz curve, assuming that the area enclosed by the Lorentz curve and the two axes is SB. And assuming that the area enclosed by diagonal and the two axes is SA + B. Since the range of the coordinate axis is 0–1, the area of SA + B is 0.5. Gini coefficient is defined as (SA + B–SB)/SA + B. In this study, it is assumed that the Lorentz curve satisfies LC = αPβ. Then, regression analysis is performed through water consumption data to obtain the values of α and β. Suppose the values of α and β are x and y, respectively. Then, SB can be calculated by the following formula:
Gini coefficient can then be expressed as:
From the formula above, Gini coefficient of water consumption in each city is calculated by a python program. In general, the larger Gini coefficient, the more polarized the quantity of water consumption.
Global Moran’s I
Global Moran’s I is an index that reflects spatial autocorrelation, and it has been widely used. Global Moran’s I is a maximum of “1” and a minimum of “−1”. If Global Moran’s I is greater than 0, indicating a positive spatial correlation. The larger the value is, the more obvious the spatial correlation. When Global Moran’s I is less than 0, it indicates a negative spatial correlation. And the smaller the value is, the greater the spatial difference is. If Global Moran’s I is equal to 0, it means that the spatial distribution of data is random. Global Moran’s I has been calculated by spatial autocorrelation analysis in the ArcGis software toolbox.
The method for classification
In order to classify water consumption patterns of 110 cities in the Yangtze River Economic Belt, both Gini coefficient and Global Moran’s I of the water consumption of 110 cities in the Yangtze River Economic Belt have been calculated, by adopting Natural Breaks, Gini coefficient and Global Moran’s I has been divided into 3°. Then, based on a two-dimensional Cartesian coordinate system, we have obtained nine types of water consumption patterns (see Figure 1). Natural Breaks is a grading and classification method according to the statistical distribution of numerical statistics. It can maximize the difference between classes. It is worth noting that among these 9 types, there is none that is better than the others.
Results and discussion
Water consumption of 110 cities in the Yangtze River Economic Belt in 2015
Figure 2 shows the location of the three major urban agglomerations of the Yangtze River Economic Belt and the water consumption of the 877 sub-cities in the Yangtze River Economic Belt in 2015. It can be seen that water consumption is related to the distribution of urban agglomerations.
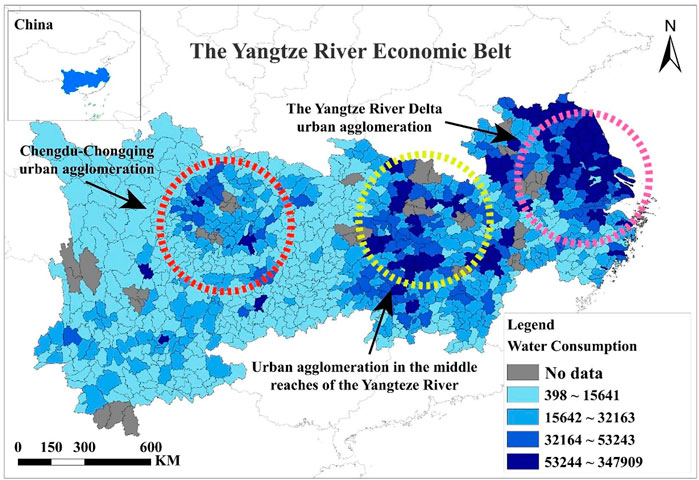
FIGURE 2. Water consumption of sub-cities in the Yangtze River Economic Belt (2015). Unit: 10,000 cubic meters.
Figure 3 shows the water consumption of 110 cities in the Yangtze River Economic Belt in 2015.
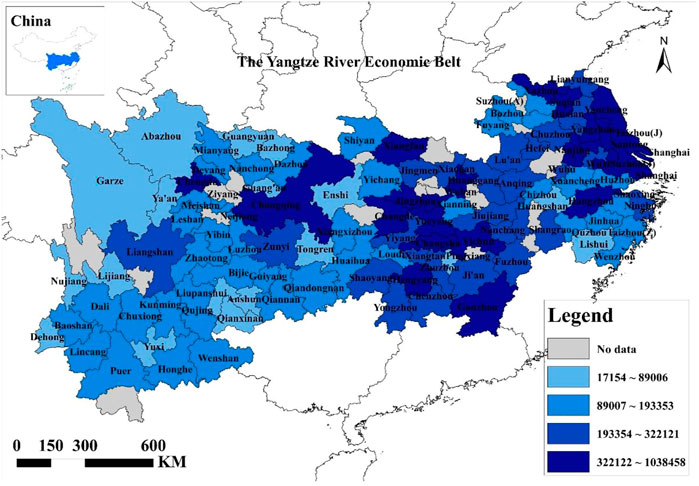
FIGURE 3. Consumption of water resources at the city scale in the Yangtze River Economic Belt (2015) Unit: 10,000 cubic meters.
The quantitative pattern of water consumption
The calculation results of Gini coefficients of 110 cities in the Yangtze River Economic Belt are shown in Figure 4. On the whole, Gini coefficients of 110 cities are almost all less than 0.5. From this result, we can draw two conclusions. Firstly, because Gini coefficient varies from 0 to 1, and Gini coefficients of 110 cities are almost in the range of 0–0.5, indicating that Gini coefficients of water consumption in 110 cities are not much different. Secondly, it can be seen that the water consumption of each region in each of the 110 cities does not vary greatly in quantity, and there is no one area or several areas that consume the entire city plays a decisive role, so we cannot reduce the overall water consumption of the city by controlling the water consumption of certain areas in the city.
Although Gini coefficients of the 110 cities are not much different, Gini coefficients of water consumption in different cities still have the following differences. For example, Gini coefficient of water consumption in Liangshan is the largest, slightly exceeding 0.5, and is 0.5082, indicating that the water consumption in various areas within the city of Liangshan has the largest difference in quantity. In 110 cities, with the exception of Liangshan, Gini coefficient of water consumption in other cities are all less than 0.5. The top 10 cities with the highest Gini coefficients are Liangshan, Leshan, Zhenjiang, Nujiang, Wuhan, Xiangyang, Yichun, Shanghai, and Chizhou. For the two municipalities Shanghai and Chongqing, Gini coefficients of water consumption are 0.4545 and 0.3420 respectively. Gini coefficients of water consumption of 13 cities in Hunan Province are almost in the range of 0.1–0.3.
From the perspective of the spatial distribution of water consumption in 110 cities, the regions with severely polarized water consumption in 110 cities in the Yangtze River Economic Belt are concentrated in the east and west of the Yangtze River Economic Belt, as shown in Figure 5. The highly polarized areas are almost all concentrated within the three major urban agglomerations of the Yangtze River Economic Belt (the locations of the three major urban agglomerations in the Yangtze River Economic Belt are shown in Figure 2). Such distribution characteristics may be one of the effects of the implementation of the Yangtze River Economic Belt strategy by the Chinese central government in 2014. In addition, it can be seen from Figure 5 and Figure 2 that the polarization phenomenon and water consumption have a great correlation with the location of the three major urban agglomerations in the Yangtze River Economic Belt. In 2018, General Secretary of China Xi Jinping pointed out, “the Yangtze River has a unique ecosystem and is an important ecological treasure trove of our country. For a long period of time now and in the future, we must put the restoration of the Yangtze River ecological environment in an overwhelming position.” This shows the Chinese government’s determination to improve the restoration of the ecological environment of the Yangtze River.
The spatial pattern of water consumption
Judging from the results of Global Moran’s I on the water consumption of 110 cities in the Yangtze River Economic Belt, Global Moran’s I is between 0–1 and 0–1. The city with the largest Global Moran’s I is Yichang, it is 0.6443; the second is Ji’an, it is 0.6205; and the third is Lishui, it is 0.6028. There are 44 cities with a positive Global Moran’s I. The larger Global Moran’s I indicates that the city’s water consumption is agglomerated the city’s water consumption. In other words, as the spatial distribution of the location is gathered, the correlation of water consumption is more significant. There are 66 cities with negative Global Moran’s I, the smallest are Dazhou, Dehong, Jingmen, and their Global Moran’s I are −0.969401, −0.96612, −0.91164, which are all close to −1.0, indicating that the water consumption situation shows a discrete phenomenon meaning as the spatial distribution location is discrete, the correlation of water consumption becomes significant. Because Global Moran’s I of 110 cities in the Yangtze River Economic Belt is distributed in the range of 0–1 and 0–1, we can draw the conclusion that the water consumption of these 110 cities in terms of water consumption spatial autocorrelation is different, but this different trend of spatial autocorrelation is temporarily uncertain (Figure 6). To determine this trend, one needs to obtain water consumption data on the sub-cities scale of these 110 cities over a long period of time.
The spatial distribution of Global Moran’s I of water consumption in 110 cities in the Yangtze River Economic Belt is shown in Figure 7. As can be seen from Figure 7, the regions with high, medium, and low global Moran indices are scattered. The areas with high Global Moran’s I and low areas are distributed in the east, middle and west of the Yangtze River Economic Belt, while the areas with medium Global Moran’s I are mainly distributed in the central and western regions of the Yangtze River Economic Belt. The distribution of Global Moran’s I is not significantly related to the distribution of the three major urban agglomerations in the Yangtze River Economic Belt.
City type and policy implications
Based on the classification method given in Part 2, we divided Gini coefficient and Global Moran’s I of the 110 cities in the Yangtze River Economic Belt into three levels by adopting Natural Breaks. Then, in the two-dimensional Cartesian coordinate system composed of Gini coefficient and Global Moran’s I, water consumption patterns of 110 cities in the Yangtze River Economic Belt are divided into nine types, and the classification results are shown in Figure 8. In the image below, each rectangle represents one type. Each pie shape in Figure 8 represents a city, and the colors of the different pie shapes represent the provinces to which the city belongs. The names of the provinces corresponding to each color are shown in Figure 8.
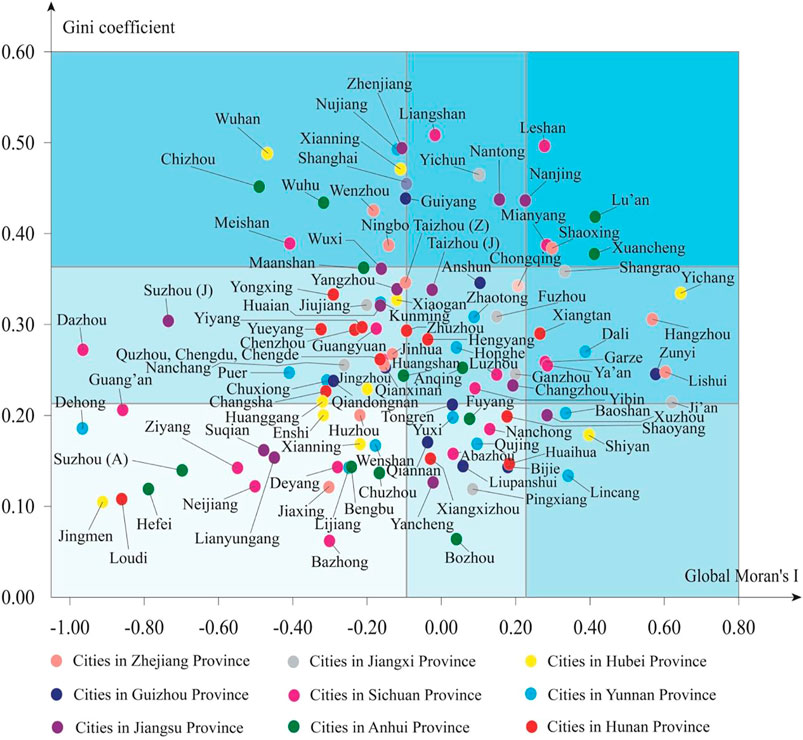
FIGURE 8. Classification of 110 cities in the Yangtze River Economic Belt based on water consumption in 2015.
As can be seen from Figure 8, each type contains at least one city. Cities tend to be located in the middle of the rectangular coordinate system. The distribution of 110 cities in the Yangtze River Economic Belt in nine types is shown in Table 2. 11 cities belong to type I, four cities belong to type II, five cities belong to type III, and there are 29 cities belong to type IV. Twelve cities belong to type V, 10 cities belong to type VI, 20 cities belong to type VII, 15 cities belong to type VIII, and four cities belonging to type IX.
It is important to note that when formulating effective policies to reduce water consumption according to different types of consumption patterns, the following principles need to be followed:
1) When Gini coefficient of the city’s water consumption is high, it indicates that the polarization of water consumption in the city is more obvious. In order to effectively reduce water consumption, effective water saving policies needs to be formulated for large water users.
2) When Gini coefficient of the city’s water consumption is low, it indicates that the polarization of water consumption in the city is not obvious. To effectively reduce water consumption, it is necessary to formulate effective water saving policies for all water users.
3) When the city’s water consumption is in a medium Gini coefficient, it indicates that the polarization of water consumption in the city is between obvious and inconspicuous. In order to effectively reduce water consumption, develop effective water-saving policies for major water users (broader users than large water users).
4) When Global Moran’s I of the city’s water consumption is at a high level, it indicates that the city’s water consumption has a significant autocorrelation in space meaning, with the accumulation of spatial distribution locations of the water consumption, the correlation becomes more significant. Therefore, in order to effectively reduce the consumption of water resources, effective water conservation policies should be formulated in several key positions as a priority.
5) When Global Moran’s I of the city’s water consumption is low, it indicates that the city’s water consumption is in a discrete form in space, that is, as the spatial distribution location is discrete, the correlation of water consumption is more significant. In order to effectively reduce the consumption of water resources, priority should be given to effective water conservation policies in some regions.
6) When Global Moran’s I of the city’s water consumption is in a medium state, it indicates that the city’s water consumption is randomly distributed in space. Therefore, in order to effectively reduce water consumption, it should be formulated in all regions. This is targeted water-saving policy.
Based on the principles above, we provide policies that can effectively reduce water consumption for nine different urban water consumption modes, as shown in Table 2. For example, in the cities of type I, water consumption is the most polarized in terms of quantity and the spatial autocorrelation is the worst. Therefore, in order to reduce water consumption to the greatest extent, it is necessary to formulate water conservation for large water users in all regions. When a city is located in type II, water consumption is highly polarized in quantity, and the spatial autocorrelation is in a medium state. It is necessary to formulate water-saving policies for large water users in some areas.
From the perspective of the provinces, the cities of Hunan Province are mainly distributed in Type IV, indicating that the polarization of water consumption in various cities in Hunan Province is at a medium level. Cities need to prioritize water conservation policies for key water users in all regions. However, the distribution of cities in other provinces in the two-dimensional rectangular coordinate system does not show obvious distribution rules.
Conclusion
Water consumption patterns of different regions are not the same. Only by fully researching water consumption patterns in different regions can more effective comprehensive water resources management and control strategies be formulated. According to the characteristics of different regions, it is necessary to formulate different and more effective water—saving policies.
Consequently, this study introduced Gini coefficient and Global Moran’s I, and divided each index into three levels by adopting Natural Breaks. In this way, all cities in the two-dimensional Cartesian coordinate system composed of Gini coefficient and Global Moran’s I. The water consumption patterns can be divided into nine types. On the one hand, from the perspective of Gini coefficient: cities with high Gini coefficients indicate that there is a significant polarization in the amount of water consumed. Therefore, by effectively managing large water users, it is possible to achieve overall reductions in water consumption. For cities with lower Gini coefficients, it means that the water consumption in those cities is less quantitative in quantity, because in order to effectively reduce water consumption, it is necessary to formulate water conservation policies for all water users. On the other hand, from the perspective of Global Moran’s I: cities with a high Global Moran’s I indicate that there is a clear spatial autocorrelation phenomenon in water consumption, and therefore effective water conservation policies need to be formulated in several key locations and for cities where Global Moran’s I is low, it shows that the city’s water consumption is spatially discrete. In order to effectively reduce water consumption, priority should be given to effective water conservation policies in some areas. This study uses the above methods to divide the water consumption patterns of 110 cities in the Yangtze River Economic Belt into nine types in 2015. Based on the above principles, targeted water conservation is given to cities that belong to each type of water consumption pattern.
At the same time, through the visualization of nine types of water consumption patterns of 110 cities in the Yangtze River Economic Belt, it was found that 110 cities belonged to the largest number of types IV, VII, and VIII. A large number of cities are located in the lower left corner of the two-dimensional Cartesian coordinate system, indicating that these cities belong to a state where the polarization phenomenon is not obvious and the spatial distribution is discrete. For this type of city, it is necessary to formulate sections for all water users in all regions.
This study provides important technical and theoretical support for effectively reducing water consumption in cities in the Yangtze River Economic Belt. At the same time, this research can provide reference significance for the formulation of policies on water consumption in other regions or cities. It is hoped that the methods used in this research can be widely applied in future research.
Data availability statement
The original contributions presented in the study are included in the article/supplementary material, further inquiries can be directed to the corresponding author.
Author contributions
BS contributed to conception and design of the study, analysis and interpretation of data, drafting the manuscript. FL wrote sections of the manuscript, acquisition of data. All authors contributed to manuscript revision, read, and approved the submitted version.
Conflict of interest
The authors declare that the research was conducted in the absence of any commercial or financial relationships that could be construed as a potential conflict of interest.
Publisher’s note
All claims expressed in this article are solely those of the authors and do not necessarily represent those of their affiliated organizations, or those of the publisher, the editors and the reviewers. Any product that may be evaluated in this article, or claim that may be made by its manufacturer, is not guaranteed or endorsed by the publisher.
References
Bian, D., Yang, X., Wu, F., Babuna, P., Luo, Y., Wang, B., et al. (2022a). A three-stage hybrid model investigating regional evaluation, pattern analysis and obstruction factor analysis for water resource spatial equilibrium in China. J. Clean. Prod. 331, 129940. doi:10.1016/j.jclepro.2021.129940
Bian, D., Yang, X., Xiang, W., Sun, B., Chen, Y., Babuna, P., et al. (2022b). A new model to evaluate water resource spatial equilibrium based on the game theory coupling weight method and the coupling coordination degree. J. Clean. Prod. 366, 132907. doi:10.1016/j.jclepro.2022.132907
Boulay, A., Bare, J., Benini, L., Berger, M., Lathuillière, M. J., Manzardo, A., et al. (2018). The WULCA consensus characterization model for water scarcity footprints: assessing impacts of water consumption based on available water remaining (AWARE). Int. J. Life Cycle Assess. 23 (2), 368–378. doi:10.1007/s11367-017-1333-8
Chang, Y., Huang, R., Ries, R. J., and Masanet, E. (2015). Life-cycle comparison of greenhouse gas emissions and water consumption for coal and shale gas fired power generation in China. Energy 86, 335–343. doi:10.1016/j.energy.2015.04.034
Cole, M. J., Bailey, R. M., Cullis, J. D. S., and New, M. G. (2018). Spatial inequality in water access and water use in South Africa. Water Policy 20 (1), 37–52. doi:10.2166/wp.2017.111
CPGPRC (The Central People’s Government of the People's Republic of China) (2010). National comprehensive water resources plan. [in Chinese] Available at: http://www.gov.cn/gzdt/2010-11/25/content_1753339.htm (Accessed December 25, 2010).
Deng, G., Li, L., and Song, Y. (2016). Provincial water use efficiency measurement and factor analysis in China: based on SBM-DEA model. Ecol. Indic. 69, 12–18. doi:10.1016/j.ecolind.2016.03.052
EPMC (Environmental Protection Ministry of China) (2017). Ecological environment protection plan of the Yangtze river economic belt. [in Chinese]Available at: http://www.mee.gov.cn/gkml/hbb/bwj/201707/t20170718_418053.htm (Accessed July 17, 2017).
Fercovic, J., Foster, W., and Melo, O. (2019). Economic development and residential water consumption in Chile. Environ. Dev. Econ. 24 (1), 23–46. doi:10.1017/s1355770x18000463
Freire-González, J. (2019). Does water efficiency reduce water consumption? The economy-wide water rebound effect. Water Resour. Manage. 33 (6), 2191–2202. doi:10.1007/s11269-019-02249-0
Gleick, P. H. (2003). Water use. Annu. Rev. Environ. Resour. 28 (1), 275–314. doi:10.1146/annurev.energy.28.040202.122849
Hoekstra, A. Y., and Chapagain, A. K. (2006). Water footprints of nations: water use by people as a function of their consumption pattern. Water Resour. Manage. 21 (1), 35–48. doi:10.1007/s11269-006-9039-x
Hu, J., Wang, S., and Yeh, F. (2006). Total-factor water efficiency of regions in China. Resour. Policy 31 (4), 217–230. doi:10.1016/j.resourpol.2007.02.001
Jaramillo, F., and Destouni, G. (2015). Local flow regulation and irrigation raise global human water consumption and footprint. Science 350 (6265), 1248–1251. doi:10.1126/science.aad1010
Jethoo, A. S., and Poonia, M. P. (2011). Water consumption pattern of Jaipur city (India). Int. J. Env. Sci. Dev. 2 (2), 152–155. doi:10.7763/ijesd.2011.v2.114
Keshavarzi, A. R., Sharifzadeh, M., Kamgar Haghighi, A. A., Amin, S., Keshtkar, S., and Bamdad, A. (2006). Rural domestic water consumption behavior: a case study in ramjerd area, fars province, I.R. Iran. Water Res. 40 (6), 1173–1178. doi:10.1016/j.watres.2006.01.021
Lebel, L., Lebel, P., and Chuah, C. J. (2019). Governance of aquaculture water use. Int. J. Water Resour. Dev. 35 (4), 659–681. doi:10.1080/07900627.2018.1457513
Liu, H., Nie, J., Cai, B., Cao, L., and Wang, X. (2019). CO2 emissions patterns of 26 cities in the Yangtze River Delta in 2015: Evidence and implications. Environ. Pollut. 252, 1678–1686. doi:10.1016/j.envpol.2019.06.102
Long, H., Lin, B., Ou, Y., and Chen, Q. (2019). Spatio-temporal analysis of driving factors of water resources consumption in China. Sci. Total Environ. 690, 1321–1330. doi:10.1016/j.scitotenv.2019.06.311
Risch, E., Loubet, P., Núñez, M., and Roux, P. (2014). How environmentally significant is water consumption during wastewater treatment?: application of recent developments in LCA to WWT technologies used at 3 contrasted geographical locations. Water Res. 57, 20–30. doi:10.1016/j.watres.2014.03.023
Sauri, D. (2019). The decline of water consumption in Spanish cities: structural and contingent factors. Int. J. Water Resour. D. 36, 909–925. doi:10.1080/07900627.2019.1634999
Silalertruksa, T., Gheewala, S., Mungkung, R., Nilsalab, P., Lecksiwilai, N., and Sawaengsak, W. (2017). Implications of water use and water scarcity footprint for sustainable rice cultivation. Sustainability 9 (12), 2283. doi:10.3390/su9122283
UN-water (2018). World water development report 2018. Available at: https://www.unwater.org/publications/world-water-development-report-2018/(Accessed March 19, 2018).
Wang, Y., Bian, Y., and Xu, H. (2015). Water use efficiency and related pollutants' abatement costs of regional industrial systems in China: a slacks-based measure approach. J. Clean. Prod. 101, 301–310. doi:10.1016/j.jclepro.2015.03.092
Willis, R. M., Stewart, R. A., Giurco, D. P., Talebpour, M. R., and Mousavinejad, A. (2013). End use water consumption in households: impact of socio-demographic factors and efficient devices. J. Clean. Prod. 60, 107–115. doi:10.1016/j.jclepro.2011.08.006
Keywords: water consumption, Yangtze River Economic Belt, Global Moran I, Gini coefficient, water resources management
Citation: Sun B and Li F (2022) Water consumption patterns of 110 cities in the Yangtze River Economic Belt in 2015. Front. Earth Sci. 10:969991. doi: 10.3389/feart.2022.969991
Received: 15 June 2022; Accepted: 29 July 2022;
Published: 24 August 2022.
Edited by:
Chunhui Li, Beijing Normal University, ChinaReviewed by:
Liu Liu, China Agricultural University, ChinaXingcai Liu, Institute of Geographic Sciences and Natural Resources Research (CAS), China
Copyright © 2022 Sun and Li. This is an open-access article distributed under the terms of the Creative Commons Attribution License (CC BY). The use, distribution or reproduction in other forums is permitted, provided the original author(s) and the copyright owner(s) are credited and that the original publication in this journal is cited, in accordance with accepted academic practice. No use, distribution or reproduction is permitted which does not comply with these terms.
*Correspondence: Boyang Sun, sunboyang@aliyun.com