- School of Maritime Economics and Management, Dalian Maritime University, Dalian, China
Inequality in the admission of migrant children is an important manifestation of inequities at the compulsory education stage in China. Spatial differences in the allocation of educational resources and the factors affecting the number of enrolled migrant children led to the following conclusions: 1) the total number of migrant children in Dalian is growing situationally, with a total increase of 29,223 from 2008 to 2020. 2) The migrant children in Dalian in 2020 are distributed in a spatially uneven manner, with most of them concentrated in the periphery of the Ganjingzi District, south of the Jinzhou area (suburban areas), where very few of them were enrolled in high-quality junior high and primary schools. 3) The distribution of educational resources differed significantly between the central urban areas and suburban areas. 4) The internal factor of schools constraining the admission of migrant children is the teacher–student ratio. For every 1% increase in the teacher–student ratio, the number of migrant children decreases by 4.12%. The main external factors affecting the admission of migrant children were rental prices and the area occupied by construction, which increased by 1% for each 2,000 m buffer range of school and 0.25% for the number of migrant children and decreased by 0.29% for each 2,000 m buffer range of the school.
1 Introduction
Since the economic reforms of the 1970s, China has been experiencing rapid industrialization and urbanization, which triggered the largest domestic urban–rural migration in the country’s history (Wang and Holland, 2011). This has resulted in a large influx of migrant workers seeking better employment opportunities and living conditions (Fang et al., 2016). The children they bring with them are called migrant children (Yang, 2005). One of the challenges migrant workers and their children face during the migration process is the inequality of migrant children’s access to educational resources, which is an important social problem in contemporary China (Ma and Wu, 2020). In 2020, there were 14,297,000 migrant children in China within the compulsory education stage, of which 85.8% went to public schools or had their tuition paid by government resources (National Bureau of Statistics, 2005). Particularly, large cities in China have seen many migrant children entering their public schools. Most migrant workers come from the inland provinces and poor rural areas in the west, who migrated to the eastern coastal regions where economic development is more advanced (Goodburn, 2009). As a large coastal city with a rapidly developing economy, population, and education system, Dalian has attracted many migrant workers. In 2020, there were 123,030 migrant children at the compulsory education stage in Dalian, accounting for 47% of all children in the Liaoning Province and 23% of school children in junior high and primary schools in Dalian. As such, it is particularly important to solve the problem of education inequality for migrant children in Dalian.
With the increase of educational inequality in large cities, scholars have grown more concerned about the household registration system, an important factor in the inequality of migrant children’s school admission (Qian and Walker, 2015; Wei and Gong, 2019). Theoretical concepts of isolation, assimilation, social mobility, social exclusion/integration, and citizenship have been widely used to study inequalities in education between urban and rural children and questioned whether migrant children could actually catch up with urban students. Because the government uses the household registration system to decide the configuration of educational resources, migrant children have poorer educational opportunities than their urban peers (Koo, 2012). Furthermore, Xiong (2015) suggested that the homogeneity of public schools under China’s education system and the household registration system is reducing the opportunities of migrant children for upward social mobility. Although improvements in policies have benefited many migrant children, household barriers are not fully eliminated yet. In fact, Qian and Walker, (2015) showed that megacities such as Shanghai and Beijing have tightened immigration control by introducing new restrictive systems to replace the original household registration system.
The standard of permanent residence is so high that it is difficult for migrant workers and their families to obtain official certificates. However, although migrant children can enter urban public schools without local household registration, they can only apply for low-quality public schools that are usually located in urban–suburban areas (Qian and Walker, 2015). High-quality public schools are usually located in urban centers, but they are reserved for local students. Therefore, school quality (not school isolation) has become one of the most important factors affecting the education of migrant children. While earlier research provided rich background information on the educational issues of migrant children and discussed policy implications from different perspectives, most of these studies were qualitative in nature (Zhou, 2006). Furthermore, many of these studies only focused on one or two school students and cannot represent the education of all migrant children in the city (Chen and Feng, 2019). Research on the education of migrant children has gradually moved from a qualitative nature to a quantitative one (Goodburn, 2009; Xiong, 2015). Yet, the existing quantitative studies have either focused on institutional barriers (Lai et al., 2014; Guo and Zhao, 2019; Wei and Gong, 2019) or individual factors (Hu, 2018; Ma et al., 2018; Wang et al., 2018). They have not interpreted the overall school distribution of migrant children in the city and the reasons for this distribution.
Points of interest (POI) data, important geographic urban space big data (Liu K. et al., 2020; Zhang D. et al., 2020), can describe the spatial and attribute information of geographical entities in real-time, such as name, address, and coordinates. They have the advantage of larger sample sizes and more meticulous information coverage. POI-based research has been fruitful in areas such as business spatial distribution (Lan et al., 2018; Qiao et al., 2019; Zhang et al., 2021a; Xu et al., 2022), urban functional area identification (Zhai et al., 2019; Zhang et al., 2021b; Yu et al., 2022), urban built-up area identification (He, 2019; Yu et al., 2021; Zhang Z. et al., 2021), and spatial balance of basic education (Zhang et al., 2022). As such, the convergence of multi-source data such as school POI, internal data, and socio-economic data can provide a more comprehensive analysis of the specifics of migrant children’s education.
In summary, the current studies on educational issues of migrant children have mainly been statistical analyses and economic measurements (Tang and Wang, 2021). Research on the analysis of migrant children's education using a geographic information system (GIS) spatial analysis and spatial econometric models is scant. In terms of data, research on migrant children’s education has mainly used statistical data from a district or several schools in the city as samples. However, it is difficult to accurately reflect the internal relationships between the number of migrant children in the city, the internal factors of the school, and socioeconomic factors with small sample size. At the present stage of compulsory education, migrant children in China’s big cities no longer have any problems attending public schools. To address educational inequality, what they need is to study in better public schools. As a megacity in China, Dalian is currently facing such a problem with migrant children in public schools. Therefore, in this study, our sample will comprise all junior middle schools and primary schools in Dalian in 2020, which will be combined with population, gross domestic product (GDP), and rental price data. Using ArcGIS 10.6, we will construct a geographic database and combine spatial analysis and measurement to analyze and interpret the educational equality of migrant children from the following three aspects: 1) change in the number of migrant children over time using districts and counties as units; 2) the distribution status of migrant children in junior high and primary schools in Dalian as well as the differences in the distribution of educational resources using schools as the unit; and 3) the influence of internal and external factors in school on the number of migrant children using the spatial metrology model and junior high/primary schools as samples. The innovation of this study lies in the richness of data types and the number of spatial measurement samples. It combines spatial visualization and spatial measurement to explore the education equality of migrant children and provide a new perspective for the research on education equality of migrant children in other cities.
2 Methodology
2.1 Study Area
Figure 1 show Dalian is an important hub and megacity along the northern coast of China covering a total area of 12,574 km2. Like most large cities in China, the development of compulsory education in Dalian is facing the problem of unequal allocation of educational spaces. Migrant children's education is particularly important for developing the equilibrium of compulsory education. In 2020, the number of migrant children in Dalian was 123,030, which was 47% of the total number of migrant children in Liaoning Province and 23% of the total number of students in junior high and primary schools in Dalian. Thus, studying the equilibrium of migrant children's education in Dalian is extremely representative. This study focuses on seven districts and one county under the Dalian jurisdiction. The main study area is the two county-level cities in the alternative tube, which provides us a sample size of 639 schools in the compulsory education stage. Out of these 639 schools, 411 are primary schools and 228 are junior middle schools, with 80,277 and 42,803 migrant children studying, respectively. We explored the spatial equitability of migrant children in the mandatory education stage and the influence of each factor on it, which can provide a valuable framework for studying the equality of migrant children's education in other cities in China.
2.2 Data Source and Processing
In this study, the number of migrant children attending junior high and primary schools in Dalian, China, was mainly derived from information provided by government agencies. The collected data included the number of school children in junior high and primary schools in Dalian in 2020, the number of dedicated teachers, the number of personnel with a professional technical title of intermediate and above, the construction area, the number of books, the number of computer stations, the value of teaching equipment, and other statistical information. We used the crawl Baidu Map API and Gaode Map API with Crawler software to get the longitude and latitude coordinates of the schools where the migrant children were located, so as to obtain the spatial attributes of the primary and secondary schools. The GDP data were obtained from the 2020 nighttime light imaging pass (https://ngdc.noaa.gov/) and are available for public download. Furthermore, Dalian 100 m * 100 m population raster data were primarily obtained from WorldPop (https://www.worldpop.org/), which is available for public download. The rental price data of Dalian were crawled using Python on the ANN dwelling website. Coordinate tangle, coordinate point counter inspection, and address information correction complement were performed to ensure data precision.
Furthermore, the whole and joint rental prices were uniformly adjusted to the whole rental price according to the housing estates, resulting in 2070 attributes with average rental price point coordinates. The data also included administrative divisions in the study area. Land under construction data were derived from the National Basic Geographic Information System Database. In this study, ArcGIS 10.6 was utilized after normalizing the aforementioned data to construct a geographic information database.
2.3 Methods
2.3.1 Spatial Interpolation
Spatial interpolation has been widely used in many fields, including deterministic, geodesic, and barrier-containing interpolation (Tian et al., 2021; Yang et al., 2021; Yu et al., 2021). The spatial data directly acquired in the research were based on point/face statistics or measured data of some monitoring points. The spatial precision of the data was lacking. Therefore, the correspondence with the space to produce high-precision full coverage was simulated by spatial interpolation to obtain continuous data surfaces. In this study, we used junior high and primary schools as basic points. The ArcGIS 10.6 software was used to construct a geographic database after processing the evaluation indicators of the impact factors on migrant children; the interpolation of inverse distance weight was used to transform each indicator into a data surface and spatially visualize it.
2.3.2 Correlation Analysis
Linear correlation analysis is commonly used to analyze the correlation between variables, and the index used to measure it is known as the linear correlation coefficient. There are three main correlation coefficients: Pearson, Spearman, and Kendall, which apply to different occasions. By calculating the correlation coefficient (mainly R-value), the value range of R is [−1, 1]. If there is a positive correlation, then r > 0; if the correlation is negative, then r < 0. Furthermore, the relationship strength is analyzed as follows: |r| > 0.95: significant correlation; |r| ≥ 0.8: highly correlated; 0.5 ≤ |r| < 0.8: moderate correlation; 0.3 ≤ |r| < 0.5: low correlation, and |r| < 0.3: weak correlation.
Pearson’s correlation coefficient:
Spearman’s rank correlation:
Kendall:
2.3.3 Spatial Econometric Model
The ordinary least squares (OLS) model is considered the standard analysis framework in econometric analysis. However, it may ignore spatial dependence, leading to biased or even invalid estimations. Many researchers who have attempted to solve this limitation have found that the most important analysis framework is actually the spatial econometric model.
Spatial econometric models can avoid the deviation of classical econometrics when analyzing spatial effects. The commonly used spatial econometric models include the spatial lag model (SLM) and the spatial error model (SEM) (Kang, 2015; Chen et al., 2017). The SLM is as follows:
where Y is the dependent variable, X is the explanatory variable, W is the spatial weight matrix, Wy is the spatial lag variable, and ρ is the spatial regression coefficient, which reflects the degree of diffusion or spillover between adjacent spatial units as well as the influence of X on Y. It is also the random error term.
The SLM mainly verifies the spatial spillover effect of dependent variables in a region. It also ensures that the influencing factors of dependent variables are applied to other regions through the spatial transmission mechanism (Liu Y. et al., 2020). In contrast to the SLM, the SEM verifies the spatial dependence in the disturbance error term and measures the error impact of the dependent variables in adjacent areas on the local dependent variables. The SEM is as follows:
where Y is the dependent variable, X is the explanatory variable, W is the spatial weight matrix,
2.3.4 Index Selection and Processing
Previous reviews on the influencing factors of equality in educational resource allocation have mainly focused on screening outside schools and screening the administrative unit with the smallest research scale being just to the streets (town) (Sajjad et al., 2022) or the regression analysis with some schools in the streets (town) as the sample; when the research scale is not fine enough, the study sample size is not enough (Omoeva et al., 2021). This study gives the indicators of geospatial attributes using ArcGIS software. Our sample included all the schools in Dalian that contained migrant children. This resulted in a large volume of data, which broke the administrative region’s boundary limit. Considering the effect of educational resources on the number of migrant children from both inside and outside the school adds credibility to the study’s results. The selected indicators are shown in Table 1.
Faculty is the core factor in the distribution of compulsory education resources, which has a decisive effect on the quality of compulsory education. Therefore, we measured the number of teachers using the student-to-teacher ratio to reflect the adequacy of the number of teachers. The proportion of primary (junior) teachers with higher (undergraduate) education and teachers with intermediate and above professional technical titles was also measured. The hardware resources include the campus area, books, computers, and teaching equipment. We also chose the area of school buildings with the students, the raw average number of books, and every hundred students having four secondary indicators of the number of computer stations.
Financial resources refer to compulsory educational funds mainly from government financial grants, which are mostly used to pay teacher salaries, acquire teaching equipment, and improve the campus environment. According to the principle of independence selected by the indicators, there is a causal relationship between educational funding and operational conditions. Therefore, this study focuses on two aspects: faculty strength and operational conditions.
Socio-economic factors can affect migrant workers’ job choices and the admission choices for migrant children according to the service radius of junior high schools as prescribed by the country. Therefore, this study focuses on junior high and primary schools. We drew a buffer radius of 2,000 m around the schools and calculated the average GDP and population within this radius, which can reflect the quality of life and employment of migrant workers. This is important since the admission of migrant children is closely related to the cost of residence and the place of work of the migrant workers. Additionally, since most migrant workers in Dalian are likely to rent, the rental amount will affect the choice of schools for migrant children and subsequently the number of migrant children in school. Therefore, we used rental prices as an important indicator to calculate the average rental price within 2,000 m radius of junior high and primary schools.
The construction area reflects the development status of industries near the school. Large construction areas provide more employment opportunities for migrant workers. However, migrant workers working near the school will affect the number of migrant children in school. Therefore, we used the amount of construction going on within the school’s 2,000 m buffer area as an important indicator.
3 Results
3.1 Spatial–Temporal Evolution of Migrant Children
Figure 2 shows the total number of migrant children in various regions from 2008 to 2020: Ganjingzi District > Jinzhou District > Lvshunkou District > Shahekou District > Xigang District > Zhongshan District > Pulandian District > Wafangdian District > Zhuanghe District > Changhai District. Jinzhou District surpassed Ganjingzi District in 2019, and the total number of migrant children increased from 93,807 in 2008 to 123,030 in 2020. The number of migrant children in Ganjingzi, Jinzhou, and Lvshunkou districts showed an upward trend, while the number of migrant children in Changhai District showed a downward trend. There was no significant change in other regions. With rapid urbanization, more employment opportunities are available to migrant workers, especially in Ganjingzi, Jinzhou, and Lvshunkou districts. These opportunities are found in construction, machinery, smelting, textiles, clothing, aquatic work, catering, and other jobs. The quality of schools in the older urban areas of Zhongshan, Xigang, and Shahekou districts remains high. However, the number of schools and student capacity is about to reach saturation, which will make it difficult for migrant children to enter these areas in large numbers. Due to the geographical location of Changhai District, migrant children are now choosing to study in alternative areas with better educational development. To see the distribution of migrant children in different regions in detail, we used the school as a unit in ArcGIS 10.6 interpolation analysis, which visually expresses the spatial distribution of migrant children.
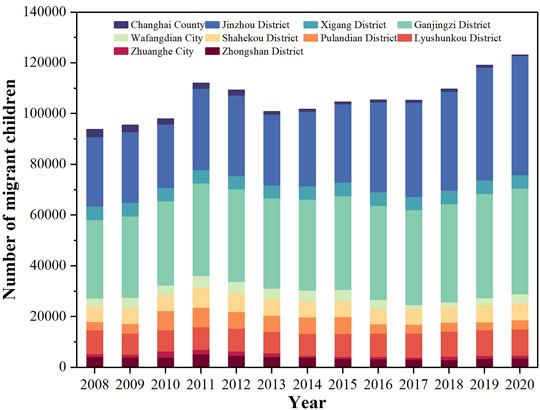
FIGURE 2. Statistical chart of changes in the number of migrant children in Dalian from 2008 to 2020.
Figure 3A shows that schools with many migrant children are mainly located in the suburbs of Dalian, including the south of Jinzhou District and the periphery of Ganjingzi and Lvshunkou districts. From the ratio of the number of migrant children to the total number of school students in Figure 3B, we can see that migrant children are mostly located in Jinzhou, Ganjingzi, and Lvshunkov districts. Jinzhou District accounted for 38% of the migrant children, Ganjingzi District, 34%, and Lvshunkou District, 8%. The number and proportion of migrant children in Wafangdian (3%), Pulandian (3%), and Zhuanghe (1%) are much lower than those of Jinzhou, Ganjingzi, and Lvshunkov, which reflects the population flow from suburban to core areas during urbanization.
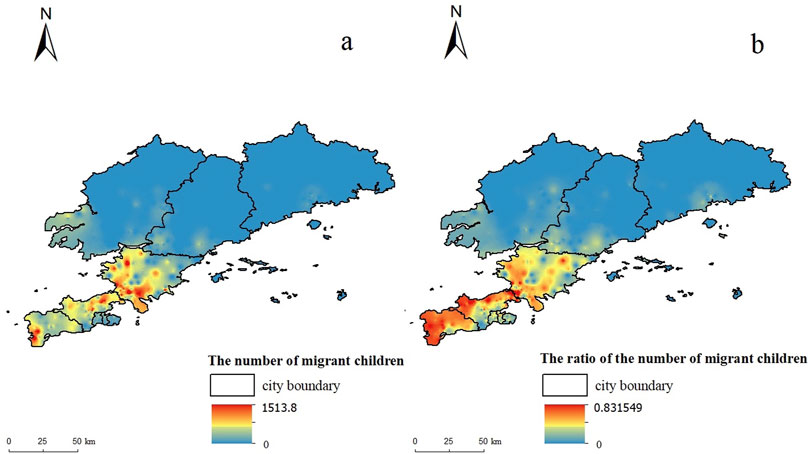
FIGURE 3. Spatial distribution of the number (A) and proportion of migrant children (B) in Dalian from 2008 to 2020.
The survey data showed that high-quality primary and secondary schools in Dalian are mainly located in urban core areas such as Shahekou, Xigang, and Zhongshan districts. However, the number and proportion of migrant children are much lower in these core areas than those of the south of Jinzhou District and the peripheral areas of Ganjingzi and Lvshunkou districts. These districts show the uneven distribution of migrant children and the difficulties migrant children face in entering high-quality junior middle and primary schools at the compulsory education stage. It also reflects the unfairness to migrant children in terms of resource allocation at the compulsory stage. To further verify the aforementioned results statistically and analyze the changes in the number of migrant children in terms of socio-economics, teacher strength, school operating conditions, and other factors, we constructed a spatial econometric model. In this model, the number of migrant children is the dependent variable. Furthermore, teacher strength, school operating conditions, and socio-economic factors were set as the independent variables for subsequent spatial metrology studies.
The indicators of influencing factors after spatial interpolation are shown in Figure 4. As shown, there are significant differences in the distribution of educational resources between the central urban area and the suburbs. The distribution of resources as reflected by the teacher–student ratio, the construction area of school buildings per student, the number of books per student, computers per student, and teaching equipment per student are highly concentrated in Lvshunkou, Ganjingzi, and Wafangdian districts and other suburbs. Higher values of average GDP, population, rental price, and construction land area within the school’s 2,000 m buffer zone were found in core areas such as Zhongshan, Shahekou, and Xigang districts and the south of Jinzhou District. The socio-economic development of suburbs containing many migrant children was weaker than that of central urban areas. Compared to the central urban areas with relatively saturated educational resources, the suburbs still have a large room for development. We can improve the school attendance of migrant children in Jinzhou, Ganjingzi, and Lvshunkou districts by increasing educational investment and fairly reallocating existing resources to promote improved educational equality.
3.2 Analysis of Influencing Factors
Table 2 shows the analysis results of index correlation. The study found that LNUG and LNMPT failed the index correlation test, while other indices passed the correlation test. At the level of 0.01 (single tail), the correlation was significant.
This study used GeoDa software to calculate the regression results. Before establishing the spatial econometric model, a spatial correlation test was conducted on the sample data. Table 3 shows that Moran’s I value was 12.903. The significance level was lower than 0.001, indicating that the spatial distribution of migrant children’s enrollment is not completely random but has a certain spatial correlation. Therefore, the regression results of OLS might deviate, so it is necessary to use the spatial econometric model while considering spatial effects for estimation. From the perspective of variable coefficient and significance, the calculation results of the model were consistent. This result indicated that the consistency of the results was high, and the R2 value increased after considering the spatial correlation (SLM = 0.692 and SEM = 0.0.687), which were both higher than the R2 value of OLS 0.592. The interpretation results of SLM and SEM were thus better than the OLS regression results.
However, the spatial lag coefficient was 0.749, and its significance level was lower than 0.001. Therefore, we can conclude that the number of migrant children in different schools is affected by the development of schools, socio-economic status, and other factors in the region. The spatial error coefficient was 0.971, and its significance level is lower than 0.001, which verifies that the number of migrant children in schools in each region is also affected by factors not considered in adjacent regions and random shocks. Combining the LM and R-LM values and comparing the fitting effects of SLM and SEM, the significance of the regression and test results of SLM was better than that of the model results of SEM. Therefore, SLM results were selected for subsequent analysis in this study.
Table 3 shows that in the regression results, LNSTR, LNPBA, LNPC, and LNHP showed significant negative effects, while LNPB, LNPTE, and LNCL showed significant positive effects. The effect degree of the significant factors also differed, and they are ranked as follows: LNSTR > LNPC > LNPTE > LNPB > LNPBA > LNHP > LNCL.
Among the internal influencing factors in schools, the most critical factor constraining the inequality in school children’s admission at this stage was LNSTR. For every 1% increase in LNSTR, the number of migrant children decreased by 4.12%. Therefore, the greater the number of migrant children in junior high and primary schools, the fewer the teaching resources they get. Core area teachers and students were relatively low because the educational resources in the urban center were saturated, the universal source of students was fulminant, the supernumerary number of classes, and the operation of school overload, giving migrant children less access to quality public secondary and primary schools.
Therefore, the number of classes should be adjusted appropriately in the city’s core area. Additionally, teachers should be allocated properly to schools with high numbers of migrant children to achieve a reasonable distribution of educational resources. From Figure 3, the higher LNSTR values were found in Wafangdian, Zhuanghe, and Pulandian districts, which was due to the low number of migrant children in these areas. This shows that migrant children migrated from the edge to the center of the city and were mainly located in the developed subcenter area, which is the peripheral area of Ganjingzi District, the south area of Jinzhou District, and Lvshunkou District. In addition, the number of migrant children decreased by 0.51% for every 1% increase in LNPBA; for every 1% increase in LNPC, the number of migrant children decreased by 2.31%, which exacerbates the inequality of migrant children in schools to a certain extent. For every 1% increase in LNPB, the number of migrant children increased by 0.57%. For every 1% increase in LNPTE, the number of migrant children increased by 0.85%, which alleviates the inequality of migrant children in schools to a certain extent.
The study also found that LNPD and LNPGDP were negative and insignificant. Since the population in economically developed regions is relatively denser (Zhu and Chen, 2022), more children were enrolled in age-appropriate schools in the core areas, which leads to eventual saturation. Therefore, although more employment opportunities in economically developed areas may attract more exotic populations, the separation of employment from high rental prices and saturated competition among age-appropriate children make it harder for migrant children to attend schools in developed core areas.
4 Discussion
This study mainly discusses the inequality that migrant children face in school admission. We focused on problems migrant children faced such as a difficult admission process and poor access to high-quality schools and educational resources. Then, we explored the effect of the internal school environment and external regional socio-economic factors on schools’ admission of migrant children. Our study has made some unique contributions to the existing literature on education equality for Chinese migrant children. We confirmed that migrant students no longer had problems accessing schools. Instead, their problem lies in the difficulty of obtaining quality education resources. The starting point of the study is that migrant children and their families face many challenges in migration destinations, such as discrimination, economic insecurity, educational inequality, lack of access to health care, risk of being expelled back to rural areas, and social exclusion. However, educational inequality is currently the most concerning issue and social problem. Inequalities in school quality stem from economic, human, and socio-emotional factors, which exist in different types of school environments where migrant children live. Considering that school quality can significantly impact differences in achievement, this conclusion awaits wide acceptance among researchers (Ma and Wu, 2019; Zhang J. et al., 2020).
On the factors affecting the school admission of migrant children, we believe that in addition to the influence of the school’s policies and governance, migrant children's admission was also affected by the working and dwelling place of their migrant worker parents. Therefore, in this study, the average rent and construction land area within a 2,000 m buffer radius of the school were used as important indicators.
In terms of research data, we combined school POI data, socio-economic statistical data, and geographical data with the support of internal government data to examine 639 junior high and primary schools in Dalian with a total enrollment of 123,030 migrant children and endow them with spatial attributes. We then analyzed the spatial distribution status of the migrant children and the inequality of access to educational resources. Our results showed that migrant children were mostly concentrated in Ganjingzi District, Jinzhou Suburban, and Lvshunkov District. Only a few migrant children had access to high-quality schools with abundant educational resources within the core area, which is consistent with the findings of Chen et al. (2017). This study has several limitations. First, our study only covered the area of Dalian due to the limitation of data. Given that the equality of migrant children's education in all large cities of China must be addressed, it is necessary to conduct future studies on the distribution of educational resources for migrant children in other large cities in China. Second, due to the inconsistencies within the data sample, this study only used cross-sectional data from 2020. In the future, we will study the evolution of fairness in migrant children's education and further explore the different influencing factors.
5 Conclusion
In this study, spatial analysis and spatial measurement were combined to analyze the spatial fairness of the distribution of migrant children and the factors affecting the number of school staff children in Dalian. Our comprehensive interpretation of the educational equality issues facing migrant children provides a new perspective for similar research in other cities in China. The main conclusions are as follows: 1) the total number of migrant children in Dalian grew steadily between 2008 and 2020; 2) the migrant children in Dalian in 2020 are distributed in a spatially uneven manner, with most of them found in the suburban areas; 3) the distribution of educational resources differed significantly in central urban areas and suburban areas; and 4) the inequality in migrant children’s school admission is closely related to rapid industrialization and urbanization in China. Therefore, regarding the inequality in migrant children’s school admission, we further analyzed the association between the number of children, school housekeeping, school quality, and socio-economic factors. We found that the most prominent factor contributing to the inequality of school admission was LNSTR; however, infrastructure conditions such as teaching equipment in the school had a weak effect on the inequality of school admission for migrant children. The difference in land use and rent prices as external socio-economic factors also exacerbated inequality in the enrollment of migrant children. In contrast to urban residents, migrant children are mostly from disadvantaged, low-income families with low-educated parents, which means that the inequitable distribution of educational resources will only intensify without government intervention.
Furthermore, the government needs to introduce policies to ensure that migrant children are treated exactly the same as local students in all aspects of education and teaching activities and incorporate the admission of migrant children into the scope of social development planning and financial security. By treating them equally, migrant children can be better integrated into school life, which promotes the growth of their physical and mental health. This can help achieve educational equity for all.
Data Availability Statement
The original contributions presented in the study are included in the article/Supplementary Material; further inquiries can be directed to the corresponding author.
Author Contributions
Methodology, WL; software and validation, ZZ and CT; formal analysis, ZZ and MS; writing—original draft preparation, ZZ and ZL; writing—review and editing, WL; visualization, ZZ; funding acquisition, WL. All authors have read and agreed to the published version of the manuscript.
Funding
This work was supported by the Key Project of the National Natural Science Foundation of China (No. 42030409) and China Postdoctoral Science Foundation (No. 2021M702789).
Conflict of Interest
The authors declare that the research was conducted in the absence of any commercial or financial relationships that could be construed as a potential conflict of interest.
Publisher’s Note
All claims expressed in this article are solely those of the authors and do not necessarily represent those of their affiliated organizations, or those of the publisher, the editors, and the reviewers. Any product that may be evaluated in this article, or claim that may be made by its manufacturer, is not guaranteed or endorsed by the publisher.
References
Chen, W. Y., Hu, F. Z. Y., Li, X., and Hua, J. (2017). Strategic Interaction in Municipal Governments' Provision of Public Green Spaces: A Dynamic Spatial Panel Data Analysis in Transitional China. Cities 71, 1–10. doi:10.1016/j.cities.2017.07.003
Chen, Y., and Feng, S. (2019). The Education of Migrant Children in China's Urban Public Elementary Schools: Evidence from Shanghai. China Econ. Rev. 54, 390–402. doi:10.1016/j.chieco.2019.02.002
Fang, L., Sun, R. C. F., and Yuen, M. (2016). Acculturation, Economic Stress, Social Relationships and School Satisfaction Among Migrant Children in Urban China. J. Happiness Stud. 17 (2), 507–531. doi:10.1007/s10902-014-9604-6
Goodburn, C. (2009). Learning from Migrant Education: a Case Study of the Schooling of Rural Migrant Children in Beijing. Int. J. Educ. Dev. 29 (5), 495–504. doi:10.1016/j.ijedudev.2009.04.005
Guo, Y., and Zhao, L. (2019). The Impact of Chinese Hukou Reforms on Migrant Students' Cognitive and Non-cognitive Outcomes. Child. Youth Serv. Rev. 101, 341–351. doi:10.1016/j.childyouth.2019.04.017
He, B.-J., et al. (2019). Towards the Next Generation of Green Building for Urban Heat Island Mitigation: Zero UHI Impact Building. Sustain. Cities Soc. 50, 101647. doi:10.1016/j.scs.2019.101647
He, Q., He, W., Song, Y., Wu, J., Yin, C., and Mou, Y. (2018). The Impact of Urban Growth Patterns on Urban Vitality in Newly Built-Up Areas Based on an Association Rules Analysis Using Geographical 'big Data'. Land Use Policy 78, 726–738. doi:10.1016/j.landusepol.2018.07.020
Hu, F. (2018). Migrant Peers in the Classroom: Is the Academic Performance of Local Students Negatively Affected? J. Comp. Econ. 46 (2), 582–597. doi:10.1016/j.jce.2017.11.001
Kang, C.-D. (2015). The Effects of Spatial Accessibility and Centrality to Land Use on Walking in Seoul, Korea. Cities 46, 94–103. doi:10.1016/j.cities.2015.05.006
Koo, A. (2012). Is There Any Chance to Get Ahead? Education Aspirations and Expectations of Migrant Families in China. Br. J. Sociol. Educ. 33 (4), 547–564. doi:10.1080/01425692.2012.678755
Lai, F., Liu, C., Luo, R., Zhang, L., Ma, X., Bai, Y., et al. (2014). The Education of China's Migrant Children: The Missing Link in China's Education System. Int. J. Educ. Dev. 37, 68–77. doi:10.1016/j.ijedudev.2013.11.006
Lan, T., Yu, M., Xu, Z., and Wu, Y. (2018). Temporal and Spatial Variation Characteristics of Catering Facilities Based on POI Data: a Case Study within 5th Ring Road in Beijing. Procedia Comput. Sci. 131, 1260–1268. doi:10.1016/j.procs.2018.04.343
Liu, K., Yin, L., Lu, F., and Mou, N. (2020a). Visualizing and Exploring POI Configurations of Urban Regions on POI-type Semantic Space. Cities 99, 102610. doi:10.1016/j.cities.2020.102610
Liu, Y., Zhang, X., Pan, X., Ma, X., and Tang, M. (2020b). The Spatial Integration and Coordinated Industrial Development of Urban Agglomerations in the Yangtze River Economic Belt, China. Cities 104, 102801. doi:10.1016/j.cities.2020.102801
Ma, G., and Wu, Q. (2020). Cultural Capital in Migration: Academic Achievements of Chinese Migrant Children in Urban Public Schools. Child. Youth Serv. Rev. 116, 105196. doi:10.1016/j.childyouth.2020.105196
Ma, G., and Wu, Q. (2019). Social Capital and Educational Inequality of Migrant Children in Contemporary China: a Multilevel Mediation Analysis. Child. Youth Serv. Rev. 99, 165–171. doi:10.1016/j.childyouth.2019.02.002
Ma, Y., Hou, X., Huang, J., Wang, W., Li, Y., Zhou, X., et al. (2018). Educational Inequality and Achievement Disparity: an Empirical Study of Migrant Children in China. Child. Youth Serv. Rev. 87, 145–153. doi:10.1016/j.childyouth.2018.02.026
National Bureau of Statistics (2005). National Bureau of Statistics of China. Beijing: State Council of the People's Republic of China.
Omoeva, C., Menezes Cunha, N., and Moussa, W. (2021). Measuring Equity of Education Resource Allocation: An Output-Based Approach. Int. J. Educ. Dev. 87, 102492. doi:10.1016/j.ijedudev.2021.102492
Qian, H., and Walker, A. (2015). The Education of Migrant Children in Shanghai: The Battle for Equity. Int. J. Educ. Dev. 44, 74–81. doi:10.1016/j.ijedudev.2015.07.009
Qiao, Z., Wu, C., Zhao, D., Xu, X., Yang, J., Feng, L., et al. (2019). Determining the Boundary and Probability of Surface Urban Heat Island Footprint Based on a Logistic Model. Remote Sens. 11 (11), 1368. doi:10.3390/rs11111368
Sajjad, M., Munir, H., Kanwal, S., and Naqvi, S. A. (2022). Spatial Inequalities in Education Status and its Determinants in Pakistan: a District-Level Modelling in the Context of Sustainable Development Goal-4. Appl. Geogr. 140, 102665. doi:10.1016/j.apgeog.2022.102665
Tang, Z., and Wang, N. (2021). School Disruption of Children in China: The Influence of Parents' Rural-Urban Migration. Child. Youth Serv. Rev. 129, 106167. doi:10.1016/j.childyouth.2021.106167
Tian, C., Luan, W., Li, S., Xue, Y., and Jin, X. (2021). Spatial Imbalance of Chinese Seafood Restaurants and its Relationship with Socioeconomic Factors. Ocean Coast. Manag. 211, 105764. doi:10.1016/j.ocecoaman.2021.105764
Wang, H., Cheng, Z., and Smyth, R. (2018). Do migrant Students Affect Local Students' Academic Achievements in Urban China? Econ. Educ. Rev. 63, 64–77. doi:10.1016/j.econedurev.2018.01.007
Wang, L., and Holland, T. (2011). In Search of Educational Equity for the Migrant Children of Shanghai. Comp. Educ. 47 (4), 471–487. doi:10.1080/03050068.2011.559701
Wei, Y., and Gong, Y. (2019). Understanding Chinese Rural-To-Urban Migrant Children's Education Predicament: A Dual System Perspective. Int. J. Educ. Dev. 69, 102066. doi:10.1016/j.ijedudev.2019.05.001
Xiong, Y. (2015). The Broken Ladder: Why Education Provides No Upward Mobility for Migrant Children in China. China Q. 221, 161–184. doi:10.1017/S0305741015000016
Xu, G., Jiang, Y., Wang, S., Qin, K., Ding, J., Liu, Y., et al. (2022). Spatial Disparities of Self-Reported COVID-19 Cases and Influencing Factors in Wuhan, China. Sustain. Cities Soc. 76, 103485. doi:10.1016/j.scs.2021.103485
Yang, J., Wang, Y., Xue, B., Li, Y., Xiao, X., Xia, J., et al. (2021). Contribution of Urban Ventilation to the Thermal Environment and Urban Energy Demand: Different Climate Background Perspectives. Sci. Total Environ. 795, 148791. doi:10.1016/j.scitotenv.2021.148791
Yu, H., Yang, J., Li, T., Jin, Y., and Sun, D. (2022). Morphological and Functional Polycentric Structure Assessment of Megacity: an Integrated Approach with Spatial Distribution and Interaction. Sustain. Cities Soc. 80, 103800. doi:10.1016/j.scs.2022.103800
Yu, Z., Song, Y., Song, D., and Liu, Y. (2021). Spatial Interpolation-Based Analysis Method Targeting Visualization of the Indoor Thermal Environment. Build. Environ. 188, 107484. doi:10.1016/j.buildenv.2020.107484
Zhai, W., Bai, X., Shi, Y., Han, Y., Peng, Z.-R., and Gu, C. (2019). Beyond Word2vec: an Approach for Urban Functional Region Extraction and Identification by Combining Place2vec and POIs. Comput. Environ. Urban Syst. 74, 1–12. doi:10.1016/j.compenvurbsys.2018.11.008
Zhang, D., Zhang, G., and Zhou, C. (2021a). Differences in Accessibility of Public Health Facilities in Hierarchical Municipalities and the Spatial Pattern Characteristics of Their Services in Doumen District, China. Land 10 (11), 1249. doi:10.3390/land10111249
Zhang, D., Zhou, C., Sun, D., and Qian, Y. (2021b). The Influence of the Spatial Pattern of Urban Road Networks on the Quality of Business Environments: the Case of Dalian City. Environ. Dev. Sustain. doi:10.1007/s10668-021-01832-z
Zhang, D., Zhou, C., and Xu, W. (2020a). Spatial-Temporal Characteristics of Primary and Secondary Educational Resources for Relocated Children of Migrant Workers: The Case of Liaoning Province. Complexity 2020, 1–13. doi:10.1155/2020/7457109
Zhang, J., Li, H., Lin, J., Zheng, W., Li, H., and Chen, Z. (2020b). Meta-analysis of the Relationship between High Quality Basic Education Resources and Housing Prices. Land Use Policy 99, 104843. doi:10.1016/j.landusepol.2020.104843
Zhang, N., Zhang, J., Chen, W., and Su, J. (2022). Block-based Variations in the Impact of Characteristics of Urban Functional Zones on the Urban Heat Island Effect: a Case Study of Beijing. Sustain. Cities Soc. 76, 103529. doi:10.1016/j.scs.2021.103529
Zhang, Z., Luan, W., Tian, C., Su, M., and Li, Z. (2021c). Spatial Distribution Equilibrium and Relationship between Construction Land Expansion and Basic Education Schools in Shanghai Based on POI Data. Land 10, 1059. doi:10.3390/land10101059
Zhou, C., Zhang, D., He, X., et al. (2021). Transportation Accessibility Evaluation of Educational Institutions Conducting Field Environmental Education Activities in Ecological Protection Areas: A Case Study of Zhuhai City. Sustainability 13 (16), 9392. doi:10.3390/su13169392
Zhou, H. (2006). Discussion and Policy Implication of Psychological Status of Migrant Children. Inst. Popul. Econ.
Keywords: migrant children, compulsory education, the spatial metrology model, influencing factors, multi-source data
Citation: Zhang Z, Luan W, Tian C, Su M and Li Z (2022) Education Equality and Its Influencing Factors for Migrant Children in the Compulsory Education Stage Based on Multi-Source Data: A Case Study of Dalian, China. Front. Earth Sci. 10:950853. doi: 10.3389/feart.2022.950853
Received: 23 May 2022; Accepted: 10 June 2022;
Published: 18 July 2022.
Edited by:
Bing Xue, Institute of Applied Ecology (CAS), ChinaReviewed by:
Dahao Zhang, Sun Yat-sen University, ChinaChenxi Li, Xi’an University of Architecture and Technology, China
Andong Guo, Zhejiang University, China
Copyright © 2022 Zhang, Luan, Tian, Su and Li. This is an open-access article distributed under the terms of the Creative Commons Attribution License (CC BY). The use, distribution or reproduction in other forums is permitted, provided the original author(s) and the copyright owner(s) are credited and that the original publication in this journal is cited, in accordance with accepted academic practice. No use, distribution or reproduction is permitted which does not comply with these terms.
*Correspondence: Weixin Luan, Z3VhbmxsMjAxOUB2aXAuMTYzLmNvbQ==