- Institute of Physics of the Earth, Russian Academy of Sciences, Moscow, Russia
The global seismic noise, recorded on a network of 229 broadband seismic stations located around the globe for 25 years, from the beginning of 1997 to the end of 2021, has been investigated. To study the noise properties a set of statistics estimated daily have been used: the multifractal singularity spectrum support width, the minimum entropy of the squared wavelet coefficients, and the wavelet-based Donoho-Johnstone (DJ) index. It is shown that the time points of local extrema of the average values of the analyzed noise properties (minima for singularity spectrum support width and DJ-index and maxima for entropy) tend to occur before strong earthquakes. The time interval from the middle of 2002 to the middle of 2003 is determined, when the trend of decreasing the average coherence of the noise properties in the auxiliary network of 50 reference points changed to an increase. Along with an increase in the average coherence, there is an increase in the radius of the spatial maximum coherences of noise properties. Both of these trends continue until the end of 2021, which is interpreted as a general sign of an increase in the degree of criticality of the state of the planet and, as a result, an increase in global seismic danger. After two mega-earthquakes close in time: 27 February 2010, M=8.8 in Chile and 11 March 2011, M=9.1 in Japan, there was an increase in the spatial scales of the strong coherence of noise parameters, which is a sign of an increase in the critical state. The response of seismic noise properties to variations in the length of the day (LOD) has been studied. An estimate of the correlation function between the mean values of the response to LOD and the logarithm of the released seismic energy in a time window of 1 year indicates a delay in energy release with respect to the maxima of the response of noise properties to LOD with a delay time of about 500 days. In connection with this result, an additional intrigue is the extreme increase in the average value of the response to LOD in 2021.
Introduction
In (Kobayashi and Nishida, 1998; Tanimoto, 2001; Rhie and Romanowicz, 2004; Tanimoto, 2005; Aster et al., 2008; Kedar et al., 2008; Nishida et al., 2008; Nishida et al., 2009; Ardhuin et al., 2011) it was shown that the energy source of low-frequency seismic noise is the processes occurring in the ocean and atmosphere. Since the earth’s crust is the propagation medium for seismic waves, changes in the lithosphere are reflected in the statistical properties of the noise. Analysis of changes in these properties makes it possible to evaluate the effects associated with the seismic process occurring in different regions of the Earth and at the global level. Changes of seismic noise spectral parameters in different frequency bands in India during the nationwide COVID-19 lockdown were estimated in (Pandey et al., 2020). Seismic noise in the frequency range 0.5–18 Hz continuously recorded by seismic network in the Central Italy before L’Aquila earthquake, 2009, was investigated in details in (Shi et al., 2020).
The article continues the studies carried out in (Lyubushin, 2014; Lyubushin, 2015; Lyubushin, 2018; Lyubushin, 2020a; Lyubushin, 2020c; Lyubushin, 2021c) to investigate the correlation and coherence properties of low-frequency seismic noise on a global scale covering the entire planet. Since 1997, the total number of broadband seismic stations from various networks has become quite large (229 stations), and their location provides satisfactory coverage of the earth’s surface. This makes it possible to estimate the spatial and temporal correlations of various properties of seismic noise and compare the identified features with the seismic process. The multifractal singularity spectrum support width, the minimum entropy of squared orthogonal wavelet coefficients, and the Donoho-Johnstone index, equal to the ratio of the number of “large” wavelet coefficients to their total number, were considered as main properties of seismic noise. These noise statistics were evaluated for each station in successive time intervals of 1 day. An auxiliary network of 50 reference points is introduced, distributed over the Earth’s surface, for each of which daily time series of median values of seismic noise properties from 5 nearest operational stations were calculated. In a sliding time window with a length of 365 days, the mean moduli of the pairwise correlation coefficients of the daily noise properties between the values at all reference points were estimated. It turned out that the behavior of average correlations for all properties has a common qualitative feature: before 2003, a decrease is observed, but after 2003, a rapid increase in correlation begins. In (Lyubushin, 2020a; Lyubushin, 2020c), it was hypothesized that the break in the average correlation trends in 2003 is due to a high-frequency anomaly in the Earth’s rotation regime, which can also be a trigger for an increase in the intensity of the strongest seismic events after the Sumatran mega-earthquake on 26 December 2004. The kink in the trend of correlations in 2003 is accompanied by a kink in the trends of the values of the seismic noise properties being analyzed: after 2003, the average widths of the carrier of the singularity spectrum support width and DJ-index decrease, and the average values of the noise entropy increase. This behavior of seismic noise properties (simplification of the statistical structure) considered as an indicator of an increase in seismic hazard (Lyubushin, 2018; Lyubushin, 2021a; Lyubushin, 2021b). Thus, the simplification of the statistical structure of seismic noise and the increase in their spatial correlations occur synchronously, and this behavior can be interpreted as an increase in the global seismic hazard.
The methodological basis of the used approach to data analysis is the general property of synchronization of the behavior of the constituent parts of complex systems as they approach critical states (Gilmore, 1981; Nicolis and Prigogine 1989). The goal of all methods used is to search for synchronization effects by estimating the coherence of seismic noise in different regions of the planet.
Seismic Noise Statistics
Let
where
Seismic noise entropy (1) was used in (Lyubushin, 2014; Lyubushin, 2015; Lyubushin, 2017; Lyubushin, 2018; Lyubushin, 2020a; Lyubushin, 2020b; Lyubushin, 2020c; Lyubushin, 2021a; Lyubushin, 2021b; Lyubushin, 2021c) to analyze seismic noise for different regions of the Earth and at the global level as a standalone tool. By its design, entropy (1) is also multiscale, like the entropy proposed in (Costa et al., 2003; Costa et al., 2005) for studying the properties of random signals. In (Koutalonis and Vallianatos, 2017; Vallianatos et al., 2019) the non-extensive entropy of Tsallis was used to analyze seismic noise. The natural time approach to the analysis of random data uses a related definition of entropy in (Varotsos et al., 2003a; Varotsos et al., 2003b; Varotsos et al., 2004; Varotsos et al., 2011).
The noise standard deviation is estimated as the standard deviation of the wavelet coefficients at the first detail level of wavelet decomposition. This estimate must be stable, i.e., insensitive to outliers in the values of the wavelet coefficients at the first level. To do this, we can use a robust median estimate of the standard deviation for a normal random variable:
where
Qualitatively, the multifractal singularity spectrum
Signal is a scale-invariant (Feder, 1988), if
The signal is mono-fractal if
Let’s consider a part of
Let
and the sum:
The value (8) could be regarded as an estimate of
The mass index
Let’s consider estimates of singularity spectrum
Multifractal analysis is an instruments which is used in geophysical studies rather actively (Ramirez-Rojas et al., 2004; Currenti et al., 2005; Ida et al., 2005; Telesca et al., 2005; Chandrasekhar et al., 2016). The multifractality of seismic noise was used for earthquake prediction and seismic hazard assessment in (Lyubushin, 2010; Lyubushin, 2012; Lyubushin, 2013; Lyubushin, 2018; Lyubushin, 2021a). The singularity spectrum support width is used to study the behavior of various nonlinear systems. A decrease in the parameter
First Principal Component
The values of the parameters
Let’s consider a multiple time series
Let
and define scalar time series
Formulae (11-14) are applied independently within each time window. According to these formulae within first time window time series
Hereinafter, a window with a length of 365 days will be used. The choice of this length is quite natural because of presence of annual periodicity in almost all background processes in Earth’s crust. Next, the first principal component is calculated, that is, the projection of all components of the 3-dimensional time series after normalizing them to unit variance onto this eigenvector. In the first time window, the principal component is equal to the projection values for all samples within this window, and for subsequent windows, only one value is taken, corresponding to the rightmost time sample of the window. Thus, outside the first time window, the principal component depends only on past values with a memory depth equal to the length of the window.
It should be noticed that values of seismic noise parameters
Global Seismic Noise Data
The data used are the vertical components of continuous seismic noise records at 1 s sampling intervals, which were downloaded from the Incorporated Research Institutions for Seismology (IRIS) website at http://www.iris.edu/forms/webrequest/from 229 broadband seismic stations of 3 networks: http://www.iris.edu/mda/_GSN, http://www.iris.edu/mda/G, http://www.iris.edu/mda/GE.
Seismic noise records with a sampling rate of 1 Hz (LHZ records) were considered for 25 years of registration (from 1 January, 1997 to 31 December, 2021). These data were converted to 1-min time series by calculating averages for successive 60-s time intervals. Estimates of spectral power densities after coming to 1-min time step were presented in (Lyubushin, 2020c). The analysis is restricted by vertical component only because behaviors of multi-fractal and entropy properties of the noise which are used in the paper are rather typical for all components of seismic noise in the investigated range of periods exceeding 2 min.
Consider an auxiliary network of 50 reference points, which are determined using a hierarchical cluster analysis of the positions of 229 seismic stations using the “far neighbor” method. This method of cluster analysis makes it possible to form compact clusters (Duda et al., 2000). The location of 229 seismic stations and 50 reference points is shown in Figure 1A.
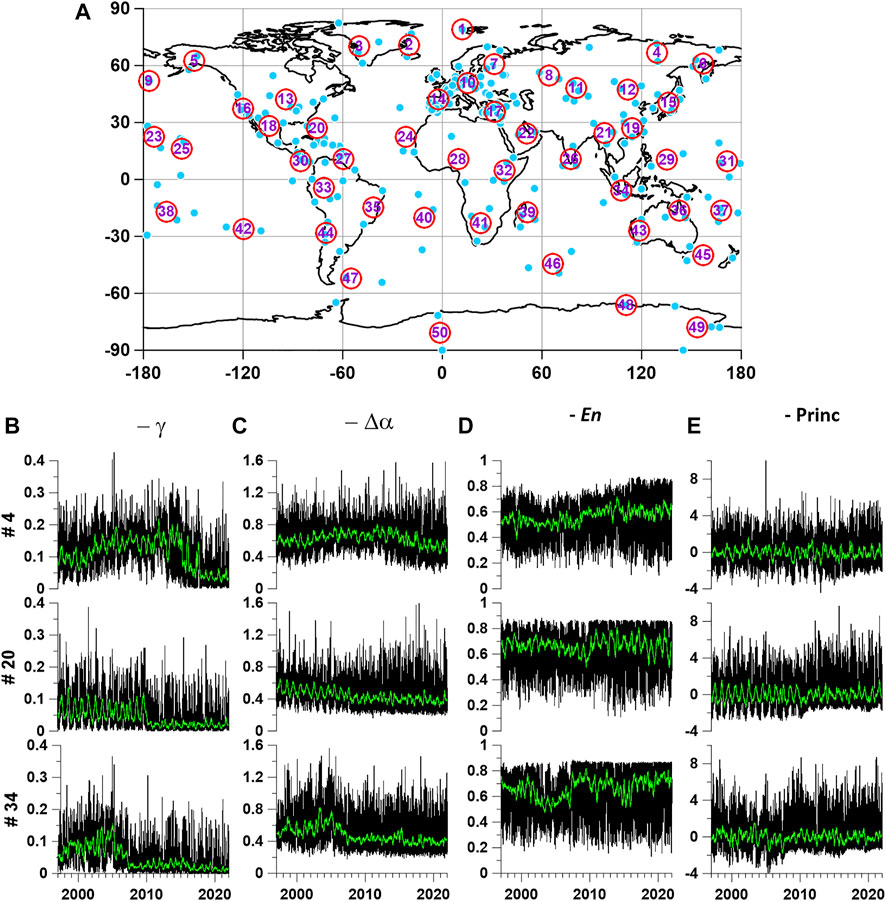
FIGURE 1. (A)—positions of 229 seismic stations (blue circles) and 50 reference points (numbered red circles); (B–D)—plots of daily values of 3 properties of seismic noise
Figures 1B–D show examples of plots of daily property values of
Analysis of the Relationship Between Extremum Points of Noise Properties and Strong Earthquakes
In (Lyubushin, 2010; Lyubushin, 2012; Lyubushin, 2013; Lyubushin, 2014; Lyubushin, 2018; Lyubushin, 2021a; Lyubushin, 2021b), the hypothesis was used that an increase in seismic hazard is preceded by a decrease in the singularity spectrum support width
For the analysis of mutual relationships between point processes, the method of influence matrices (Lyubushin and Pisarenko, 1994), developed to analyze the relationships between several sequences of seismic events from different regions, was used. Further, a simplified version of the model is used for the case of analysis of relationships between two sequences. It can be considered as an analogue of the classical cross-correlation method.
Let
where
According to formula (15), the weight of the event with the number
It is necessary to find the maximum of functions (16) with respect to the parameters
Since the parameters
Substitute the expression
where
- The average value of the influence function. Substituting
where
Function (21) is convex with a negative definite Hessian (Lyubushin and Pisarenko, 1994) and, therefore, problem (21-22) has a unique solution. Having solved this problem numerically for a given relaxation time
The value
Figure 2 shows the results of assessing the elements of the influence matrix (24) to analyze the relationships between local extrema of the average value of the DJ parameter
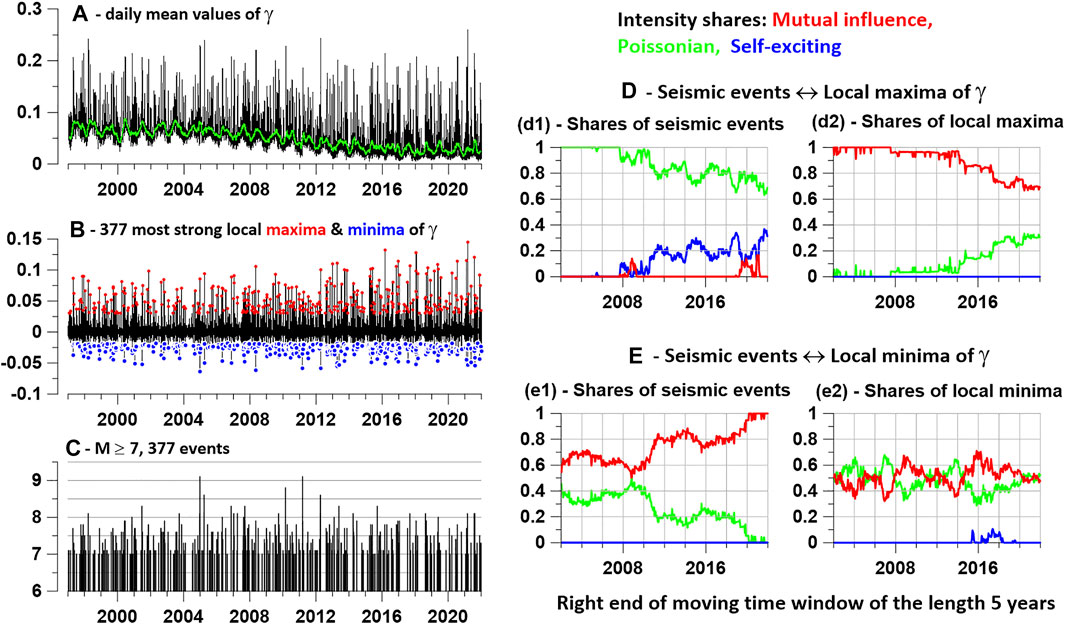
FIGURE 2. (A)—graph of daily average values of the index
Graphs 2 (e1) and 2 (e2) describe changes in intensity shares when considering the interaction of a pair of “seismic events”—“minima
The analysis, the results of which are presented in Figure 2, was carried out for local extrema of the singularity spectrum support width
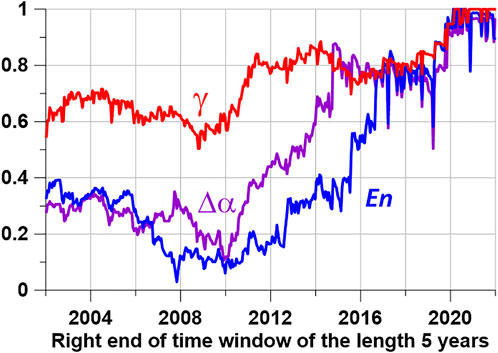
FIGURE 3. Intensity shares corresponding to the “excitation” of seismic events
A notable feature of the graphs in Figure 3 is the simultaneous reaching of the maximum value equal to 1 for all curves describing the “prognostic power” of local extrema of the seismic noise properties under study. Taking into account the fact that the length of the time window is 5 years, the time interval during which the “predictive power” of all 3 properties reached its maximum takes the time interval 2015–2021. At the same time, the DJ-index
Maps of Noise Properties and Distribution of Their Extreme Values
Having values at 50 points distributed around the world makes it possible to map the spatial distribution of seismic noise properties. To build a map, consider a regular grid of 50 nodes in latitude and 100 nodes in longitude, covering the entire earth’s surface. Let
The smoothing radius
Consider the values of the noise property as a function of two-dimensional vectors
Here
Figure 4 shows the average maps of the distribution of 3 properties of seismic noise, obtained by interpolating the values from the reference points to the entire surface of the Earth using formula (15), as well as maps of the probability density distribution of extreme values calculated using formula (16). It can be seen that the distributions of noise property values are strongly correlated with each other. In this case, it should be taken into account that the maxima of
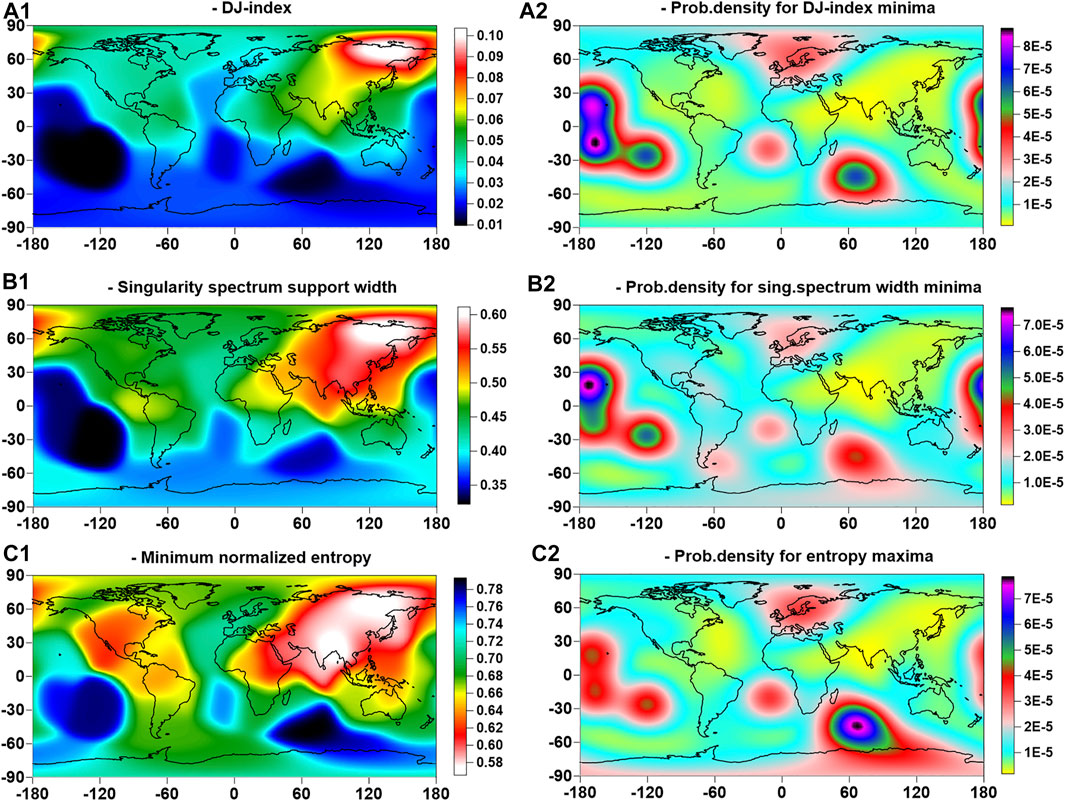
FIGURE 4. On the left—(A1–C1)—` averaged maps of the distribution of seismic noise parameters
Coherence Spectra
For the further analysis it is necessary to estimate coherence spectra between 2 time series within sliding time window. A parametric model of vector autoregression will be used which provides a better frequency resolution with respect to methods of spectra and cross-spectra estimates based on Fourier expansion (Marple, 1987). For the multiple time series
Here
where
Here
First Principal Components at Reference Points and Maximum Coherences
As follows from the distribution maps of noise properties and their extreme values in Figure 4, the behavior of these properties has much in common, but there are also individual differences. To extract the common components of noise properties, we calculate the first principal component of 3 properties
Let
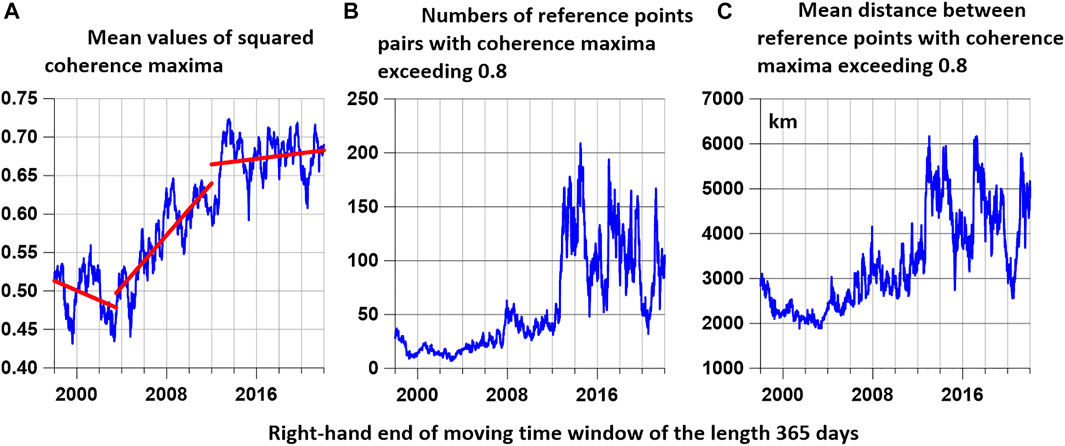
FIGURE 5. (A)—plot of the mean values of the quadratic coherence maxima between the values of the first principal component of the 3 seismic noise properties in all pairs of the network of 50 reference points on the Earth’s surface estimated in a sliding time window of 365 days with a shift of 3 days, the red lines represent linear trends on the time intervals of the right ends of the window 1998–2003.5, 2003.5–2012 and 2012–2022. (B) is a graph of the number of pairs of reference points for which the maximum coherence exceeded the threshold of 0.8. (C) is the graph of mean distance between reference points with high maximum squared coherence.
In the dependence
Mean Correlations Between Seismic Noise Properties
Besides the analysis of first principal components of seismic noise properties, their correlation can also be used to extract the hidden general features of seismic noise behavior. Since the property values are tied to a network of reference points, it becomes possible to calculate at each point the average values of absolute pairwise correlations between daily values
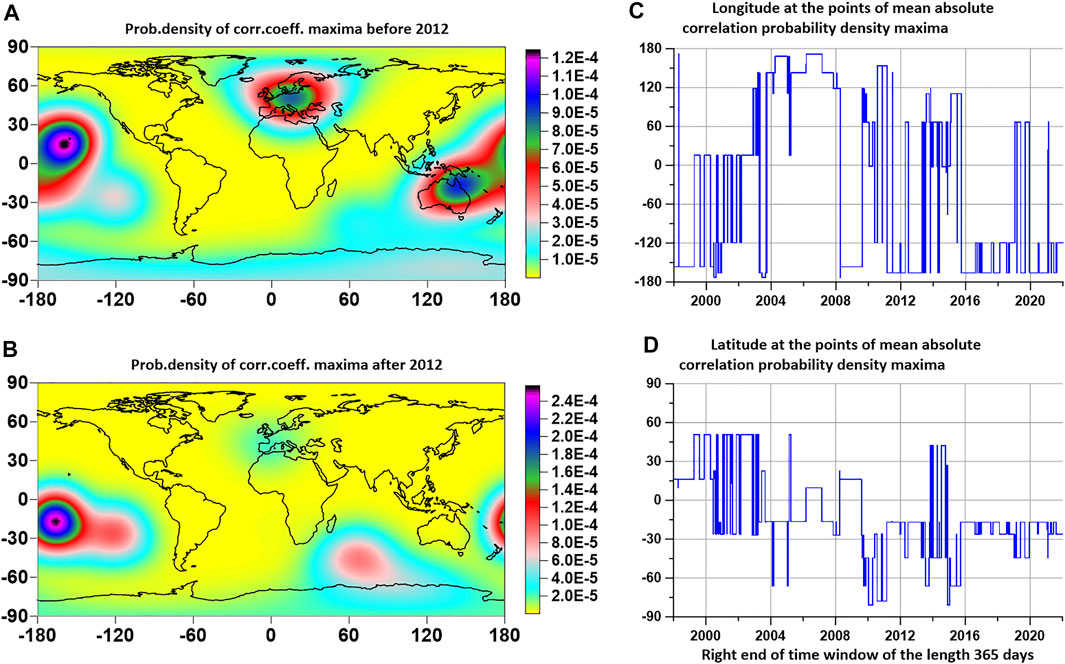
FIGURE 6. (A,B) are maps of probability density distributions of the maxima of the average values of the modules of pairwise correlation coefficients between the values of seismic noise properties
It can be seen from the distribution of maximum correlation values in Figures 6A,B that the maxima are concentrated mainly in the western part of the Pacific Ocean, and after 2012 the points of maximum correlations completely shifted to the Southern Hemisphere, and after 2016 they were mainly concentrated in the region islands of Tonga, where the largest volcanic eruption Hunga Tonga-Hunga Ha’apai occurred on 15 January, 2022 (Poli and Shapiro, 2022).
Relationship Between the Properties of Seismic Noise and Irregular Rotation of the Earth
The uneven rotation of the Earth is mainly explained by the influence of processes in the atmosphere (Zotov et al., 2017). At the same time, some researchers pointed to a connection between the irregular rotation of the Earth and seismicity (Shanker et al., 2001; Levin et al., 2017). A possible trigger mechanism for the influence of Earth rotation variations on the seismic process was studied in (Bendick, and Bilham, 2017). Estimates of the impact of a strong earthquake on the length of the day are given in (Xu and Sun, 2012). Length of day (LOD) data are available from the website at: https://hpiers.obspm.fr/iers/eop/eopc04/eopc04.62-now.
The maximum quadratic coherences between the LOD and the first principal components of the seismic noise properties at each reference point were estimated. The coherences were evaluated in sliding time windows of 365 days with a shift of 3 days using a 5th order vector (two-dimensional) autoregressive model. Similar DJ-index response estimates distributed over the Earth’s surface in a network of reference points were previously used in (Lyubushin, 2021c). Figure 7 shows examples of graphs of the maximum quadratic coherence between the first principal components at three reference points with numbers 4, 20, and 34 (the same as in Figure 1) and LOD.
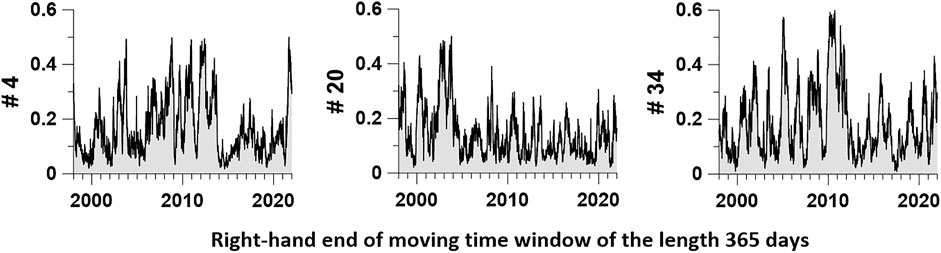
FIGURE 7. Plots of maximum values of quadratic coherences between day length LOD and the first principal component of 3 seismic noise properties
Figure 8 shows graphs of two synchronous curves: Figure 8A shows the logarithm of the released seismic energy (in joules) in a sequence of time intervals 365 days long, taken with a shift of 3 days; Figures 8B—average values of maximum coherences between time series of LOD and daily values of the first components of noise properties at 50 control points. The behavior of the curve in Figure 8B can be divided into 3 sections. The first 2 time stamps of the right ends of the windows before and after 2012 differ significantly from each other in the average values represented by the horizontal red lines. The final section is due to the most recent data (orange line) and it is characterized by abnormal spike. Note that the behavior of the average maximum pairwise coherences exceeding the threshold of 0.8 in Figure 5B is also very different for the timestamps of the right ends of the windows before and after 2012. Thus, the response of seismic noise properties to the irregularity of the Earth’s rotation turned out to be dependent on the degree of spatial connectivity of strong coherences of noise properties.
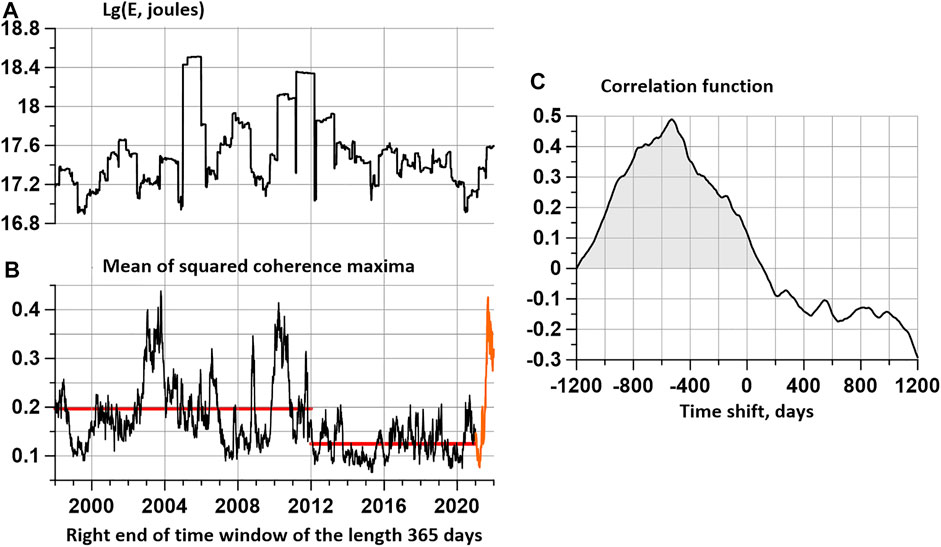
FIGURE 8. (A)—plot of the values of the decimal logarithm of the released seismic energy (joules) in a sliding time window 365 days long with a shift of 3 days; (B)—the black line represents the average of the quadratic coherence maxima between the LOD and the first principal component of the 3 seismic noise properties in a 365-day window with a 3-day offset for the positions of the right end of the time window in the interval 1998–2021, the red horizontal lines represent the average coherence values for time intervals 1998–2012 and 2012–2021. The orange line in Figure (B) represents the plot of the mean coherence for the positions of the right end of the time window in the interval 2021–2022. The plot in Figure (C) represents the correlation function between the logarithm of the released seismic energy and the mean quadratic coherence for time shifts of ±1200 days. A strong shift of the correlation function plot towards negative time shifts with a maximum for a shift of -530 days confirms the hypothesis that bursts of coherence between LOD and seismic noise properties precede seismic energy releases with a delay of about 1.5 years.
The third time interval is represented by the orange line and relates to the processing of the most recent time-stamped right end windows after 2021, then referring to the time span of 2020–2021. The selection of a short 3rd segment based on the results of data processing in 2021 is due to an unusually large burst in the response of noise properties to LOD. An additional intrigue to the occurrence of this burst is introduced by the estimation of the correlation function between the logarithm of the released seismic energy in Figure 8A and the average maximum coherence between the noise and LOD properties in the GCP network in Figure 8B, represented by the plot in Figure 8C) for time shifts of ±1200 days. The correlation function has a significant asymmetry and is shifted to the area of negative time shifts, which correspond to the advance of the coherence maxima of the seismic energy emission maxima. The maximum correlation falls on a time shift of -530 days. This estimate of cross-correlations suggests that the burst represented by the orange lines in Figure 8B may precede a major earthquake with an average delay of 1.5 years.
Although the value of correlation near 0.5 is rather small and it could not be an argument for statistically significant linear connection between 2 random variables, I suppose that main purpose of correlation function estimate is establishing the fact that one variable is shifted with respect to other. The strong asymmetry of correlation function at Figure 8C confirms preceding of coherence burst to burst of seismic energy which could be noticed visually by comparing graphs at Figures 8A,B.
Discussion
The main result of the article is that since 2003 there has been an increase in the global seismic hazard, and in the process of this increase, attention should be paid to the phenomena characteristic of the last annual time windows. First of all, these are graphs of the behavior of the “prognostic forces” of the points of local extrema of the analyzed properties of seismic noise in relation to the sequence of the strongest earthquakes in Figure 3. This figure shows that for all analyzed noise properties, the graphs of their predictive power reached the maximum value equal to 1. The graphs in Figure 3 show that the DJ index
In addition, a strong spike in mean maximum coherence values between the principal components of the seismic noise properties in the network of reference points and the LOD time series represented by the orange line in Figure 8B is a sign of increasing current hazard. Visually, when comparing the graphs in Figures 8A,B, it is noticeable that bursts of coherence precede seismic energy emissions, which is confirmed by the evaluation of the correlation function in Figure 8C.
Summarizing, we can distinguish two critical time intervals when the properties of seismic noise have changed significantly. Considering that the properties of seismic noise are estimated in sliding time windows of 365 days, the critical time intervals are also determined with an accuracy of 1 year.
The first time interval 2002.5–2003.5 refers to the change in the trend of the average maximum coherence between the values of the first components of the noise properties at the reference points. After this interval, a systematic increase in the average maximum coherence is observed (Figure 5A), which is superimposed by periodic fluctuations with a period of about 1000 days. The second critical time interval in the behavior of global seismic noise refers to 2011–2012. After it, chaotic pulsations begin with a high amplitude of change in the number of pairs of reference points and average distances between reference points, for which a strong pairwise coherence has arisen that exceeds the threshold of 0.8 (Figures 5B,C).
Estimating the place of seismic hazard concentration by studying the properties of seismic noise, according to the method used, is reduced to determining the places where extreme values are most often observed, that is, to assessing the spatial distribution densities of the minima for
It should be noticed that the used data analysis technique has a lot of common features with method of investigating properties of high-frequency seismic noise in Central Italy before L’Aquila earthquake in 2009 such as using spectral matrices and entropy of spectral matrices eigenvalues (Shi et al., 2020). The main differences consist in frequency range and in using nonlinear transform from initial seismic records to their properties (wavelet-based minimum entropy, DJ-index and multi-fractal singularity spectrum support width) estimated within adjacent daily time intervals.
Conclusion
In this paper, a phenomenological approach is applied to the study of complex multicomponent systems, which include the earth’s crust, based on the general property of an increase in the radius and degree of strong coherence of random fluctuations in the parameters of a complex system as it approaches a sharp change in its properties, as a result of its own dynamics. As a result of the study of long-term continuous recordings of low-frequency seismic noise on a network of broadband seismic stations covering the entire globe, it was possible to establish characteristic time points for changing trends in the coherence of seismic noise properties and to determine the relationship between changes in these properties and the uneven rotation of the Earth.
Data Availability Statement
Publicly available datasets were analyzed in this study. This data can be found here: Incorporated Research Institutions for Seismology (IRIS) website at http://www.iris.edu/forms/webrequest/from 229 broadband seismic stations of 3 networks: http://www.iris.edu/mda/_GSN, http://www.iris.edu/mda/G, http://www.iris.edu/mda/GE INTERNATIONAL EARTH ROTATION AND REFERENCE SYSTEMS SERVICE, https://hpiers.obspm.fr/iers/eop/eopc04/eopc04.62-now.
Author Contributions
The author confirms being the sole contributor of this work and has approved it for publication.
Conflict of Interest
The author declares that the research was conducted in the absence of any commercial or financial relationships that could be construed as a potential conflict of interest.
Publisher’s Note
All claims expressed in this article are solely those of the authors and do not necessarily represent those of their affiliated organizations, or those of the publisher, the editors and the reviewers. Any product that may be evaluated in this article, or claim that may be made by its manufacturer, is not guaranteed or endorsed by the publisher.
Acknowledgments
The work was carried out within the framework of the state assignment of the Institute of Physics of the Earth of the Russian Academy of Sciences.
References
Ardhuin, F., Stutzmann, E., Schimmel, M., and Mangeney, A. (2011). Ocean Wave Sources of Seismic Noise. J. Geophys. Res. 116, C09004. doi:10.1029/2011jc006952
Aster, R. C., McNamara, D. E., and Bromirski, P. D. (2008). Multidecadal Climate-Induced Variability in Microseisms. Seismol. Res. Lett. 79, 194–202. doi:10.1785/gssrl.79.2.194
Bendick, R., and Bilham, R. (2017). Do weak Global Stresses Synchronize Earthquakes? Geophys. Res. Lett. 44, 8320–8327. doi:10.1002/2017gl074934
Chandrasekhar, E., Prabhudesai, S. S., Seemala, G. K., and Shenvi, N. (2016). Multifractal Detrended Fluctuation Analysis of Ionospheric Total Electron Content Data during Solar Minimum and Maximum. J. Atmos. Sol.-Terr. Phys. 149, 31–39. doi:10.1016/j.jastp.2016.09.007
Costa, M., Goldberger, A. L., and Peng, C. K. (2005). Multiscale Entropy Analysis of Biological Signals. Phys. Rev. E Stat. Nonlin Soft Matter Phys. 71, 021906. doi:10.1103/PhysRevE.71.021906
Costa, M., Peng, C.-K., Goldberger, A. L., and Hausdorff, J. M. (2003). Multiscale Entropy Analysis of Human Gait Dynamics. Phys. A Stat. Mech. its Appl. 330, 53–60. doi:10.1016/j.physa.2003.08.022
Cox, D. R., and Lewis, P. A. W. (1966). The Statistical Analysis of Series of Events. London: Methuen.
Currenti, G., del Negro, C., Lapenna, V., and Telesca, L. (2005). Multifractality in Local Geomagnetic Field at Etna Volcano, Sicily (Southern Italy). Nat. Hazard. Earth Syst. Sci. 5, 555–559. doi:10.5194/nhess-5-555-2005
Donoho, D. L., and Johnstone, I. M. (1995). Adapting to Unknown Smoothness via Wavelet Shrinkage. J. Am. Stat. Assoc. 90 (432), 1200–1224. Available at: http://statweb.stanford.edu/∼imj/WEBLIST/1995/ausws.pdf. doi:10.1080/01621459.1995.10476626
Duda, R. O., Hart, P. E., and Stork, D. G. (2000). Pattern Classification. New York, Chichester, Brisbane, Singapore, Toronto: Wiley-Interscience Publication.
Gilmore, R. (1981). Catastrophe Theory for Scientists and Engineers. New York, NY: John Wiley & Sons, 666.
Hardle, W. (1990). Applied Nonparametric Regression. (Biometric Society Monographs No. 19.). Cambridge: Cambridge University Press.
Ida, Y., Hayakawa, M., Adalev, A., and Gotoh, K. (2005). Multifractal Analysis for the ULF Geomagnetic Data during the 1993 Guam Earthquake. Nonlin. Process. Geophys. 12, 157–162. doi:10.5194/npg-12-157-2005
Ivanov, P. C., Amaral, L. A., Goldberger, A. L., Havlin, S., Rosenblum, M. G., Struzik, Z. R., et al. (1999). Multifractality in Human Heartbeat Dynamics. Nature 399, 461–465. doi:10.1038/20924
Jolliffe, I. T. (1986). Principal Component Analysis. New York, NY: Springer-Verlag. doi:10.1007/b98835
Kantelhardt, J. W., Zschiegner, S. A., Konscienly-Bunde, E., Havlin, S., Bunde, A., and Stanley, H. E. (2002). Multifractal Detrended Fluctuation Analysis of Nonstationary Time Series. Phys. A Stat. Mech. Appl. 316 (1-4), 87–114. doi:10.1016/s0378-4371(02)01383-3
Kedar, S., Longuet-Higgins, M., Webb, F., Graham, N., Clayton, R., and Jones, C. (2008). The Origin of Deep Ocean Microseisms in the North Atlantic Ocean. Proc. R. Soc. A 464, 777–793. doi:10.1098/rspa.2007.0277
Kobayashi, N., and Nishida, K. (1998). Continuous Excitation of Planetary Free Oscillations by Atmospheric Disturbances. Nature 395, 357–360. doi:10.1038/26427
Koutalonis, I., and Vallianatos, F. (2017). Evidence of Non-extensivity in Earth's Ambient Noise. Pure Appl. Geophys. 174, 4369–4378. doi:10.1007/s00024-017-1669-9
Levin, B. W., Sasorova, E. V., Steblov, G. M., Domanski, A. V., Prytkov, A. S., and Tsyba, E. N. (2017). Variations of the Earth's Rotation Rate and Cyclic Processes in Geodynamics. Geodesy Geodyn. 8 (3), 206–212. doi:10.1016/j.geog.2017.03.007
Lyubushin, A. A. (2014). Analysis of Coherence in Global Seismic Noise for 1997-2012. Izv. Phys. Solid Earth 50 (3), 325–333. doi:10.1134/s1069351314030069
Lyubushin, A. A. (2017). Long-Range Coherence between Seismic Noise Properties in Japan and California before and after Tohoku Mega-Earthquake. Acta Geod. Geophys 52, 467–478. doi:10.1007/s40328-016-0181-5
Lyubushin, A. A., and Pisarenko, V. F. (1994). Research on Seismic Regime Using Linear Model of Intensity of Interacting Point Processes. Izv. Phys. Solid Earth 29, 1108–1113.
Lyubushin, A. A. (2015). Wavelet-Based Coherence Measures of Global Seismic Noise Properties. J. Seismol. 19 (2), 329–340. doi:10.1007/s10950-014-9468-6
Lyubushin, A. (2020b). Connection of Seismic Noise Properties in Japan and California with Irregularity of Earth's Rotation. Pure Appl. Geophys. 177, 4677–4689. doi:10.1007/s00024-020-02526-9
Lyubushin, A. (2020c). Global Seismic Noise Entropy. Front. Earth Sci. 8, 611663. doi:10.3389/feart.2020.611663
Lyubushin, A. (2021c). Global Seismic Noise Wavelet-Based Measure of Nonstationarity. Pure Appl. Geophys. 178, 3397–3413. doi:10.1007/s00024-021-02850-8
Lyubushin, A. (2013). How Soon Would the Next Mega-Earthquake Occur in Japan? Nat. Sci. 5 (8A1), 1–7. doi:10.4236/ns.2013.58A1001
Lyubushin, A. (2021b). Low-Frequency Seismic Noise Properties in the Japanese Islands. Entropy 23, 474. doi:10.3390/e23040474
Lyubushin, A. (2010). “Multifractal Parameters of Low-Frequency Microseisms,” in Synchronization and Triggering: From Fracture to Earthquake Processes. GeoPlanet: Earth and Planetary Sciences 1. Editors V. de Rubeis, Z. Czechowski, and R. Teisseyre (Berlin Heidelberg: Springer-Verlag), 253–272. Chapter 15. doi:10.1007/978-3-642-12300-9_15
Lyubushin, A. (2012). Prognostic Properties of Low-Frequency Seismic Noise. Nat. Sci. 04, 659–666. doi:10.4236/ns.2012.428087
Lyubushin, A. (2021a). Seismic Noise Wavelet-Based Entropy in Southern California. J. Seismol. 25, 25–39. doi:10.1007/s10950-020-09950-3
Lyubushin, A. (2018). “Synchronization of Geophysical Field Fluctuations,” in Complexity of Seismic Time Series: Measurement and Applications. Editors T. Chelidze, L. Telesca, and F. Vallianatos (Amsterdam, Oxford, Cambridge: Elsevier 2018), 161–197. Chapter 6. doi:10.1016/b978-0-12-813138-1.00006-7
Lyubushin, A. (2020a). Trends of Global Seismic Noise Properties in Connection to Irregularity of Earth's Rotation. Pure Appl. Geophys. 177, 621–636. doi:10.1007/s00024-019-02331-z
Mallat, S. (1999). A Wavelet Tour of Signal Processing. Second edition. San Diego, London, Boston, New York, Sydney, Tokyo, Toronto: Academic Press.
Marple, S. L. (1987). Digital Spectral Analysis with Applications. Englewood Cliffs, New Jersey: Prentice-Hall.
Nicolis, G., and Prigogine, I. (1989). Exploring Complexity, An Introduction. New York, NY: W.H. Freedman and Co., 328.
Nishida, K., Kawakatsu, H., Fukao, Y., and Obara, K. (2008). Background Love and Rayleigh Waves Simultaneously Generated at the Pacific Ocean Floors. Geophys. Res. Lett. 35, L16307. doi:10.1029/2008gl034753
Nishida, K., Montagner, J.-P., and Kawakatsu, H. (2009). Global Surface Wave Tomography Using Seismic Hum. Science 326 (5949), 112. doi:10.1126/science.1176389
Pandey, A. P., Singh, A. P., Bansal, B. K., Suresh, G., and Prajapati, S. K. (2020). Appraisal of Seismic Noise Scenario at National Seismological Network of India in COVID-19 Lockdown Situation. Geomatics Nat. Hazards Risk 111, 2095–2122. doi:10.1080/19475705.2020.1830187
Pavlov, A. N., and Anishchenko, V. S. (2007). Multifractal Analysis of Complex Signals. Phys. Usp. 50 (8), 819–834. doi:10.1070/pu2007v050n08abeh006116
Poli, P., and Shapiro, N. M. (2022). Rapid Characterization of Large Volcanic Eruptions: Measuring the Impulse of the Hunga Tonga Ha’apai Explosion from Teleseismic Waves. Geophys. Res. Lett. 49 (8), e2022GL098123. doi:10.1029/2022GL098123
Ramírez-Rojas, A., Muñoz-Diosdado, A., Pavía-Miller, C. G., and Angulo-Brown, F. (2004). Spectral and Multifractal Study of Electroseismic Time Series Associated to the Mw=6.5 Earthquake of 24 October 1993 in Mexico. Nat. Hazards Earth Syst. Sci. 4, 703–709. doi:10.5194/nhess-4-703-2004
Rhie, J., and Romanowicz, B. (2004). Excitation of Earth's Continuous Free Oscillations by Atmosphere-Ocean-Seafloor Coupling. Nature 431, 552–556. doi:10.1038/nature02942
Sarlis, N. V., Skordas, E. S., Varotsos, P. A., Nagao, T., Kamogawa, M., and Uyeda, S. (2015). Spatiotemporal Variations of Seismicity before Major Earthquakes in the Japanese Area and Their Relation with the Epicentral Locations. Proc. Natl. Acad. Sci. U. S. A. 112, 986–989. doi:10.1073/pnas.1422893112
Shanker, D., Kapur, N., and Singh, V. P. (2001). On the Spatio Temporal Distribution of Global Seismicity and Rotation of the Earth - A Review. Acta Geod. Geoph. Hung. 36, 175–187. doi:10.1556/ageod.36.2001.2.5
Shi, P., Seydoux, L., and Poli, P. (2020). Unsupervised Learning of Seismic Wavefield Features: Clustering Continuous Array Seismic Data during the 2009 L’Aquila Earthquake. J. Geophys. Res. Solid Earth 126, e2020JB020506. doi:10.1029/2020JB020506
Tanimoto, T. (2001). Continuous Free Oscillations: Atmosphere-Solid Earth Coupling. Annu. Rev. Earth Planet. Sci. 29, 563–584. doi:10.1146/annurev.earth.29.1.563
Tanimoto, T. (2005). The Oceanic Excitation Hypothesis for the Continuous Oscillations of the Earth. Geophys. J. Int. 160, 276–288. doi:10.1111/j.1365-246X.2004.02484.x
Telesca, L., Colangelo, G., and Lapenna, V. (2005). Multifractal Variability in Geoelectrical Signals and Correlations with Seismicity: A Study Case in Southern Italy. Nat. Hazards Earth Syst. Sci. 5, 673–677. doi:10.5194/nhess-5-673-2005
Vallianatos, F., Koutalonis, I., and Chatzopoulos, G. (2019). Evidence of Tsallis Entropy Signature on Medicane Induced Ambient Seismic Signals. Phys. A Stat. Mech. Appl. 520, 35–43. doi:10.1016/j.physa.2018.12.045
Varotsos, P. A., Sarlis, N. V., and Skordas, E. S. (2003b). Attempt to Distinguish Electric Signals of a Dichotomous Nature. Phys. Rev. E Stat. Nonlin Soft Matter Phys. 68, 031106. doi:10.1103/PhysRevE.68.031106
Varotsos, P. A., Sarlis, N. V., Skordas, E. S., and Lazaridou, M. S. (2004). Entropy in the Natural Time Domain. Phys. Rev. E 70 (10), 011106. doi:10.1103/PhysRevE.70.011106
Varotsos, P. A., Sarlis, N. V., and Skordas, E. S. (2003a). Long-Range Correlations in the Electric Signals that Precede Rupture: Further Investigations. Phys. Rev. E Stat. Nonlin Soft Matter Phys. 67, 021109. doi:10.1103/PhysRevE.67.021109
Varotsos, P. A., Sarlis, N. V., and Skordas, E. S. (2011). “Natural Time Analysis: The New View of Time,” in Precursory Seismic Electric Signals, Earthquakes and Other Complex Time Series (Berlin Heidelberg: Springer-Verlag). doi:10.1007/978-3-642-16449-1
Varotsos, P. A., Sarlis, N. V., and Skordas, E. S. (2014). Study of the Temporal Correlations in the Magnitude Time Series before Major Earthquakes in Japan. J. Geophys. Res. Space Phys. 119, 9192–9206. doi:10.1002/2014ja020580
Xu, C., and Sun, W. (2012). Co-Seismic Earth's Rotation Change Caused by the 2012 Sumatra Earthquake. Geodesy Geodyn. 3 (4), 28–31. doi:10.3724/SP.J.1246.2012.00028
Keywords: seismic noise, global seismicity, wavelet-based entropy, DJ-index, singularity spectrum, length of day, coherence
Citation: Lyubushin A (2022) Investigation of the Global Seismic Noise Properties in Connection to Strong Earthquakes. Front. Earth Sci. 10:905663. doi: 10.3389/feart.2022.905663
Received: 27 March 2022; Accepted: 13 June 2022;
Published: 05 July 2022.
Edited by:
Stelios M. Potirakis, University of West Attica, GreeceReviewed by:
A. P. Singh, Ministry of Earth Sciences, IndiaPiero Poli, Université Grenoble Alpes, France
Copyright © 2022 Lyubushin. This is an open-access article distributed under the terms of the Creative Commons Attribution License (CC BY). The use, distribution or reproduction in other forums is permitted, provided the original author(s) and the copyright owner(s) are credited and that the original publication in this journal is cited, in accordance with accepted academic practice. No use, distribution or reproduction is permitted which does not comply with these terms.
*Correspondence: Alexey Lyubushin, bHl1YnVzaGluQHlhbmRleC5ydQ==