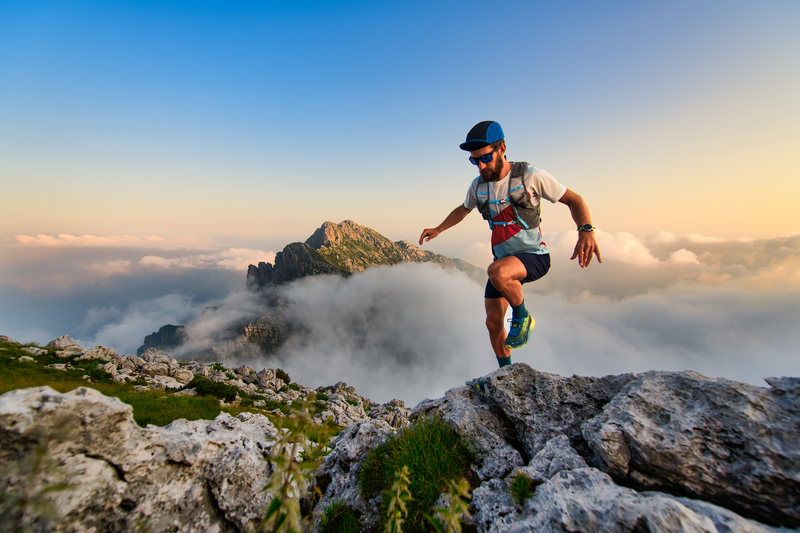
95% of researchers rate our articles as excellent or good
Learn more about the work of our research integrity team to safeguard the quality of each article we publish.
Find out more
EDITORIAL article
Front. Earth Sci. , 22 July 2022
Sec. Geohazards and Georisks
Volume 10 - 2022 | https://doi.org/10.3389/feart.2022.964753
This article is part of the Research Topic Application of Remote Sensing and GIS in Earthquake-Triggered Landslides View all 14 articles
Editorial on the Research Topic
Application of remote sensing and GIS in earthquake-triggered landslides
Earthquakes have long been recognized as one of the main triggers for landslides across the Earth (Keefer 1984; Tian et al., 2022). The earthquakes we experienced in the last few decades (e.g., the 1994 Mw6.7 Northridge, United States, the 2008 Mw7.9 Wenchuan, China, the 2015 Mw7.8 Gorkha, Nepal, and the 2018 Mw6.6 Hokkaido, Japan, earthquakes) showed that moderate and large earthquakes in mountainous terrain, can produce clusters of several hundred to thousands of landslides in a very short period. In turn, the earthquake-triggered landslides threaten our society by their direct and indirect, long-term effects such as damaged infrastructure, increased debris flows and floods associated with landslide dam failures and downstream river aggradations. As a result, the investigations of earthquake-triggered landslides have received much attention in recent years, due to their probable results of the tragic loss of life and economic devastation. With the rapid development of GIS and remote sensing technologies, valuable progress has been made in the earthquake-triggered landslides research field. The ability and efficiency of extracting large-scale and massive earthquake-triggered landslide data have been greatly improved. The number and quality of earthquake-triggered landslide records are increasing. The susceptibility, hazard, and risk assessment models of earthquake-triggered landslides are becoming more advanced and precise, and the ability to process large-scale and high-precision data is more robust. Remote sensing and GIS technologies have greatly improved the ability of information acquisition and big data analysis of earthquake-triggered landslides. Furthermore, remote sensing and GIS technologies have significantly promoted the progress of identification, mapping, monitoring, early warning, and risk evaluation of earthquake-triggered landslides.
To present the latest achievements in this direction, we organized a Research Topic entitled Application of remote sensing and GIS in earthquake-triggered landslides. This Research Topic includes 13 papers covering regional earthquake-triggered landslide mapping and spatial distribution analysis, monitoring and analysis of large-scale individual landslides, landslide susceptibility and hazard assessment, landslide evolution, and changes in rainfall thresholds of debris flow in earthquake-affected areas, etc. Remote sensing and GIS technologies have played an important role in these studies.
Landslide inventory maps are an essential basis for related research and have achieved rapid development in recent years (Harp et al., 2011; Xu 2015), mainly due to the capability to identify landslides based on high-resolution remote sensing images and to manage and analyze massive landslides based on GIS platform. In this Research Topic, there are three papers in this field. Martinez et al. made an inventory map including 43 coseismic classified landslides triggered by the 2018 Anchorage Mw7.1 earthquake in Alaska, United States, based on comprehensive methods such as field investigations, multi-spectral optical satellite images, LiDAR data, and SAR data. They compared the performance of these techniques, and the results show that the comparison of NDVI data derived from optical satellite images pre- and post-quake, and LiDAR data, are more suitable for identifying soil slumps and rapid soil flows. However, those landslides with small surface deformation can only be detected through field investigations. This study provides a reference for the selection of coseismic landslide mapping methods in subarctic and urban areas.
Building an inventory map of ancient landslides and establishing their connection with historical earthquakes are other relevant works. The old landslide distribution database is one of the critical issues. In this Research Topic, Peng et al. identified 6,876 large-scale active and old landslides in the Southwest of Ordos, China, based on the satellite images from the Google Earth platform. They analyzed the spatial distribution characteristics of these landslides and explored their relationship with a historical earthquake, the 780 BC Qishan earthquake. This work provides an interesting case study of the relationship between ancient landslides and historical earthquakes.
Although the remote sensing image-based visual interpretation method is currently the most crucial approach for establishing landslide inventory maps, the automatic extraction technology of landslides based on remote sensing images has also received extensive attention. In this Research Topic, Comert took the Mw6.7 earthquake in Hokkaido, Japan, on 6 September 2018, as an example and used the random forest method to carry out an experimental study on automatic identification of coseismic landslides and studied the influence of five different sizes of training samples on the results of automatic landslide identification. The datasets include pre- and post-quake differential images and only post-quake images. The results show that the latter had better performance, and the increase in the number of training samples will slightly improve the result accuracy. This work provides a reference for the preprocessing of data and the selection of sample size to automatically identify landslides based on remote sensing data.
GIS has dramatically promoted the spatial distribution analysis of massive landslides. Taking the Mw7.8 earthquake landslide in Gorkha, Nepal, 2015, as an example, Tian et al. analyzed the geometric characteristics of 2,059 landslides with an area larger than 10,000 m2 based on a landslide inventory map obtained from the visual identification of pre- and post-quake optical satellite images (Xu et al., 2018). They separated these landslides into three types according to their geometry, and the relationships between these landslides and environmental factors are analyzed and compared, respectively. The results showed that landslides with different geometric shapes are more likely to occur in different environments, which provides a case study for further understanding the spatial distribution characteristics of earthquake-triggered landslides. Based on the data of nearly 200,000 coseismic landslides triggered by the 2008 Mw 7.9 Wenchuan, China earthquake (Xu et al., 2014), Liu X. et al. selected some typical watersheds and analyzed the area-frequency distribution relationship of coseismic landslides, and the obtained parameters reflect the landslide density and the proportion of large-scale landslides. They also carried out a hot spot analysis of landslides to evaluate the relationship between the obtained parameters and the spatial distribution of the landslide cluster. This work provides a case study to better understand the landslide area-frequency distribution law.
Remote sensing and GIS technology play an increasingly important role in large-scale individual landslide research. The occurrence of many large-scale landslides has a process of initial deformation, deformation acceleration, and failure. Remote sensing and GIS technologies are effective methods for detecting slope deformation. For example, the Baige landslide on the Jinsha River in China in 2018 had significant deformations up to tens of meters within a few years before the final sliding (Cui et al., 2020). To analyze the creep process of the landslide, Guo et al. examined the vegetation changes on the landslide based on multi-temporal SPOT satellite data. The study showed that the landslide has been creeping about 50 m in the past few years, and the vegetation coverage on the back wall and the body of the landslide decreases significantly as it is closer to the final sliding, which revealed a strong correlation between vegetation change rate and landslide creeping. This work provides a case study for analyzing the deformation process of landslides based on multi-temporal optical remote sensing data. Hu et al. investigated the age of the residual deposits probable from a large landslide on the east bank of the Qiaojia reach of the Jinsha River based on UAV mapping technology and dating technology. They considered the landslide event might be related to an earthquake in AD 624, which provides a shred of evidence from the ancient landslide for subsequent seismic hazard analysis in this area. This work provides a case study for analyzing regional seismic hazards based on large individual landslides supported by remote sensing technology.
Monitoring the in-situ seismic response of rock slopes using seismological or geophysical methods can be considered an application of generalized remote sensing technology in the field of landslide monitoring. Based on this method, Huang et al. investigated the response of a high bedrock slope in the mountainous area of southwest China related to the 2019 Changning, China Mw5.7 earthquake. They used environmental noise interferometry to monitor the performance of the rocky slope during the earthquake and located a coseismic rock rupture at a depth of 75 m in the slope that recovered after 2 weeks. This work demonstrates the feasibility of using seismological techniques to analyze coseismic deep fissures in bedrock.
Remote sensing and GIS technologies are essential for regional earthquake-triggered landslide susceptibility and hazard assessments. Remote sensing is important for preparing completed and detailed landslide inventory maps and producing environmental thematic maps. The combination of GIS and machine learning models is one of the main methods for this aspect. Machine learning methods and Newmark physical model are the two most common methods. In this Research Topic, we solicited one paper for each of these two methods. In machine learning methods, the selection of landslide samples is an important task. Chen et al. applied a one-class classifier method to generate landslide negative sample data, and performed a rapid earthquake-induced landslide susceptibility analysis in an area affected by the 2008 Wenchuan Mw7.9 earthquake in China. This work provided a method reference for the production of landslide negative samples in landslide susceptibility and hazard assessments. The application of the Newmark model to regional seismic landslide hazard analysis has received much attention in recent decades, in which the thickness of landslide mass is an important, but difficult, to obtain parameter. Maharjan et al. obtained the median landslide thickness based on pre- and post-quake high-resolution DEMs for subsequent landslide hazard assessment. The results have good spatial consistency with the real distribution of coseismic landslides. It shows that it is feasible to obtain the pre- and post-quake DEMs based on remote sensing technology to obtain the thickness of the landslide mass and conduct a more objective and rapid earthquake-triggered landslide susceptibility and hazard mapping.
Massive landslides and strength reduction in hillslope materials related to large earthquakes will have serious secondary effects and severe secondary disasters are likely to occur under subsequent strong aftershocks and heavy rainfall (Fan et al., 2019). Therefore, it is of great significance to study the evolution of landslides in affected areas and the changes in landslide susceptibility after earthquakes. Tanyaş et al. prepared a sequence of multi-temporal landslide inventory maps in three tropics areas affected by major earthquakes. Based on the landslide inventory maps, they analyzed the landslide susceptibility levels pre- and post-quake and concluded that landslide susceptibility returned to pre-quake levels in less than a year. Since the study areas are only parts of the total earthquake-affected area, they are cautious that these observations may not represent the entire area affected by these earthquakes but only the phenomena in the study areas. Guo et al. conducted a study on landslide susceptibility changes in the 2017 Ms7.0 Jiuzhaigou, China earthquake based on machine learning, remote sensing, and GIS technologies. They produced a coseismic landslide inventory map and a post-event landslide inventory map 2 years after the earthquake and found that the total landslide area increased by 1.2 km2. They compared and analyzed the relationship between the two landslide inventory maps and the influencing factors. The results show that the amplitude in susceptibility in high landslide susceptibility areas is smaller than that in low susceptibility areas. This indicates that the landslide susceptibility in the earthquake-affected area is not a constant state, and reminds us to pay attention to the change of the landslide susceptibility in earthquake-affected areas. Earthquakes have also an important impact on rainfall thresholds of debris flows. Liu S. et al. analyzed the changes in the pre- and post-quake rainfall thresholds for debris flows under the influence of different seismic intensities based on the debris flow records in the affected areas of the 2008 Ms8.0 Wenchuan, 2013 Ms7.0 Lushan and 2017 Ms7.0 Jiuzhaigou earthquakes in China. The study shows that the post-quake rainfall threshold for debris flows is much lower than that of the pre-quake. In addition, they also considered the influence of earthquake magnitude and focal depth on the change of rainfall thresholds. This study provides support for more objective post-quake debris flow early warning.
In recent years, with the development of remote sensing, GIS, big data, artificial intelligence, and other high-tech, studies on landslides, especially earthquake-triggered landslides, have achieved rapid expansion, and relevant publications show a growing trend year by year (Huang et al., 2022). The 13 papers in this Research Topic involve multiple directions of remote sensing- and GIS-based seismic landslide research, including landslide inventory mapping, automatic extraction, spatial distribution, and evolution analysis, which have accumulated knowledge for the excellent development of this discipline. However, these studies also raised a few new questions for researchers. For example, in the aspect of automatic landslide extraction, although many advanced landslide extraction models based on remote sensing data have emerged in recent years, it is still a noteworthy direction to develop more scientific and reasonable models and further improve the accuracy. As for landslide inventory maps and their spatial distribution analysis, more high-quality earthquake-triggered landslide mapping is needed to reveal the more general temporal and spatial distribution law of earthquake-triggered landslides. The research on the relationship between ancient landslides and historical earthquakes has always been a concern of researchers (Jibson 1996), but most of the existing work is based on individual landslides or local areas. Remote sensing and GIS technology provide sufficient conditions for creating high-quality ancient landslide inventory maps in broad areas, even in the world. Of course, this great work requires the joint efforts of lots of researchers. As most large landslide that can be preserved for hundreds or even thousands of years, it will undoubtedly be a direction worthy of long-term development. Applying generalized remote sensing such as seismological and geophysical methods in large landslides or seismic slope response is a very meaningful attempt, and it is also a subject growth direction worthy of in-depth exploration.
The increased capacity in remote sensing, geographic information technologies, and computational power contribute to a more thorough understanding of geoenvironmental and earth surface processes. Natural disaster prevention, mitigation, and relief are the eternal subjects of human survival and development. We hope that this Research Topic can promote the development of earthquake-triggered landslide research and attract more researchers to pay more attention to the application of high-tech, such as remote sensing and GIS, in the field of earthquake-triggered landslides.
All authors contributed to the critical review of the papers published in this Research Topic. CX has provided an initial draft of this Editorial which was revised and approved by all the authors.
This study was supported by the National Institute of Natural Hazards, Ministry of Emergency Management of China (ZDJ2021-14).
We deeply thank all the authors for their submissions to this Research Topic and all the reviewers for their valuable comments and suggestions, the Frontiers Editorial Office, and especially Josie Langdon and Ursula Rabar, for their strong supports in all phases of the realization of this Research Topic.
The authors declare that the research was conducted in the absence of any commercial or financial relationships that could be construed as a potential conflict of interest.
All claims expressed in this article are solely those of the authors and do not necessarily represent those of their affiliated organizations, or those of the publisher, the editors and the reviewers. Any product that may be evaluated in this article, or claim that may be made by its manufacturer, is not guaranteed or endorsed by the publisher.
Cui, Y., Bao, P., Xu, C., Fu, G., Jiao, Q., Luo, Y., et al. (2020). A big landslide on the jinsha river, tibet, china: geometric characteristics, causes, and future stability. Nat. Hazards. 104 (3), 2051–2070. doi:10.1007/s11069-020-04261-9
Fan, X., Scaringi, G., Korup, O., West, A. J., van Westen, C. J., Tanyas, H., et al. (2019). Earthquake-induced chains of geologic hazards: Patterns, mechanisms and impacts. Rev. Geophys. 57 (2), 421–503. doi:10.1029/2018rg000626
Harp, E. L., Keefer, D. K., Sato, H. P., and Yagi, H. (2011). Landslide inventories: the essential part of seismic landslide hazard analyses. Eng. Geol. 122 (1-2), 9–21. doi:10.1016/j.enggeo.2010.06.013
Huang, Y., Xu, C., Zhang, X., and Li, L. (2022). Bibliometric analysis of landslide research based on the WOS database. Nat. Hazards Res.. doi:10.1016/j.nhres.2022.02.001
Jibson, R. W. (1996). Use of landslides for paleoseismic analysis. Eng. Geol. 43 (4), 291–323. doi:10.1016/S0013-7952(96)00039-7
Keefer, D. K. (1984). Landslides caused by earthquakes. Geol. Soc. Am. Bull. 95 (4), 406. doi:10.1130/0016-7606(1984)95<406:lcbe>2.0.co;2
Tian, Y., Xu, C., and Yuan, R. (2022). Earthquake-triggered landslides. Treatise on geomorphology 2, 583–614. doi:10.1016/B978-0-12-818234-5.00120-6
Xu, C. (2015). Preparation of earthquake-triggered landslide inventory maps using remote sensing and GIS technologies: Principles and case studies. Geosci. Front. 6 (6), 825–836. doi:10.1016/j.gsf.2014.03.004
Xu, C., Tian, Y., Shen, L. L., Xu, X., Zhou, B. G., Ma, S., et al. (2018). Database of landslides triggered by 2015 Gorkha (Nepal) Mw7.8 earthquake. Seismol. Geol. 40 (5), 1115–1128. doi:10.3969/j.issn.0253-4967.2018.05.011
Keywords: remote sensing, GIS, landslide susceptibility, landslide evolution, landslide inventory map, landslide monitoring, landslide mechanism, earthquake-triggered landslides
Citation: Xu C, Gorum T and Tanyas H (2022) Editorial: Application of remote sensing and GIS in earthquake-triggered landslides. Front. Earth Sci. 10:964753. doi: 10.3389/feart.2022.964753
Received: 09 June 2022; Accepted: 30 June 2022;
Published: 22 July 2022.
Edited and reviewed by:
Stefano Luigi Gariano, Institute for Geo-Hydrological Protection (IRPI), ItalyCopyright © 2022 Xu, Gorum and Tanyas. This is an open-access article distributed under the terms of the Creative Commons Attribution License (CC BY). The use, distribution or reproduction in other forums is permitted, provided the original author(s) and the copyright owner(s) are credited and that the original publication in this journal is cited, in accordance with accepted academic practice. No use, distribution or reproduction is permitted which does not comply with these terms.
*Correspondence: Chong Xu, eGMxMTExMTExMUAxMjYuY29t
Disclaimer: All claims expressed in this article are solely those of the authors and do not necessarily represent those of their affiliated organizations, or those of the publisher, the editors and the reviewers. Any product that may be evaluated in this article or claim that may be made by its manufacturer is not guaranteed or endorsed by the publisher.
Research integrity at Frontiers
Learn more about the work of our research integrity team to safeguard the quality of each article we publish.