- 1Key Laboratory of Watershed Geographic Sciences, Nanjing Institute of Geography and Limnology, Chinese Academy of Sciences, Nanjing, China
- 2College of Resources and Environment, University of Chinese Academy of Sciences, Beijing, China
- 3College of Nanjing, University of Chinese Academy of Sciences, Nanjing, China
- 4Suzhou University of Science and Technology, Suzhou, China
- 5China Three Gorges Corporation, Wuhan, China
Investigating the contributions of the factors influencing lake water level and their related changes with regard to hydraulic facilities is vital for understanding the driving mechanism of water level variations under the manifold pressures from anthropogenic activities and climate change. In this study, a random forest (RF) model was used to investigate the changes of the relationship between water level and discharge of the Yangtze River and local tributaries in Poyang Lake, China, based on daily hydrological data from 1980 to 2018. The results indicated that RF exhibited robust capability for water level prediction in Poyang Lake, with average R2 of 0.95, 0.88, 0.92, and 0.94 for the dry, rising, wet, and recession seasons, respectively. Predictor importance analysis showed that the discharge of the Yangtze River had greater influence on the water level than the discharge of local tributaries except for the dry season in Poyang Lake, where the influence on the water level was evident with discharge less than 5,000 m3/s. The influence of the Yangtze River also showed a clear attenuation pattern as the distance from the outlet of the lake increased, where the water level was constantly regulated by the Yangtze River. In addition, the partial dependence plots also indicated that the Yangtze River discharge changes after the TGD operation have resulted in remarkable water level decreases in the wet and recession seasons, especially for the recession period. Meanwhile, a slight increase in water level was predicted under identical discharge of local catchment in the dry season, which was only concentrated in the outlet of the lake. This study indicated the RF model as a robust technique for water level predictions and attribution analysis under multiple temporal and spatial scales. Moreover, this study confirmed the uneven influences of the Yangtze River and local tributaries on water level across different seasons, gauging stations, and phases.
1 Introduction
Water level fluctuations are dominant forces controlling the physical and ecological processes of lake ecosystems (Leira and Cantonati, 2008; Wantzen et al., 2008; Khanal et al., 2021). As the regulation of lake systems for anthropogenic purposes has become increasingly common across the world (Poff and Schmidt, 2016), the amplitude and direction of lake water level fluctuations are undergoing dramatic changes and have led to various water security issues, such as seasonal water shortage, dryness of lake wetland, and eutrophication (Guo et al., 2012; Feng et al., 2013; Jeppesen et al., 2015). Understanding the major influencing factors of lake water level variations could shed light on lake ecosystem management under intensified anthropogenic activities, such as upstream hydraulic facilities construction. However, water level variations may involve complex nonlinear processes integrating inflow from tributaries, lake bathymetry, and blocking the effects of downstream rivers, which is particularly true for floodplain lakes in the Yangtze River basin (e.g., Poyang Lake) in China.
Poyang Lake is the largest freshwater lake in China and is located approximately 1,050 km downstream of the largest dam in the world (Three Gorges Dam, TGD). Poyang Lake, which suffers from great intra- and inter-annual water level fluctuations, provides vital ecosystem services, such as water resources, flood regulation, and nutrient retention (Li et al., 2022). In addition, periodic inundation and exposure to wetlands make the lake a globally important ecoregion for migrating birds and other endangered species (Ramsar Convention, 2012). Poyang Lake receives water from the local catchment and direct rainfall within the lake region, which then drains into the Yangtze River through a narrow channel at the north of the lake. The blocking, emptying effects, and occasional intrusion of the Yangtze River on the lake make the water level variations even more complex at spatial and temporal scales (Hu et al., 2007; Dai et al., 2015; Li Y. et al., 2017). The water level of Poyang Lake was jointly influenced by the discharge of its tributaries and of the downstream Yangtze River (Hu et al., 2007; Zhang et al., 2012). For example, discharge of inflowing rivers and water level in the Yangtze River are adopted as major boundary conditions when establishing hydrodynamic models of Poyang Lake (Li et al., 2019). The rare floods of the Poyang Lake region since the 2000s could be attributed to the decrease in rainfall over the middle and lower Yangtze River basin which caused low streamflow of both the Yangtze River and Poyang Lake basin (Li et al., 2015). The operation of the TGD since 2003 has further altered the interaction between the Yangtze River and Poyang Lake in recent decades, and the associated water level and outflow variations of Poyang Lake have since attracted substantial attention (Guo et al., 2012; Gao et al., 2014; Mei et al., 2015). Evident water level decreases during the TGD impoundment period were reported, which could be largely attributed to the intensified emptying effect of the Yangtze River (Zhang et al., 2012). In addition, concurrent droughts of the lake catchment and the upper Yangtze River in spring, summer and autumn have intensified droughts in the lake through reduction of inflowing discharge since 2003 (Zhang et al., 2017). Zhang et al. (2015) have also indicated that the conjunction of the extreme drought in the upper Yangtze coinciding with the TGD operation was the primary cause of seasonal water shortage in Poyang Lake. Investigations of extensive hydrological data indicated that the Yangtze River discharge has a greater influence on the annual lake water level than the local catchment flow (Ye et al., 2014). Meanwhile, the local catchment discharge was reported to have obvious effects on the water level in the central areas (Zhang et al., 2022). However, the importance of the Yangtze River and the local catchment to the water level of Poyang Lake across different hydrological seasons and lake stations still remains to be elucidated.
Better understanding of the roles of the Yangtze River and local catchment in regulating the spatial and temporal water level variations in Poyang Lake is vital for the attribution analysis of the recent water security problems in the lake (Zhang et al., 2014). Many numeric models were established to investigate the water level variations of Poyang Lake (Li et al., 2018; Yao et al., 2018; Li et al., 2019). Hydrodynamic modeling indicated that the intensified draining effects of the Yangtze River induced by the TGD operation had a much greater impact on the seasonal dryness than the local catchment (Zhang et al., 2014). Yao et al. (2016) reported different contributions of the local catchment and the Yangtze River on the water level in spring and autumn for two typical years using a physical process–based model. Li X. et al. (2016) found that the discharges of both the Yangtze River and local catchment were significant contributors of the flood development and their contributions were uneven in space and time. However, the physical process–based models commonly demand exhaustive data and are time consuming (Zhang et al., 2012). Meanwhile, machine learning techniques, such as the random forest (RF) model, have been proven to have comparable water level prediction accuracy and could provide a measure of predictor importance (Li B. et al., 2016). Despite a “black box,” the RF could show how the predictions partially depend on the selected input predictors and have been widely used to identify the main impact factors in water-related studies (Li B. et al., 2017; Alnahit et al., 2022; He et al., 2022).
In the present study, the RF model was utilized to investigate the importance of the Yangtze River and the local catchment on the water level among different hydrological seasons and lake stations, with special attention to the TGD operation since 2003. The specific objectives of this study are as follows: 1) investigation of spatiotemporal variations of the standardized importance of the Yangtze River and the local catchment through the RF model and 2) clarification of spatiotemporal partial dependence of water level on the discharge of the Yangtze River and local tributaries.
2 Materials and Methods
2.1 Study Area
Poyang Lake (115°47′–116°45′E, 28°22′–29°45′N) is located on the southern bank of the Yangtze River and is one of the two remaining lakes that are freely connected with the Yangtze River in the middle reach of the Yangtze River Basin. The lake receives discharge from five main tributaries, that is, the Gan, Fu, Xiu, Rao, and Xin rivers. After a natural impoundment process, the water drains into the Yangtze River through a narrow waterway in the northern part of the lake (Figure 1). The water level in the Yangtze River greatly influences the outflow capacity of the lake. Specifically, the lake water smoothly flows into the Yangtze River when its water level is lower than in the lake. A block effect, even backflow events, would occur when the water level in the Yangtze River increased, thus impeding the lake water outflow and resulting in the maximum water level in the lake within the year. The water level of Poyang Lake generally follows dry (from December to March), rising (from April to May), wet (from June to September), and recession (from October to November) patterns, with the seasonal water level ranging from less than 8 m to over 20 m (Dai et al., 2015; Yao et al., 2018). The TGD, operating since September of 2003, is located in the upstream of the Yangtze River. The general operating scheme of the TGD is to empty the storage before July to guarantee flood mitigation, to reduce the flood peaks during the flood season, and to impound water from late September to October, which is in accordance with the recession period of the lake. In addition, 27 large reservoirs (volume >1×108 m3) were constructed in the Poyang Lake basin, with a total storage capacity over 17 billion m3 (Xu et al., 2020). However, no evidence was found for the annual streamflow variations induced by these reservoirs (Zhang et al., 2011), the scheming and operation of large reservoirs play a supplementary role in runoff reduction in the wet season and increase in the dry season (Shao et al., 2017). Limited impacts of these reservoirs on water level were reported by a multireservoir optimization model including the TGD and Poyang Lake tributaries (Yang et al., 2021).
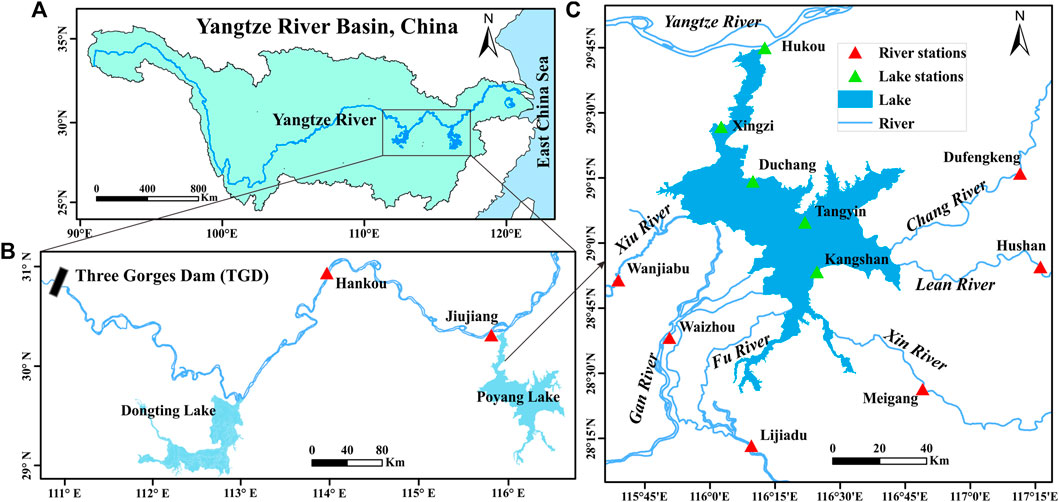
FIGURE 1. Sketch map of study area. (A) location of Poyang Lake in the Yangtze River basin, (B) location of Poyang Lake and the Three Gorges Dam and (C) related hydrological stations in Poyang Lake region.
2.2 Data Source
The combined impacts of the Yangtze River and local catchment on the daily water level in Poyang Lake were considered in this study. Daily discharge observations of the hydrological stations in the lake catchment (i.e., Waizhou, Lijiadu, Meigang, Wanjiabu, Hushan, and Dufengkeng) and in the upstream of Yangtze River (Hankou) from 1980 to 2018 were collected. Notably, Jiujiang station is closer to Poyang Lake than the Hankou station and may be more suitable to represent the effects of the Yangtze River, whereas limited data are available for Jiujiang station. Moreover, discharge in Jiujiang and Hankou showed strong correlation from 1988 to 2018, with a Spearman correlation coefficient of 0.994 (p < 0.001). Therefore, the discharge of Hankou station was adopted in the present study. In addition, the daily water level observations of the five gauging stations within the lake (i.e., Hukou, Xingzi, Duchang, Tangyin, and Kangshan) from 1980 to 2018 were collected accordingly. All data were obtained from and quality controlled by the Hydrological Bureau of Poyang Lake.
2.3 RF Model
The RF model is known as an ensemble machine learning technique used to deal with nonlinear and complex relationships (Breiman, 2001). RF does not require any assumption of input data distribution and overcomes the disadvantages of overfitting and instability through the bootstrap out-of-bag sampling technique and ensemble of decision trees. The basic idea of the RF model is to independently generate a large amount of decision trees on randomly selected variables and subset of training data. The predictions of each tree are averaged to generate a more robust model with high generalizability. The input data were partitioned into an in-bag subset for training and an out-of-bag subset for testing through a bootstrap sampling procedure, the latter of which was not included in the process of creating trees. The sample size of the in-bag and out-of-bag subsets for a single tree is usually set as 2:1 of the input dataset. Partitioning is unique for each tree in the forest and hence provides a significant internal validation. The built-in bootstrap-based out-of-bag sampling technique has been proven to generate an unbiased error rate (Breiman, 2001; Matthew, 2011). Accordingly, no extra testing subsets or cross-validation procedures are needed for the RF model. The three key parameters that should be calibrated in the RF model include the number of the trees in the forest (ntree), the number of randomly selected predictors at each node (mtry), and the minimum number of observations at the terminal nodes of the trees (nodesize). In addition, the RF model provides a nonparametric measure of the importance of each predictor, which is determined by comparing the OOB mean-squared error of the whole forest before and after permuting a predictor (Breiman, 2001). If the permuted predictor has an influence on the response variable, the model accuracy would decrease. Specifically, for the OOB dataset, the permutation importance of the jth predictor is defined as:
where Y is the response observation,
Meanwhile, partial dependence plots were used to depict the functional relationship between the predictors and the responses. Partial dependence provides a quantitative depiction of the dependence of a variable on the response and indicates the effect of each variable on the response variable after considering the average effect of all the other variables in the model (Elith et al., 2008). The partial dependence of the jth predictor could be calculated as follows:
where Xj is the interested predictor in the RF model whose prediction function is
In the present study, seven predictors consisting of discharge from both the Yangtze River and the local catchment were used as input variables for the water level modeling of the five gauging stations in Poyang Lake. The whole dataset was divided into eight subsets according to four seasons (i.e., the dry, rising, wet, and recession seasons) and two phases (i.e., 1980–2002 denoted as “pre the TGD” and 2003–2018 denoted as “aft the TGD”). The RF regression was implemented using multivariate regression in the R-package randomForestSRC for each subset (Ishwaran and Kogalur, 2022). The daily observations of five gauging stations were used as multivariate targets (Multivar function) at the same time to ensure comparability among the different stations (Kourgialas et al., 2015). The optimal mtry and nodesize were calibrated using out-of-bag errors, with mtry ranging from 1 to 7 and nodesize ranging from 1 to 100. The prediction performance on the training and testing subsets provided complementary information for model validation. We used the coefficient of determination (R2) and root mean square error (RMSE) between the observed and the simulated water levels to evaluate the model’s performance. In addition, the joint importance of six hydrological stations from the local tributaries were calculated using the vimp function to make the importance of the Yangtze River and local catchment comparable for each RF model. All important values were standardized by dividing by the variance of the response variables. To further exclude the randomness of the model prediction, 100 model runs were implemented, and the average performance metrics and important values were used. Moreover, the partial dependence of the water level of different seasons and phases on the discharge of the Yangtze River and local catchment were calculated using the gg_partial_coplot function. Notably, the partial dependence of water level on the Yangtze River discharge assumes constant values of the other predictors and vice versa for the local catchment.
3 Results
3.1 RF Model Performance
The optimal parameters of the RF were calibrated according to the out-of-bag error for each model (Figure 2 as a typical example). The prediction error showed a sharp decrease with the number of trees ranging from 0 to 100, and then slowly decreased. The ntree of 500 was thus adopted in the RF models to get the best prediction performance. In addition, the prediction error showed clear response with both the nodesize and mtry. The optimal combinations of mtry and nodesize were thus identified for each model, which are 6–7 and 1, respectively. This procedure was repeated eight times for the RF models of four seasons and two phases to obtain the best prediction performance.
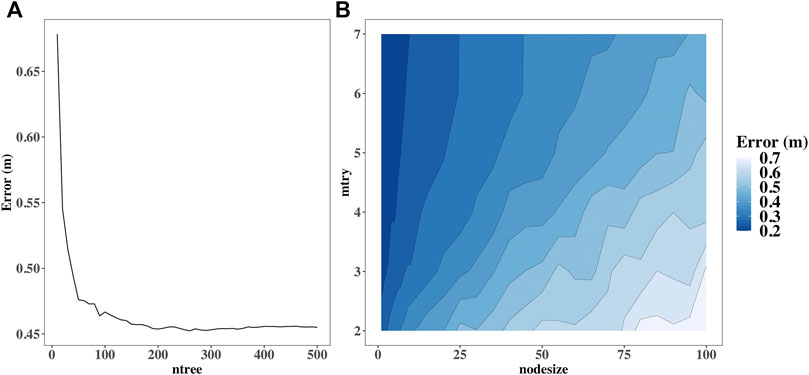
FIGURE 2. Tune of the three key parameters in the RF model. (A) Tune of ntree and (B) tune of nodesize and mtry (A typical example for the model of wet season before the TGD operation).
The RF models were constructed with 500 trees based on the optimal parameters searched using the tune function. Approximately 33.33% of the samples were randomly drawn from the input dataset as the out-of-bag testing sets for a single tree. Figure 3 shows the observed and simulated water levels of the five gauging stations during four seasons for the automatic generated testing sets. The average R2 values of the RF models for the dry, rising, wet, and recession seasons were 0.95, 0.88, 0.92, and 0.94, respectively. Meanwhile, the average RMSEs of the developed RF model for the dry, rising, wet, and recession seasons were 0.32, 0.50, 0.67, and 0.41 m, respectively. These metrics indicated that RF effectively performed in the water level predictions under different seasons. In addition, the average R2 values before and after the TGD phases were 0.90 and 0.92, respectively, denoting a relatively high prediction ability after the TGD operation. A clear pattern of water level changes for lake gauging stations from north to south could be observed in Figure 3, with an increasing water level from the Hukou to the Kangshan station for both phases. Moreover, distinct seasonal water level variations for all the lake gauging stations could also be indicated, with the highest water level in the wet season and lowest water level in the dry season. The comparison of the water level before and after the TGD operation demonstrated a significant decline in the water level for all the lake gauging stations and seasons, especially for the wet and dry seasons (p<0.001). Taking the Hukou station as an example, the average water level during the wet and dry seasons significantly decreased by 1.09 m and 2.11 m, respectively (p<0.001).
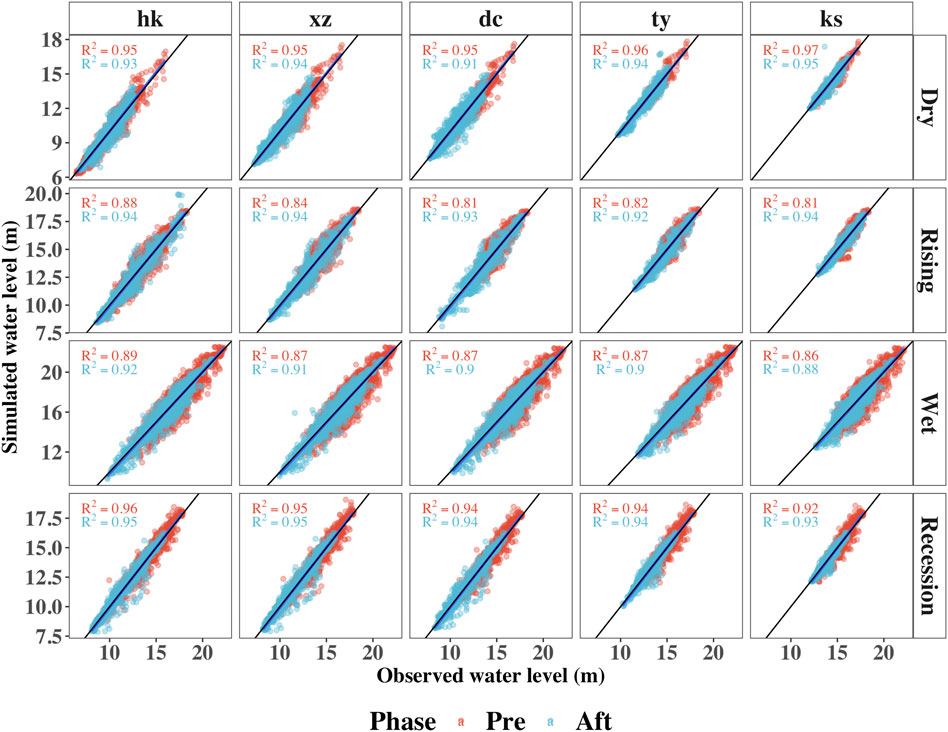
FIGURE 3. Observed and simulated water levels of the five gauging stations in the dry, rising, wet, and recession seasons for the out-of-bag testing sets. The black lines represent line of 1:1.
3.2 Variations of the Importance of the Yangtze River and Local Catchment
Beyond the water level prediction, the RF models also calculate the relative importance of predictors. To make the results comparable, joint importance of the six hydrological stations from the local tributaries were calculated. The average relative importance of both the Yangtze River and local tributaries across the five stations and two phases was compared for four seasons, as shown in Figure 4. The variance of importance analysis from the 100 model runs was relatively stable, indicating robust important analysis results for each prediction procedure. The discharge from the Yangtze River showed strong influences on Poyang Lake water level of all seasons except for the dry season, with the water level of the Hukou station holding the closest relationship with the Yangtze River. In addition, the importance of the Yangtze River gradually decreases from the northern to the southern part of the lake, with the water level of the Kangshan station showing the most limited relationship with the Yangtze River, especially for the dry season. Opposite results could be observed for the importance variations of the local tributaries, which showed increasing trend from the Hukou to the Kangshan station. Moreover, the importance of the Yangtze River on the Poyang Lake water level slightly increases for the wet and recession seasons after the TGD operation, especially for the gauging stations located in the northern part of the lake (i.e., Hukou, Xingzi, and Duchang stations). Notably, the rate of importance increase of the Yangtze River is much larger in the recession season than in other seasons. However, the importance of the local tributaries significantly increases after the TGD operation in the dry season (p<0.01), especially for the gauging stations located in the southern part of the lake (i.e., the Tangyin and Kangshan stations).
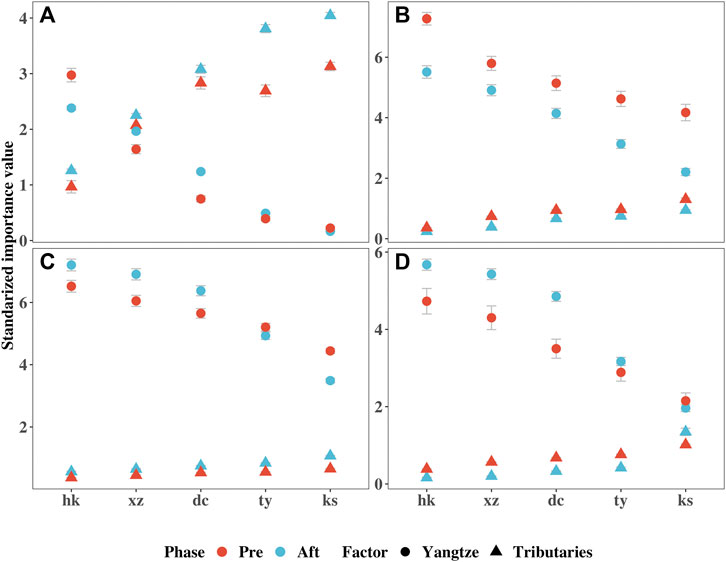
FIGURE 4. Relative importance of the Yangtze River and local tributaries on the water level across the five gauging stations and two phases for the (A) dry, (B) rising, (C) wet, and (D) recession seasons.
3.3 Variations of the Partial Dependence of Water Level Predictions on the Yangtze River and Local Tributaries
A partial dependence curve of one predictor for the water level responses measures the dependence of the water level on this predictor by considering the average effect of all other variables in the model. The partial dependence plots of the Yangtze River discharge and water level across the five gauging stations and four seasons are shown in Figure 5. The general increase of the water level with an increased discharge of the Yangtze River could be observed for all five gauging stations and four seasons. However, the slope of the partial dependence curve showed a pattern of flattening from the northern to southern part of the lake. The relationship between discharge changes in the Yangtze River and water level in the Hukou, Xingzi, and Duchang stations could be seen as convex types, with the linear slope decreasing after the discharge exceeded 30,000 m3/s, whereas the partial dependence curves of Tangyin and Kangshan stations acted as concave types, with the linear slope increasing after the discharge exceeded 30,000 m3/s. These results indicated that the sensitivity of the water level to discharge of the Yangtze River gradually decreases from the Hukou to the Kangshan station. In addition, the water level responses to the discharge changes in the Yangtze River were of lower magnitude in the dry season. Specifically, under the same Yangtze River discharge, the predicted water level was lower in the dry season than that in the other seasons, especially for the gauging stations located in the southern part of the lake. Moreover, no obvious variations of the partial dependence curves could be observed before and after the TGD operation, indicating that the impacts of the discharge from the local tributaries were not significant during the two phases.
The partial dependence plots of the local tributary discharge and water level across the five gauging stations and four seasons are shown in Figure 6. Similarly, the water level exhibited an increasing pattern with the increase in the discharge of the local tributaries. However, clear differences could be observed when compared with the relationships between the Yangtze River and the water level predictions. A close relationship between the discharge from the local tributaries and water level was found with the discharge less than 5,000 m3/s, which showed approximately linear responses, especially for the Tangyin and Kangshan stations. The partial dependence curves remain relatively stable with the further increase in the discharge, indicating that the discharge of the local catchment has limited influences on the water level in Poyang Lake afterward. Distinct seasonal responses could also be observed, with the highest and lowest water levels in the wet and dry seasons under the same discharge conditions, respectively. In addition, in contrast with the Yangtze River impacts, the sensibility of discharge from the local tributaries gradually increases from the northern to southern part of the lake, with the Kangshan station showing the closest responses. Moreover, under the assumptions of the average state of the Yangtze River discharge before and after the TGD operation, the partial dependence curves showed clear decline in the wet and recession seasons after the TGD operation scenarios. This notion indicates that under the equivalent discharge from the local catchment, water level during the wet and recession seasons were greatly impacted by the Yangtze River. This impact also showed a decreasing pattern from the Hukou to the Kangshan stations, as shown by the changes of decline rates of the partial dependence curves for the five gauging stations. Meanwhile, despite the significant water level decrease in the dry season after 2003, which could be attributed to the increasing outflow capacity induced by lake bathymetry changes and the Yangtze River channel incision (Lai et al., 2014a; Yao et al., 2018). The partial dependence plot showed a slight water level increase under identical discharge of local tributaries for the Hukou station after the TGD operation in the dry season, indicating the replenishment discharge in the Yangtze River during this season after the TGD, the effect of which is limited as shown in other stations.
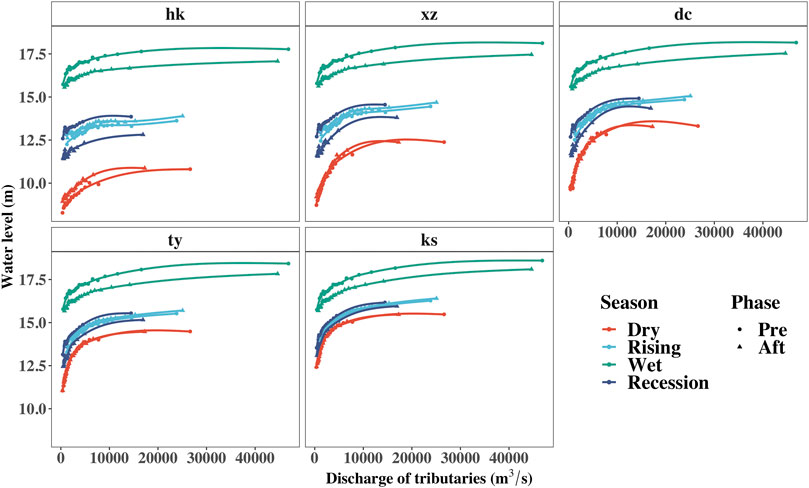
FIGURE 6. Partial dependence plots of the discharge of the local tributaries and predicted water level.
4 Discussion
4.1 Responses of Water Level to Discharge of the Yangtze River and Local Tributaries
The water level generally showed close correlations with the discharges of the Yangtze River and local catchment in Poyang Lake. However, the relative importance of the Yangtze River and local tributaries varied across different seasons, gauging stations, and phases. The results obtained by the importance analysis showed that the discharge in the Yangtze River had stronger influences than the local tributaries except for the dry season, which was further confirmed by the slope variations of the partial dependence plots. The strong influences of the Yangtze River on water level have also been reported by previous research studies using other methods (Lai et al., 2014b; Ye et al., 2014; Li X. et al., 2016). The discharge of the Yangtze River determines whether water could steadily drain into the Yangtze River (Min 1995; Dai et al., 2015). For example, the large discharge of the Yangtze River exerts a strong blocking effect on the lake and can even cause backflow from the Yangtze River to Poyang Lake in the wet season (Li et al., 2015). The slight increase of importance of the Yangtze River in the wet season was found for the northern part of the lake after the TGD operation. This result could be attributed to the weakening of the blocking effect by the Yangtze River induced by climatic anomalies in the upstream of the Yangtze River and TGD operation that further promoted the outflow of the lake (Liu et al., 2013). Meanwhile, the water level showed a strong link with the discharge of the local tributaries only in the dry season, which is further confirmed by the partial dependence plot showing an approximately linear relationship with the local catchment discharge (discharge less than 5,000 m3/s). Moreover, the importance of the local catchment discharge also increased after the TGD operation, especially for the Tangyin and Kangshan stations. This could be possibly attributed to the relatively low discharge and water level, without extreme values intensifying the relationship between them. The obvious importance increase of the Yangtze River was found for the northern lake after the TGD operation in the recession season. This could be largely attributed to the operating scheme of the TGD, which started to impound water from late September. Zhang et al. (2014) demonstrated that the draining effect of the Yangtze River was the primary causal factor of the lake area and volume reduction for the period of 2001–2010, compared to those of 1970–2000. In addition, the importance of the Yangtze River decreased for all five lake stations after the TGD operation. However, no evident explanation could be provided currently, to our knowledge. A speculation of reason would be the difference of model performance before and after the TGD water level predictions (Figure 3).
The influence of the discharge from the Yangtze River decreased with the enlargement of the distance from the Yangtze River and vice versa for the influence of discharge from the local catchment. The water level at the Hukou station exhibited the closest linkage relation with the Yangtze River, which is consistent with previous studies denoting that the water level at the Hukou station is considered to reflect the effects of the Yangtze River (Guo et al., 2012). In addition, the effect of the Yangtze River is larger in the northern area than in the southern part of the lake (Zhang et al., 2022). The hydrodynamic modeling of the Yangtze River-to-lake also demonstrated that the effects of the Yangtze River attenuated with distance from the lake outlet (Li Y. et al., 2017). The results obtained by the partial dependence plots showed that the significant water level decline was predicted for the wet and recession seasons due to the discharge changes in the Yangtze River after the TGD operation in Poyang Lake, whereas no obvious effects were found for the effects of the local tributaries’ discharge changes. Consistent results have been drawn by other scholars using other methods (Min 1995). Huang et al. (2021) revealed that the lake water level is significantly correlated with the TGD operating scheme using the gated recurrent unit method, releasing or blocking of which at certain times could cause large changes in the lake water level. The lowering of flood peaks caused by the TGD impoundment and regulation have reduced the flood risk of Poyang Lake by 7% (Bing et al., 2018). In addition, the remarkable water level decline in the recession was also indicated to be largely attributed to the impoundment of the TGD during this period (Zhang et al., 2012; Zhang et al., 2014). Wang et al. (2019) indicated that the contributions of the TGD operation on the water level decline could reach 59% in early October, which is larger than the effects of the main channel erosion. Meanwhile, regional climate change also contributed to the seasonal dryness of Poyang Lake (Liu et al., 2013; Li et al., 2015). For example, the fewer floods in Poyang Lake since 2003 could be attributed to less rainfall over the local basin (Li et al., 2015). The droughts in the Poyang Lake Basin and upper Yangtze River played a crucial role in the low water level in Poyang Lake in the 2000s (Zhang et al., 2015). Moreover, incision of lake bathymetry in the northern part of the lake induced by sandmining and erosion has resulted in a great increase of the outflow capacity of the lake (Lai et al., 2014a; Yao et al., 2018). In addition, a slight water level increase in response to the discharge of the Yangtze River after the TGD operation was identified only in the dry seasons at the Hukou station, thus indicating the limited replenishment effect of the TGD by recharging the flow of the Yangtze River during this period. This result was also consistent with that of Dai et al. (2021), who reported that the effect of the Yangtze River flow increases during the dry season and diminishes quickly downstream of the dam.
4.2 Source of Uncertainty and Limitation
The RF exhibited generally good performance modeling water level and was comparable with our previous study that also considers previous water level as the model input (Li B. et al., 2016). However, the model performance differed across the spatiotemporal scales, with relatively low R2 values in the rising and wet seasons. The main uncertainty in the water level prediction models may lie in the sample size for the training and input predictors. First, the whole dataset was divided into eight subsets according to seasons and phases to investigate importance variations of the predictors, which will inevitably cause uneven observations for each RF model. Although a large training sample was desired to achieve a better modeling performance (Li B. et al., 2016), the relatively small dataset may cause the model to be undertrained, especially for conditions with extreme water levels. Moreover, the performance is better in the after TGD models than in the before TGD models, which could be attributed to the relatively low water levels without extreme values during the after TGD phase. These results have been indicated by Zhang et al. (2022) that the TGD contributed to reducing the variation amplitude of water levels anywhere in Poyang Lake and controlling the maximum and minimum water levels. Furthermore, this study only incorporated the discharge of the Yangtze River and of the local catchment in the model development processes. The meteorological factors and local ungauged inflows may also have certain influences on the water level. For example, Li et al. (2020) estimated that the ungauged inflows accounts for 12.2% of the yearly inflows through the water balance analysis. However, the study mainly focuses on the relationship between the water level and the discharge from the Yangtze River and local tributaries, which have been commonly recognized as the major contributors of the hydrological variations in Poyang Lake (Zhang et al., 2014; Li X. et al., 2016; Li et al., 2020).
The water level of the five gauging stations for a single model was simultaneously simulated using the multivariate regression. Accordingly, comparisons of the relative importance variations of the Yangtze River and local tributaries on the water level across different gauging stations could be deemed as unbiased. Limitations may also exist when comparing the variations of the relative importance across different seasons and phases, possibly due to the differences in the model structure for each water level prediction model. However, we used a standardized importance measure to efficiently deal with this limitation. The variations of the importance of the Yangtze River before and after the TGD operation are believed to adjoin unbiased but are to be interpreted with caution. Consequently, the partial dependence plots were further used to examine the relationship changes between the water level and the discharge from the external systems after the TGD operation.
5 Conclusion
In this study, the RF model was used to predict the daily water level in Poyang Lake by incorporating the influence of the discharge from the local catchment and the Yangtze River, where the impacts on spatiotemporal water level were further investigated using importance analysis and partial dependence plots. Generally, the RF exhibits a robust capability to predict water level from 1980 to 2018 in Poyang Lake, with average R2 values of 0.95, 0.88, 0.92, and 0.94 for the dry, rising, wet, and recession seasons, respectively. The discharge of the Yangtze River demonstrated overall stronger influences on the water level in all seasons, except for the dry season, when the discharge of the local tributaries showed approximately linear impacts. The influence of the Yangtze River showed a clear attenuation pattern with the distance increase from the outlet of the lake, of which the water level was constantly regulated by the Yangtze River. In addition, the partial dependence plots showed that the Yangtze River discharge changes have resulted in remarkable water level decreases in the wet and recession seasons after the TGD operation, especially for the recession period. The results of the impacts of the Yangtze River and local catchment on the water level obtained by RF modeling showed great consistency with previous studies using physically based modeling, demonstrating that the RF is a robust technique for water level prediction and attribution analysis of multiple temporal and spatial scales.
Data Availability Statement
The original contributions presented in the study are included in the article/Supplementary Material; Further inquiries can be directed to the corresponding authors.
Author Contributions
BL: Conceptualization, methodology, software, formal analysis, visualization, and writing—original draft; GY: Funding acquisition, investigation, supervision, conceptualization, and resources; RW: Resources, investigation, supervision, and writing—reviewing and editing; YW: Methodology, software, and visualization; CX: Visualization; DW: Investigation and funding acquisition; and CM: Writing—reviewing and editing.
Funding
This work was jointly supported by the National Scientific Foundation of China (Grants U2240219 and 42071146) and the Project of China Three Gorges Corporation (201903144).
Conflict of Interest
Authors DW and CM were employed by China Three Gorges Corporation.
The remaining authors declare that the research was conducted in the absence of any commercial or financial relationships that could be construed as a potential conflict of interest.
Publisher’s Note
All claims expressed in this article are solely those of the authors and do not necessarily represent those of their affiliated organizations, or those of the publisher, the editors, and the reviewers. Any product that may be evaluated in this article, or claim that may be made by its manufacturer, is not guaranteed or endorsed by the publisher.
References
Alnahit, A. O., Mishra, A. K., and Khan, A. A. (2022). Stream Water Quality Prediction Using Boosted Regression Tree and Random Forest Models. Stoch. Eev. Res. Risk A, 1–20. doi:10.1007/s00477-021-02152-4
Bing, J., Deng, P., Xiang, Z., Lv, S., Marco, M., and Xiao, Y. (2018). Flood Coincidence Analysis of Poyang Lake and Yangtze River: Risk and Influencing Factors. Stoch. Env. Res. Risk A 32 (4), 879–891. doi:10.1007/s00477-018-1514-4
Dai, X., Wan, R., and Yang, G. (2015). Non-stationary Water-Level Fluctuation in China's Poyang Lake and its Interactions with Yangtze River. J. Geogr. Sci. 25 (3), 274–288. doi:10.1007/s11442-015-1167-x
Dai, X., Yu, Z., Yang, G., Xu, C. Y., and Wan, R. (2021). Investigation of Inner‐basin Variation: Impact of Large Reservoirs on Water Regimes of Downstream Water Bodies. Hydrol. Process. 35 (5), e14241. doi:10.1002/hyp.14241
Elith, J., Leathwick, J. R., and Hastie, T. (2008). A Working Guide to Boosted Regression Trees. J. Anim. Ecol. 77 (4), 802–813. doi:10.1111/j.1365-2656.2008.01390.x
Feng, L., Hu, C., Chen, X., and Zhao, X. (2013). Dramatic Inundation Changes of China's Two Largest Freshwater Lakes Linked to the Three Gorges Dam. Environ. Sci. Technol. 47 (17), 9628–9634. doi:10.1021/es4009618
Gao, J. H., Jia, J., Kettner, A. J., Xing, F., Wang, Y. P., Xu, X. N., et al. (2014). Changes in Water and Sediment Exchange between the Changjiang River and Poyang Lake under Natural and Anthropogenic Conditions, China. Sci. Total Environ. 481, 542–553. doi:10.1016/j.scitotenv.2014.02.087
Guo, H., Hu, Q., Zhang, Q., and Feng, S. (2012). Effects of the Three Gorges Dam on Yangtze River Flow and River Interaction with Poyang Lake, China: 2003-2008. J. Hydrology 416-417, 19–27. doi:10.1016/j.jhydrol.2011.11.027
He, S., Wu, J., Wang, D., and He, X. (2022). Predictive Modeling of Groundwater Nitrate Pollution and Evaluating its Main Impact Factors Using Random Forest. Chemosphere 290, 133388. doi:10.1016/j.chemosphere.2021.133388
Hu, Q., Feng, S., Guo, H., Chen, G., and Jiang, T. (2007). Interactions of the Yangtze River Flow and Hydrologic Processes of the Poyang Lake, China. J. Hydrol. 347 (1-2), 90–100. doi:10.1016/j.jhydrol.2007.09.005
Huang, S., Xia, J., Zeng, S., Wang, Y., and She, D. (2021). Effect of Three Gorges Dam on Poyang Lake Water Level at Daily Scale Based on Machine Learning. J. Geogr. Sci. 31 (11), 1598–1614. doi:10.1007/s11442-021-1913-1
Ishwaran, H., and Kogalur, U. (2022). Fast Unified Random Forests for Survival, Regression, and Classification (RF-SRC). R package version 3.1.0. Available at: https://cran.r-project.org/package=randomForestSRC.
Jeppesen, E., Brucet, S., Naselli-Flores, L., Papastergiadou, E., Stefanidis, K., Nõges, T., et al. (2015). Ecological Impacts of Global Warming and Water Abstraction on Lakes and Reservoirs Due to Changes in Water Level and Related Changes in Salinity. Hydrobiologia 750 (1), 201–227. doi:10.1007/s10750-014-2169-x
Khanal, R., Uk, S., Kodikara, D., Siev, S., and Yoshimura, C. (2021). Impact of Water Level Fluctuation on Sediment and Phosphorous Dynamics in Tonle Sap Lake, cambodia. Water Air Soil Pollut. 232 (4), 1–15. doi:10.1007/s11270-021-05084-5
Kourgialas, N. N., Dokou, Z., and Karatzas, G. P. (2015). Statistical Analysis and ANN Modeling for Predicting Hydrological Extremes under Climate Change Scenarios: The Example of a Small Mediterranean Agro-Watershed. J. Environ. Manag. 154, 86–101. doi:10.1016/j.jenvman.2015.02.034
Lai, X., Huang, Q., Zhang, Y., and Jiang, J. (2014b). Impact of Lake Inflow and the Yangtze River Flow Alterations on Water Levels in Poyang Lake, China. Lake Reserv. Manag. 30 (4), 321–330. doi:10.1080/10402381.2014.928390
Lai, X., Shankman, D., Huber, C., Yesou, H., Huang, Q., and Jiang, J. (2014a). Sand Mining and Increasing Poyang Lake's Discharge Ability: A Reassessment of Causes for Lake Decline in China. J. Hydrology 519, 1698–1706. doi:10.1016/j.jhydrol.2014.09.058
Leira, M., and Cantonati, M. (2008). Effects of Water-Level Fluctuations on Lakes: an Annotated Bibliography. Hydrobiologia 613, 171–184. doi:10.1007/s10750-008-9465-2
Li, B., Yang, G., Wan, R., Dai, X., and Zhang, Y. (2016). Comparison of Random Forests and Other Statistical Methods for the Prediction of Lake Water Level: a Case Study of the Poyang Lake in China. Hydrol. Res. 47 (S1), 69–83. doi:10.2166/nh.2016.264
Li, B., Yang, G., Wan, R., Hörmann, G., Huang, J., Fohrer, N., et al. (2017). Combining Multivariate Statistical Techniques and Random Forests Model to Assess and Diagnose the Trophic Status of Poyang Lake in China. Ecol. Indic. 83, 74–83. doi:10.1016/j.ecolind.2017.07.033
Li, B., Yang, G., Wan, R., Lai, X., and Wagner, P. D. (2022). Impacts of Hydrological Alteration on Ecosystem Services Changes of a Large River-Connected Lake (Poyang Lake), China. J. Environ. Manag. 310, 114750. doi:10.1016/j.jenvman.2022.114750
Li, B., Yang, G., Wan, R., and Li, H. (2018). Hydrodynamic and Water Quality Modeling of a Large Floodplain Lake (Poyang Lake) in China. Environ. Sci. Pollut. Res. 25 (35), 35084–35098. doi:10.1007/s11356-018-3387-y
Li, X., Yao, J., Li, Y., Zhang, Q., and Xu, C.-Y. (2016). A Modeling Study of the Influences of Yangtze River and Local Catchment on the Development of Floods in Poyang Lake, China. Hydrol. Res. 47 (S1), 102–119. doi:10.2166/nh.2016.198
Li, X., Zhang, Q., Xu, C.-Y., and Ye, X. (2015). The Changing Patterns of Floods in Poyang Lake, China: Characteristics and Explanations. Nat. Hazards 76 (1), 651–666. doi:10.1007/s11069-014-1509-5
Li, Y., Zhang, Q., Cai, Y., Tan, Z., Wu, H., Liu, X., et al. (2019). Hydrodynamic Investigation of Surface Hydrological Connectivity and its Effects on the Water Quality of Seasonal Lakes: Insights from a Complex Floodplain Setting (Poyang Lake, China). Sci. Total Environ. 660, 245–259. doi:10.1016/j.scitotenv.2019.01.015
Li, Y., Zhang, Q., Liu, X., and Yao, J. (2020). Water Balance and Flashiness for a Large Floodplain System: A Case Study of Poyang Lake, China. Sci. Total Environ. 710, 135499. doi:10.1016/j.scitotenv.2019.135499
Li, Y., Zhang, Q., Werner, A. D., Yao, J., and Ye, X. (2017). The Influence of River-To-Lake Backflow on the Hydrodynamics of a Large Floodplain Lake System (Poyang Lake, China). Hydrol. Process. 31 (1), 117–132. doi:10.1002/hyp.10979
Liu, Y., Wu, G., and Zhao, X. (2013). Recent Declines in China's Largest Freshwater Lake: Trend or Regime Shift? Environ. Res. Lett. 8 (1), 014010. doi:10.1088/1748-9326/8/1/014010
Matthew, W. (2011). Bias of the Random Forest Out-Of-Bag (OOB) Error for Certain Input Parameters. Open J. Stat. 1 (3), 1–7. doi:10.4236/ojs.2011.13024
Mei, X., Dai, Z., Du, J., and Chen, J. (2015). Linkage between Three Gorges Dam Impacts and the Dramatic Recessions in China's Largest Freshwater Lake, Poyang Lake. Sci. Rep. 5 (1), 18197–18199. doi:10.1038/srep18197
Min, Q. (1995). On the Regularities of Water Level Fluctuations in Poyang Lake. J. Lake Sci. 7 (3), 281–288. (in Chinese). doi:10.18307/1995.0312
Poff, N. L., and Schmidt, J. C. (2016). How Dams Can Go with the Flow. Science 353 (6304), 1099–1100. doi:10.1126/science.aah4926
Ramsar Convention (2012). Report of the Secretary General on the Implementation of the Convention at the Global Level. Gland, Switzerland: Ramsar COP11 DOC, 7.
Shao, W., Chen, X., Zhou, Z., Liu, J., Yan, Z., Chen, S., et al. (2017). Analysis of River Runoff in the Poyang Lake Basin of China: Long-Term Changes and Influencing Factors. Hydrological Sci. J. 62 (4), 575–587. doi:10.1080/02626667.2016.1255745
Wang, D., Zhang, S., Wang, G., Han, Q., Huang, G., Wang, H., et al. (2019). Quantitative Assessment of the Influences of Three Gorges Dam on the Water Level of Poyang Lake, China. Water 11 (7), 1519. doi:10.3390/w11071519
Wantzen, K. M., Rothhaupt, K.-O., Mörtl, M., Cantonati, M., G.-Tóth, L., and Fischer, P. (2008). Ecological Effects of Water-Level Fluctuations in Lakes: an Urgent Issue. Hydrobiologia 613, 1–4. doi:10.1007/s10750-008-9466-1
Xu, D., Lyon, S. W., Mao, J., Dai, H., and Jarsjö, J. (2020). Impacts of Multi-Purpose Reservoir Construction, Land-Use Change and Climate Change on Runoff Characteristics in the Poyang Lake Basin, China. J. Hydrology Regional Stud. 29, 100694. doi:10.1016/j.ejrh.2020.100694
Yang, G., Chen, J., Zhang, Q., and Jiang, X. (2021). River Lake Interactions in the Middle and Lower Yangtze River: Evolution, Effects and Regulation. Beijing: Science Press. (in Chinese).
Yao, J., Zhang, Q., Li, Y., and Li, M. (2016). Hydrological Evidence and Causes of Seasonal Low Water Levels in a Large River-Lake System: Poyang Lake, China. Hydrol. Res. 47 (S1), 24–39. doi:10.2166/nh.2016.044
Yao, J., Zhang, Q., Ye, X., Zhang, D., and Bai, P. (2018). Quantifying the Impact of Bathymetric Changes on the Hydrological Regimes in a Large Floodplain Lake: Poyang Lake. J. Hydrology 561, 711–723. doi:10.1016/j.jhydrol.2018.04.035
Ye, X., Li, Y., Li, X., and Zhang, Q. (2014). Factors Influencing Water Level Changes in China's Largest Freshwater Lake, Poyang Lake, in the Past 50 Years. Water Int. 39 (7), 983–999. doi:10.1080/02508060.2015.986617
Zhang, D., Chen, P., Zhang, Q., and Li, X. (2017). Copula-based Probability of Concurrent Hydrological Drought in the Poyang Lake-Catchment-River System (China) from 1960 to 2013. J. Hydrology 553, 773–784. doi:10.1016/j.jhydrol.2017.08.046
Zhang, Q., Li, L., Wang, Y. G., Werner, A. D., Xin, P., Jiang, T., et al. (2012). Has the Three‐Gorges Dam Made the Poyang Lake Wetlands Wetter and Drier? Geophys. Res. Lett. 39 (20). doi:10.1029/2012gl053431
Zhang, Q., Sun, P., Jiang, T., Tu, X., and Chen, X. (2011). Spatio-temporal Patterns of Hydrological Processes and Their Responses to Human Activities in the Poyang Lake Basin, China. Hydrological Sci. J. 56 (2), 305–318. doi:10.1080/02626667.2011.553615
Zhang, Q., Ye, X.-c., Werner, A. D., Li, Y.-l., Yao, J., Li, X.-h., et al. (2014). An Investigation of Enhanced Recessions in Poyang Lake: Comparison of Yangtze River and Local Catchment Impacts. J. Hydrology 517, 425–434. doi:10.1016/j.jhydrol.2014.05.051
Zhang, Z., Chen, X., Xu, C.-Y., Hong, Y., Hardy, J., and Sun, Z. (2015). Examining the Influence of River-Lake Interaction on the Drought and Water Resources in the Poyang Lake Basin. J. Hydrology 522, 510–521. doi:10.1016/j.jhydrol.2015.01.008
Keywords: water level fluctuations, random forest, partial dependence, Poyang Lake, TGD operation
Citation: Li B, Yang G, Wan R, Wang Y, Xu C, Wang D and Mi C (2022) Unraveling the Importance of the Yangtze River and Local Catchment on Water Level Variations of Poyang Lake (China) After the Three Gorges Dam Operation: Insights From Random Forest Modeling. Front. Earth Sci. 10:927462. doi: 10.3389/feart.2022.927462
Received: 24 April 2022; Accepted: 06 June 2022;
Published: 08 July 2022.
Edited by:
Xijun Lai, Nanjing Institute of Geography and Limnology (CAS), ChinaReviewed by:
Mei Xuefei, East China Normal University, ChinaJingqiao Mao, Hohai University, China
Zengxin Zhang, Hohai University, China
Copyright © 2022 Li, Yang, Wan, Wang, Xu, Wang and Mi. This is an open-access article distributed under the terms of the Creative Commons Attribution License (CC BY). The use, distribution or reproduction in other forums is permitted, provided the original author(s) and the copyright owner(s) are credited and that the original publication in this journal is cited, in accordance with accepted academic practice. No use, distribution or reproduction is permitted which does not comply with these terms.
*Correspondence: Guishan Yang, Z3N5YW5nQG5pZ2xhcy5hYy5jbg==