- 1School of Earth Science, Chengdu University of Technology, Chengdu, China
- 2Institute of Exploration Technology, Chinese Academy of Geological Sciences, Chengdu, China
Landslides pose huge challenges to the economic activities in mountainous areas at present, while large numbers of landslide disasters have developed in the Hengduan Mountains area in the eastern part of the Tibetan Plateau. Accurate landslide susceptibility mapping (LSM) serves as a critical measure to predict the serious risks that may be encountered in engineering activities. However, previous landslide susceptibility assessment can only play a limited role in the real-time analysis of current activities of slopes. In this study, the deformation rates of the slopes along the Lancang River were determined using the SBAS-InSAR technique. Meanwhile, the landslide susceptibility along the north Lancang River was assessed using the frequency ratio (FR), random forest and FR-RF models, and the precision of the assessment results was verified according to receiver operating characteristic curves (ROCs). Finally, a refined landslide susceptibility map was developed by integrating the deformation rates and landslide susceptibility indexes (LSIs) using a contingency matrix. As indicated by the deformation rates calculated using the SBAS-InSAR technique according to ascending and descending data show that the RADARSAT-2 descending data yielded more precise deformation results. The area-under-the-curve (AUC) values of the three assessment models were 0.866, 0.897, and 0.916, respectively, indicating that the assessment results obtained with the FR-RF model are the most precise. In the upgraded landslide susceptibility map, the areas with high and very high landslide susceptibility increased by 2.97%. Meanwhile, a total of 563,430 grid cells showed an increase in landslide susceptibility, accounting for 11.15% of all the grid cells. Most especially, the Xueru and Ritong areas exhibited a significant increase in landslide susceptibility, and it has been verified by remote sensing images and field surveys that both areas are subject to landslide risks. Therefore, the upgraded landslide susceptibility map has a better prediction performance and can provide valuable support for the decision making in the construction of major engineering facilities and the prevention and remediation of landslides.
1 Introduction
Landslides are the major challenges of the economic activities in mountainous areas at present. In many countries, landslides have a greater influence on the society and economy than any other natural disasters (Glade 1998; Wallemacq et al., 2018) and have seriously affected the operation and management of engineering facilities of humankind (Turner 2018; Wei et al., 2018; Celik et al., 2021). In China, the most severe landslides occur in mountainous areas and plateaus (Shi. 2016), which will become more notable with the aggravation of climate change (Turner 2018; Pánek 2019). The Hengduan Mountains area in the eastern part of the Tibetan Plateau (where Jinsha, Lancang, and Nujiang rivers flow together) features high mountains and canyons, large numbers of loose cuttings, and sudden heavy rainfall. As a result, a series of serious and frequent geo-hazards have struck the area, such as rock collapse, high-elevation landslides, glacier-related debris flows, cutting creep, soil creep, and rate avalanches (Dai and Deng 2020; Zhang et al., 2011; Chen et al., 2013; Wang et al., 2017; Dai and Deng 2020; Lyu et al., 2021; Hu et al., 2021). In 2018, the landslide occurring in Baige village even directly blocked the Jinsha River and broke the ridge over the river, causing huge economic losses for the lower reaches (Xiong et al., 2020; An et al., 2021). All these geo-hazards have greatly damaged the engineering facilities of humankind and the surrounding environment. The Sichuan-Tibet Railway is a major railway project under planning in China, along which the terrain, landform, and geological tectonic conditions are extremely complex and landslides and other geo-hazards are extremely developed (Lu and Cai, 2019; Guo et al., 2021). Therefore, it is highly important to assess the landslide susceptibility along the major rivers around the Tibetan Plateau, especially the susceptibility to geo-hazards at the upper and lower reaches of the railway. LSM is used to describe the spatial distribution of the occurrence probabilities of landslides in a certain area according to the geographical environment and is considered a common countermeasure against the effects of landslides (Huang and Zhao 2018; Merghadi et al., 2020). To obtain more accurate LSM, this study employed the FR, RF, and FR-RF models to assess the landslide susceptibility along the north Lancang River where the Sichuan-Tibet railway passes. However, since traditional assessment models take historical landslide data as input, inaccurate historical landslide data tend to cause classification errors in LSM. Therefore, a method is required to improve the reliability of LSM.
The interferometric synthetic aperture radar (InSAR) technique serves as a reliable way to improve the identification and monitoring of landslides. Most especially, it can provide valuable information on the current activity of regional landslides (Dong et al., 2017; Introeri et al., 2017; Zhang et al., 2021). It has attracted increasing attention and has been applied to landslide identification (Bürgmann et al., 2000; Rott and Nagler,2006; Altamira 2017; Yao et al., 2017; Ge et al., 2019; Xu et al., 2019) and establish optimized landslide assessment models (Ciampalini et al., 2016; Zhao et al., 2019; Dimitris and Skevi Perdikou 2021) in the past 20 years. However, this method has not been used in the big rivers along the Sichuan-Tibet Railway, where the high-locality slope deformation information is very important, for the reason that the steep terrain is more prominent. In addition, the previous research always used one InSAR data source along the north Lancang River (Yao et al., 2020), but for the canyon area, multi-source data of ascending and descending orbits are very necessary, which is the advantages of this study compared with previous studies.
On-site mapping and monitoring of landslide susceptibility are complex and time-consuming and involve the collection and analysis of field data. Therefore, it is considered being impractical to obtain the latest changes of landslides through regular and repeated field surveys, especially on a large scale. Given this, the small baseline subset interferometric synthetic aperture radar (SBAS-InSAR) was used in this study to obtain the activity of slopes along the Lancang River, which is an indicator for susceptibility assessment. This technique can overcome the limitation of temporal incoherence and avoid the decoherence induced by too large intervals of SAR data, spatial incoherence, and atmospheric effects of traditional interferometry, thus producing more continuous spatial-temporal deformation results of lands (Berardino et al., 2002; Lanari et al., 2004). The SBAS-InSAR technique is applicable to the monitoring of long-sequence slow linear and nonlinear deformation and has been widely used in the identification and monitoring of land subsidence, earthquakes, active faults, and slope instability such as creep and landslides (Lanari et al., 2007; Qu et al., 2013; Chen et al., 2018; Li et al., 2018; Zhang et al., 2018).
To sum up, this study assessed the application of LSM in the north Lancang River using the FR, RF, and FR-RF models. Then it verified the accuracy of assessment results according to the AUC values of ROC curves and selected the most accurate assessment results for LSI refining. Specifically, the LSI was refined according to the deformation results of the study area from 2018 to 2020 obtained by the SBAS-InSAR method. As a result, the upgraded LSM can provide more reliable bases for the construction of major projects and the prevention and remediation of landslides.
2 Study Area
The Sichuan-Tibet Railway and the Lancang River—one of the main rivers in a north-south direction in the Hengduan Mountains area—intersect in Changdu City, Tibet Autonomous Region. The study area spans 160 km in the north-south direction between the upper and lower reaches of the Lancang River section of the Sichuan-Tibet Railway and 10 km in the east-west direction around the two banks of the main stream of the Lancang River, which is the most intensive area of human activity (Figure 1). It is located in the middle-upper reach of the Lancang River and lies in the Changdu City overall. In terms of tectonic position, it is a part of the Hengduan Mountains in the eastern part of the Tibetan Plateau and belongs to the Jinsha River-Lancang River-Nujiang River tectonic belt. The outcrops in the study area primarily include Triassic and Jurassic sandstones and mudstones, as well as a small number of magmatic rocks and limestones. The hugely thick Triassic and Jurassic sandstone and mudstone strata constitute multiple NW-SE-trending folds, and the faults in the study area are also mainly in the NW-SE trending. The study area is at an elevation of 2500–4500 m and is a typical area with high mountains and canyons due to the development of medium-high mountains, widely distributed gullies, and deep valleys. These provide favorable terrain conditions for the occurrence of slope-related geo-hazards such as landslides and collapse. Owing to the high elevation, the study area has a relatively dry climate, with annual precipitation of less than 500 mm (70% occurring from July to September). Meanwhile, it has a low vegetation coverage, which is predominated by bushes.
3 Data and Method
As shown in Figure 2, the research method mainly consists of six steps: 1) preparation of data including historical landslide inventory, landslide conditioning factors, and the InSAR interpretation of current landslide deformation; 2) correlation analysis, mainly involving the correlation among landslide conditioning factors; 3) landslide susceptibility assessment using the FR, RF, and FR-RF models; 4) verification of landslide susceptibility assessment results according to ROCs; 5) correction of slope deformation information, processing of multi-source data, and conversion to the deformation rates in the slope direction; 6) optimization of landslide susceptibility assessment results, including refining the susceptibility assessment results according to slope deformation rates and the verification using typical points.
3.1 Data and Variables
3.1.1 Landslide Inventory
In this study, a total of 82 landslides were confirmed in the study area based on field survey data as well as remote sensing images and historical data and are present in the form of polygons in GIS. They are dominated by small-medium-scale shallow landslides but also include huge-scale deep rock landslides. Their minimum and maximum volume are 5 × 102 m3 and 1.8 × 108 m3, respectively. The 82 landslide polygons were divided into 46,458 grid cells (valued as 1) under the resolution of 30 m. Meanwhile, the same number of non-landslide grid cells (valued as 0) were randomly selected (i.e., a total of 92,916 grid cells were used for model training). These grid cells were randomly divided into a training and test data set at a ratio of 7:3 (Tien et al., 2016). That is, the training and the test data sets include 65,042 and 27,874 grid cells, respectively.
3.1.2 Landslide Conditioning Factors
There are no available general criteria for the selection of independent variables of landslide susceptibility, while the variables should be selected on the principle that they must be operable, inconsistent, measurable, and non-redundant (Lulseged and Yamagishi, 2005). According to the geological environment characteristics of the study area, the landslide susceptibility variables selected in this study include elevation, slope, aspect, profile curvature, terrain humidity index (TWI), normalized difference vegetation index (NDVI), lithology, and distance from a fault. The data sources of this study mainly include: 1) SRTM digital elevation model (DEM) with a resolution of 30 m, used to extract geomorphic parameters and hydrological environmental factors such as terrain humidity; 2) 1:250,000 geologic maps, used to extract the data on lithology and faults; 3) remote sensing images with a resolution of 30 m (images from paths/rows of 134/38 and 134/39 of Landsat 8 OLI_TIRS on August 13, 2013), based on which NDVI values were extracted using software ENVI. All conditioning factors were mapped as the grid cells with a resolution of 30 m.
The multicollinearity among landslide conditioning factors can be determined according to the variance inflation factor (VIF) and tolerance (TOL; Bai et al., 2010; Tien et al., 2011; Colkesen et al., 2016). VIF values of greater than 10 or TOL values of less than 0.1 indicate potential significant multicollinearity (O'brien 2007; Tien et al., 2011). The multicollinearity diagnosis was performed using the software SPSS, and the VIF and TOL values obtained are shown in Table 1. According to data analysis, the maximum VIF and the minimum TOL were 2.842 and 0.956, respectively, indicating no multicollinearity among the eight landslide conditioning factors. The eight variables were classified using the method of Jenks natural breaks. They were present in layers and assigned to the training data set to facilitate the operation of the susceptibility model (Figure 3).
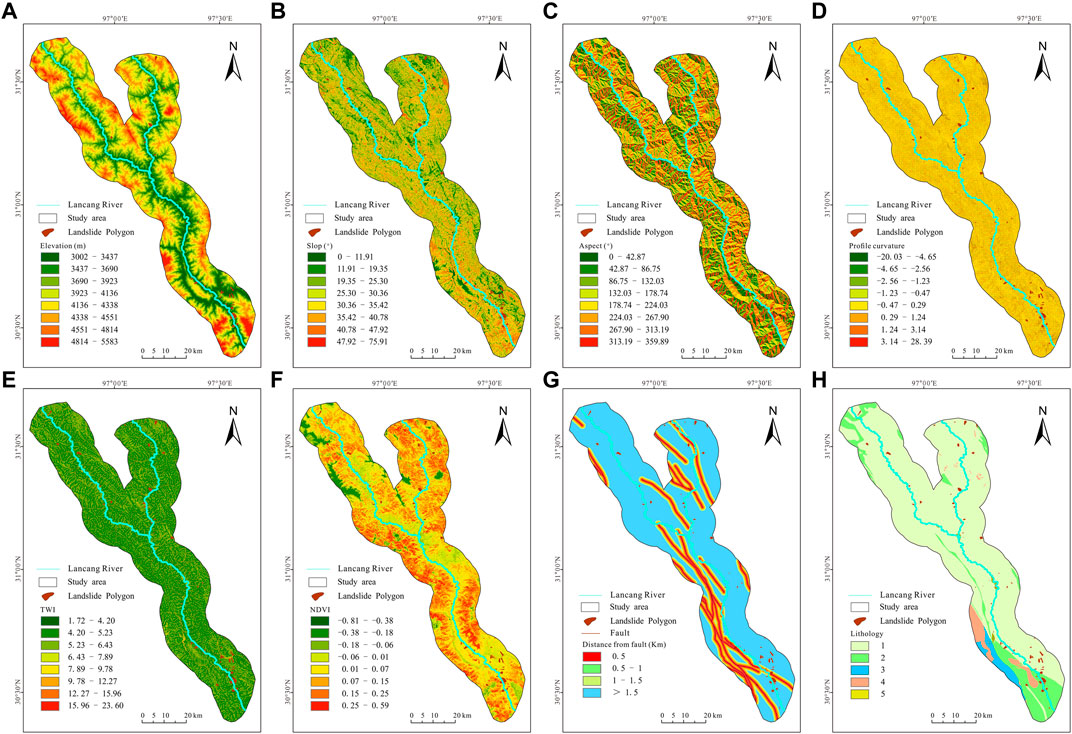
FIGURE 3. Landslide conditioning factors: (A) elevation, (B) slope, (C) aspect, (D) profile curvature, (E) TWI, (F) NDVI, (G) distance from a fault, (H) lithology. Note: for the legends in (H), “1” represents the conglomerate, sandstone, and mudstone with bedding developing; “2” represents limestone, slate, dolomite, and marble; “3” represents gneiss, granulite, and quartz schist; “4” represents blocky granite, diorite, and magmatic dike, and “5” represents loose gravel, sand, and detritus soil.
3.2 Landslide Susceptibility Assessment Models
3.2.1 Frequency Ratio Model
The FR model is used to analyze the spatial relationships between landslide distribution and various environmental factors. It can be summarized as the ratio of two percentages, namely the percentage of the landslide grid cells corresponding to a classification interval of a certain factor accounting for all landslide grid cells and the percentage of all the grid cells corresponding to a classification interval of a certain factor accounting for all grid cells in the study area (Li et al., 2017; Aditian et al., 2018; Guo et al., 2019), as shown in Formula (1):
Where, n is the number of all classification intervals of a certain factor; NLSpix is the number of the landslide grid cells corresponding to a classification interval of a certain factor; NCpix is the number of the grid cells corresponding to a classification interval of a certain factor. Ratios greater than 1 indicate that the classification interval of a certain factor is favorable for the occurrence of landslides. Otherwise, the classification interval of a certain factor is unfavorable of the occurrence of landslides.
3.2.2 Random Forest Model
RF is an integrated learning method for prediction, in which the bagging method is employed to generate multiple independent sample sets and multiple classification and regression trees (CARTs), and the results are determined by the highest or average votes (Breiman 2001). The main idea behind the RF model is that multiple weak classifiers are combined according to a certain strategy to form an integrated model that has a better prediction performance than a single classifier. This model has well been applied in landslide susceptibility assessment (Youssef et al., 2015). In this study, the RF model in the R language and environment was adopted to assess the landslide susceptibility, flowchart was shown as Figure 4. Dependent variables were present in the form of landslide probability pixels on the landslide susceptibility map and the bagging technique was employed to conduct RF feature selection. At each node of the CARTs, samples of variables and observation were randomly incorporated into the training data set for model calibration. Since the random selection of the training data set may affect the results of the model, multiple trees were adopted to balance the model. Meanwhile, unselected cases (outside the bags) were used to calculate the error of the model (OOB Error), which is equal to the standard deviation between predicted and observed values.
3.2.3 Frequency Ratio-Random Forest Model
The FR and RF models were superimposed by replacing the values of the influencing factors with the FRs calculated using Formula (1). Specifically, the FRs of each independent variable factor obtained using the FR model were input into the RF model under the R language and environment for learning. Then the FR-RF model was adoped to predict the landslide susceptibility of the whole study area to obtain the landslide susceptibility index (LSI) values.
3.2.4 Verification of Model Accuracy
ROCs were adopted to verify and compare the performance of the three models. In a ROC, the y-axis represents the true positive rate (sensitivity), which is used to characterize the percentage of correctly predicted landslide grid units, and the x-axis denotes the false positive rate (1-Specificity), which is utilized to characterize the percentage of wrongly predicted landslide grid cells (Van et al., 2006). Therefore, the closer the ROC to the upper left corner of the graph, the more accurate the test results. The area under the ROC is the AUC value, which varies in the range of 0.5–1. The higher the AUC value, the more accurate the model.
3.3 SBAS-InSAR
Given the average development degree of the vegetation in the study area, interpretation was individually conducted using 10 phases of RADARSAT-2 (descending) data and 45 phases of Sentinel-1 (ascending) data of C-band from August 2018 to February 2020. The basic parameters of the two types of data are shown in Table 2. The interpretation results of the two types of data were compared and those with higher precision were taken as the final deformation results. The data of SRTM1 DEM with a resolution of 30 m were used as external data to eliminate the terrain-contributed interference phases.
Data processing was conducted using the open-source software StamPS (Hooper et al., 2012), SBAS-InSAR processing flow was consistent with Yang et al., 2022. For RADARSAT-2 data, the spatial and temporal baselines were set at 300 m and 96 days, respectively, obtaining 14 interferometric pairs in total (Figure 5A). For Sentinel-1 data, the spatial and temporal baselines were set at 150 m and 24 days, respectively, obtaining 80 interferometric pairs in total (Figure 5B). Afterward, the interferometric pairs obtained through filtering using a Goldstein filter were filtered again to improve the signal-to-noise ratio and to further improve the precision of phase unwrapping and prediction results. Phase unwrapping was conducted using the minimum cost flow (MCF) algorithm. To avoid the effects of the unwrapping errors of low-coherence areas on the final results, the coherence value threshold was set at 0.35 (i.e., phase unwrapping was only performed for the areas with coherence values higher than 0.35). After that, ground control points (GCPs) were adopted to refine the interferometric pairs to remove orbit-induced residual errors. The GCPs were selected from the stable areas that are far away from deformation and have coherence values higher than 0.7 (Zhu et al., 2011; Gaber et al., 2017). Furthermore, a three-order inversion model was adopted to remove terrain-induced residual phases. In this way, the initial deformation information was obtained. To eliminate atmosphere-induced residual errors, the thresholds of the low- and high-pass filters of the atmosphere were set at 1000 m and 365 days, respectively. Finally, the time-series nonlinear line-of-sight (LOS) deformation was estimated using the least square solution determined by the method of singular-value decomposition (Lanari et al., 2007).
The deformation rates in the LOS direction (Vlos) were converted into those in the slope direction (Vslope) using Formula (2). To prevent extremely high absolute values from occurring during the conversion of Vlos into Vslope, Herrera et al. took the empirical value index = ± 0.3 as fixed thresholds (Zhang et al., 2018). In detail, Index was set at −0.3 in the case of −0.3 < index < 0 and at 0.3 in the case of 0 < index < 0.3. Then deformation points with non-negative deformation rates in the slope direction were removed from the results obtained. In this way, the deformation rates in the slope direction were determined.
Index = nlos·nslope
Where:
Vlos—deformation rate in LOS direction;
α—direction of slop (°);
φ—slope (°);
θ—incident angle of radar beam (°);
αS—the angle between the direction of satellite orbit and the true north.
3.4 Refining
The deformation results were integrated and refined by establishing a contingency matrix between the LSI values and the deformation rates obtained by the SBAS-InSAR method (Table 3). The computation of the contingency matrix was carried out in GIS. In detail, the deformation rates were spatially connected with the LSM obtained using the FR-RF model and then the contingency matrix was calculated using a field calculator. As a result, the refined landslide susceptibility map was exported. In the contingency matrix, the deformation rate intervals were determined according to the standard deviation of the deformation rates (δ = −13 mm/a; Zhao et al., 2019), and the susceptibility grades of LSI from 1 to 5 represent very low, low, moderate, high, and very high susceptibility, respectively. Based on this, the deformation obtained by the SBAS-InSAR method was classified into different zones according to susceptibility grades. Meanwhile, the susceptibility grades corresponding to the original LSI values were increased by 1–4 according to the deformation rates. The higher the deformation rates, the higher the landslide susceptibility and the susceptibility grades shall not be greater than 5. Slopes are highly active when their deformation rates exceed a certain value according to field survey results. In this case, the landslide susceptibility grades should be high and very high. The above integration helps to reduce misclassification probability. Meanwhile, for areas that have low susceptibility but are subject to landslides soon, their susceptibility can be corrected to a high grade after the integration (Table 2).
4 Results
4.1 Results of the Frequency Ratio Model
The FRs of all groups of the landslide conditioning factors of the study area were calculated using Formula (1), as shown in Supplementary Table S1. Based on this, the weight of each landslide conditioning factor was determined. Then LSI of the study area was calculated using the Raster Calculator tool in the ArcGIS, which fell within the range of 1.43–17.02. The higher the LSI, the higher the occurrence probability of landslides, and vice versa. The LSI range was divided into five intervals by the method of Jenks natural breaks, namely [1.43, 3.82), [3.82, 4.98), [4.98, 6.10), [6.10, 7.68), and [7.68, 17.02], corresponding to the very low, low, moderate, high, and very high susceptibility grades, respectively. Based on this, the landslide susceptibility map was formed, as shown in Figure 6A. According to this figure, the areas with very low and low susceptibility are the largest, with area percentages of 30.46 and 26.21%, respectively. In contrast, the areas with moderate, high, and very high susceptibility are smaller, with area percentages of 18.15, 17.23, and 7.95%, respectively. The accuracy of the model was verified using 14,416 landslide grid cells in the test data set. Among them, 11,465 grid cells (79.53%) fell in the scope of high and very high susceptibility.
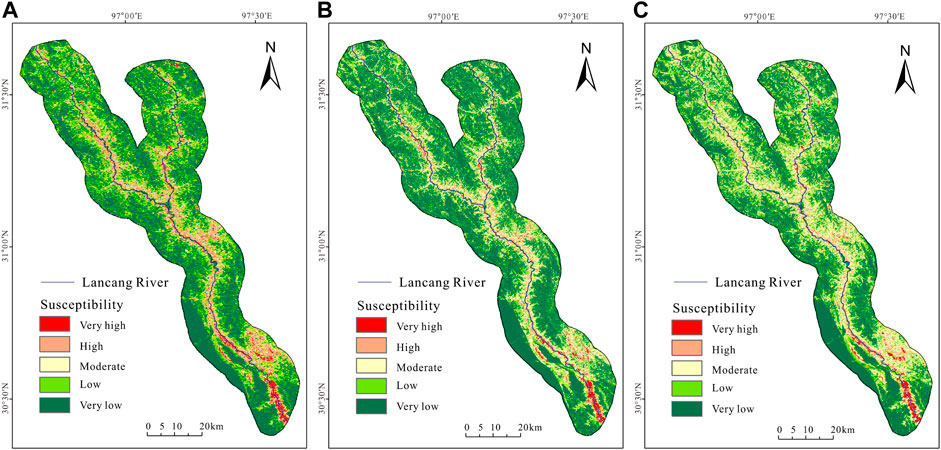
FIGURE 6. Landslide susceptibility maps obtained using the three models. (A) FR model; (B) RF model; (C) FR-RF model.
4.2 Results of the Random Forest Model
The LSI of the study area obtained using the RF model varied in the range of 0–0.98. Similarly, the LSI range was divided into five intervals by the method of Jenks natural breaks, namely [0, 0.14), [0.14, 0.28), [0.28, 0.45), [0.45, 0.63), and [0.63, 0.98], which correspond to the very low, low, moderate, high, and very high susceptibility, respectively. The landslide susceptibility map formed is shown in Figure 6B. According to this figure, the areas with very low, low, moderate, high, and very high susceptibility have area percentages of 40.25, 21.76, 18.82, 12.13, and 7.04%, respectively. The accuracy of the model was verified using the remaining 14,416 landslide grid cells in the test data set. Among them, 12,484 grid cells (86.60%) fell in the scope of high and very high susceptibility.
4.3 Results of the Frequency Ratio-Random Forest Model
The FRs obtained using the FR model were taken as input data of the RF model. Then the landslide susceptibility assessment of the whole study area was also conducted using the R language, obtaining the LSI of the study area. The results show that the LSI fell within the range of 0–1. Similarly, the LSI range was divided into five intervals by the method of Jenks natural breaks, namely [0, 0.15), [0.15, 0.31), [0.31, 0.53), [0.53, 0.77), and [0.77, 1], corresponding to the very low, low, moderate, high, and very high susceptibility grades, respectively. The landslide susceptibility map formed is shown in Figure 6C. According to this figure, the areas with very low, low, moderate, high, and very high susceptibility have area percentages of 35.90, 25.06, 18.74, 13.73, and 6.57%, respectively. Similarly, the accuracy of the model was verified using the remaining 14,416 landslide grid cells in the test data. Among them, 13,875 grid cells (96.25%) fell in the scope of high and very high susceptibility.
With the landslide probability predicted using the FR, RF, and FR-RF models as the LSI and based on the verification of these models using the remaining 30% landslide test data, the ROCs of the prediction results of all the models were plotted (Figure 7) and their AUC values were calculated. Based on this, the prediction precision of all the models was compared. According to Figure 7, the final AUC values of the prediction results of the FR, RF, and FR-RF models were 0.866, 0.897, and 0.916, respectively. Therefore, the FR-RF model possesses the highest prediction precision. Meanwhile, it was verified by existing landslides that the FR-RF model had the highest accuracy. Therefore, the assessment results of the FR-RF model were taken as the final susceptibility results and were further optimized.
4.4 SBAS-InSAR Results
For the slopes in the study area, the deformation rates in the LOS direction obtained using the Sentinel-1 ascending data varied in the range of −58–21 mm/a (Figure 8A). Then they were converted into the deformation rates in the slope direction, obtaining 2,392,676 coherent points in total. In contrast, the deformation rates in the LOS direction obtained using the RADARSAT-2 descending data varied in the range of −42–16 mm/a and a total of 5,787,774 coherent points were obtained after conversion (Figure 8B). The descending data obviously yielded a higher density of interference points and can present more details of hidden hazards. Therefore, the descending data were utilized to identify the slope deformation and identification results as follows. The average density of deformed points was 3687.19/km2, which was higher than other studies previously considered high density (Meisina et al., 2008; Zhao et al., 2019), shows the density is acceptable. The maximum deformation rate in the slope direction was up to −128 mm/a (the critical stable interval: −13–0 mm/a; Figure 8C). The deformation results obtained by the SBAS method failed to cover the whole study area since ice, snow, and water are locally distributed in the study area.
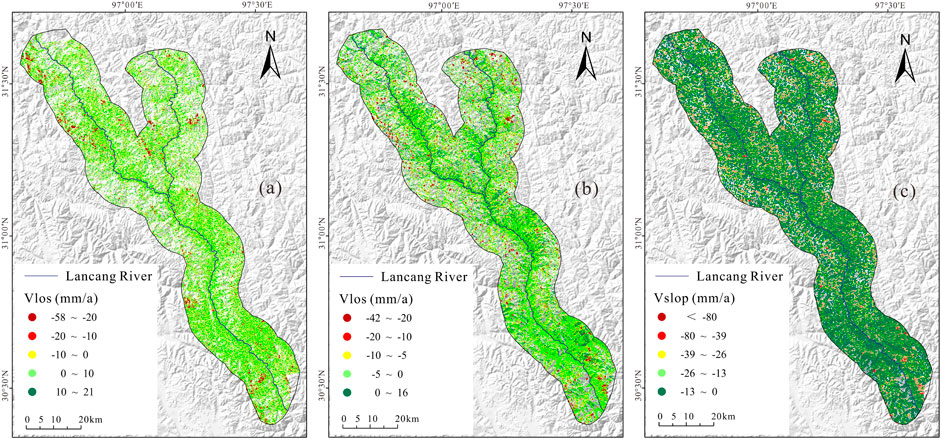
FIGURE 8. (A) Deformation results in LOS direction obtained using Sentinel-1 data; (B) Deformation results in LOS direction obtained using RADARSAT-2 data; (C) Deformation results in slope direction after conversion.
With the standard deviation (−13 mm/a) of RADARSAT-2 data as the threshold of stable points, the areas with statistically reliable deformation obtained by the SBAS method accounted for 54.22% of the entire study area. Through remote sensing interpretation and field verification of the deformation results, a total of 113 points with hidden landslide hazards were delineated and after excluding land subsidence points in flat areas and a few points denoting snow and ice movements, field verification confirms the reliability of InSAR interpretation results (Zhang et al., 2021). According to the map of slope deformation rates, there exist multiple deformation centers in the study area. In detail, continuous high deformation values are visible on the left bank of the Lancang River in Kagong area in the southern section of the study area, multiple abnormal deformation areas exist on the left bank of the Lancang River in the Chaiwei-Ritong areas in the northern section of the study area, and there are abnormal deformation areas on the right bank of the Lancang River near the Shagong area. As shown by the statistical results, only 39.24% of landslides newly identified by the SBAS method and the FR-RF model feature high or very high landslide susceptibility. Therefore, the results are reliable and can be used to optimize the landslide susceptibility assessment results.
4.5 Refining of Assessment Results
The refined landslide susceptibility map is shown in Figure 9A. Compared with the susceptibility results before refining, the landslide susceptibility of multiple areas was notably increased, especially in the Ritong and Xueru areas (Figure 9B). Table 4 shows the changes in the number percentages of the grid cells of all grades before and after the optimization. According to this table, the grid cells with susceptibility grades of 1, 2, 3, 4, and 5 after refining accounted for 31.01, 26.55, 19.17, 15.64, and 7.63%, respectively. By comparison with unrefined assessment results, the areas with high and very high susceptibility increased by 2.97% in the refined assessment results. Meanwhile, 563,430 grid cells showed changes in landslide susceptibility, accounting for 11.15% of the total grid cells. Among them, the landslide susceptibility of 412,563, 113,775, 36,985, and 106 grid cells increased by one, two, three, and four grades, respectively.
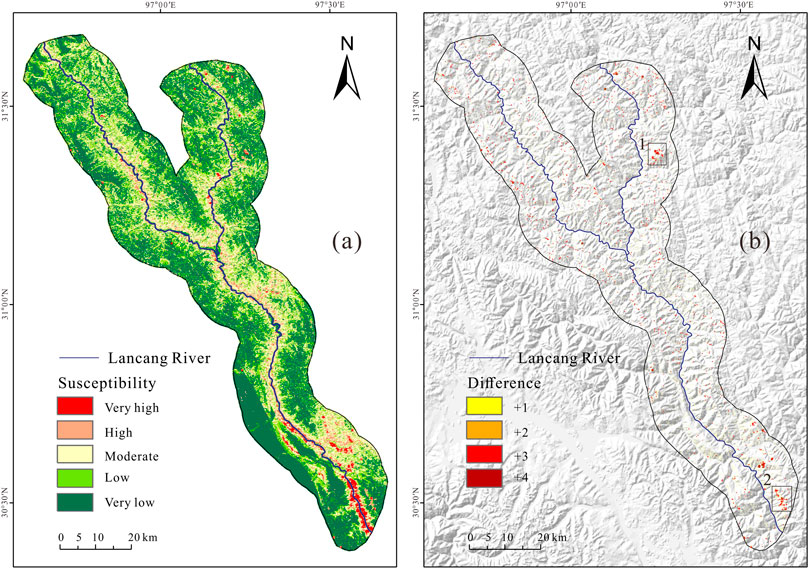
FIGURE 9. (A) landslide susceptibility assessment results after processing using a correction matrix; (B) differences between the unrefined and refined landslide susceptibility grades of each grid cell. Notes: the rectangular areas marked with 1 and 2 denote the Ritong and Xueru areas, respectively.
4.6 Research Results of Typical Areas
A detailed analysis was carried out on two areas with the greatest changes in landslide susceptibility (marked with 1 and 2 in Figure 9B). Area No. 1 lies in the Ritong area in the northern part of the study area. In addition to Jurassic sandstones and mudstones, magmatic rocks such as island-shaped Paleogene granite porphyries and ordinary porphyries have developed in the area. Owing to differential weathering, the magmatic rock masses formed convex and isolated peaks with a slope greater than 50°. Furthermore, their strength decreased as a result of long-term weathering and denudation, posing the risks of instability and collapse. Field surveys show that clastic materials accumulated due to the weathering of high-elevation rock masses may cause landslides or other instability events, thus endangering the roads and villages at the slope foot. This possibility was revealed by the deformation information obtained by the SBAS-InSAR method. Meanwhile, the RLSM also shows a significant increase in the landslide susceptibility of this area compared to the LSM (Figure 10).
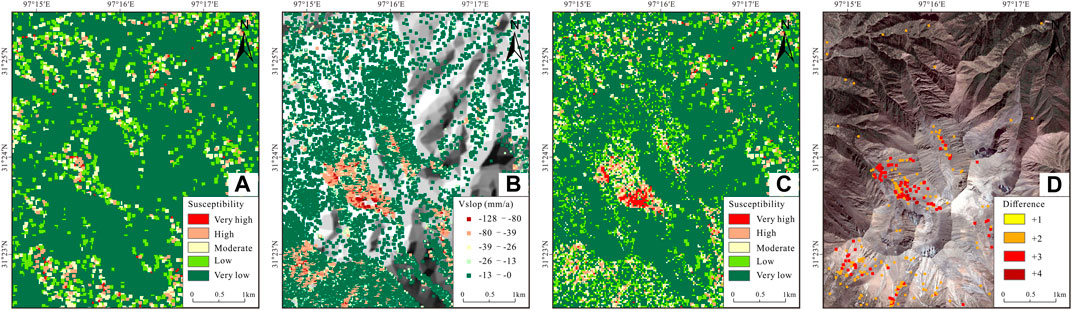
FIGURE 10. Landslide assessment results of area No. 1 (Ritong area). (A) landslide susceptibility assessment results obtained using the FR-RF model. (B) deformation rates in the slope direction obtained by the SBAS-InSAR method. (C) optimized landslide susceptibility assessment results. (D) changes in landslide susceptibility grades before and after refining.
Area No. 2 is located in the Xueru area on the eastern side of the Lancang River in the southern part of the study area. In terms of lithology, the strata in this area consist of schists and slates of the Carboniferous Kagong Formation in the tectonic melanges of the Lancang River. Meanwhile, the Lancang River fault zone runs through this area. Therefore, the rock masses in area No. 2 are relatively broken and show high susceptibility to high-elevation collapse and landslides. However, since this area lies in a high and steep slope on the eastern side of the Lancang River, its adverse high-elevation geological phenomena tend to be ignored. According to the latest SBAS-InSAR interpretation results, multiple areas with high deformation rates exist in the middle-upper part of the slope and they are prone to landslides. The RLSM shows the differences of area No. 2 before and after optimization and meanwhile, some areas with low susceptibility were corrected into areas with high susceptibility (Figure 11).
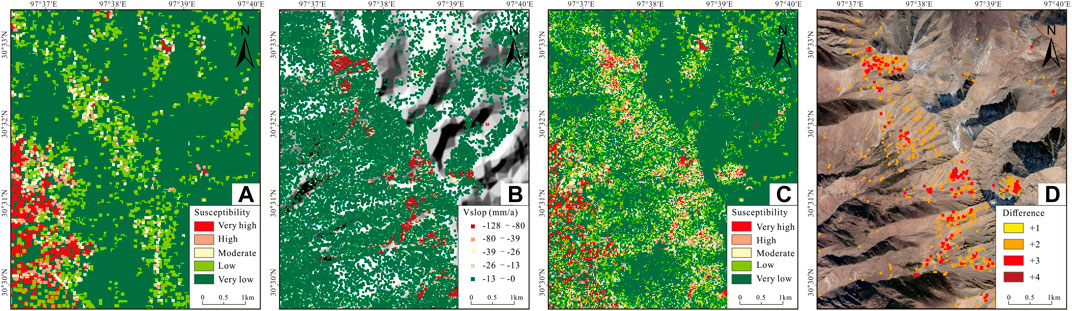
FIGURE 11. Landslide assessment results for area No. 2 (Xueru area). (A) landslide susceptibility assessment results obtained using the FR-RF model. (B) Deformation rates in the slope direction obtained by the SBAS-InSAR method. (C) optimized landslide susceptibility assessment results. (D) changes in landslide susceptibility grades before and after refining.
5 Discussion
In this study, the FR, RF, and FR-RF models were adopted to evaluate the application of LSM along the north Lancang River. The purpose is to compare the accuracy of mathematical models and machine learning models and to further obtain more accurate landslide susceptibility assessment results. Therefore, it is highly necessary to verify the accuracy of the results. To this end, a test data set is required to verify the ability of the models to identify new samples, and then the test errors are taken as the approximation of generalization error. It is generally assumed that the collection of samples for verification is independent of the true distribution of samples. In addition, the test and training data sets should be mutually exclusive as much as possible. However, they should be determined in such a way that their data distribution should be kept consistent as far as possible to avoid the effects of data segmentation on the final results. In this study, 70% of the samples were used for training, while the rest were used for testing.
In this study, both the Sentinel-1 data and the RADARSAT-2 data were utilized for deformation monitoring by the InSAR method. They are ascending and descending data, respectively and have the same monitoring duration. Their advantages and disadvantages were assessed according to the deformation point density that was finally calculated using analytical data. The purpose was to avoid errors that may be caused by a single data source. Finally, the RADARSAT-2 data were selected. Since RADARSAT-2 has a revisit cycle of 14 days, the InSAR method is only applicable to slow and very slow ground surface movements but can only yield average effects in monitoring quick ground surface movements such as collapse and rockfalls. There is no perennial cover of snow and ice and the vegetation coverage is low in the study area. Therefore, the C-band data of RADARSAT-2 are fit for the InSAR interpretation of the study area.
In this study, the LSM was performed using the FR, RF, and FR-RF models. However, the results obtained were limited by the data quality related to each landslide conditioning factor and the quality of landslide samples, which may lead to classification errors. For the data quality related to each landslide conditioning factor, regional susceptibility assessment tends to be carried out using the terrain and landform data with a resolution of 90 m or even higher and the lithologic fault data mostly sourced from 1:500,000 regional geologic maps (Li et al., 2017; Zhao et al., 2019). Owing to the limited area of the study area, the terrain and landform data used in this study have a resolution of 30 m and the lithologic fault data are on a scale of 1:250,000, thus ensuring the data quality of landslide conditioning factors to the highest extent. For the quality of landslide samples, it is a common practice to use historical landslide data in landslide susceptibility assessment (Alkhasawneh et al., 2014; Wang et al., 2015; Tsangaratos and Ilia 2016; Chen et al., 2017). However, the evidence of landslide activities may be lost due to landslide evolution, erosion process, vegetation growth, and the effects of human activities. Historical landslides can be effectively supplemented through the timely identification of active landslides. In this way, the accuracy of LSM can be improved. Furthermore, the landslides in this study area mostly occur in low-elevation areas due to the landform consisting of high mountains and canyons of the Lancang River. This causes the lack of samples of high-elevation landslides, which tend to be inaccessible in traditional surveys, thus leading to serious classification errors in the LSM. The comparison of results before and after InSAR refining also reveals that the slopes with great changes in landslide susceptibility grades are mostly located in high-altitude areas. This indicates the advantages of InSAR refining in this study and the necessity of optimizing the LSM by the InSAR method.
It should be noted that, for the deformation results determined by LSM along with InSAR, there are fewer errors that the areas subject to slope deformation are wrongly classified as the areas with very low and low sensitivity. In contrast, the LSM based on traditional models only presents the regional distribution of the landslides predicted and cannot provide the dynamic deformation process of slopes. However, the evolution of landslides with time is the greatest concern for decision makers (Xie et al., 2017). RLSM allows the current state of landslides to be revealed and can be applied to the preliminary landslide surveying and mapping and quantitative risk management on a regional scale (Chalkias et al., 2014).
To sum up, landslide susceptibility assessment and its mapping serve as a major step in the research of landslide risks and can be used to assess the risks in areas prone to landslides and areas with infrastructures. It has been proposed that the Sichuan-Tibet Railway under planning run through the study area in the form of a bridge. Therefore, it is obvious that more accurate LSM can better serve the planning and risk control of the Sichuan-Tibet Railway. The deformation results obtained using LSM and InSAR individually can both achieve required goals, while the combination of them can further optimize and refine LSM. Compared with the landslide susceptibility maps obtained using traditional models, the landslide susceptibility map refined using the InSAR results yielded more accurate sorting of landslide susceptibility along Lancang River. Therefore, it is believed that the refined landslide susceptibility map will be valuable for effective land use management and project planning in the Lancang River region.
6 Conclusion
In this study, the FR, RF, and FR-RF models were adopted to evaluate the application of the LSM along the north Lancang River. The ascending and descending data of satellites were individually adopted to calculate the deformation rates of slopes using the SBAS-InSAR method. As a result, more accurate deformation results obtained with RADARSAT-2 data were selected to optimize the LSM through a contingency matrix, thus improving the precision of the LSM. The above technologies were combined to further improve the accuracy of the susceptibility assessment results by considering the current deformation state of slopes and accordingly to reduce potential landslide risks.
The LSI values were obtained using the FR, RF, and FR-RF models. They were divided into five grades, namely very low, low, moderate, high, and very high. The AUC values of the assessment results obtained with FR, RF, and FR-RF models were 0.866, 0.897, and 0.916, respectively. Meanwhile, it was verified by existing landslides that the FR-RF model had the highest accuracy. Therefore, the FR-RF model was selected for LSM optimization. Afterward, a contingency matrix was established according to LSI values and the deformation rates in the slope direction were converted. Based on this, the susceptibility results obtained using the traditional model were updated. As a result, the areas with high and very high susceptibility increased by 2.97% and 563,430 grid cells showed changes in landslide susceptibility, accounting for 11.15% of all grid cells. Most especially, the Xueru and Ritong areas showed more notable increases in landslide susceptibility than other areas, and it has been verified by remote sensing images and field surveys that landslide risks exist in both areas.
This study is greatly significant for the refining of LSM, especially for areas where the SBAS-InSAR method is applicable. The refined landslide susceptibility map can provide valuable support for the decision making in the disaster prevention and mitigation and the management of major engineering facilities in the upper reaches of the Lancang River.
Data Availability Statement
The original contributions presented in the study are included in the article/Supplementary Material, further inquiries can be directed to the corresponding author.
Author Contributions
JZ designed the model and the computational framework and analysed the data. LC and YL carried out the historical landslide data collecting and field suverys. BG and JZ carried out the InSAR interpretation. JZ and HH wrote the manuscript with input from all authors. HH and DY helped supervise the project.
Funding
This study was funded by China Geological Survey Project (No. DD20221741), the Second Tibetan Plateau Scientific Expedition and Research (STEP) Program (Grant No. 2019QZKK0902) and Key laboratory of Deep-Earth Dynamics of Ministry of Natural Resources Open Project (No. J1901).
Conflict of Interest
The authors declare that the research was conducted in the absence of any commercial or financial relationships that could be construed as a potential conflict of interest.
Publisher’s Note
All claims expressed in this article are solely those of the authors and do not necessarily represent those of their affiliated organizations, or those of the publisher, the editors and the reviewers. Any product that may be evaluated in this article, or claim that may be made by its manufacturer, is not guaranteed or endorsed by the publisher.
Acknowledgments
The Sentinel-1 images, geologic maps, and landsat8 images used in this study were provided by the European Space Agency (ESA), the China Geological Survey, and the National Aeronautics and Space Administration of the United States of America (NASA), respectively.
Supplementary Material
The Supplementary Material for this article can be found online at: https://www.frontiersin.org/articles/10.3389/feart.2022.901889/full#supplementary-material
References
Aditian, A., Kubota, T., and Shinohara, Y. (2018). Comparison of GIS-Based Landslide Susceptibility Models Using Frequency Ratio, Logistic Regression, and Artificial Neural Network in a Tertiary Region of Ambon, Indonesia. Geomorphology 318 (1), 101–111. doi:10.1016/j.geomorph.2018.06.006
Alkhasawneh, M. S., Tay, L. T., Ngah, U. K., Al-Batah, M. S., and Mat Isa, N. A. (2014). Intelligent Landslide System Based on Discriminant Analysis and Cascade-Forward Back-Propagation Network. Arab. J. Sci. Eng. 39 (7), 5575–5584. doi:10.1007/s13369-014-1105-8
An, H., Ouyang, C., and Zhou, S. (2021). Dynamic Process Analysis of the Baige Landslide by the Combination of DEM and Long-Period Seismic Waves. Landslides 18, 1625–1639. doi:10.1007/s10346-020-01595-0
Bai, S., Wang, J., Lü, G., Zhou, P., Hou, S., and Xu, S. (2010). GIS-based Logistic Regression for Landslide Susceptibility Mapping of the Zhongxian Segment in the Three Gorges Area, China. Geomorphology 115 (1), 23–31. doi:10.1016/j.geomorph.2009.09.025
Berardino, P., Fornaro, G., Lanari, R., and Sansosti, E. (2002). A New Algorithm for Surface Deformation Monitoring Based on Small Baseline Differential SAR Interferograms. IEEE Trans. Geosci. Remote Sens. 40, 2375–2383. doi:10.1109/tgrs.2002.803792
Bui, D. T., Lofman, O., Revhaug, I., and Dick, O. (2011). Landslide Susceptibility Analysis in the Hoa Binh Province of Vietnam Using Statistical Index and Logistic Regression. Nat. Hazards 59 (3), 1413–1444. doi:10.1007/s11069-011-9844-2
Bürgmann, R., Rosen, P. A., and Fielding, E. J. (2000). Synthetic Aperture Radar Interferometry to Measure Earth’s Surface Topography and its Deformation. Ann. Rev. Earth Planet 28, 169–209.
Çelik, S., Özyazıcıoğlu, M., Şahin, R., Uysal, R., Çakıcı, F. Z., Kalkan, E., et al. (2021). The Destruction of Erzurum Ski-Jumping Complex by a Landslide: Evaluation of an Engineering Design Failure. Nat. Hazards 107, 475–496. Available at: http://377.rm.cglhub.com/10.1007/s11069-021-04591-2.
Chalkias, C., Ferentinou, M., and Polykretis, C. (2014). Gis-based Landslide Susceptibility Mapping on the Peloponnese Peninsula, Greece. Geosciences 4, 176–190. doi:10.3390/geosciences4030176
Chen, G., Zhang, Y., Zeng, R., Yang, Z., Chen, X., Zhao, F., et al. (2018). Detection of Land Subsidence Associated with Land Creation and Rapid Urbanization in the Chinese Loess Plateau Using Time Series Insar: A Case Study of Lanzhou New District. Remote Sens. 10, 270. doi:10.3390/rs10020270
Chen, J., Dai, F., Lv, T., and Cui, Z. (2013). Holocene Landslide-Dammed Lake Deposits in the Upper Jinsha River, SE Tibetan Plateau and Their Ages. Quat. Int. 298, 107–113. doi:10.1016/j.quaint.2012.09.018
Chen, W., Pourghasemi, H. R., and Naghibi, S. A. (2017). A Comparative Study of Landslide Susceptibility Maps Produced Using Support Vector Machine with Different Kernel Functions and Entropy Data Mining Models in China. Bull. Eng. Geol. Environ., 1–18. doi:10.1007/s10064-017-1010-y
Ciampalini, A., Raspini, F., Lagomarsino, D., Catani, F., and Casagli, N. (2016). Landslide Susceptibility Map Refinement Using PSInSAR Data. Remote Sens. Environ. 184, 302–315. doi:10.1016/j.rse.2016.07.018
Colkesen, I., Sahin, E. K., and Kavzoglu, T. (2016). Susceptibility Mapping of Shallow Landslides Using Kernel-Based Gaussian Process, Support Vector Machines and Logistic Regression. J. Afr. Earth Sci. 118, 53–64. doi:10.1016/j.jafrearsci.2016.02.019
Dai, F. C., and Deng, J. H. (2020). Development Characteristics of Landslide Hazards in Three-Rivers Basin of Southeast Tibetan Plateau. Adv. Eng. Sci. 52 (5), 3–15.
Dimitris, K., and Skevi, P. (2021). The Application of DInSAR and Bayesian Statistics for the Assessment of Landslide Susceptibility. Nat. hazards 105, 2957–2985.
Dong, J., Zhang, L., Li, M., Yu, Y., Liao, M., Gong, J., et al. (2017). Measuring Precursory Movements of the Recent Xinmo Landslide in Mao County, China with Sentinel-1 and ALOS-2 PALSAR-2 Datasets. Landslides 15 (1), 135–144. doi:10.1007/s10346-017-0914-8
Gaber, A., Darwish, N., and Koch, M. (2017). Minimizing the Residual Topography Effect on Interferograms to Improve Dinsar Results: Estimating Land Subsidence in Port-Said City, Egypt. Remote Sens. 9, 752. doi:10.3390/rs9070752
Ge, D. Q., Dai, K. R., Guo, Z. C., and Li, Z. H. (2019). Early Identification of Serious Geological Hazards with Integrated Remote Sensing technologies:Thought and and Recommendations. Geomatics Inf. Sci. Wuhan Univ. 44 (7), 949–956.
Glade, T. (1998). Establishing the Frequency and Magnitude of Landslide-Triggering Rainstorm Events in New Zealand. Environ. Geol. 35 (2–3), 160–174. doi:10.1007/s002540050302
Guo, C. B., Wu, R. A., Jiang, L. W., Zhong, N., Wang, Y., and Wang, D. (2021). Typical Geohazards and Engineering Geological Problems along the Ya’an-Linzhi Section of the Sichuan-Tibet Railway, China. Geoscience 35 (1), 1–17.
Guo, Z. Z., Yin, K. L., and Huang, F. M. (2019). Evaluation of Landslide Susceptibility Based on Landslide Classification and Weighted Frequency Ratio Model. Chin. J. Rock Mech. Eng. 38 (2), 287–300.
Hooper, A., Bekaert, D., Spaans, K., and Arıkan, M. (2012). Recent Advances in SAR Interferometry Time Series Analysis for Measuring Crustal Deformation. Tectonophysics 514-517, 1–13. doi:10.1016/j.tecto.2011.10.013
Hu, M. M., Wu, Z. H., Reicherter, K., Ali, S., Huang, X. L., and Zuo, J. M. (2021). A Historical Earthquake-Induced Landslide Damming Event at the Qiaojia Reach of the Jinsha River, SE Tibetan Plateau: Implication for the Seismic Hazard of the Xiaojiang Fault. Front. Earth Sci. 9. doi:10.3389/feart.2021.649543
Huang, Y., and Zhao, L. (2018). Review on Landslide Susceptibility Mapping Using Support Vector Machines. Catena 165, 520–529. doi:10.1016/j.catena.2018.03.003
Intrieri, E., Raspini, F., Fumagalli, A., Lu, P., Del Conte, S., Farina, P., et al. (2017). The Maoxian Landslide as Seen from Space: Detecting Precursors of Failure with Sentinel-1 Data. Landslides 15 (1), 123–133. doi:10.1007/s10346-017-0915-7
Lanari, R., Casu, F., Manzo, M., and Lundgren, P. (2007). Application of the Sbas-Dinsar Technique to Fault Creep: A Case Study of the Hayward Fault, California. Remote Sens. Environ. 109, 20–28. doi:10.1016/j.rse.2006.12.003
Lanari, R., Mora, O., Manunta, M., Mallorqui, J. J., Berardino, P., and Sansosti, E. (2004). A Small-Baseline Approach for Investigating Deformations on Full-Resolution Differential SAR Interferograms. IEEE Trans. Geosci. Remote Sens. 42, 1377–1386. doi:10.1109/tgrs.2004.828196
Li, J., Li, Z.-w., Wu, L.-x., Xu, B., Hu, J., Zhou, Y.-s., et al. (2018). Deriving a Time Series of 3d Glacier Motion to Investigate Interactions of a Large Mountain Glacial System with its Glacial Lake: Use of Synthetic Aperture Radar Pixel Offset-Small Baseline Subset Technique. J. Hydrology 559, 596–608. doi:10.1016/j.jhydrol.2018.02.067
Li, L. P., Lan, H. X., and Guo, C. B. (2017). Geohazard Susceptibility Assessment along the Sichuan-Tibet Railway and its Adjacent Area Using an Improved Frequency Ratio Method. Geosciences 31 (5), 911–929.
Liu, W., Yan, S., and He, S. (2018). Landslide Damage Incurred to Buildings: A Case Study of Shenzhen Landslide. Eng. Geol. 247, 69–83. doi:10.1016/j.enggeo.2018.10.025
Lu, C., and Cai, C. (2019). Challenges and Countermeasures for Construction Safety during the Sichuan-Tibet Railway Project. Engineering 5 (5), 833–838. doi:10.1016/j.eng.2019.06.007
Lulseged, A., and Yamagishi, H. (2005). The Application of Gis-Based Logistic Regression for Landslide Susceptibility Mapping in the Kakuda-Yahiko Mountains, Central Japan. Geomorphology 65, 15–31.
Lyu, L. Q., Xu, M. Z., Wang, Z. Y., Qi, L. J., and Li, X. (2021). Impact of Densely Distributed Debris Flow Dams on River Morphology of the Grand Canyon of the Nu River (Upper Salween River) at the East Margin of the Tibetan Plateau. Landslides 18 (5), 979–991. doi:10.1007/s10346-020-01536-x
Meisina, C., Zucca, F., Notti, D., Colombo, A., Cucchi, A., Savio, G., et al. (2008). Geological Interpretation of PSInSAR Data at Regional Scale. Sensors 8, 7469–7492. doi:10.3390/s8117469
Merghadi, A., Yunus, A. P., Dou, J., Whiteley, J., ThaiPham, B., Bui, D. T., et al. (2020). Machine Learning Methods for Landslide Susceptibility Studies: a Comparative Overview of Algorithm Performance. Earth-Science Rev. 207, 103225. doi:10.1016/j.earscirev.2020.103225
O’brien, R. M. (2007). A Caution Regarding Rules of Thumb for Variance Inflation Factors. Qual. Quantity 41 (5), 673–690.
Pánek, T. (2019). Landslides and Quaternary Climate Changes-The State of the Art. Earth-Science Rev. 196, 102871. doi:10.1016/j.earscirev.2019.05.015
Qu, C., Zhang, G., Shan, X., Zhang, G., Song, X., and Liu, Y. (2013). Coseismic Deformation Derived from Analyses of C and L Band SAR Data and Fault Slip Inversion of the Yushu Ms7.1 Earthquake, China in 2010. Tectonophysics 584, 119–128. doi:10.1016/j.tecto.2012.05.011
Rott, H., and Nagler, T. (2006). The Contribution of Radar Interferometry to the Assessment of Landslide Hazards. Adv. Space Res. 37, 710–719. doi:10.1016/j.asr.2005.06.059
Shi, P. J. (2016). Natural Disasters in China. doi:10.1007/978-3-662-50270-9Natural Disasters in China
Tien Bui, D., Tuan, T. A., Klempe, H., Pradhan, B., and Revhaug, I. (2016). Spatial Prediction Models for Shallow Landslide Hazards: a Comparative Assessment of the Efficacy of Support Vector Machines, Artificial Neural Networks, Kernel Logistic Regression, and Logistic Model Tree. Landslides 13 (2), 361–378. doi:10.1007/s10346-015-0557-6
Tre, A. (2017). Data in Focus: Precursor of Maoxian Landslide Measured from Space. Available at: http://tre-altamira.com/news/datafocus-precursor-maoxian-landslide-measured-space.
Tsangaratos, P., and Ilia, I. (2016). Comparison of a Logistic Regression and Naïve Bayes Classifier in Landslide Susceptibility Assessments: The Influence of Models Complexity and Training Dataset Size. CATENA 145, 164–179. doi:10.1016/j.catena.2016.06.004
Turner, A. K. (2018). Social and Environmental Impacts of Landslides. Innov. Infrastruct. Solut. 3, 70. doi:10.1007/s41062-018-0175-y
Van Den Eeckhaut, M., Vanwalleghem, T., Poesen, J., Govers, G., Verstraeten, G., and Vandekerckhove, L. (2006). Prediction of Landslide Susceptibility Using Rare Events Logistic Regression: a Case-Study in the Flemish Ardennes (Belgium). Geomorphology 76, 392–410. doi:10.1016/j.geomorph.2005.12.003
Wallemacq, P., Below, R., and McLean, D. (2018). Economic Losses, Poverty and Disasters 1998–2017, United Nations Office for Disaster Risk Reduction (UNDRR) and Centre for Research on the Epidemiology of Disasters. (CRED) publications.
Wang, L.-J., Guo, M., Sawada, K., Lin, J., and Zhang, J. (2015). Landslide Susceptibility Mapping in Mizunami City, Japan: A Comparison between Logistic Regression, Bivariate Statistical Analysis and Multivariate Adaptive Regression Spline Models. Catena 135, 271–282. doi:10.1016/j.catena.2015.08.007
Wang, P., Chen, J., Dai, F., et al. (2017). Chronology of Relict Lake Deposits Around the Suwalong Paleo Landslide in the Upper Jinsha River, SE Tibetan Plateau: Implications to Holocene Tectonic Perturbations. Geomorphology 217, 193–203.
Xie, Z., Chen, G., Meng, X., Zhang, Y., Qiao, L., and Tan, L. (2017). A Comparative Study of Landslide Susceptibility Mapping Using Weight of Evidence, Logistic Regression and Support Vector Machine and Evaluated by SBAS-InSAR Monitoring: Zhouqu to Wudu Segment in Bailong River Basin, China. Environ. Earth Sci. 76, 313. doi:10.1007/s12665-017-6640-7
Xiong, Z., Feng, G., Feng, Z., Miao, L., Wang, Y., Yang, D., et al. (2020). Pre- and Post-failure Spatial-Temporal Deformation Pattern of the Baige Landslide Retrieved from Multiple Radar and Optical Satellite Images. Eng. Geol. 279, 105880. doi:10.1016/j.enggeo.2020.105880
Xu, Q., Dong, X. J., and Li, W. L. (2019). Integrated Space-Airground Early Detection, Monitoring and Warning System for Potential Catastrophic Geohazards. Geomatics Inf. Sci. Wuhan Univ. 44 (7), 957–966.
Yang, F., Jiang, Z. R., and Ren, J. Q. (2022). Monitoring, Prediction, and Evaluation of Mountain Geological Hazards Based on InSAR Technology, 2022. Scientific Programming, 2022. doi:10.1155/2022/2227049
Yao, X., Deng, J. H., Liu, X. H., Zhou, Z. K., Yao, J. M., and Dai, F. C. (2020). Primary Recognition of Active Landslides and Development Rule Analysis for Pan Three-River-Parallel Territory of Tibet Plateau. Adv. Eng. Sci. 52 (5), 16–37. doi:10.15961/j.jsuese.202000529
Yao, X., Li, L., Zhang, Y., Zhou, Z., and Liu, X. (2017). Types and Characteristics of Slow-Moving Slope Geo-Hazards Recognized by TS-InSAR along Xianshuihe Active Fault in the Eastern Tibet Plateau. Nat. Hazards 88 (3), 1727–1740. doi:10.1007/s11069-017-2943-y
Youssef, A. M., Pourghasemi, H. R., Pourtaghi, Z. S., and Al-Katheeri, M. M. (2015). Landslide Susceptibility Mapping Using Random Forest, Boosted Regression Tree, Classification and Regression Tree, and General Linear Models and Comparison of Their Performance at Wadi Tayyah Basin, Asir Region, Saudi Arabia. Landslides 13, 839–856. doi:10.1007/s10346-015-0614-1
Zhang, J. J., Gao, B., liu, J. K., Chen, L., Huang, H., and Li, J. (2021). Early Landslide Detection in the Lancangjiang Region along the Sichuan-Tibet Railway Based on SBAS-InSAR Technology. Geosciences 35 (1), 64–73.
Zhang, Y., Meng, X., Jordan, C., Novellino, A., Dijkstra, T., and Chen, G. (2018). Investigating Slow-Moving Landslides in the Zhouqu Region of China Using Insar Time Series. Landslides 15, 1299–1315. doi:10.1007/s10346-018-0954-8
Zhang, Y., Meng, X., Jordan, C., Novellino, A., Dijkstra, T., and Chen, G. (2018). Investigating Slow-Moving Landslides in the Zhouqu Region of China Using InSAR Time Series. Landslides 15 (7), 1299–1315. doi:10.1007/s10346-018-0954-8
Zhang, Y., Zhao, X., Lan, H., and Xiong, T. (2011). A Pleistocene Landslide-Dammed Lake, Jinsha River, Yunnan, China. Quat. Int. 233 (1), 72–80. doi:10.1016/j.quaint.2010.10.020
Zhao, F., Meng, X., Zhang, Y., Chen, G., Su, X., and Yue, D. (2019). Landslide Susceptibility Mapping of Karakorum Highway Combined with the Application of SBAS-InSAR Technology. Sensors 19 (12), 2685. doi:10.3390/s19122685
Keywords: landslide susceptibility mapping, SBAS-InSAR, FR-RF, Lancang River, Tibetan Plateau
Citation: Zhang J, Gao B, Huang H, Chen L, Li Y and Yang D (2022) SBAS-InSAR-Based Landslide Susceptibility Mapping Along the North Lancang River, Tibetan Plateau. Front. Earth Sci. 10:901889. doi: 10.3389/feart.2022.901889
Received: 22 March 2022; Accepted: 13 June 2022;
Published: 04 July 2022.
Edited by:
Marcel Hürlimann, Universitat Politecnica de Catalunya, SpainReviewed by:
Jianqi Zhuang, Chang'an University, ChinaChong Xu, Ministry of Emergency Management, China
Copyright © 2022 Zhang, Gao, Huang, Chen, Li and Yang. This is an open-access article distributed under the terms of the Creative Commons Attribution License (CC BY). The use, distribution or reproduction in other forums is permitted, provided the original author(s) and the copyright owner(s) are credited and that the original publication in this journal is cited, in accordance with accepted academic practice. No use, distribution or reproduction is permitted which does not comply with these terms.
*Correspondence: Jiajia Zhang, amltamlhMjAwOEAxNjMuY29t