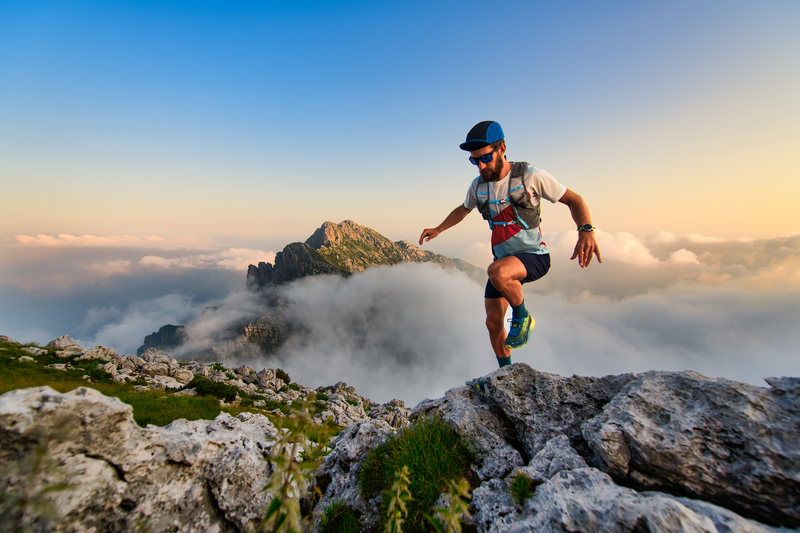
94% of researchers rate our articles as excellent or good
Learn more about the work of our research integrity team to safeguard the quality of each article we publish.
Find out more
BRIEF RESEARCH REPORT article
Front. Earth Sci. , 23 June 2022
Sec. Geohazards and Georisks
Volume 10 - 2022 | https://doi.org/10.3389/feart.2022.908032
This article is part of the Research Topic Deep Rock Mass Engineering: Excavation, Monitoring, and Control View all 22 articles
With the shortage of land resources, there has been a trend toward increasingly deep foundation pit engineering in urban areas. It is extremely important to reflect on the behavior and safety of deep foundation pits and conduct risk assessments in time. A nonhomologous and multi-indicator deep foundation pit risk assessment model was studied for nine types of nonhomologous on-site data monitored in the deep foundation pit. Based on the BIM (Building Information Modeling) technology, a family of monitored points was created that reflects the information on site from a deep foundation pit. The data visualization module was redeveloped by using Visual Studio 2019 to map the on-site data monitored to the components in the BIM model, which can visualize the data monitored in the BIM model of deep foundation pits. On this basis, the assessment of the safety level of deep foundation pits was realized in combination with a risk assessment model. Through the instance of the deep foundation pit of Zibo light rail, the analysis shows that the new visualization and risk assessment method can help construction workers locate dangerous units and formulate corresponding prevention and control measures better and faster.
By the end of 2019, China’s urbanization rate had reached 60.6%. With a large number of people moving into cities, a large amount of infrastructure development is required. However, land resources are becoming increasingly scarce in cities. As a result, there are more and more deep foundation pits in the construction of infrastructure projects. Urban deep foundation pit engineering has two main characteristics: the first is that urban deep foundation pits are often adjacent to complex surrounding environments such as rail transit infrastructure, old buildings, and integrated underground pipelines; the second is the complex geological conditions, such as water-rich soft soil, soil-gravel composite stratum, fault zones, and intrusive rocks. The behavior and impact of urban deep foundation pit engineering on the surrounding environment have been studied by many scholars. Research has been carried out through theoretical calculations, on-site monitored, and numerical simulation (Yuan et al., 2019) on the stability of deep foundation pits during excavation and their impact on the surrounding environment; the results of these studies have guided the safe construction of urban deep foundation pits. The research approach of this study is to map the information of deep foundation pits to BIM through secondary development based on the BIM technology and to further visualize the behavior of deep foundation pits and assess the risk level of foundation pit engineering.
Building information modeling (BIM), is described as a shared digital representation of any built object’s physical and functional properties that serves as a trustworthy basis for decisions (ISO, 2016), has been transforming the architecture, engineering, and construction (AEC) business in many nations (Azhar, 2011). A construction project, in general, entails a number of stages, ranging from planning and design to building and upkeep. Because of its 3D modeling capability, data utilization and modification, and visualization capabilities, BIM may be used as a data management tool at any stage of the process. Practitioners in the AEC business began to use BIM in projects in the mid-2000s. Various studies on technical BIM challenges have been undertaken over the previous decade to increase BIM adoption. BIM research has become more diverse over the previous decade, with more developing technologies being integrated into BIM. For example, BIM can help with 3D printing implementation (Arayici et al., 2012) and has been used in the 3D printing of small-scale models and large-scale buildings (Wu et al., 2016). By combining 3D laser scanning with BIM, (Mahdjoubi et al., 2013) created a model to aid in the delivery of real estate services. (Wang et al., 2013) established a conceptual framework that combines BIM and augmented reality (AR) to allow for real time visualization of the physical context of any construction activity or task. (Tang et al., 2010) investigated strategies for automating the process of recreating as-built building information models from laser-scanned point clouds. (Cerovsek, 2011) reviewed the data exchange standards and features of over 150 AEC/O (Architecture, Engineering, Construction, and Operations) tools and digital models, and offered a framework for improving both BIM tools and schemata. However, limited efforts have been made to research the application of BIM visualization technology to practical engineering.
At present, the application of the BIM technology in the architectural field is mainly in the design stage to conduct top-down design, for detecting pipeline collisions, for engineering calculations, and for visual construction management at the construction stage. These functions are mainly realized by the built-in functions of the BIM software and users only need to learn how to use the software. (Oscar and Zhang, 2021) used the BIM model to analyze architectural design information; (Qi et al., 2021) conducted BIM modeling and UAV aerial oblique photography data collection for Huali Expressway; Furthermore, researchers have connected BIM and the Internet of Things (IoT) so that BIM can display on-site conditions (Lu et al., 2020; Zhao and Liu, 2021), perform a series of functions such as seismic assessment (Chen et al., 2020), and the mutual calling between finite elements (Wen et al., 2020) based on the BIM technology. The aforementioned applications have greatly promoted the digital and informatization development of intelligent engineering activities. With the further promotion and application of BIM, its existing conventional functions cannot serve current engineering requirements. The Construction Standard Regulation [2020] No. 8 issued by the Ministry of Housing and Urban-Rural Development in 2020 pointed out that the integrated application of the BIM technology should be accelerated during the total life cycle of new buildings. The development trend of BIM is to model the behavior of the buildings and perform safety assessments throughout their life cycle (Su et al., 2021). It is necessary to visualize monitored information obtained through sensors in the digital shadow created from a BIM. Meanwhile, it is necessary to further study the safety risk assessment methods and visualize the research results during the total life of the BIM digital shadow. It requires users to gain better capabilities to further develop the software platform. As an emerging technology, the BIM digital shadow technology can collect various data from a physical model in the real world, and map the information to an accurate digital model in the digital space to update the data with the physical model. It can not only achieve modeling but also a simulation of the life cycle. The application of BIM digital shadow for the assessment and visualization of the project’s behavior has rarely been reported.
This study aims to use the BIM digital shadow technology to map the on-site monitoring information to the BIM model of the deep foundation pit in real time, and to make the behavior assessment of the deep foundation pit through the relevant risk assessment model. In this study, an on-site family of monitored points of the deep foundation pits is created in a BIM model, and the Revit software is used to re-develop the data visualization module of the on-site monitored data; a risk assessment model considering conditional information entropy was established and embedded in the visualization development module; this module allows for the visualization of the deep foundation pit’s abnormal units and enables the comprehensive assessment of the risk grade. It has broken through the current application of calculation and collision in BIM and realized the visualization techniques of the deep foundation pits’ behavior, which can better provide support for the safety assessment throughout the construction’s life cycle.
Zibo rail transit station can seamlessly transfer to a high-speed rail station. The embedded section of the station is about 740 m long and 23 m wide. The standard section excavation depth is 16.05–18.2 m and the end well excavation depth is 19.5–20.8 m. The deep foundation pit of the station has been constructed through the open-cut method. The north side of the site is the construction site of the high-speed railway station, and the rest is farmland. Concurrent construction projects around the site include a drop-off platform, a ramped bridge, a square east-west road, a station-overlying steel structure, and a bus station. The safety level of the deep foundation pit is level Ⅰ.
According to the design documents, the standard section of the main deep foundation pit enclosure structure in the field adopts the form of φ800@1000 bored pile plus an internal support enclosure. The station is equipped with three supports along the longitudinal direction and one support replacement is added to the end well. The first support adopts an 800 mm × 800 mm concrete support with a support spacing of about 5.0–9.0 m. The other supports are steel pipe supports with a diameter of 609 mm and a wall with a thickness of 16 mm and a spacing of about 3 m. The foundation pit at the entrance of the underground first floor adopts the structure of a pile plus anchor cable with a horizontal spacing of about 2 m. A double-row pile with a φ800@1000 enclosure structure is adopted near the pile foundation of the high-speed railway station building. The borehole histogram and support design of the standard section are shown in Figure 1.
The lithological composition of the soil layer in the field is complex and the soil layer changes significantly, being cultivated soil, silty clay, silt, fine sand, medium sand, and round gravel soil (Li et al., 2022). According to the stratum distribution characteristics of the site, the permeability of the stratum in the site also varies significantly. The groundwater is slightly low confined (Zhu et al., 2022) and primarily recharged by lateral underground runoff with secondary downward surface water percolation (Wang et al., 2022). The stable groundwater table is at a depth of 19.48–20.34 m and the stable groundwater elevation is 4.93–5.66 m. Judging from the on-site excavation, no obvious groundwater was seen until the foundation pit was excavated to the bottom.
At present, the monitoring of deep foundation pits is carried out by setting on-site monitored points (Wang et al., 2019). During the establishment of the deep foundation pit model in BIM, the monitored points and sensors cannot be displayed in the model (Tong et al., 2019). To reflect the monitored point information in the established BIM digital shadow, it is necessary to load the on-site monitored points and sensor information into BIM in the form of a family of monitored points. It is necessary to create a monitored family of points in the BIM of the deep foundation pit. According to deep foundation pit support design documents and monitored requirements, deep foundation pit monitored information generally includes data on pile deformation, the vertical and horizontal displacement of the pile top, subsidence (Wang et al., 2021), the horizontal and vertical displacement of the slope top, the axial force of the internal support, ground subsidence (Su et al., 2021), groundwater (Wu et al., 2021), and the subsidence of surrounding buildings, pipelines, and roads (Dou et al., 2021), etc. In this study, the family of monitored points was categorized as foundation pit slope top displacement, surrounding building subsidence, road and pipeline subsidence, deep soil displacement, and anchor rod (an anchor cable) axial force. All the aforementioned monitored points are collectively called monitored nails in the BIM. First, the plane coordinates and elevation of the monitored nails were set according to the geometric and spatial relationships of the components in the design drawings. Second, the monitored nails were assigned the material parameters of the different supporting components, and then the definitions were saved as a new monitored nails family. Third, the saved files of each of the families were loaded into the project file and the refined modeling was completed by changing the position of the axis net and the specific values of the elevation. The file of the family of monitored points in the BIM of the foundation pit was created according to the process shown in Figure 2. Based on the family file, the AutoCAD plan of the deep foundation pit supporting structure and the monitored point layout were imported into the Revit software to create a BIM digital shadow model of the deep foundation pit.
Revit provides a packaged API interface. By using Visual Studio 2019 and Revit SDK, the corresponding programming environment was selected to create the function plug-in. The specific steps are as follows:
1. In Visual Studio 2019, the source program of the visualization plug-in was compiled for the early warning alerts of the foundation pit and then files in the *. dll format was generated.
2. The external plug-in loading tool, Addin-In Manager, was added to the Revit software by installing the Revit SDK software. Then, the external plug-in loading tool was used to load the *. dll file and generate the plug-in loading files in the *. addin format.
3. The plug-in installation package was converted to the *.exe format using the Visual Studio 2019 software and the *. dll file and *. addin file were integrated into the plug-in installation package.
4. The created plug-in was installed.
5. The plug-in was used to import the data from MS Excel into the database of the BIM three-dimensional visualization model to provide on-site data support for visualization and risk assessment. The specific implementation path and key technologies of the aforementioned steps are shown in Figure 3.
When monitoring the behavior of the deep foundation pit, the arrangement of all the monitored points is discrete (Liu et al., 2014). When only one of the multiple monitored indicators requires early warning at a certain point, further studies need to be performed to understand how to assess the risk level at the monitored point and categorize the warning level (Zheng et al., 2016). This study proposes a comprehensive assessment method considering information entropy and embeds the assessment model into the assessment module through secondary development to assess the risk of deep foundation pits and to visualize the assessment results. The effect is apparently obvious through on-site observation.
The steps to comprehensively assess the risk grade of deep foundation pit behavior are as follows:
1. For the deep foundation pit to be assessed, the monitored assessment indexes that affect the safety level of the foundation pit need to be determined to classify them.
Each assessment index is classified according to the corresponding foundation pit safety monitored specifications (GB 50911-2013, 2012) and actual conditions. The 9 indexes are shown in Table 1. The result of the safety classification of each index is shown in Table 1 (H is the depth of the foundation pit excavation; F is the prestress control value).
1. Then, we calculate the conditional information entropy, attribute significance, and weight of each assessment index according to Eqs 1, 2, and Eq. 3 to obtain a normalized weight matrix considering information entropy
2. The normalized weight matrix is
Among them,
Then, the normalized weight matrix can be obtained:
3. Then, we identify whether each assessment index is a quantifiable factor and select the corresponding membership function to obtain the membership assessment matrix
The selected assessment indexes are classified based on whether they are quantitative or qualitative. For the qualitative indexes, the Karwowski membership function (Ning et al., 2020) is used for classification while for the quantitative indexes, Eq. 4 is used. To unify the qualitative and quantitative classifications, a five-level classification is adopted, corresponding to the following: normal, basically normal, slightly abnormal, seriously abnormal, and malignantly abnormal.
Among them,
Among the 9 indicators selected, only the groundwater level is not quantified according to the specifications so that qualitative methods are used to assess the membership degree of this index. Combining on-site geological conditions and on-site monitored data, the other indexes are classified according to Eq. 4 and the 9 selected assessment indexes are finally obtained as shown in Table 3.
4. According to Table 3, the membership degree assessment matrix is obtained:
From the second and third steps, the comprehensive assessment matrix of the safety level of the deep foundation pit can be obtained by
5. Combining the principle of the maximum membership degree, the maximum value of the foundation pit safety grade assessment matrix
It is seen in Table 4 that the comprehensive assessment of the foundation pitfalls within the interval (0.8, 0.6], which is relatively safe.
A deep foundation pit model was established in Revit and the secondary development plug-in, including monitored nails, foundation pit monitored, and management modular presented in Section 3 of this study, was loaded. The on-site monitored data was imported into BIM through the plug-in. The abnormal status of the foundation pit’s supporting unit caused by the deformation of the foundation pit due to excavation was displayed on the three-dimensional model after rendering, as shown in brown in Figure 4.
FIGURE 4. Details of the abnormal units and trend diagram of the abnormal units. (A) Indicator details, (B) Indicator change trend.
In the safety risk assessment and early warning interface, the monitored indexes were clicked to get the details of the monitored data; this data can effectively help construction staff locate dangerous units and take timely and correct remedial measures. In the 7th supporting unit of this foundation pit, the steel support axial force, horizontal displacement of the pile top, and displacement of the pile deformation exceed the specified range values, as shown in Figure 4. Clicking the trend on the interface allows one to see the trend of the abnormal unit in the next few days, which can help construction staff identify and analyze possible dangerous situations and take protective measures in time. The reason for the abnormality at the location of the 7th unit of the foundation pit was analyzed on-site. It may be that the inner steel support was not installed in time during the excavation of the foundation pit, resulting in excessive stress on the inner support at the adjacent location. Meanwhile, the vertical cast-in-place pile has no horizontal internal support force to restrict its lateral deformation so that the pile has a corresponding lateral displacement. Although the 7th monitored unit is abnormal, the results of the comprehensive risk assessment of the deep foundation pit based on conditional information entropy proposed in this study show that the foundation pit is in a relatively safe state on the whole. However, construction staff should combine the visualization method and the safe early warning system rendered through the BIM technology to install the internal steel support at the abnormal unit as soon as possible and apply sufficient prestress. In addition, the rate of on-site construction should be reduced, especially the speed of earth excavation, and then restored to a normal speed after the supporting measures have been put in place.
In this study, a comprehensive risk assessment method considering conditional information entropy through secondary development of Revit was developed; this risk assessment method can better facilitate application on-site. The conclusions are as follows:
(1) By establishing a connection between the monitored nails and the on-site monitored points in BIM, the deep foundation pit behavior could be reproduced in real time, and the true meaning of a deep foundation pit’s digital shadow based on the BIM technology was realized.
(2) Using the Revit software, an early warning management plug-in of the deep foundation pit was developed through Visual Studio 2019 and Revit SDK tools. The plug-in could achieve 3D modeling, data import, foundation pit calculation, safety level assessment, and visualization of abnormal units.
(3) The comprehensive assessment method based on conditional information entropy effectively and comprehensively assessed the risk of the monitored area of the deep foundation pit; some alerts were generated by a few individual monitored components; these alerts can effectively help the construction staff to identify and analyze possible dangerous situations and take protective measures in time.
The original contributions presented in the study are included in the article/Supplementary Material; further inquiries can be directed to the corresponding author.
CY was responsible for the original concept. All authors then contributed to the development of the concept as is presented in this manuscript. FC and LL developed software and ran simulations. MY wrote the first draft of the manuscript. CY wrote sections of the manuscript. YH, GY, and JL contributed to the figures. All authors contributed to manuscript revision and have approved the submitted version.
This study was supported by the Natural Science Foundation of Shandong Province (ZR2017MEE017), Key R&D Project of Shandong Province (Public Welfare Projects) (2018GSF120005), and National Natural Science Foundation of China (51174124).
Author JL is employed by China Construction Fifth Engineering Division Corp.
The remaining authors declare that the research was conducted in the absence of any commercial or financial relationships that could be construed as a potential conflict of interest.
All claims expressed in this article are solely those of the authors and do not necessarily represent those of their affiliated organizations, or those of the publisher, the editors, and the reviewers. Any product that may be evaluated in this article, or claim that may be made by its manufacturer, is not guaranteed or endorsed by the publisher.
The authors would like to acknowledge the funding for support. Finally, they would also like to thank the associate editor of this special edition and the reviewers who provided constructive feedback that has, without doubt, improved this manuscript.
Arayici, Y., Egbu, C., and Coates, P. (2012). Building Information Modelling (BIM) Implementation and Remote Construction Projects: Issues, Challenges, and Critiques. J. Inf. Technol. Constr. 17, 75–92.
Azhar, S. (2011). Building Information Modeling (BIM): Trends, Benefits, Risks, and Challenges for the AEC Industry. Leadersh. Manage. Eng. 11 (3), 241–252. doi:10.1061/(asce)lm.1943-5630.0000127
Cerovsek, T. (2011). A Review and Outlook for a 'Building Information Model' (BIM): A Multi-Standpoint Framework for Technological Development. Adv. Eng. Inf. 25 (2), 224–244. doi:10.1016/j.aei.2010.06.003
Chen, P., Shi, J., and Jiang, L. (2020). Research on Evaluation Method of Building Seismic Performance Based on BIM and Ontology. China Civ. Eng. J. 53 (09), 52–59+67. doi:10.15951/j.tmgcxb.2020.09.006
Dou, Z., Tang, S., Zhang, X., Liu, R., Zhuang, C., Wang, J., et al. (2021). Influence of Shear Displacement on Fluid Flow and Solute Transport in a 3D Rough Fracture. Lithosphere 2021, 1569736. doi:10.2113/2021/1569736
GB 50911-2013 (2012). Code for Monitoring Measurement of Urban Rail Transit Engineering (GB 50911-2013). Beijing: China Architecture Publishing & Media Co.,Ltd.
ISO (2016). ISO 29481-1: 2016 Building Information Models - Information Delivery Manual - Part 1: Methodology and Format. Geneva, Switzerland: International Organization for Standardization.
Li, X. S., Li, Q. H., Hu, Y. J., Chen, Q. S., Peng, J., Xie, Y., et al. (2022). Study on Three-Dimensional Dynamic Stability of Open-Pit High Slope under Blasting Vibration. Lithosphere 2022. Article ID 6426550, 17 pages. doi:10.2113/2022/6426550
Liu, J., Ou, X., Zhang, X., Liang, Q., Yang, J., and Liu, X. (2014). Study on Effects of Group Foundation Pits Excavation on Heaving/Settlement of Adjacent Metro Tunnel in Operation. Mod. Tunn. Technol. 51 (4), 81–87. doi:10.13807/j.cnki.mtt.2014.04.012
Lu, Y., Zhuo, Y., Si, J., and Zhang, J. (2020). Research on BIM Construction Application of Gaoligongshan Tunnel on Dali-Ruili Railway. Tunn. Constr. 40 (10), 1516–1524. doi:10.3973/j.issn.2096-4498.2020.10.016
Mahdjoubi, L., Moobela, C., and Laing, R. (2013). Providing Real-Estate Services through the Integration of 3D Laser Scanning and Building Information Modelling. Comput. Industry 64 (9), 1272–1281. doi:10.1016/j.compind.2013.09.003
Ning, X., Li, J., Wang, L., Yang, B., and Wang, X. (2020). Research on Calculation Method of Finite Element Model Transformation Based on BIM Design. Highway 65 (09), 107–113.
Oscar, W. C., and Zhang, J. (2021). Logic Representation and Reasoning for Automated BIM Analysis to Support Automation in Offsite Construction. Automation Constr. 129, 103756. doi:10.1016/j.autcon.2021.103756
Qi, Z., Xu, Y., Wang, Q., and Huang, G. (2021). Application of Monitored Platform Based on BIM and Oblique Photography Technology in Huali Expressway. Highway 66 (06), 284–287.
Su, Y.-q., Gong, F.-q., Luo, S., and Liu, Z.-x. (2021). Experimental Study on Energy Storage and Dissipation Characteristics of Granite under Two-Dimensional Compression with Constant Confining Pressure. J. Cent. South Univ. 28 (3), 848–865. doi:10.1007/s11771-021-4649-2
Tang, P., Huber, D., Akinci, B., Lipman, R., and Lytle, A. (2010). Automatic Reconstruction of As-Built Building Information Models from Laser-Scanned Point Clouds: A Review of Related Techniques. Automation Constr. 19 (7), 829–843. doi:10.1016/j.autcon.2010.06.007
Tong, Y., Yang, M., Kong, X., Liu, S., and Li, Y. (2019). Application of BIM Technology in Deep and Large Foundation Pit and Super High-Rise Projects. Build. Technol. 50 (10), 1265–1268.
Wang, F., Huang, Q., Xu, D., and Tao, Y. (2019). Application and Analysis of BIM Technology in the Whole Process of Building Construction. Eng. Sci. 22, 32–33. doi:10.19537/j.cnki.2096-2789.2019.22.014
Wang, Q., He, M. C., Li, S. C., Jiang, Z. H., Wang, Y., Qin, Q., et al. (2021). Comparative Study of Model Tests on Automatically Formed Roadway and Gob-Side Entry Driving in Deep Coal Mines. Int. J. Min. Sci. Technol. 31 (04), 591–601. doi:10.1016/j.ijmst.2021.04.004
Wang, X., Love, P. E. D., Kim, M. J., Park, C.-S., Sing, C.-P., and Hou, L. (2013). A Conceptual Framework for Integrating Building Information Modeling with Augmented Reality. Automation Constr. 34, 37–44. doi:10.1016/j.autcon.2012.10.012
Wang, Y., Yang, H. N., Han, J. Q., and Zhu, C. (2022). Effect of Rock Bridge Length on Fracture and Damage Modelling in Granite Containing Hole and Fissures under Cyclic Uniaxial Increasing-Amplitude Decreasing-Frequency (CUIADF) Loads. Int. J. Fatigue 158, 106741. doi:10.1016/j.ijfatigue.2022.106741
Wen, Y., Chen, J., and Yang, Y. (2020). Application Research on Lean Project Management of Cross-Bao-Mao Expressway Bridge Based on BIM. Highway 65 (10), 245–252.
Wu, P., Wang, J., and Wang, X. (2016). A Critical Review of the Use of 3-D Printing in the Construction Industry. Automation Constr. 68, 21–31. doi:10.1016/j.autcon.2016.04.005
Wu, Z.-j., Wang, Z.-y., Fan, L.-f., Weng, L., and Liu, Q.-s. (2021). Micro-failure Process and Failure Mechanism of Brittle Rock under Uniaxial Compression Using Continuous Real-Time Wave Velocity Measurement. J. Cent. South Univ. 28 (2), 556–571. doi:10.1007/s11771-021-4621-1
Yuan, C., Yu, H., Yuan, Z., and Wang, Y. (2019). Numerical Simulation of Impact Caused by Construction of High-Rise Building upon Adjacent Tunnels. Geotech. Geol. Eng. 37 (4), 3171–3181. doi:10.1007/s10706-019-00834-z
Zhao, F., and Liu, G. (2021). Research and Application of Prefabricated Assembly Building Information Management Platform Based on BIM and Internet of Things. J. Inf. Technol. Civ. Eng. Archit. 13 (03), 101–106. [2021-07-04]. doi:10.16670/j.cnki.cn11-5823/tu.2021.03.15
Zheng, G., Zhu, H., Liu, X., and Yang, G. (2016). Control of Safety of Deep Excavations and Underground Engineering and its Impact on Surrounding Environment. China Civ. Eng. J. 49 (6), 53–65. doi:10.15951/j.tmgcxb.2016.06.001
Keywords: bim, deep foundation pit, digital shadow, visualization, risk assessment
Citation: Yuan M, Yuan C, Chen F, Li L, Hong Y, Yu G and Lei J (2022) BIM Digital Shadow Technology and Risk Assessment Method of the Deep Foundation Pit’s Behavior for Zibo Light Rail. Front. Earth Sci. 10:908032. doi: 10.3389/feart.2022.908032
Received: 30 March 2022; Accepted: 11 May 2022;
Published: 23 June 2022.
Edited by:
Zhiqiang Yin, Anhui University of Science and Technology, ChinaReviewed by:
Zhigang Tao, China University of Mining and Technology, ChinaCopyright © 2022 Yuan, Yuan, Chen, Li, Hong, Yu and Lei. This is an open-access article distributed under the terms of the Creative Commons Attribution License (CC BY). The use, distribution or reproduction in other forums is permitted, provided the original author(s) and the copyright owner(s) are credited and that the original publication in this journal is cited, in accordance with accepted academic practice. No use, distribution or reproduction is permitted which does not comply with these terms.
*Correspondence: Changfeng Yuan, eXVhbmNoYW5nZmVuZ0BxdXQuZWR1LmNu
Disclaimer: All claims expressed in this article are solely those of the authors and do not necessarily represent those of their affiliated organizations, or those of the publisher, the editors and the reviewers. Any product that may be evaluated in this article or claim that may be made by its manufacturer is not guaranteed or endorsed by the publisher.
Research integrity at Frontiers
Learn more about the work of our research integrity team to safeguard the quality of each article we publish.